Fig. 5.1
John Snow Pub, Broadwick Road, London, UK
The scope of epidemiology is much broader than the study of epidemics; it also involves the study of causal factors for all kinds of diseases, including both acute and chronic disease states. In cancer, epidemiology has had major public health impacts in defining causal associations between smoking and second hand smoke with lung cancer, hepatitis B infections and hepatocellular carcinoma and exposure to aromatic amines and bladder cancer.
To get a better understanding of epidemiology it is important to clarify some of the terminology and methods commonly used.
5.2 Measures of Disease Occurrence
To study the occurrence of a disease, the frequency and distribution of the events of interest must be measured. Prevalence describes the number of people living with a defined disease state at a certain point in time, whereas incidence describes how many people were diagnosed with a defined disease state during a specified time. For example, it is estimated that worldwide in 2012 there were 952,000 people living with stomach cancer [2]. One can also define how many people are still living with a defined outcome five years post the development of this outcome. Worldwide, the five-year prevalence of stomach cancer is estimated at 1,548,000 in 2012. In addition, the incidence of stomach cancer was estimated to be about 18 per 100,000 men per year in 2012 worldwide, whereas this was about 7 per 100,000 per year for women [2]. These incidences are reported as age-standardised rates, which allow comparisons of the incidences between different countries or geographical areas independent of the different age distribution in the areas.
Mortality rates are estimated as the number of people who died of a defined outcome during a specified time in a population. For instance, worldwide 13 per 100,000 men and 7 per 100,000 women died of stomach cancer in 2012 [2]. One key attribute of mortality rates is that the denominator is the number of individuals in the population, rather than the number of individuals diagnosed with the disease.
Another central measure in epidemiology is risk, or a person’s probability of developing a health state during a certain time period. Risk is defined as a probability between zero and one (often expressed as a percentage).
5.3 Exposure and Outcome
In addition to information about the occurrence of disease, information about measurement of exposures is needed to study different disease patterns. An exposure denotes a factor that one wants to study in relation to the incidence or the risk of an outcome. For instance, in the case of the cholera epidemic, Snow mapped cholera deaths to the distribution of water from different pumps and concluded that infected water, the exposure, was causing cholera, the outcome. Smoking as an exposure or risk factor for developing bladder cancer is another example and would refer to the etiology of bladder cancer.
The distinction between causation and association is imperative in epidemiology. Using different study designs, measurements and biostatistical methods, epidemiology can strictly only study the associations between exposures and outcomes. As can be seen from the examples above, however, we often aim conceptually at a study of cause and effect. David Savitz, in his book “Interpreting Epidemiologic Evidence”, explains this as follows: “In our epidemiological studies we can in principle only look at statistical associations between exposure and outcome, but by interpreting evidence given the pros and cons in a given study, we can make an interpretation of whether what we have studied is causal” [3]. Detailed descriptions of epidemiological statistical methods can be found elsewhere [4].
Austin B. Hill proposed in 1965 a set of criteria to help assess causation in biomedical research [5]. Apart from strength and consistency of the statistical association, he suggested that specificity in terms of outcome, temporality (exposure before outcome), biological gradient, theoretical plausibility, coherence between observational and experimental evidence and analogy also play a role in judging whether an exposure is causing an outcome. All these criteria may help in interpreting evidence for causality, but none of them (except that the exposure must precede the effect) are a formal test of causation.
Kenneth Rothman has provided another useful framework for reasoning about causation by introducing the concept of sufficient and component causes [6]. This concept aims to take into account the complexity of a chain of events and their potential interactions in study design as well as interpretation. For instance, to understand why some chain-smokers never get lung cancer and others are at high risk even after only a few years of cigarette smoking, we can move away from measuring just the average risk in smokers by looking at multiple putative causative factors of those exposed to get a better understanding of the different causative components in developing lung cancer.
