Systems biology and genomics
Saima Hassan, MD, PhD, FRCSC Laura M. Heiser, PhD
Joe W. Gray, Ph.D.
Overview
Cancers are complex, adaptive systems comprised of cancer cells, the proximal and distal cells, and soluble and insoluble proteins that influence the behavior of the cancer cells. Features pertaining to the cancer are referred to as intrinsic to the cancer and those comprising the proximal and distal microenvironments are referred to as extrinsic to the cancer. Genomics studies seek to develop comprehensive cellular and molecular profiles of cancers and cancer systems biology studies seek to develop the experimental and theoretical methods needed to understand how the components work together to determine cancer function. The overall goal of these efforts is to develop the ability to predict the behavior of cancers—including progression and response to therapy—from measurements of the intrinsic and extrinsic molecular and cellular components of the cancers. Here, we review recent progress in international efforts to measure the genomic, epigenomic, and proteomic features (aka omic features) of major tumor types. We summarize work on establishing associations between omic features and cancer behavior. Finally, we summarize the computational and experimental models that are currently being used to understand and manipulate the behavior of complex cancer systems.
Introduction
Studies of the behavior of biological systems have traditionally been reductionist, focusing on specific genes or pathways. Today, efforts to generate molecular profiles of cancers are well established, momentum is building to establish associations between features and biomedical behavior, and efforts to establish mechanistic theoretical models are beginning. Systems biology approaches to cancer include studies of the intrinsic molecular features of cancer cells, as well as studies of how signals from the extrinsic microenvironment influence cancer cell signaling and phenotypic response (Figure 1).
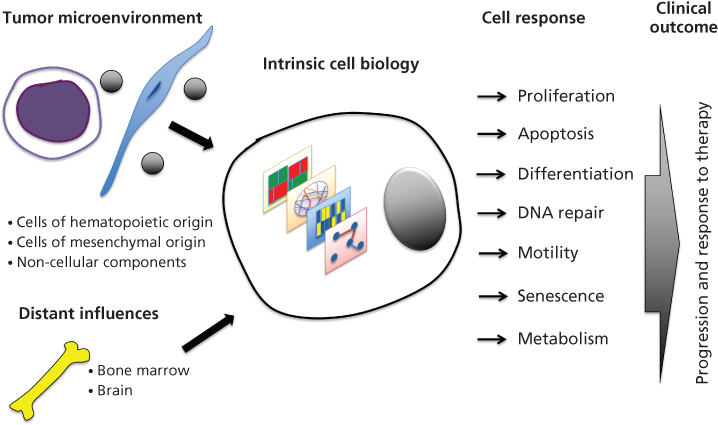
Figure 1 A schematic illustration of the influence of extrinsic and intrinsic systems biology upon cellular function with the potential to identify progression and response to therapy in patients. The extrinsic systems biology component consists of the tumor microenvironment including cells of hematopoetic origin, mesenchymal origin, and noncellular components, in addition to distant influences, from the bone marrow and other multiple organs. Together, with a systems biology approach, cellular function can be studied using various endpoints, including cellular proliferation, apoptosis, differentiation, DNA repair, motility, senescence, and metabolism. Our laboratory is striving to combine these endpoints to better understand tumor progression and response to therapy in the laboratory, and how this may correlate to patients in the clinic.
Intrinsic cancer systems biology, the study of malignant cells that comprise the tumor mass, has evolved with advancements in genomic and epigenomic profiling technologies. These technologies produce comprehensive measurements of the genomic aberrations, and transcriptional and proteomic levels that comprise individual tumors. Several recent studies have identified recurrent events that define cancer subsets, have led to a better understanding of cancer heterogeneity, have identified prognostic subgroups and predictors of response to therapy, and have facilitated discovery of novel therapeutic targets.1, 2
Extrinsic systems biology focuses on understanding external signals that alter the behavior of tumor cells. Extrinsic signals may come from microenvironments in close proximity to the tumor cells (e.g., invading immune cells, cancer-associated fibroblasts (CAFs), and vascular endothelial cells)3 as well as from distal organs, especially the brain.4, 5 Several recent observations point to the clinical importance of the tumor microenvironment (TME): stromal components can predict outcome,6 the TME can mediate therapeutic response and resistance,7 and the immune microenvironment has been shown to mediate tumorigenesis, disease progression,8 and therapeutic response.9–11
Together, intrinsic and extrinsic systems biology approaches strive to enable an all-encompassing understanding of the cancer cell and its interactions with its environments. This chapter will discuss both intrinsic and extrinsic systems biology. The intrinsic systems biology section will focus on the clinical impact of high-throughput genomics and the use of cancer cell lines as predictive models of therapeutic response in patients. The extrinsic systems biology section will discuss its different constituents, the clinical significance, and how the microenvironment can be incorporated into preclinical models.
