Fig. 25.1
(a) Manual and automated identification of antigen-specific MHC class I multimer-positive CD8+ T lymphocytes among PBMC of a HLA-A2+ healthy donor. Top panel shows a manual gating strategy to identify CD8+ T cells specific for three HLA-A*0201-restricted epitopes derived from a EBV, influenza, and CMV viruses. From left to right, the plots show gates to exclude artifacts due to flow stream bubbles or clumps (count/time), find singlets (FSC-A/FSC-H), exclude nonviable cells (FSC-A/Aqua LiveDead), identify lymphocytes (FSC-A/SSC-A), exclude B lymphocytes (CD8/CD19), and quantify CD8+ T cells binding to EBV BRFL1 peptide-MHC multimers (CD8/PE), influenza matrix peptide-MHC multimers (CD8/APC), and CMV pp65 peptide-MHC multimers (QDot605). Bottom panel shows the corresponding peptide-MHC binding CD8+ T cells identified using an automated analysis approach that fitted a Dirichlet Process Gaussian Mixture Model with 256 components to the data [103]. Essentially identical frequencies of peptide-MHC multimer positive cells are found with manual and automated analyses. (b) Manual and automated analysis of antigen-specific T cells among PBMC of a second HLA-A2+ healthy donor tested in an intracellular cytokine staining (ICS) assay after incubation with a synthetic peptide corresponding to an HLA-A*0201-restricted epitope of pp65 CMV. Manual analysis finds cells positive for IFN and TNF, and a few events positive for IL-2. Without further gating, it is not possible to tell if the IFN- and TNF-positive events come from two separate or a single bifunctional population. Automated analysis reveals that there is indeed a single-cell population positive for IFN and TNF, with no evidence for an IL-2-positive population. Again, the frequencies of antigen-specific events identified by expert gating and automated analysis are almost equivalent
Finally, we note that most of these automated analysis tools are developed under open source licenses and so free to use without restriction. Some packages require a modicum of programming ability to use effectively (e.g., R or Python scripting skills) and others are available online, but in general, these algorithms are probably not easily used by the average flow operator in a clinical research laboratory. In the coming years, we expect that these automated analysis tools will become increasingly accessible to the average flow operator with the following developments – developers of these tools will continue to improve their ease of use; the most successful algorithms will be incorporated into commercial software analysis packages; and more workshops will be organized to train people in the use and potential pitfalls of these exciting new technologies.
25.8 New Methods and Technologies
Flow cytometry has played an instrumental role in our comprehension of the immune system and its interplay with human tumors. The technique has recently experienced dramatic advances and the methods and technologies are evolving continuously. Due to space limitations, we focus here on the recent innovations that in our opinion have the potential to transform the field of general cytometry and are directly relevant for cancer immunotherapy.
Since the first description of a tumor Ag targeted by human T cells [114], many tumor-associated proteins and HLA-class I- and class II-restricted epitopes have been identified. However, the antitumor T-cell immune response as a whole, i.e., the repertoire of Ag specificities recognized by T cells of individual patients, has only rarely been dissected [115, 116]. This is indeed a difficult task, due to the inherent complexity of such projects (many Ags and HLA-allele restrictions have to be taken into account), along with the limited amount of patient material generally available, and high requirements in terms of cost and time. Two groups simultaneously described a combinatorial encoding method which is a very elegant way to circumvent most of these hurdles [117, 118]. The technique is based on the combination of many HLA-peptide multimers, whereby a single multimer is coupled to several (two or three) fluorochromes, generating a color code for each tested TCR specificity. Currently, up to 27 HLA-multimers labeled with eight fluorochromes can be combined in routine analysis [117]. Coupled to the production of HLA-monomers by the UV exchange technology, this high-throughput method represents an important technical achievement for the T-cell immunology field and has started to deliver precious information by dissecting the anti-melanoma TIL repertoire in melanoma patients [119, 120]. Combinatorial staining could easily be implemented for monitoring vaccination trials, for example, when applying cocktails of antigenic peptides for which many specificities need to be tested in a single PBMC sample.
