A vision for twenty-first century healthcare
Leroy Hood, MD, PhD Kristin Brogaard, PhD
Nathan D. Price, PhD
Overview
The convergence of system medicine, big data, and patient-activated social networks is leading to a medicine that is predictive, preventive, personalized, and participatory (P4). P4 medicine has two central thrusts—optimizing wellness and demystifying disease. To bring P4 medicine into the healthcare system, we initiated a longitudinal pilot study generating individual data clouds for 108 well people. The integration of the individual data clouds identified “actionable possibilities” that allow individuals to optimize wellness or avoid disease. We believe that this approach will transform healthcare by decreasing costs, increasing quality, and promoting innovation in a new healthcare sector—scientific wellness.
A vision for healthcare
Healthcare is one of the most significant challenges of our time. Costs are rapidly growing worldwide and are not delivering commensurate improvements in health.1 For example, even though the United States is the biggest healthcare spender in the world, it ranks near the bottom of the top 17 developed nations in survival among those 50 years of age and older.1, 2 Moreover, studies on the drivers of health and disease show that approximately 30% is attributable to genetic causes, 60% to environmental and behavior causes, and only about 10% to healthcare itself, with interactions among these factors.3 Thus, the focus of today’s healthcare—which is almost entirely on disease—ignores major aspects of the actual drivers of health. This focus is problematic because once biological systems are altered in disease, they often cannot be restored to their fully functional pre-disease state. Clearly, major systemic changes are needed to provide the healthcare we need for the twenty-first century.
Quantifying wellness and demystifying disease
To transform the current healthcare system, we suggest the adoption of two major thrusts—quantifying wellness and demystifying disease by studying its origins. Wellness is critical to both optimizing human potential (or capital) and providing fundamental insights into disease and disease prevention through its ability to examine the earliest wellness to disease transitions so as to study disease at the point of detectable inception. On the contrary, contemporary healthcare generally analyzes and treats patients only after they become sick.
The key to this transformation is to take a systems biology approach to disease, which leads naturally to systems medicine and its conviction that healthcare of the future must be predictive, preventive, personalized and participatory—P4 medicine. The fundamental question is how to apply P4 medicine to patients and introduce it into the contemporary healthcare landscape.
In this chapter, we outline a powerful new approach to quantifying wellness and minimizing disease that will serve as a model for transforming healthcare. It will be introduced through an extended, data intense, longitudinal study of 100,000 “well” people. This model will provide the basis for establishing quantitative metrics of wellness and for identifying the transition states from wellness to most common diseases.
The emergence of P4 medicine
As background, we provide a personal view of how this P4 vision of medicine has emerged over the past 40 years, and how its implementation will impact the healthcare system of the twenty-first century.
The early stages
Let us review the state of biomedical research five decades ago. When one of us (Lee Hood) began as an assistant professor at Caltech in 1970, it was obvious that biology and disease were far more complex than most researchers assumed at that time—and that studying biological systems one gene or one protein at a time was not likely to decipher this complexity.4 The well-known parable of the elephant and six blind men is apt: each man felt a different part of the elephant (trunk, leg, tusk, etc.) and characterized the elephant by that observation. So, to one person the elephant was like a snake (trunk) while to another it was like a fan (ear) and so forth. Understanding the elephant requires a holistic approach that integrates the data. The study of biology and medicine suffers from analogous complexities, where we measure one or a few genes or proteins and fail to see the whole picture. We need to integrate different global or comprehensive types of biological information to decipher disease complexity. The emergence of this new integrative discipline—now called systems science—has led to the transformation of biology and medicine.
What became obvious in the 1970s was that scientists lacked the approach (systems science), the technologies, and the systems-driven strategies to address biological complexity. During this period, I read Thomas Kuhn’s The Structure of Scientific Revolutions,5 which highlighted the difficulties in catalyzing paradigm changes in physics due to scientists’ conservatism and reluctance to move beyond conventional wisdom to think outside the box. It became clear to me that there needed to be paradigm shifts in the field of biology, to move beyond reductionist thinking to a broader systems approach. At that point I made a decision to dedicate my career to deciphering in various ways biological complexity. Most of these efforts were initially viewed with considerable skepticism—as predicted by Kuhn.