Key Points
Potential of omics
- TCGA studies have used patient samples to identify new tumor classifications that have prognostic significance and novel therapeutic targets
- Panels of cancer cell lines have been used to identify gene signatures that are predictive of therapeutic response and present in patient samples
- Data analytical tools are being developed and validated in order to integrate different “omic” data types
Intrinsic systems biology and genomics in cancer
Since the mapping of the human genome, numerous technological advancements have enabled the “omics” era in which high-throughput profiling efforts provide comprehensive assessment of changes in DNA, RNA, and protein level in tumors and associated stroma that occur during cancer genesis, progression and response to therapy.12 Much work today is aimed at developing strategies to use these data to improve the clinical management of cancer. The bottleneck now lies in data processing and interpretation.13 Removing the bottleneck will require novel bioinformatic approaches that can effectively identify actionable molecular events and druggable therapeutic targets. Although the robustness of platforms and validation of gene signatures are some of the major concerns regarding their clinical utility,14, 15 there are multiple pragmatic issues that must be addressed to better integrate genomic platforms into the clinic. For example, next-generation tumor banks now require greater infrastructure with a larger team of health-care providers that acquire and manage relevant tissue and associated clinical metadata.16 Tissues for study include surgical specimens, tissue biopsies, and blood samples, each requiring different standard operating protocols and sample preparation techniques.16 Nonetheless, several institutions have been using genomic platforms to guide clinical trials or in clinical contexts, particularly for diseases where there is little evidence supporting current management practices such as tumors of unknown origin.12, 17, 18
This section is divided into three parts: (1) Genomic analyses of patient samples to provide a better understanding of cancer biology and help fuel future clinical trials. (2) Preclinical models to select cancer subtypes that will better respond to therapy. (3) Bioinformatics approaches to integrate the different genomic platforms.
High-throughput genomics
High-resolution genome analysis techniques are being used in international cancer genome analysis efforts to catalog aberrations driving the pathophysiology of nearly all major cancer types. Together, The Cancer Genome Atlas19 (TCGA, http://cancergenome.nih.gov/) project and the International Cancer Genome Consortium20, 21 (ICGC, http://www.icgc.org/) have assessed aberrations in over 12,000 samples in 55 separate tumor lineages.22 The broad goal of these efforts is to improve the prevention, diagnosis, and treatment of cancer patients.19 In these projects, patient samples have been profiled on multiple platforms that examined whole-genomes and whole-exomes,23–26 mRNA, DNA methylation,27 miRNA,28, 29 and protein and phosphoprotein levels.30 Results for several cancers are already publically available25, 31–33 and several recent papers have described integrative analyses of the characteristics of a dozen human cancer types.34–36 Genome aberrations37 found to be important in human cancers include (1) somatic changes in DNA copy number that increase or decrease the levels of important coding and noncoding RNA transcripts; (2) somatic mutations that alter gene expression, protein structure, and protein stability and/or change the way transcripts are spliced, (3) structural changes that change transcript levels by altering gene-promoter associations or create new fusion genes,38, 39 and (4) epigenomic modifications that alter gene expression.40, 41
Analysis of these rich datasets has yielded clinically relevant insights into how we may better treat different cancers. For example, urothelial bladder cancers were found to be enriched in mutations in chromatin-regulatory genes, suggesting the potential use of therapeutic agents that target chromatin modifications.23 Half of the high-grade serous ovarian cancers analyzed were found to be defective in homologous recombination, increasing their likelihood to be more susceptible to poly (ADP-ribose) polymerase (PARP) inhibitors.25 Classification systems are now emerging that stratify tumors into biologically distinct subtypes and are demonstrating greater prognostic and predictive significance in comparison to the commonly used histologic classifications. Illustrative classification systems are now emerging for cancers of the breast,42, 43 colon,44 pancreas,45 ovary,25 lung,46 and gastric cancer.24 The METABRIC cohort is a particularly well-developed cohort comprised of nearly 2000 breast tumors that were profiled to assess changes in copy number and gene expression.49 Analysis of the copy number profiles identified 10 breast cancer subpopulations, each with a unique spectrum of alterations and disease-specific survival rate.
