FREQUENCIES IN CHROMOSOMAL ABERRATION IN HUMAN HEPATOCELLULAR CARCINOMAa
Bioinformatic analysis of CGH data was recently used to develop a progression model for human HCC.11 Based on an evolutionary tree constructed from statistically significant CGH events, three subgroups of patients with different patterns of HCC progression were identified. The subgroups reflect the extent of tumor progression as indicated by the number of chromosomal aberrations, tumor stage, tumor size, and disease outcome. Gains of 1q21-23 and 8q22-24 appear to be early genomic events in development of HCC and gain of 3q22-24 a late genomic event, the latter associated with tumor recurrence and poor survival. The HCC progression model uncovered chromosomal imbalances associated with clinical pathologic characteristics of the disease and explained a significant part of the variations in clinical outcome among the HCC patients.11
These two studies illustrate the power of CGH analysis to identify the functional significance of genomic alterations in human HCC. Nevertheless, because CGH only analyzes genomic DNA, additional studies are required to measure and integrate data on global gene expression to confirm the roles of candidate genes. This can be accomplished by adapting the expression imbalance map method and array CGH analysis (reviewed in ref. 12). This approach was recently applied to human HCC.13 Using regional pattern recognition approaches, the authors discovered the most probable copy number-dependent regions and 50 potential driver genes (Table 18.2). At each step of the gene selection process, the functional relevance of the selected genes was evaluated by estimating the prognostic significance of the selected genes. Further validation using small interference RNA-mediated knockdown experiments showed proof-of-principle evidence for the potential driver roles of the genes in HCC progression (i.e., NCSTN and SCRIB). In addition, systemic prediction of drug responses implicated the association of the 50 genes with specific signaling molecules (mTOR, AMPK, and EGFR). It was concluded that the application of an unbiased and integrative analysis of multidimensional genomic data sets can effectively screen for potential driver genes and provides novel mechanistic and clinical insights into the pathobiology of HCC.
It seems inevitable that new and improved array designs for both CGH and gene expression and the advent of whole genome sequencing combined with better software for statistical analysis of the data will continue to emerge.
CLASSIFICATION AND PROGNOSTIC PREDICTION OF HEPATOCELLULAR CARCINOMA
The application of microarray technologies to characterize tumors on the basis of global gene expression has had a significant impact on both basic and clinical oncology.7 The goal of tumor microarray studies generally includes discovery of subsets of tumors (class discovery), which enables diagnostic classification (class comparison), prediction of clinical outcome (class prediction), and mechanistic analysis. Verification and validation of the primary results are essential for discovery of oncogenic pathways and identification of therapeutic targets (for technical details on microarray analysis see Chapter 2 and ref. 7). Analysis of global gene expression in selected tumors has successfully classified them into homogeneous groups and predicted the clinical outcome and survival (reviewed in ref. 7).
The goal of all staging systems is to separate patients into homogeneous prognostic groups to permit the selection of the most appropriate therapy for each group. Although much work has been devoted to establishment of prognostic models for HCC by using clinical information and pathologic classification,6 many issues still remain unresolved. For example, a staging system that reliably separates patients into homogeneous early and intermediate-to-advanced HCC groups with respect to prognosis does not exist. This is of particular importance because the natural course of early HCC is unknown, and the natural progression of intermediate and advanced HCC is known to be quite variable.6 Because the accuracy of imaging techniques is rapidly evolving and affording detection of early HCC,14 the inability to predict the prognosis of these early lesions poses a therapeutic dilemma. Although the accurate pathologic diagnosis of high-grade DNs and early HCC is currently difficult, it is likely that many HCC evolve from DN.15
A new insight on the transition of DN into early HCC was recently obtained.16 A gene expression profiling on cirrhotic (regenerative) and DNs as well as early HCC was performed, and 460 differentially expressed genes were detected between DN and early HCC. Functional analysis of the significant gene set identified the MYC oncogene as a plausible driver gene for malignant conversion of the dysplastic nodules. In addition, gene set enrichment analysis revealed global activation of the MYC up-regulated gene set in early HCC versus dysplasia. Presence of the MYC signature significantly correlated with increased expression of CSN5 gene as well as with higher overall transcription rate of genes located in the 8q chromosome region. Furthermore, a classifier constructed from MYC target genes could robustly discriminate early HCC from high- and low-grade DNs. Importantly, this study identified unique expression patterns associated with the transition of high-grade DNs into early HCC and demonstrated that activation of the MYC transcription signature is strongly associated with the malignant conversion of pre-neoplastic liver lesions.