Facing these challenges, I participated in five paradigm changes over the next four decades that addressed biological complexity and led to the emergence of systems biology, systems medicine, and the concept of a medicine that was predictive, preventive, personalized, and participatory—P4 medicine.6, 7 These paradigm changes were as follows:
- 1. Bringing engineering to biology through the development of six instruments that allowed the reading and writing of DNA and proteins. These instruments introduced the concept of high-throughput biological data generation and its associated big data and analytics.8–10
- 2. One of these instruments, the automated DNA sequencer, enabled the Human Genome Project, which produced a complete list of genes, and by inference proteins—parts lists that made systems science possible.9, 11, 12
- 3. The emergence of cross-disciplinary biology—bringing together biologists, chemists, computer scientists, engineers, mathematicians, physicists, and physicians under one roof—learning one another’s languages and working together in effective teams to enable “big biology” efforts to solve very complex biological problems. This collaborative effort fueled the cycle of biological discovery driving technology that, in turn, drives analytics to understand the mechanisms of biology. Bill Gates played a critical role in catalyzing the shift to cross-disciplinary biology in 1992 by recruiting me to establish the Department of Molecular Biotechnology at the University of Washington Medical School, which pioneered key technologies and analytics in genomics, proteomics, cell sorting, and large-scale DNA synthesis.13 This department also produced two of the 16 centers involved in sequencing the human genome.
- 4. In 2000, I co-founded the Institute of Systems Biology to pioneer systems science and its necessary technologies and analytics.14, 15 This global systems approach was the key to addressing biological complexity. Its application to disease led to the fifth paradigm change.
- 5. Systems medicine and P4 medicine emerged from the application of systems science to disease.16–18 Thus, the first four paradigm changes led to a revolutionary new approach to study disease, wellness, and medicine.
In retrospect, these experiences generated the following useful insights about how to catalyze paradigm changes in modern society:
- New ideas need new organizational structures—each of these five changes required the creation of new organizational entities to serve as their champions. Longstanding bureaucratic cultures can only rarely adopt new ideas as they were honed by outdated needs and experiences of the past, and thus often have trouble adapting to the present, let alone the future.
- If you want to persuade organizations to adopt new ideas, you must engage the organization’s leadership. Middle-level managers often fail to understand the paradigm changes as they are mired in day-to-day operations.
- Each of these ideas was initially met with considerable skepticism. Determined optimism is essential for moving ideas forward in the face of widespread resistance.
- Each of these changes fundamentally altered how we viewed and practiced biology and medicine.
- It is essential to bring new ideas to the broader community of biological scientists, and ultimately to the public. At the Institute for Systems Biology (ISB), the Logan Center for Education is committed to bringing modern science to these audiences—and to promoting K-12 science education in this new context.
The book of life—four levels of biological information
These paradigm changes provided the conceptual framework and the tools to understand information in biology in a far more holistic manner than ever before—as four discrete levels of information—DNA, RNA, proteins, and biological networks or systems (Figure 1). They also demonstrated the need to be able to integrate and model biological information to address its complexity.
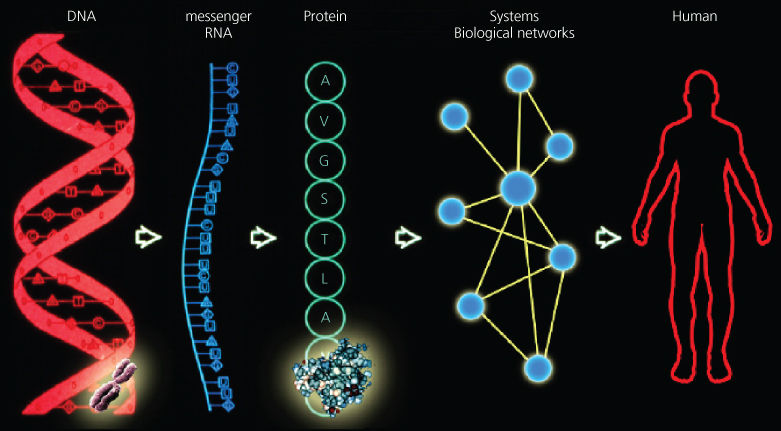
Figure 1 A schematic representation of the many different data types that are present in individual data clouds.