Our understanding of the molecular landscape of breast cancer is particularly advanced. A key observation from the TCGA breast cancer study33 is that there are a few common mutations that occur in >5% of tumors (e.g., TP53, PIK3CA, CDH1, MLL3, GATA3, and MED12) and hundreds more that occur in <1% of breast cancers. The genomic aberrations in an individual tumor are comprised of a mix of “driver” and “passenger” aberrations. Driver aberrations are selected during tumor progression because they enable one or more aspects of the cancer pathophysiology that allow initiation, progression, and determination of cancer behavior, including response to therapy.48 Passenger aberrations do not contribute to cancer pathophysiology but arise by chance during progression in a genomically unstable tumor.
Recent analyses of glioblastoma have illustrated the strong interplay between the genome and treatment. The initial TCGA studies31, 50 demonstrated a link between MGMT promoter methylation and a hypermutator phenotype consequent to mismatch repair deficiency in temozolomide-treated glioblastomas. Johnson et al.51 performed exome sequencing for initial and recurrent low-grade gliomas. Of 10 tumors treated with adjuvant temozolomide, 6 were hypermutated when they recurred and carried driver mutations involving the AKT-mTOR pathways. This suggests a role for therapeutic agents that target the AKT-mTOR pathways in patients with recurrent low-grade gliomas previously treated with temozolomide. In many cases, the genome landscapes of the recurrent tumors were dramatically different than those of the primary tumor, illustrating the need for longitudinal following of patients during treatment.
A pan-cancer approach was launched in 2012 to analyze genomic aberrations among the panel of TCGA cancers36 with the goal of identifying genomic features common to multiple cancer types. Initial studies have focused on 13 cancers.52, 53 Integrative analysis of genomic and proteomic platforms identified novel cancer subtypes that were associated with survival.34 Interestingly, the pan-cancer approach to proteomic profiling revealed an elevated expression of HER2 in several cancers, including endometrial cancer, bladder cancer, and lung adenocarcinoma.30 Because endometrial cancer has a higher expression of HER2 in comparison to breast cancer, this suggests that anti-HER2 agents, such as T-DM1, may be effective in treating endometrial cancer.30 The pan-cancer approach lends itself to basket-style clinical trials, which are guided by specific molecular aberrations across different tumor types, as opposed to the traditional clinical trial designs that use large populations based on histologic classifications.54
Organization of these large-scale data for convenient visual and computational analyss is critical to enable community-wide. Useful tools for this are now emerging, and several of the most popular are listed in Table 1.
Table 1 Computational and visual analytical tools available for community wide use
Tool | Uniform resource locator (url) |
UCSC Cancer Genome Browser | https://genome-cancer.ucsc.edu/ |
cBioPortal for Cancer Genomics | http://www.cbioportal.org |
Sage Synapse | https://www.synapse.org |
Catalog of Somatic Mutations in Cancer | http://cancer.sanger.ac.uk/cosmic |
The Cancer Proteome Atlas | http://app1.bioinformatics.mdanderson.org/tcpa/_design/basic/index.html |
Gene signatures—prognostic and predictive biomarkers of response
Tissue histology and locally invasive properties are still commonly used as surrogate markers for distant metastatic spread and indicators of outcome. However, in the last decade, conventional classification strategies have been supplemented by omic-based strategies. These efforts began in 1999 with the demonstration that gene expression analysis could be used to distinguish between acute myeloid leukemia and acute lymphoblastic leukemia.55 This was quickly followed by an analysis of gene expression in breast cancer that identified intrinsic breast cancer subtypes, designated as hormone receptor-positive luminal A and luminal B, HER2-enriched, basal-like, and normal-like.56 The classification of these subtypes was validated in several independent cohorts and was found to have strong prognostic significance.56
Several efforts are now underway to use this information to develop clinically validated prognostic signatures. In breast cancer, for example, van’t Veer et al.57 identified a 70-gene signature that could predict recurrence in a select group of younger patients with early breast cancers. This signature, now marketed by Agendia BV as MammaPrint, was validated in independent cohorts and outperformed Adjuvant! Online software in clinicopathologic risk assessment.58, 59 Although smaller retrospective studies have demonstrated the use of MammaPrint as a prognostic marker,59, 60 no prospective data is yet available that demonstrates its benefit in the adjuvant setting. Another test, marketed by Genomic Health as OncotypeDx, was developed in 2004 using an RT-PCR assay on RNA extracted from formalin-fixed paraffin-embedded tumor blocks. This 21-gene assay was tested retrospectively in two clinical trials, and was found to have prognostic significance. It is now widely used in the clinic to inform patients with estrogenreceptor-positive and early breast cancer of their risk of distant recurrence and potential benefit from chemotherapy.61, 62 Several competing prognostic and predictive gene expression signatures have been published63, 64 including a 17-gene signature for estrogen receptor-positive, node-negative breast cancer,65 a 50-gene PAM50 risk of recurrence signature,66 and a 44-gene Rotterdam signature.67