The combination of extracellular phenotyping with determination of intracellular changes in phosphorylation patterns upon stimulation is starting to provide new insights into signaling pathways in healthy and disease conditions [121, 122]. The binding of cytokines to their specific cell surface receptors generally results in the activation (i.e., phosphorylation) of the downstream signal transducers and activators of transcription (STATs), which in turn regulate the expression of many genes involved in cell growth, survival, differentiation, and polarization. Next to cytokines, the effect of unspecific mitogenic stimuli such as phorbol myristate acetate (PMA), phytohemagglutinin (PHA), or MHC-peptide complexes binding to the T-cell receptor (TCR) can be studied by measuring the level of other key signaling molecules such as phosphorylated (p)-Erk, p-S6, and p-NF-ĸB in T and B cells, whereas Toll-like receptor (TLR) ligand-induced activation can be followed with p-Akt, p-Erk, and p-NF-ĸB in B cells and monocytes. The proof of principal for a “single-cell network profiling (SCNP) method” was obtained on healthy donors PBMCs [123]. In this initial study, age as well as race differences were observed, whereas intra-donor variability needs to be established by testing blood samples taken at different time points over time. As T-cell signaling defects have been described in cancer patients [124, 125], insights in the intracellular phosphorylation patterns of T cells, including during immunotherapy, may soon deliver precious information.
A fundamental advance in flow cytometry in recent years is an increase in the number of parameters that can be simultaneously evaluated on single cells. Access to an increasing number of reagents and fluorochromes including tandem conjugates, semiconductor nanocrystals (quantum dots or eFluors), and organic polymers (brilliant violet family) [126–128], together with the wide availability of sophisticated flow cytometers, is making polychromatic analysis mainstream.
However, spectral overlap ultimately limits the number of fluorochromes in a single panel to an upper bound of approximately 20, as described in Sect. 25.3. An exciting new technology that has the potential to greatly increase the number of measurable parameters is mass cytometry (CyTOF), which uses stable heavy metal ions tagged to Abs (or, e.g., MHC multimers) in place of fluorochromes. These isotope labels are detected by time-of-flight mass spectrometry after vaporization of the cell. Although isotope labels generally produce a signal of low intensity, they have a lower background and virtually no spillover, making the measurement of a much larger number of markers feasible.
Mass spectrometry has been reported to be qualitatively and quantitatively equivalent to flow cytometry, with the simultaneous analysis of more than 30 parameters being already possible [129]. However, this promising new technology has the current following limitations as compared to traditional flow cytometry: lower label sensitivity, substantial cell loss, low acquisition rate, and the impossibility to sort living cells. Nevertheless, this method has started to reveal the complexity of healthy hematopoietic cells and of CD8+ T lymphocytes subsets and will certainly mature to become an indispensable technique in cancer immunology and immunotherapy [129, 130].
25.9 Concluding Remarks
Flow cytometry is the prototypical multiparameter single-cell assay, with applications in cancer immunotherapy ranging from epitope screening to immune monitoring of clinical studies. Due to its ability to characterize complex immune phenotypes and flexibility in measuring multiple immune functions such as Ag binding, expression of activation and inhibitory markers, cytokine production, cytotoxicity, and proliferation, flow cytometry is indispensable in cancer immunology research. However, because of the complexity of the assay and the fragility of the sample, it is challenging to apply and maintain robustness, sensitivity, and reproducibility, especially across multiple laboratories. Factors to consider when using flow cytometry in clinical research include understanding the range of flow-based assays available, as well as best practices for instrument, reagent, sample, and data analysis.
In order to harmonize laboratory protocols, practices, and analysis strategies, flow cytometry proficiency testing programs have been organized to learn and raise awareness of best practices. We believe that participation in proficiency testing programs, along with other initiatives delivering protocols, assay guidelines and reporting frames, is critical for raising the standard of flow cytometry analysis and strongly recommend that all clinical research laboratories that perform immune monitoring for clinical trials join such programs.
Acknowledgments
CG, SW, MJP, SvB, CO, and CB are members of the steering committee of the CIMT Immunoguiding Program (CIP). The CIP and CC are supported by a grant of the Wallace Coulter Foundation (Miami, Florida). CG is supported by a grant of the Deutsche Forschungsgemeinschaft SFB685. CC is supported by grants to the Duke University Center for AIDS Research and EQAPOL program funded by NIH grant 5P30 AI064518 and NIH contract HHSN272201000045C, respectively. We thank S Heidu for excellent technical assistance.