Many studies on HCC gene expression profiling, as well as several reviews, have appeared during the last 5 years.17 Interpretation of molecular profiling studies of HCC poses more challenges than other human tumors, mainly because of the complex pathogenesis of this cancer.5 HCC arises in diverse settings ranging from infection with HBV or HCV, to chronic metabolic diseases as varied as diabetes, nonalcoholic fatty liver disease, and hemochromatosis. These different diseases represent complex assortments of genetic and epigenetic aberrations as well as altered molecular pathways.5,6 Nevertheless, because of its extraordinary power of resolution, global gene expression profiling currently offers the most appropriate technology to resolve the complex molecular pathogenesis of HCC. Indeed, gene expression profiling of HCC has already generated impressive data sets that represent a remarkable progress toward the elucidation of the molecular pathogenesis of HCC, and may ultimately improve diagnosis and outcome prognosis for HCC patients.17
A class discovery approach applying hierarchical clustering of gene expression data from human HCC18 revealed two subclasses of HCC that are strongly associated with survival of the patient. In this study, several independent prediction algorithms determined whether gene expression patterns could be used to predict survival. HCC patients were randomly divided into two equal groups, that is, a training set that was used to develop the HCC classifiers and a validation set that was used to evaluate the test. All the classifiers successfully separated patients with shorter survival from patients who survived longer. Gene expression patterns strongly associated with patient survival, and the reproducibility of these gene expression-based predictors, were robust. Moreover, application of a Cox regression model identified genes whose expression was highly correlated with the length of survival. Survival-associated genes could also be used for highly accurate subclass prediction without the application of sophisticated prediction models. The knowledge-based annotation of the survival genes provided insight into the underlying biological differences between the two subclasses of HCC.18 Among several biological groups of the survival genes, the cell-proliferation group was the best predictor of an unfavorable outcome of the disease. Expression of proliferating cell nuclear antigen, a typical cell-proliferation marker, and cell cycle regulators such as CDK4, CCNB1, CCNA2, and CKS2 was greater in the poor survival subclass (cluster A). Not surprisingly, many genes that were more highly expressed in the poor survival subclass had antiapoptotic functions. Higher expression of genes involved in ubiquitination and sumoylation also characterized the poor survival subclass. Enhanced activation of ubiquitin-dependent protein degradation may account for deregulation of cell cycle control and faster cell proliferation in the poor survival subclass. This study demonstrates that gene expression profiling can identify previously unrecognized, clinically relevant subclasses of HCC in a robust and reproducible manner.
TABLE 18.2
LIST OF 50 POTENTIAL DRIVER GENESa
Using a similar approach, Boyault et al.19 further refined the transcriptome classification of HCC. Unsupervised transcriptome analysis identified six robust subgroups of HCC (G1-G6) associated with clinical and genetic characteristics of the tumors. G1 tumors had low HBV copy number and overexpressed genes controlled by parental imprinting in fetal liver. G2 tumors included HCC infected with a high copy number of HBV and mutations in PIK3CA and TP53. Specific activation of the AKT pathway was detected in both groups. G3 tumors were characterized by TP53 mutations and overexpression of genes controlling the cell cycle. G4 was a heterogeneous subgroup of tumors that included both HCC and hepatocellular adenomas with TCF1 mutations. G5 and G6 tumors contained β-catenin mutations that activate the WNT pathway. These results emphasize the genetic diversity of human HCC and provide specific identifiers for classifying the tumors. Also, this new classification shows that WNT and AKT pathways are activated in about 50% of the tumors, suggesting attractive potential therapeutic targets. Most recently Hoshida et al.20 performed a meta-analysis of gene expression profiles in data sets from eight independent patient cohorts across the world. A total of 603 patients were analyzed, representing the major etiologies of HCC (hepatitis B and C) collected from Western and Eastern countries. Three robust HCC subclasses (termed S1, S2, and S3) were observed, each correlated with clinical parameters such as tumor size, extent of cellular differentiation, and serum alpha-fetoprotein levels. An analysis of the components of the signatures indicated that S1 reflected aberrant activation of the WNT signaling pathway, S2 was characterized by proliferation as well as MYC and AKT activation, and S3 was associated with hepatocyte differentiation. Functional studies indicated that the WNT pathway activation signature characteristic of S1 tumors was not simply the result of β-catenin mutation but rather was the result of transforming growth factor-β activation, thus representing a new mechanism of WNT pathway activation in HCC. These experiments establish the first attempt to develop consensus classification framework for HCC based on gene expression profiles and highlight the power of integrating multiple data sets to define a robust molecular taxonomy of the disease.