Not surprisingly, cancer subtype signatures are being developed for a growing number of other cancers. In colon cancer, for example, three unique gene signatures have been identified: Oncotype Dx colon cancer assay (Genomic Health, Inc.), ColoPrint (Agendia), and ColDx (Almac).71 Although these tests have been shown to be independent prognostic biomarkers, their predictive value is still unknown.72 In a positive move, academic efforts are now underway to reconcile diverse colorectal cancer classification schemes-based gene expression signatures.44 For example, gene expression analysis identified basal-like and luminal subtypes associated with overall and disease-specific survival.73 A pancreatic cancer classifier has been reported that identified three subtypes of pancreatic ductal adenocarcinoma that demonstrated a differential prognosis response to therapy.45 Importantly, an ongoing community-wide classification effort is now underway that is attempting to integrate genomic and proteomic profiles to identify subtypes that transcend tumor origin. A recent report from this group defined a unified classification of tumors into 11 major subtypes.34 This approach will become more powerful as additional tumor types are added to the analysis.
Experimental models of patient tumors for pharmacogenomics studies
Identification of molecular features that are causally related to clinical outcomes such as cancer progression or response to therapy requires experimental models in which cells carrying aberrant genes and networks can be manipulated. Collections of cell lines derived from patient tumors are widely used as laboratory models of cancer.74 Cell lines are an attractive model system for studying cell-intrinsic biology for several reasons, including that they are: (1) a renewable resource; (2) can be manipulated in the laboratory setting; (3) are amenable to genomic profiling; and (4) can be used to assess therapeutic responses can be quickly assessed.74, 75 Although the first tumor-derived cell lines were established in the 1950s, their use as an experimental tool gained traction with the development of the National Cancer Institute 60 (NCI60) platform.76, 77 The NCI60 consists of 60 human tumor cell lines, representing nine cancer types, and has been used to screen over 100,000 compounds for therapeutic efficacy.78 Analysis of these data with the COMPARE algorithm has provided a quantitative method for identifying associations between molecular features of cells and sensitivity to particular compounds.78
Since the demonstration of the utility of the NCI60, several other groups have developed panels of cancer cell lines, including pan-cancer79–81 and tissue-specific collections (e.g., breast,82 lung,83, 84 and melanoma85). One of the most well-developed panels, comprised of ∼70 breast cancer cell lines, has been used to assess gene function and to identify mechanisms of therapeutic response and resistance.82, 86, 87 Recently, very large cell line collections representing multiple tumor types have been used to identify associations between molecular features and response to molecular perturbants across tumor types.80, 81, 88 Comparison of the genomic and epigenomic features of cell lines with those measured from primary tumors showed that the cell lines mirror many aspects of “omic” diversity in primary tumors that are likely to influence therapeutic response. Cell line and tumor similarities include: (1) recurrent copy number changes and mutations,82, 86, 89 (2) transcriptional subtypes,86, 89 and (3) pathway activity.86 However, there are significant exceptions. For example, cell lines grown on plastic have been reported to change epigenomic status90 and some cell lines fail to retain genomic aberrations that were present in the tumors from which they were derived. Glioblastoma is a notable example of the latter, as cells grown on plastic usually fail to retain a region of amplification involving the EGFR oncogene that is frequently present in primary tumors.91
In breast cancer, analysis of correlations between drug sensitivity and molecular features revealed that approximately 30% of the compounds tested are associated with subtype or genome copy number aberration,86 and robust integrated predictive signatures of sensitivity can be identified for ∼50% of compounds.86, 87 Importantly, many of the in vitro signatures can be observed in primary patient samples80, 81, 87 suggesting that cell line studies may be used to guide the development of signatures that can be used to stratify patients in the clinic. Evidence from other tissue types also supports the notion that cell lines are a powerful system for studying the molecular underpinnings of therapeutic response. For example, in vitro model systems accurately recapitulated several clinical observations, including that (1) lung cancers with EGFR mutations respond to gefitinib,92 (2) breast cancers with HER2/ERBB2 amplification respond to trastuzumab and/or lapatinib,82, 93 and (3) tumors with mutated or amplified BCR-ABL respond to imatinib mesylate.94 Of course, cell lines grown on plastic do not model many aspects of human cancers. A particular weakness is that they do not model the impact of the microenvironment on cancer cell behavior. Models the include signals from the microenvironment as covered later in this chapter.
