INTRODUCTION
The Department of Biostatistics at the University of Texas MD Anderson Cancer Center works on developing innovative designs for clinical trials and biological experiments, analysis of complex data, and consulting and collaborating with clinical and biological investigators. Thousands of clinical trials are conducted at MD Anderson Cancer Center each year. Most of them are phase I/II trials, and a small number are phase III trials organized by biopharmaceutical companies. A large fraction of these phase I/II trials are initiated by MD Anderson investigators. Faculty members in the Department of Biostatistics are responsible for the statistical designs of these trials.
Although many statistical designs have been developed for phase I/II trials, most were not developed specifically for oncology clinical trials. Oncology trials usually have a small sample size due to the heterogeneity of patients, the large number of competing trials, and the fact that each particular subtype of cancer is a rare disease. Oncology trials need to consider multiple end points, such as tumor response, patient survival, and toxicity. To accommodate the special needs of oncology trials, the biostatisticians develop innovative adaptive designs to maximize the benefits of both patients participating in the trial and future patients, to make the most efficient use of patients as a valuable resource for competing trials, and to accelerate the drug development, discovery, and testing processes.
STATISTICAL RESEARCH ON CLINICAL TRIAL DESIGN
Faculty in the Department of Biostatistics advocate adaptive clinical trial designs (1,2,3,4,5,6,7) and other innovative early-phase designs (8,9,10,11,12,13,14,15). Some examples of adaptive designs are discussed next. We developed a statistical design for phase II clinical trials that better selects drug candidates for phase III trials (11). Currently, most phase II oncology trials use complete remission (CR) of the cancer as the primary end point. Drugs associated with higher CR rates are evaluated in subsequent phase III trials, which are usually required to demonstrate that the drug increases the patient’s survival time. Although achieving CR is necessary to prolong survival, it is not a sufficient measurement because patients may experience cancer relapse shortly after achieving CR. This discrepancy is one of the major reasons for the high failure rates (60%) of phase III trials (15). Thus, it is desirable to evaluate survival outcomes in phase II trials. Based on these considerations, our phase II design uses information on both CR and survival. There are several innovative features of this design. It makes full use of the information that accumulates in all stages of the trial and thus saves valuable patient resources. Interim stopping rules for toxicity, futility, and efficacy are defined. To evaluate the efficacy of treatments, patients are assigned to therapy in a randomized adaptive fashion. This means that patients in the trial tend to receive the more effective treatment, and the important aspects of the dose-response relationship are determined in an efficient manner. In particular, simulation studies show that the design has better operating characteristics than traditional trial designs.
The statistical aspects of this innovative design were published in Statistics in Medicine (11), and results of the actual trial were published in Leukemia & Lymphoma (16). Free computer software to implement this design is available on the website of the Department of Biostatistics for public use. Since the software was posted in 2009, it has been downloaded hundreds of times by people around the world, and the authors have replied to numerous e-mail inquiries. The URL for the software website is https://biostatistics.mdanderson.org/SoftwareDownload/.
We developed another statistical design to make the results of phase II or III clinical trials more reliable (6). In clinical trials with a relatively small sample size, patient characteristics among different treatment arms may not be well balanced. This may lead to an invalid inference. For trials that utilize response-adaptive randomization, this problem may be even more severe than for trials that use equal randomization. We developed a patient allocation scheme to adjust this imbalance during response-adaptive randomization. This design ensures that the observed differences between different treatments are real, rather than being due to an imbalance of patient characteristics between the different treatment groups. Because individuals with cancer are highly heterogeneous and oncology trials usually have relatively small sample sizes (compared with trials for some other diseases), clinical investigators have always had the concerns described. This design solves this important problem. The related research has been published in Statistics in Medicine (6).
Innovative statistical designs can help conduct clinical trials more ethically and more efficiently. For example, the Department of Leukemia at MD Anderson conducted a randomized phase II study of clofarabine alone versus clofarabine in combination with low-dose cytarabine (ara-c) in previously untreated patients who were 60 years of age or older and who had acute myeloid leukemia (AML) or high-risk myelodysplastic syndrome (MDS). The maximum sample size was set to be 108. The first 20 patients were equally randomized to the two treatment arms. After that, we designed an algorithm to assign more patients to the better-performing treatment arm. The trial would be stopped early if at any time the probability that one arm was superior was greater than or equal to 95%. Using this early stopping rule, the trial was stopped after 70 patients became evaluable for response. At that time, the treatment arm of clofarabine alone had 16 patients, 5 of whom (31%) had CR. The combination arm had 54 patients, 34 of whom (63%) had CR. By using this innovative statistical design, we not only reduced the sample size (from 108 to 70), but also assigned more patients (54 vs 16) to the treatment that showed better performance. These results were published in Blood (17). Many such efficient and ethical clinical trials have been conducted (18,19,20,21,22,23,24,25,26,27,28,29,30).