The genome can be described as a book containing the digital instructions for life.11 The genome is a four-letter language of DNA (G, C, A, T) and is packaged in 23 pairs of human chromosomes that are present in each of an individual’s 1013 cells. The human genome (diploid) contains about 6 billion letters or about 6 m of DNA. Since your body contains 1013 cells, it has sufficient DNA to reach from the earth to the sun 600 times. The genome is divided into about 20,000 units of information termed genes.
The genes can be read as digital four-letter copies (or transcripts) of the DNA language—these copies are termed RNA. The genes can be modified chemically or through their interactions with proteins, thus modifying their ability to be expressed. These are termed epigenetic modifications. The generation of RNA transcripts for individual genes allows for their differential amplification according to their use needs (up to millions of RNA copies per cell) and it allows the transcripts to be altered chemically to modify their information content. For example, we studied one human gene, neurexin, that had at least 2000 different RNA transcripts—and the fascinating question was how many of these unique transcripts represented different units of information (the others are presumably noise).19
Most RNA transcripts can be translated by a complex biological machine into proteins, which represent the third level of information. A 20-letter language corresponding to the 20 amino acids encodes proteins. This language directs the folding of each protein into a unique and complex three-dimensional structure that executes the specific functions of life. Proteins may function alone or through interactions with other proteins and/or informational molecules to create complex molecular machines or biological networks.
Networks, the fourth level of information beyond DNA, RNA, and proteins, arise from interactions between proteins and DNA, RNA, other proteins, and small molecules of life (metabolites) in the context of the various biological networks of life (e.g., gene regulatory networks, protein interaction networks, and metabolic networks). These networks are dynamical, changing over time, and are formed both by the information from their genes and the information from their environment. Thus networks encode the dynamics of life that occur via the integration of both genetic and environmental information. Biological networks mediate critical life processes including development, physiology, and aging. Perturbations in biological networks cause human disease.
Classical molecular biology involves the study of life, analyzing one or a few genes or proteins at a time. Systems biology involves analysis of the dynamics of networks in developmental processes, physiological responses, and aging. To facilitate systems analysis of these dynamical networks, it has been critical to begin developing tools that enable the sequence analysis of all genes in the genome; all gene modifications (epigenome); all RNAs (transcriptome); all the proteins in a given cell, organ, or creature (the proteome); and the metabolites present in a given cell, organ, or creature (the metabolome). These collectively are termed “omic” analyses. It is also important to measure at least some higher level physiological and psychological phenotypes.
Today, there are several approaches to understanding biology and medicine. For example, the term genomic medicine is very popular and it addresses the role that the genome plays in disease. In contrast, systems medicine (a systems approach to disease) takes a holistic and integrative approach to the dynamics of life and disease, which includes the genome but also the transcriptomes, epigenomes, proteomes, metabolomes and more complex phenotypes. Systems medicine focuses on understanding the roles that both the genome and the environmental changes play in wellness and disease, in part through a study of its dynamical biological networks.
Systems medicine: a tipping point
Systems medicine has two central features.
First, each individual will have a virtual, personalized, dynamical cloud of billions of data points (Figure 2). The data are heterogeneous in type and multiscale in dimension. They range from molecular, cellular, and organ data to social networks that link individuals together—and these data are dynamical in that they change with time and environmental perturbations. The ISB is developing the analytical tools to integrate and model these data to identify “actionable possibilities” that will enable each individual to respond to these opportunities in order to optimize their wellness and/or minimize the severity or chances of disease.