Principles of integrative analysis
Several computational tools have been developed to identify molecular signatures associated with biological behavior. One of the first of these is gene set enrichment analysis (GSEA).95 The rationale behind GSEA is to analyze the expression of predefined groups of genes that share a common biological function, chromosomal location, or regulation, to determine whether they show wholesale differences in gene expression between comparator populations. For example, GSEA analysis identified the RAS, NGF, and IGF1 pathways as differentially expressed in TP53 mutant versus TP53 wild-type cancers.95
The network analysis tool PARADIGM96 was designed to identify pathways whose activities differ between comparator populations. PARADIGM integrates multiple omic data types, including DNA copy number and gene expression, to calculate integrated pathway levels (IPLs) for over 1300 curated signal transduction, transcriptional, and metabolic pathways from publicly available databases (e.g., the NCI Pathway Interaction Database KEGG, Reactome, and BioCarta). These IPLs can then be used to identify subnetworks that differ between comparator populations (e.g., responsive versus resistant tumors). The subnetworks are composed of interlinked pathway features (genes, proteins, complexes, families, processes, etc.) that take on activities distinctive in one class of tumors compared to another. For example, PARADIGM analysis of TCGA breast cancer samples identified HIF1-α/ARNT pathway activity as high in basal-like breast cancers, suggesting that these malignancies might be susceptible to angiogenesis inhibitors and/or bioreductive drugs that become activated under hypoxic conditions.33
One problem with tools that incorporate pathway information is that some genes and networks have been more extensively studied than others, and so there is inherent bias in the resultant pathways. HotNet2 is an algorithm to find aberrant networks using a strategy that tries to avoid curation bias. HotNet2 uses a modified diffusion process and considers the source, or directionality, of heat flow in the identification of subnetworks that reduces the impact of curation bias. This tool was applied in recent pan-cancer network analyses to identify 16 frequently mutated networks that affect well-known cancer phenotypes.97
Crowd sourcing to rapidly advance systems biology
Advances in profiling technologies and subsequent generation of large, complex datasets require robust analytical methods to facilitate the conversion of “big data to knowledge” (http://bd2k.nih.gov/). This effort is still in its infancy; many competing methods are being developed so it is difficult to determine which perform best. One novel approach to identify effective algorithms is using crowd-sourced community-wide challenges. The DREAM project (http://dreamchallenges.org) is one example of the power of this approach. DREAM brings communities of researchers together to address complex problems in systems biology, while also developing methods for identifying the most effective novel algorithms. A key aspect of these challenges is to eliminate the “self-assessment trap” in which data generation, data analysis, and model validation are combined within the same study.98 Moreover, the advancements that arise through these collaborative efforts come at a much more rapid pace than can be achieved through traditional single-team research approaches.
Two recent DREAM challenges demonstrate the power of this crowd-sourcing approach. In the NCI-DREAM7 challenge, unpublished drug sensitivity measurements along with transcriptional and proteomic profiles for a panel of breast cancer cell lines were made freely available to the scientific community.99 Over 40 international groups developed predictors of drug response using a broad range of machine learning and statistical algorithms. A meta-analysis of all methods demonstrated that modeling nonlinear relationships and incorporating existing knowledge are essential features for generating robust predictive biomarkers. Another DREAM challenge sought to assess the ability of computational strategies to predict breast cancer survival from clinical features, gene expression, and copy number profiles in breast cancer.100 This challenge used data from the METABRIC breast cancer cohort,49 and in multiple rounds of blinded evaluations, assessed the performance of more than 1400 models. It showed that the best-performing modeling strategy significantly outperformed a benchmark first-generation 70-gene risk predictor.58 Interestingly, this study also demonstrated the “wisdom of the crowd” as the aggregation of the predictions from all the models performed as well or better than the best model. These results demonstrate the power of crowd sourcing to advance algorithm development and identify clinically relevant biomarkers.