The transcriptomic analyses have also been used to address lineage heterogeneity of HCC. The two major adult liver cancers are HCC and cholangiocarcinoma (CC). The existence of combined hepatocellular-cholangiocarcinoma (CHC), a histopathologic intermediate form between HCC and CC, suggests phenotypic overlap between these tumors. Woo et al.21 applied an integrative oncogenomic approach to address the clinical and functional implications of the overlapping phenotype between these tumors. By performing gene expression profiling of human HCC, CHC, and CC, the authors identified a novel HCC subtype, that is, cholangiocarcinoma-like HCC (CLHCC), which expressed cholangiocarcinoma-like traits (CC signature). Similar to CC and CHC, CLHCC showed an aggressive phenotype with shorter recurrence-free and overall survival. In addition, CLHCC coexpressed embryonic stem cell-like expression traits (ES signature) suggesting its derivation from bipotent hepatic progenitor cells. By comparing the expression of CC signature with previous ES-like, hepatoblast-like, or proliferation-related traits, the authors demonstrated that the prognostic value of the CC signatures was independent of the expression of those signatures (Fig. 18.1). These data suggest that the acquisition of cholangiocarcinoma-like expression traits plays a critical role in the heterogeneous progression of HCC.
FIGURE 18.1 Comparison of the CC signature (cholangiocarcinoma-like traits) with ES signatures (embryonic stem cell-like expression traits). A: The enrichment of ES signatures and polycomb target gene sets in C1 and C2 classes in HCCcomp. The enrichment scores of ES signatures without proliferation signature (noprol; right bar). B: Bar plots for the enrichment scores of the CC_UP (top) and CC_DOWN (bottom) signatures and the CC_UP and CC_DOWN signatures subtracted by ES (noES), HB (noHB), or ES and HB (noESnoHB) signatures. C: The enrichment ES signatures in six independent data sets are shown. For each data set, the group enrichment in C1 and C2 tumors is indicated in the right bars (P <.05). D: Kaplan-Meier plots analyses for RFS (left) and OS (right) based on the expression status of CC and ES signatures in the integrated Laboratory of Experimental Carcinogenesis and Seoul National University data sets (n = 209). The CC+represents C1 tumors, and ES+ represents the tumors that express ES1 signature. RFS, recurrence-free survival; OS, overall survival. For details, see ref. 21. (From ref. 21, with permission.)
COMPARATIVE FUNCTIONAL GENOMICS
Despite the fact that transgenic and knockout mouse models have greatly enhanced the understanding of human cancers, in most cases we still rely on casual correlation between human and mouse models owing to lack of methods for direct comparisons.22 Based on the neutral theory of molecular evolution, it is possible to identify both protein coding sequences and functional noncoding sequences in a genome.22 Because regulatory elements of evolutionarily related species are conserved, it is likely that gene expression signatures reflecting similar phenotypes in different species could be also conserved. Based on this hypothesis, cross-comparison of global gene expression data from human HCC and mouse models of HCC has been undertaken to identify aberrant phenotypes that reflect evolutionarily conserved molecular pathways. Lee et al.23 integrated gene expression data from human and transgenic mouse models of liver cancer. Hierarchical clustering analysis of integrated and standardized datasets revealed that HCC induced in mice by Myc, E2f1, and the combination of Myc/E2f1 transgenes had the highest similarity with those of the better survival group of human HCC. However, the expression patterns in HCC induced by Myc/Tgfa transgenes or by the chemical carcinogen diethylnitrosamine were most similar to those of the poor survival group of human HCC. The results suggest that these mouse models might recapitulate the molecular patterns of the two subclasses of human HCC. In contrast, gene expression patterns of HCC from Acox1-/- and ciprofibrate-treated mice, in which development of HCC was driven by peroxisome proliferation in the liver, were least similar to those observed in either subclass of human HCC. These results suggest that the molecular pathways underlying hepatocarcinogenesis induced by peroxisome proliferation in mice are not frequent in humans.23
The similarity of gene expression profiles between human and mouse models is in good agreement with the phenotypic characteristics of the tumors,23 that is, the human tumors with increased proliferation, decreased apoptosis, and worse prognosis pair with mouse models with the same features. The fact that these findings were first uncovered by unsupervised methods and later validated by supervised methods suggests that the underlying principles in gene expression changes are conserved between mouse and human HCC. These results strongly support the hypothesis that well-defined gene expression signatures from experimental conditions or animal models can be used to stratify human cancer patients into more homogeneous groups based on molecular similarity. The unique molecular identities of each subclass of HCC uncovered by comparative analysis of a genomewide survey of gene expression from human and animal models will facilitate the development of new therapeutic strategies.