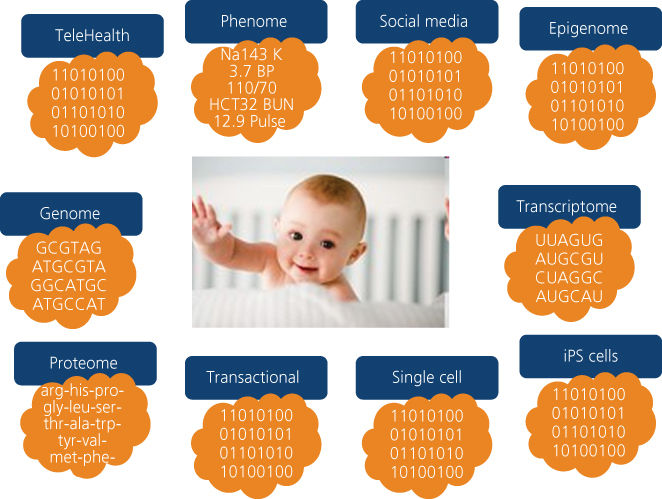
Figure 2 A schematic diagram of the “network of networks.” Biological networks can operate at the level of genes, of proteins and other molecules, of cells, of organs, and even of social networks of individuals. In disease, some of these networks are altered to become disease perturbed. The difference between normal and disease-perturbed networks provides insights into disease mechanisms, early diagnosis, and improved identification of drug target candidates.
These big-data clouds present an enormous signal to noise challenge stemming from the inherent variability (noise) in biological information that is irrelevant to the health questions at hand. Thus, systems approaches are critical to mining these large datasets for actionable information, identifying potentially small signals from amidst large amounts of noise.20
Second, dynamical biological networks manage, integrate, and transport biomolecules and associated information in living organisms, and these in turn mediate normal development and physiological responses. These networks become perturbed in disease,21, 22 and thus alter the information they process (Figure 3). Assessing the differences between normal and disease-perturbed networks provides deep insights into disease mechanisms, novel diagnostics approaches, and new therapeutic target candidates.
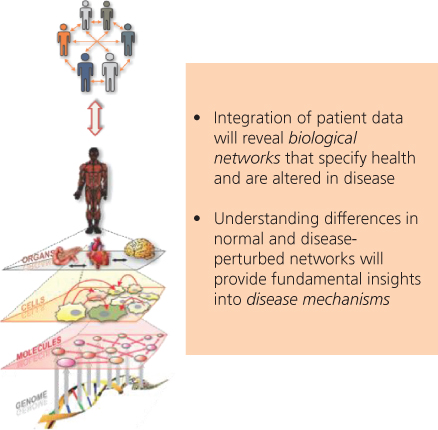
Figure 3 A schematic diagram of the four hierarchical levels of biological information—DNA to RNA to proteins to interactions, networks, and biological systems.
In 2008, ISB initiated a $100 million partnership with the state of Luxembourg. It provided $20 million annually to ISB for 5 years to develop the strategies and technologies necessary for the advancement of systems medicine. This funding was flexible, thus allowing the institute to pursue high-risk high-reward strategies. These efforts were extremely successful and led to the development of nine novel technologies and systems-driven strategies that accelerated the enablement of translational medicine, as demonstrated by the following three examples.
- First, we pioneered complete genome sequencing of entire families and this accelerated our ability to identify disease genes.23
Stay updated, free articles. Join our Telegram channel

Full access? Get Clinical Tree
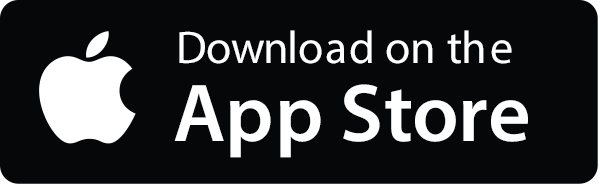