5.4 Measures of Association
Measuring the association between an exposure and an outcome requires an estimate of the difference of disease occurrence between exposed and non-exposed study subjects. Ratios, i.e. an incidence rate ratio or a risk ratio, belong to the family of relative risks (RR), while the differences between incidences or risks are referred to as absolute differences. The relative risk is a measure of the strength of the association between the exposure and outcome, and thus important when trying to understand the aetiology of a disease. However, when the baseline risk is low, even a seemingly important relative risk for instance 2 (doubling of risk) or 0.5 (halving of risk) may have limited clinical or public health impacts, when we look at the absolute difference. To get the full picture of an association between an exposure and an outcome, both absolute and relative measures are needed. For instance, in a study of radical prostatectomy compared to watchful waiting [7], radical prostatectomy reduced the relative risk of dying from prostate cancer by 38 % (RR = 0.62). However, the absolute risk difference as measured at 15 years was reduced from 21–15 %, an absolute difference of 6 %. In this example, one can see that the interpretation of the strength of the association may differ when looking at the relative versus absolute differences. Another key epidemiological concept is the Number Needed to Treat (NNT) to avoid one event. This can be calculated by taking 1/absolute difference. In the above example, this would be estimated as 1/0.06 = 15 treated to avert 1 prostate cancer death.
5.5 Study Population
A research question about cause and effect can thus be addressed with an investigation of an association between an exposure and an outcome. In doing so it is essential to ascertain the group of persons in which we can quantify this association. In the typical epidemiological study we need some follow-up time for the outcomes of interest to develop. Hence, the quantification of the above-mentioned incidences and risks relies on using the observed person-time in our studied individuals. It is common to refer to this defined group of people as the study population and the total person-year experience as the study base. To enumerate the total person-time in the study base it is thus necessary to clearly define the time at risk that each person contributes to a study. In a study of prognosis in breast cancer, the study population could be women diagnosed with invasive breast cancer in a certain institution, person-time could be time from breast cancer diagnosis until breast cancer death, death from other causes or end of study, whichever comes first.
The term target population refers to the target for our findings to be generalised to. Generalisability relates to the validity of a study by relating the people in the study population to people outside the study population. For instance, a study based on men with prostate cancer diagnosed between 2008 and 2013 at Guy’s Hospital in London (study population) to identify the effect of ethnicity (exposure) on prostate cancer-specific death (outcome) aims to be generalisable for men with prostate cancer in the UK (target population).
5.6 Study Design
There is a wide variety of study designs available in epidemiology. For a detailed description of each study design we refer to Modern Epidemiology by Rothman et al. [8]. A brief description of the most common study designs is provided below.
Study designs can be classified as prospective and retrospective. If the researcher has collected information about the exposure before the outcome event, a study is considered to be prospective. In contrast, if information about the exposure is collected after the outcome, the study is retrospective. Thus, this classification refers to time of information collection.
5.6.1 Experimental Studies
In an experimental study investigators manipulate the conditions under study, i.e. they assign the study subjects to the exposure, most often a medical intervention. However, it is often very expensive to conduct a large experimental study and sometimes it is ethically not possible to allow for the investigator to assign people to different exposures (i.e. smoking or histopathological tumour type), so that observational or non-experimental studies are required.
A randomised clinical trial (RCT) is the gold standard of experimental studies. It studies the effect of an intervention applied by the investigator to two or more groups that are built up by random assignment. The major advantage of a trial over an observational study is its ability to circumvent selection bias, which is a major threat to the validity of observational studies (see below). By randomising participants to the intervention or the control arm, it is assumed that all baseline characteristics of the study population (i.e. demographics, medical history) are distributed similarly between both arms.
Another advantage with experimental studies is the possibility to design and follow a strict protocol, not only for the assignment and conduct of the intervention, but also for the information collection. Randomisation helps preventing selection bias, but there is no guarantee against information bias (see below). To ensure that information about treatment effects is obtained as objective as possible, studies are often designed as double-blinded, i.e. neither the investigators nor the participants know who was given the treatment and who was not. Thus, blinding refers to whether the participant, treating physician or researchers have knowledge about randomisation to the intervention arm. However, sometimes blinding is only possible in one-way (single-blinded) or even impossible due to the exposure being studied (i.e., new surgical techniques).
In the context of testing new therapies, experimental studies are often subdivided into phase I, II, III and IV studies. This categorisation depends on the stage of testing. Once preclinical or experimental studies have been conducted, a phase I study is performed to test the safety of the therapy in a few ‘unblinded’ volunteers. When the phase I study has shown safe results, a phase II study is conducted to test tolerability and define intensity of dose of the intervention. A phase III study is a much larger RCT to test the effect of the new therapy on the specified clinical outcome. Following a successful outcome of a phase III study, a new therapy may get approved by the relevant agencies (i.e. the Food and Drug Administration in the United States). However, the approval still requires follow-up with a phase IV study to identify the rate of serious adverse effects over a longer follow-up time. This study is either performed as an RCT or as an observational study.