References
1.
Zola H, Swart B, Banham A, Barry S, Beare A, Bensussan A, et al. CD molecules 2006 – human cell differentiation molecules. J Immunol Methods. 2007;319(1–2):1–5.PubMed
2.
Van Damme N, Baeten D, De Vos M, Demetter P, Elewaut D, Mielants H, et al. Chemical agents and enzymes used for the extraction of gut lymphocytes influence flow cytometric detection of T cell surface markers. J Immunol Methods. 2000;236(1–2):27–35.PubMed
3.
Donnenberg VS, Landreneau RJ, Pfeifer ME, Donnenberg AD. Flow cytometric determination of stem/progenitor content in epithelial tissues: an example from nonsmall lung cancer and normal lung. Cytom A. 2013;83(1):141–9.
4.
Walter S, Weinschenk T, Stenzl A, Zdrojowy R, Pluzanska A, Szczylik C, et al. Multipeptide immune response to cancer vaccine IMA901 after single-dose cyclophosphamide associates with longer patient survival. Nat Med. 2012;18:1254–61.PubMed
5.
Hamann D, Baars PA, Rep MH, Hooibrink B, Kerkhof-Garde SR, Klein MR, et al. Phenotypic and functional separation of memory and effector human CD8+ T cells. J Exp Med. 1997;186(9):1407–18.PubMedCentralPubMed
6.
Caruso A, Licenziati S, Corulli M, Canaris AD, De Francesco MA, Fiorentini S, et al. Flow cytometric analysis of activation markers on stimulated T cells and their correlation with cell proliferation. Cytometry. 1997;27(1):71–6.PubMed
7.
Chattopadhyay PK, Roederer M. Good cell, bad cell: flow cytometry reveals T-cell subsets important in HIV disease. Cytom A. 2010;77(7):614–22.
8.
Zielinski CE, Corti D, Mele F, Pinto D, Lanzavecchia A, Sallusto F. Dissecting the human immunologic memory for pathogens. Immunol Rev. 2011;240(1):40–51.PubMed
9.
de Vos van Steenwijk PJ, Heusinkveld M, Ramwadhdoebe TH, Lowik MJ, van der Hulst JM, Goedemans R, et al. An unexpectedly large polyclonal repertoire of HPV-specific T cells is poised for action in patients with cervical cancer. Cancer Res. 2010;70(7):2707–17.
10.
Romero P, Zippelius A, Kurth I, Pittet MJ, Touvrey C, Iancu EM, et al. Four functionally distinct populations of human effector-memory CD8+ T lymphocytes. J Immunol. 2007;178(7):4112–9.PubMed
11.
Gattinoni L, Lugli E, Ji Y, Pos Z, Paulos CM, Quigley MF, et al. A human memory T cell subset with stem cell-like properties. Nat Med. 2011;17(10):1290–7.PubMedCentralPubMed
12.
Sallusto F, Lenig D, Forster R, Lipp M, Lanzavecchia A. Two subsets of memory T lymphocytes with distinct homing potentials and effector functions. Nature. 1999;401(6754):708–12.PubMed
13.
Appay V, van Lier RA, Sallusto F, Roederer M. Phenotype and function of human T lymphocyte subsets: consensus and issues. Cytom A. 2008;73(11):975–83.
14.
Derhovanessian E, Maier AB, Hahnel K, Beck R, de Craen AJ, Slagboom EP, et al. Infection with cytomegalovirus but not herpes simplex virus induces the accumulation of late-differentiated CD4+ and CD8+ T-cells in humans. J Gen Virol. 2011;92(Pt 12):2746–56.PubMed
15.
Altman JD, Moss PA, Goulder PJ, Barouch DH, McHeyzer-Williams MG, Bell JI, et al. Phenotypic analysis of antigen-specific T lymphocytes. Science. 1996;274(5284):94–6.PubMed
16.
Melenhorst JJ, Scheinberg P, Chattopadhyay PK, Lissina A, Gostick E, Cole DK, et al. Detection of low avidity CD8(+) T cell populations with coreceptor-enhanced peptide-major histocompatibility complex class I tetramers. J Immunol Methods. 2008;338(1–2):31–9.PubMedCentralPubMed
17.