DATA ANALYSIS
Faculty in the Department of Biostatistics devote great effort into genomic research (31,32,33,34,35,36,37,38,39,40,41,42,43,44,45,46,47,48), imaging data analysis (49,50,51,52,53), survival analysis (54,55,56,57,58,59,60,61), biomarker data analysis (62,63), drug interactions (64), causal inference (65,66,67), epidemiology studies (68,69,70,71,72), and many other diverse topics. Next is a discussion of some issues in the analyses of disease recurrence, cancer screening, and biomarker studies.
Although curing cancer remains a challenge, medical advancements have successfully transitioned many types of cancer from a rapidly fatal disease to a chronic disease. After their initial treatments, patients may experience a few disease recurrences and receive different salvage treatments after each such recurrence. That is, cancer patients usually experience the following process:
This is a long and complicated process. For many forms of cancer, a number of therapeutic options are available at the initial and at subsequent salvage treatment stages. In these circumstances, instead of considering only the effect of a treatment on the time to the next disease recurrence, it is important to understand the long-term effects of each treatment on the overall survival time. Optimizing treatment decisions during this process can minimize treatment toxicity, reduce drug resistance, prolong the survival time, and enhance the patient’s quality of life.
The duration of disease-free survival is commonly used in medical research to compare different treatments. This method considers the time to disease recurrence or death, whichever happens first. Equating death with disease recurrence in this manner does not give sufficient penalty to treatments associated with high death rates. This is a serious problem when the lifetime after disease recurrence can be substantial. Consequently, disease-free survival is not the best basis for making treatment decisions.
A number of statistical methods have been developed to address the shortcomings of methods based on the end point of disease-free survival (73,74,75,76,77,78). We have been using frailty models to analyze recurrent events and a terminal event, such as death (73,74,75). We provide estimation of the effects on survival by treatment sequence (76). We provide new and easily implemented statistical approaches to optimize treatment sequences for recurrent diseases (77,78). The optimized treatment sequences are personalized; that is, treatment decisions depend on a patient’s previous response, current disease status, characteristics, and genetic biomarkers. These methods can be applied to data from randomized or nonrandomized studies.
The importance of dealing with the problem of informative censoring is well known in statistics, but it remains a challenge. We have developed a frailty model for informative censoring (79) and a test for informative censoring in clustered survival data (80). They can be used to test the presence of informative censoring, to estimate the degree of association between censoring and the risks affecting survival, and to estimate treatment effects while accounting for the informative censoring. This model can also be used to assess the correlation between different competing risks. We have developed a method for conducting sensitivity tests for survival analysis (81).
Screening for risk factors or early evidence of disease is important for cancer prevention. The distribution of the preclinical duration of cancer is unobservable, but knowledge of this distribution would be of great help in many situations. For example, such knowledge can help in making recommendations about optimal cancer-screening frequencies. We have developed a nonparametric method to estimate the preclinical duration distribution using data from a randomized early cancer detection trial (82). This estimation method is expected to have good practical use.
An important aspect of modern cancer research is the identification of molecular and genetic markers that predict an individual’s cancer risk and future response to a treatment and the validation of these identified markers. We have provided a method to use in building and validating a prognostic index for biomarker studies (83). This method is especially useful when there are many markers under consideration, which is currently true of typical biomarker trials because of the use of high-throughput arrays and other modern biomedical technologies.