Recent studies in different human tumors have provided further insights into how comparison of gene expression patterns from human and mouse tumors may permit the direct identification of common aberrant molecular pathways.24,25 Comparison of gene expression profiles from a Myc-driven mouse prostate cancer model and human prostate cancer enabled Ellwood-Yen et al.24 to identify a conserved expression module of human genes that corresponded to Myc signature genes defined in the mouse model. The Myc signature genes permitted the definition of a subset of MYC-like human cancers that are probably driven by MYC amplification or other mechanisms of MYC pathway activation. In a similar study, Sweet-Cordero et al.25 detected a KRAS2 gene expression signature by comparing a KRAS2-mediated mouse model of lung cancer with human lung cancer. It should be noted that when the investigators examined gene expression data from human lung adenocarcinomas alone, no statistically significant gene expression pattern correlated with KRAS2 mutation status between wild type and mutated KRAS2 tumors. The authors were able to identify a gene expression signature that reflected the KRAS2 mutation in human adenocarcinomas only by comparing gene expression in tumors from humans and mice.
Functional genomics has the potential power to identify the cell of origin of cancer and the cellular pathway by which cancer subsequently evolves. This notion was recently tested in HCC.26 Because HCC can originate from both adult hepatocytes and hepatic progenitor cells, our experimental strategy involved the integration of gene expression data generated from rat fetal hepatoblasts and adult hepatocytes with expression data from human HCC and mouse HCC models.26 Patients with HCC that shared a gene expression pattern with fetal hepatoblasts had a poor prognosis. The gene expression program that distinguished this subtype from other types of HCC included markers of hepatic oval cells, suggesting that this HCC subtype may arise from hepatic progenitor cells. Furthermore, analyses of gene networks in these tumors showed that activation of AP-1 transcription factors in this newly identified HCC subtype might have a key role in tumor development.
CONCLUSION AND PERSPECTIVE
DNA microarray technology has provided an extraordinary opportunity for integrative analysis of the cancer transcriptome. Array-based gene expression profiling not only has advanced our understanding of cancer biology, but has begun to influence decisions in clinical oncology and ultimately may allow for the development of more effective therapies. The power of gene expression profiling of HCC can be further enhanced by cross-comparison analysis of multiple gene expression data sets from human HCC and the rich database of HCC in animal models.22 The success of these new analytical approaches, comparative and/or integrative functional genomics, suggests that integration of independent data sets will enhance our ability to identify robust predictive markers. For example, a model to predict progression of mammary cancer has been recently developed based on a wound-healing gene expression signature.27 Patients displaying the wound-healing signature had a significantly increased risk of metastasis compared with those without the signature, indicating that genomic data from normal physiological conditions may help to predict the prognosis of cancer patients. It is important to obtain additional tumor-independent signatures from multiple species that are unique for different physiological conditions, such as liver development, regeneration, hepatic stem cell activation, that can be integrated with gene expression patterns from human HCC. Although the clinical application of gene expression profiling to identify prognostic markers and therapeutic targets for HCC is still immature, the progress over the last few years suggest that prospective clinical studies are well justified and indeed needed.
It seems reasonable to predict that the genomic technologies will play an increasingly important role in clinical oncology. The immediate focus undoubtedly will be on using the current genomic technologies to improve the diagnosis and treatment of cancer. However, with the beginning of affordable whole genome sequencing and the current expansion of the cancer genome atlas,28 one can certainly expect significant progress in the treatment of liver cancer.
References
1. Parkin DM, Bray F, Ferlay J, Pisani P. Global cancer statistics, 2002. CA Cancer J Clin 2005;55:74.
2. El Serag HB. Hepatocellular carcinoma: recent trends in the United States. Gastroenterology 2004,127: S27.
3. El-Serag HB, Hampel H, Javadi F. The association between diabetes and hepatocellular carcinoma: a systematic review of epidemiologic evidence. Clin Gastroenterol Hepatol 2006;4(3):369.