Chattopadhyay PK, Melenhorst JJ, Ladell K, Gostick E, Scheinberg P, Barrett AJ, et al. Techniques to improve the direct ex vivo detection of low frequency antigen-specific CD8+ T cells with peptide-major histocompatibility complex class I tetramers. Cytom A. 2008;73(11):1001–9.
18.
Wooldridge L, Lissina A, Cole DK, van den Berg HA, Price DA, Sewell AK. Tricks with tetramers: how to get the most from multimeric peptide-MHC. Immunology. 2009;126(2):147–64.PubMedCentralPubMed
19.
Slingluff Jr CL, Petroni GR, Olson WC, Smolkin ME, Ross MI, Haas NB, et al. Effect of granulocyte/macrophage colony-stimulating factor on circulating CD8+ and CD4+ T-cell responses to a multipeptide melanoma vaccine: outcome of a multicenter randomized trial. Clin Cancer Res. 2009;15(22):7036–44.PubMedCentralPubMed
20.
Speiser DE, Lienard D, Rufer N, Rubio-Godoy V, Rimoldi D, Lejeune F, et al. Rapid and strong human CD8+ T cell responses to vaccination with peptide, IFA, and CpG oligodeoxynucleotide 7909. J Clin Invest. 2005;115(3):739–46.PubMedCentralPubMed
21.
Coulie PG, Karanikas V, Colau D, Lurquin C, Landry C, Marchand M, et al. A monoclonal cytolytic T-lymphocyte response observed in a melanoma patient vaccinated with a tumor-specific antigenic peptide encoded by gene MAGE-3. Proc Natl Acad Sci U S A. 2001;98(18):10290–5.PubMedCentralPubMed
22.
Filipazzi P, Pilla L, Mariani L, Patuzzo R, Castelli C, Camisaschi C, et al. Limited induction of tumor cross-reactive T cells without a measurable clinical benefit in early melanoma patients vaccinated with human leukocyte antigen class I-modified peptides. Clin Cancer Res. 2012;18(23):6485–96.PubMed
23.
Appay V, Dunbar PR, Callan M, Klenerman P, Gillespie GM, Papagno L, et al. Memory CD8+ T cells vary in differentiation phenotype in different persistent virus infections. Nat Med. 2002;8(4):379–85.PubMed
24.
Baitsch L, Baumgaertner P, Devevre E, Raghav SK, Legat A, Barba L, et al. Exhaustion of tumor-specific CD8(+) T cells in metastases from melanoma patients. J Clin Invest. 2011;121(6):2350–60.PubMedCentralPubMed
25.
Davis C, Wu X, Li W, Fan H, Reddy M. Stability of immunophenotypic markers in fixed peripheral blood for extended analysis using flow cytometry. J Immunol Methods. 2011;363(2):158–65.PubMed
26.
Cecconi V, Moro M, Del Mare S, Dellabona P, Casorati G. Use of MHC class II tetramers to investigate CD4+ T cell responses: problems and solutions. Cytom A. 2008;73(11):1010–8.
27.
Widenmeyer M, Griesemann H, Stevanovic S, Feyerabend S, Klein R, Attig S, et al. Promiscuous survivin peptide induces robust CD4+ T-cell responses in the majority of vaccinated cancer patients. Int J Cancer. 2012;131(1):140–9.PubMed
28.
Welters MJ, Kenter GG, de Vos van Steenwijk PJ, Lowik MJ, Berends-van der Meer DM, Essahsah F, et al. Success or failure of vaccination for HPV16-positive vulvar lesions correlates with kinetics and phenotype of induced T-cell responses. Proc Natl Acad Sci U S A. 2010;107(26):11895–9.PubMedCentralPubMed
29.