The statistical analyses we have conducted for numerous biomarker studies are crucial for the translation of laboratory research results into clinical innovations, with a frequent goal of replacing toxic chemotherapies with safe and effective targeted therapies. A good example of targeted therapy is the use of tyrosine kinase inhibitors (TKIs, such as imatinib) to successfully control chronic myeloid leukemia (CML). Working with the Department of Leukemia, we found that CCL3 (MIP-1a) plasma levels were associated with the risk of disease progression in chronic lymphocytic leukemia (CLL) (84). Then, we helped design a phase I/II study to determine the effects of an Syk-JAK inhibitor on this biomarker. We also found that DNA methylation predicted survival and response to therapy in patients with MDS (28). Another finding was that the gene that produces the protein survivin was highly expressed in leukemic stem cells and predicted poor clinical outcomes in AML (85). These are examples of the biomarker discoveries to which we have contributed (86,87,88,89,90,91,92
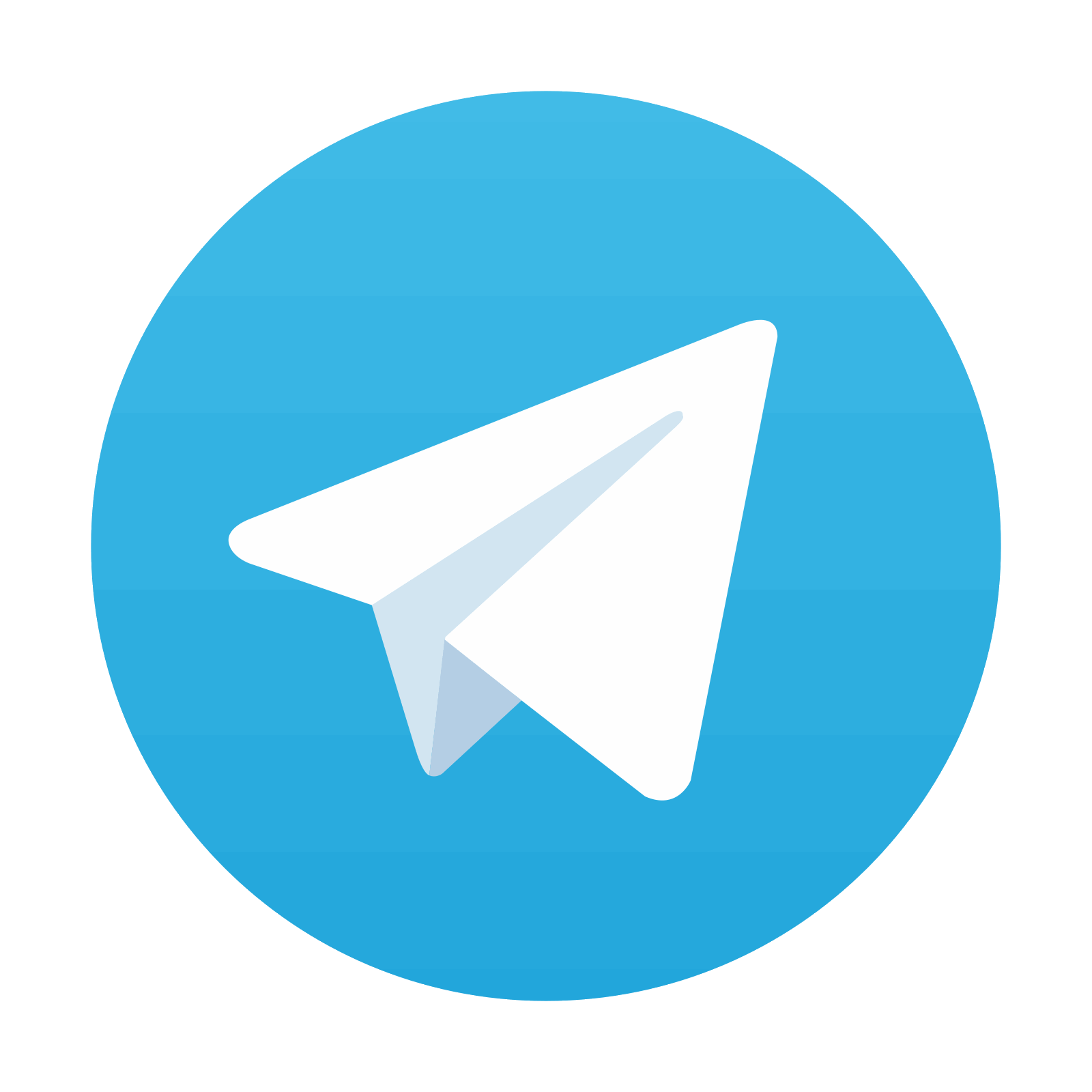
Stay updated, free articles. Join our Telegram channel

Full access? Get Clinical Tree
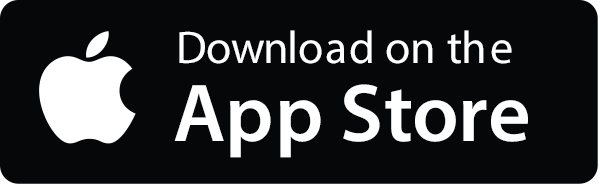

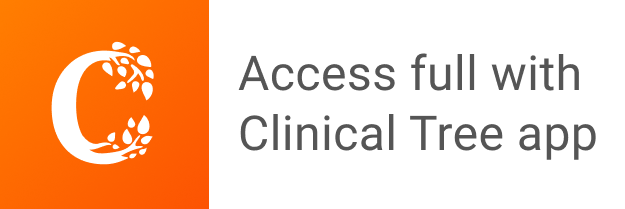