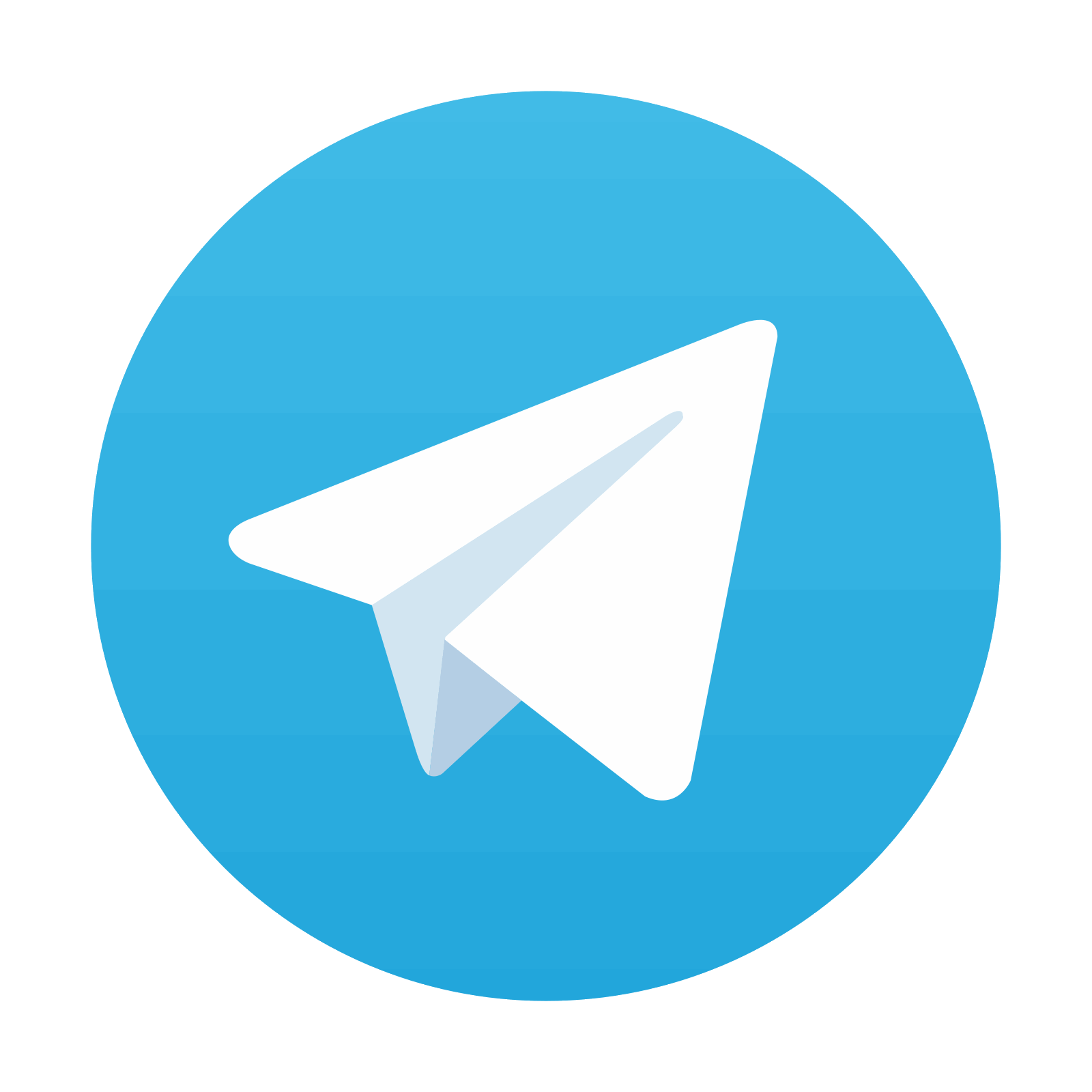
Stay updated, free articles. Join our Telegram channel

Full access? Get Clinical Tree
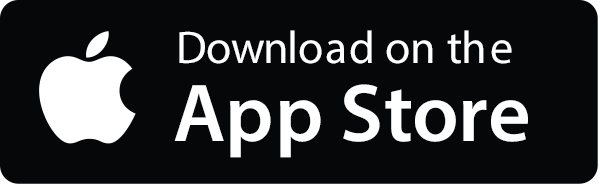