Weide B, Zelba H, Derhovanessian E, Pflugfelder A, Eigentler TK, Di Giacomo AM, et al. Functional T cells targeting NY-ESO-1 or Melan-A are predictive for survival of patients with distant melanoma metastasis. J Clin Oncol. 2012;30(15):1835–41.PubMed
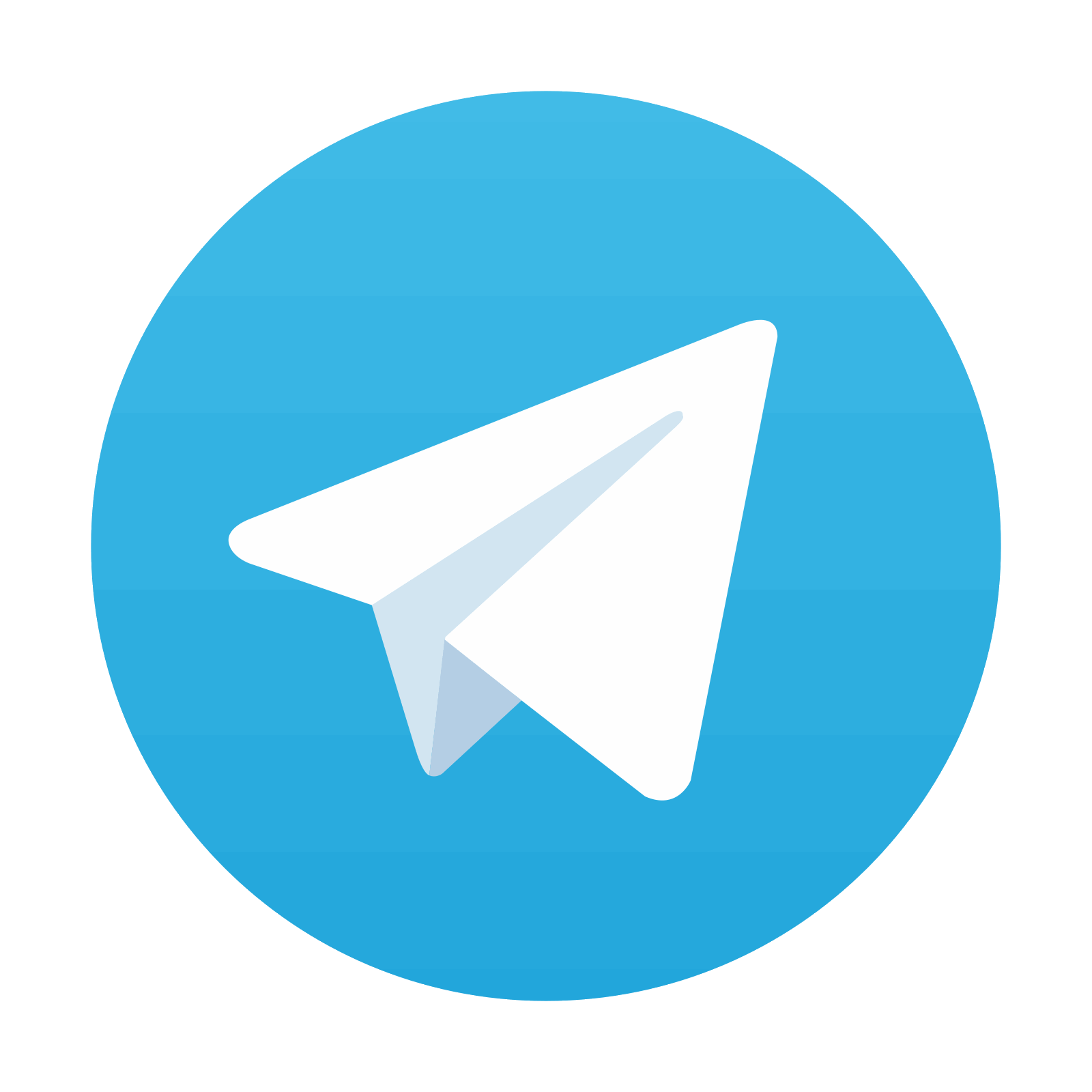
Stay updated, free articles. Join our Telegram channel

Full access? Get Clinical Tree
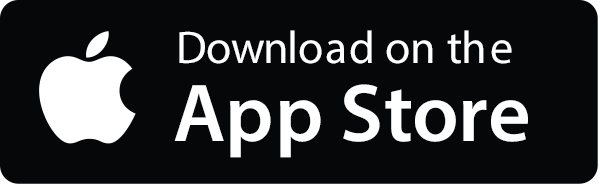