Key Points
The tumor microenvironment (TME)
- The TME is comprised of multiple cellular and noncellular components
- The TME composition influences cancer progression and patient outcome
- The TME is implicated in therapeutic response and resistance
- Preclinical modeling of TME is challenging but now amenable to high-throughput platforms
- Patient-derived xenografts can predict response to therapy and can potentially be used alongside clinical trials
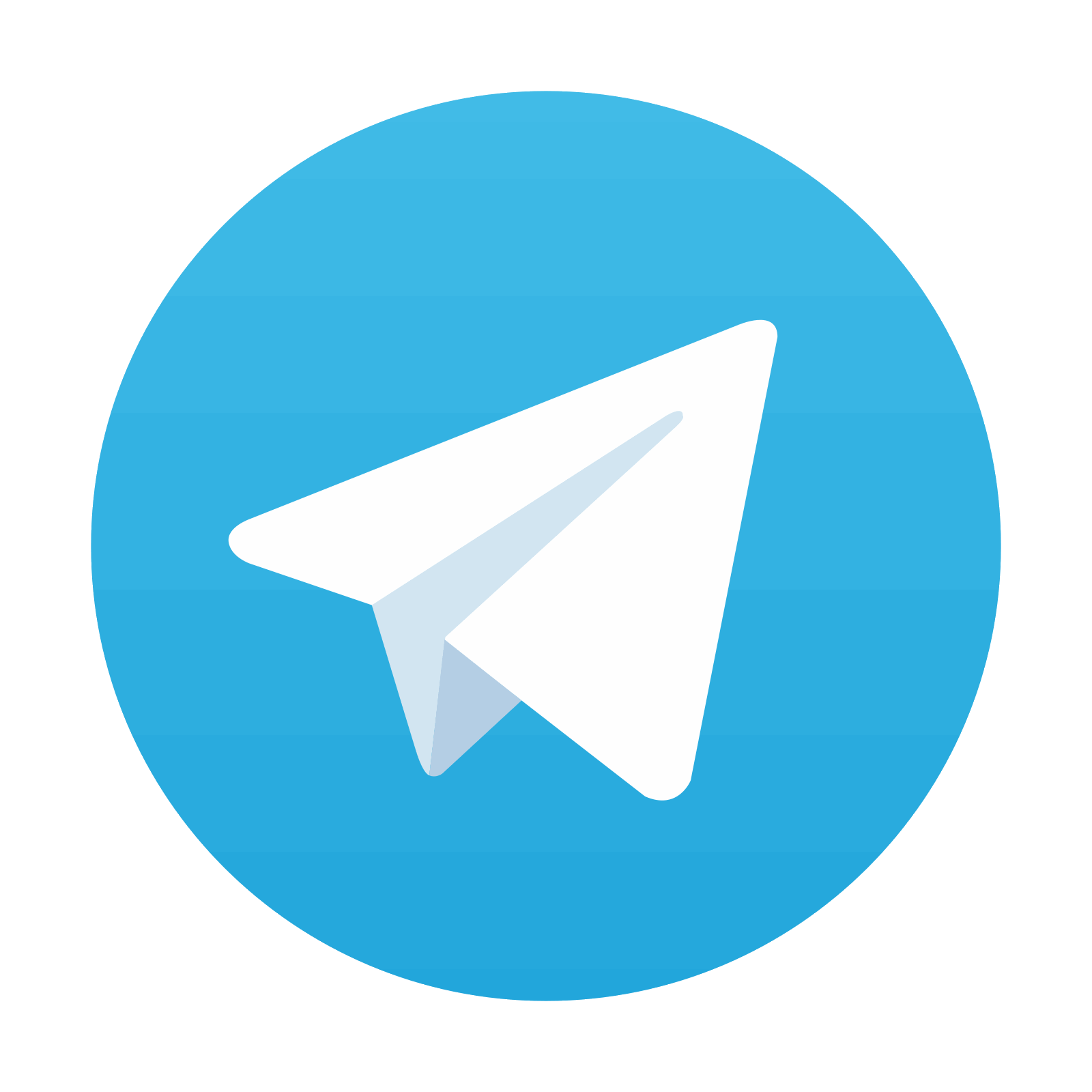
Stay updated, free articles. Join our Telegram channel

Full access? Get Clinical Tree
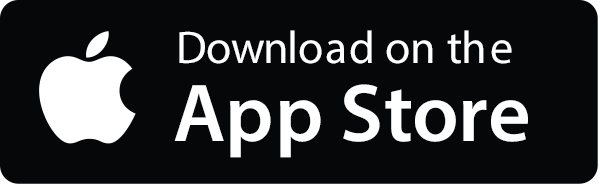