4. Libbrecht L, Desmet V, Roskams T. Preneoplastic lesions in human hepatocarcinogenesis. Liver Int 2005;25(1):16.
5. Thorgeirsson SS, Grisham JW. Molecular pathogenesis of human hepatocellular carcinoma. Nat Genet 2002;31:339.
6. Bruix J, Boix L, Sala M, Llovet JM. Focus on hepatocellular carcinoma. Cancer Cell 2004;5:215.
7. Quackenbush J. Microarray analysis and tumor classification. N Engl J Med 2006;354(23):2463.
8. Hood L, Heath JR, Phelps ME, Lin B. Systems biology and new technologies enable predictive and preventative medicine. Science 2004;306:640.
9. Pinkel D, Albertson DG. Array comparative genomic hybridization and its applications in cancer. Nat Genet 2005;37(Suppl):S11.
10. Moinzadeh P, Breuhahn K, Stutzer H, Schrmacher P. Chromosome alterations in human hepatocellular carcinomas correlate with aetiology and histological grade—results of an explorative CGH meta-analysis. Br J Cancer 2005;14:92:935.
11. Poon TCW, Wong N, Lai PBS, et al. A tumor progression model for hepatocellular carcinoma: bioinformatics analysis of genomic data. Gastroenterology 2006;131:1262.
12. Davies JJ, Wilson IM, Lam WL. Array CGH technologies and their applications to cancer genomes. Chromosome Res 2005;13: 237.
13. Woo HG, Park ES, Lee JS, et al. Identification of potential driver genes in human liver carcinoma by genomewide screening. Cancer Res 2009; 69(9):4059
14. Kim CK, Lim JH, Lee WJ. Detection of hepatocellular carcinomas and dysplastic nodules in cirrhotic liver: accuracy of ultrasonography in transplant patients. J Ultrasound Med 2001;20:99.
15. Kojiro M, Roskams T. Early hepatocellular carcinoma and dysplastic nodules. Semin Liver Dis 2005;25:133.
16. Kaposi-Novak P, Libbrecht L, Woo HG, et al. Central role of c-Myc during malignant conversion in human hepatocarcinogenesis. Cancer Res 2009;69 (7):2775.
17. Thorgeirsson SS, Lee JS, Grisham JW. Molecular prognostication of liver cancer: end of the beginning. J Hepatol 2006;44(4):798.
18. Lee JS, Chu IS, Heo J, et al. Classification and prediction of survival in hepatocellular carcinoma by gene expression profiling. Hepatology 2004;40:667.
19. Boyault S, Rickman DS, de Reynies A, et al. Transcriptome classification of HCC is related to gene alterations and to new therapeutic targets. Hepatology 2007;45(1):42.
20. Hoshida Y, Nijman SM, Kobayashi M, et al. Integrative transcriptome analysis reveals common molecular subclasses of human hepatocellular carcinoma. Cancer Res 2009;69(18):7385.
21. Woo HG, Lee JH, Yoon JH, et al. Identification of a cholangiocarcinoma-like gene expression trait in hepatocellular carcinoma. Cancer Res 2010;70(8):3034.
22. Lee JS, Thorgeirsson SS. Comparative and integrative functional genomics of HCC. Oncogene 2006;25(27):3801.
23. Lee JS, Chu IS, Mikaelyan A, et al. Application of comparative functional genomics to identify best-fit mouse models to study human cancer. Nat Genet 2004;36(12):1306.
24. Ellwood-Yen K, Graeber TG, Wongvipat J, et al. Myc-driven murine prostate cancer shares molecular features with human prostate tumors. Cancer Cell 2003;4:223.
25. Sweet-Cordero A, Mukherjee S, Subramanian A, et al. An oncogenic KRAS2 expression signature identified by cross-species gene-expression analysis. Nat Genet 2005; 37:48.
26. Lee JS, Heo J, Libbrecht L, et al. A novel prognostic subtype of human hepatocellular carcinoma derived from hepatic progenitor cells. Nat Med 2006;12(4):410.
27. Chang HY, Nuyten DS, Sneddon JB, et al. Robustness, scalability, and integration of a wound-response gene expression signature in predicting breast cancer survival. Proc Natl Acad Sci U S A 2005;102:3738.
28. International Cancer Genome Consortium. International network of cancer genome projects. Nature 2010;464(7291):993.
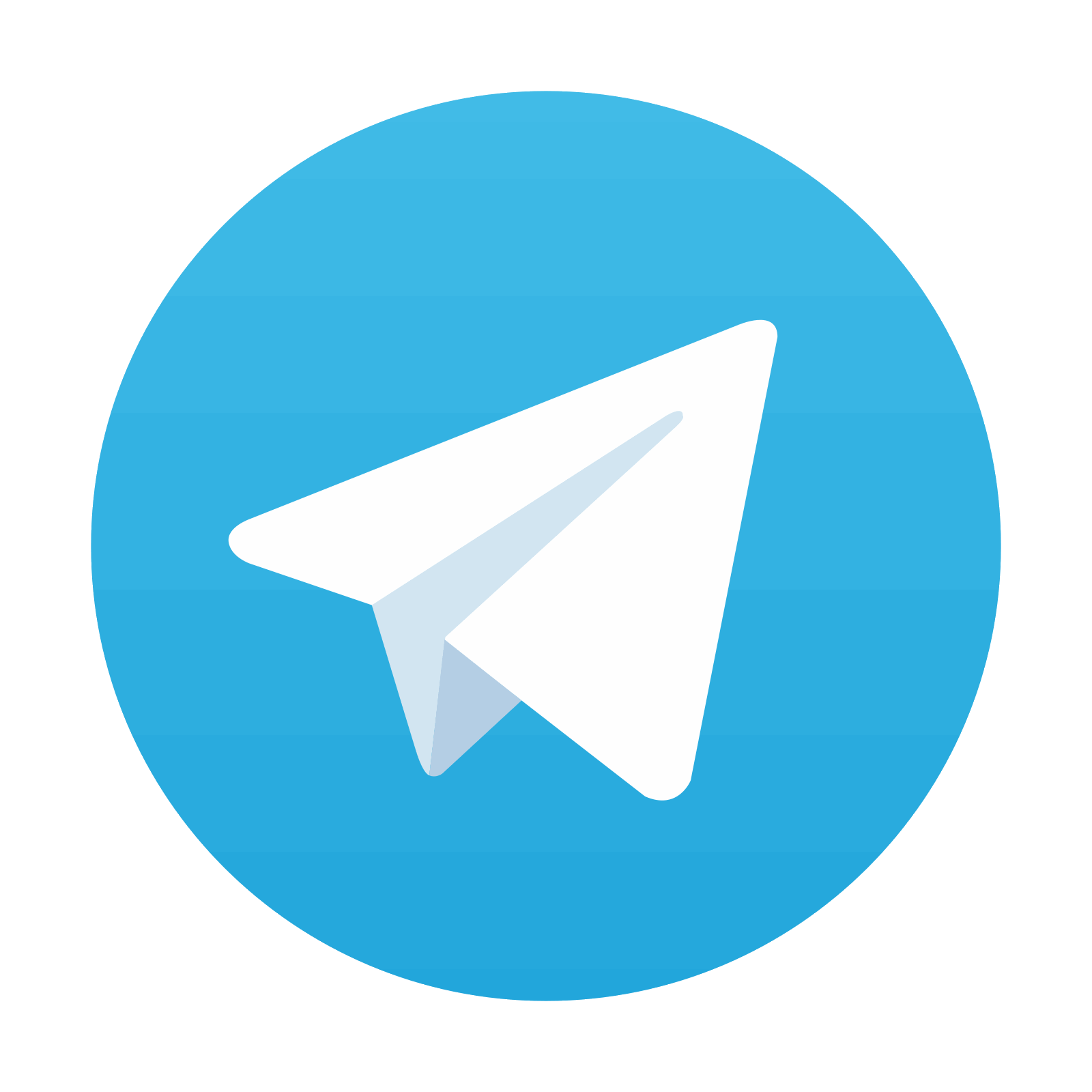
Stay updated, free articles. Join our Telegram channel

Full access? Get Clinical Tree
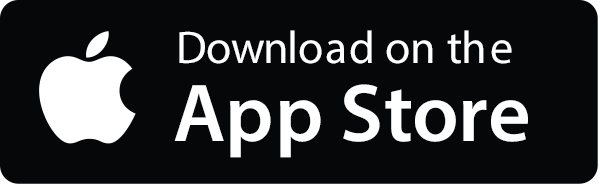
