Trial purpose
Aim
Design
Breast cancer-specific examples
Breast cancer prevention
To identify ways to reducing the incidence of breast cancer
Cohort studies of lifestyle impacts, use of drugs in randomised trials
IBIS I and II
Breast screening
To identify effective ways to increase early diagnosis
Randomised trials, cohort study with bias correction
Swedish Two-County and other screening trials
MARIBS trial of breast MRI screening
Diagnostic
To evaluate the efficacy of diagnostic tests, predictive and prognostic biomarker studies
Observational cohort or randomised trials to evaluate accuracy, sensitivity and specificity of new diagnostic tests
Treatment
To assess the efficacy of new drugs, surgical techniques and radiotherapy regimes
In surgery: often observational studies of case series and cohorts, some randomised studies [10, 11].
RCTs widely used in systemic therapy trials often with large meta-analyses to confirm findings
The AMAROS trial comparing axillary clearance and radiotherapy
The ALMANAC trial comparing axillary clearance and SLNB
Quality of life and supportive care
To look at ways of improving the quality of life of cancer patients during and after treatment
Quantitative questionnaire design, validated quality of life tools, PROMs and qualitative research
Another common way to classify trials is according to their phase as they progress from testing the basic physiological impact of the new intervention in vitro, in animal models (preclinical) and humans, to dose finding and ultimately confirmatory studies of safety and efficacy, at which point regulatory approval is usually granted. Refinements of use are then made in late phase studies (◘ Table 63.2). All have a role in the evaluation of new treatments.
Table 63.2
Table describing the common phases of clinical trials
Phase | Aim |
---|---|
Preclinical studies | In vitro and animal studies |
Phase 0 | First in human studies to define pharmacodynamics and pharmacokinetics |
Phase 1 | Safety screening |
Phase 2 | Efficacy and dose finding |
Phase 3 | Comparative efficacy |
Phase 4 | Use optimisation in clinical practice |
63.4 Specific Research Methodologies and Research Quality Standards
63.4.1 Randomised Trials and Meta-Analyses
Randomised controlled trials (RCTs) and meta-analyses of data from such trials are considered the highest levels of evidence supporting clinical practice in oncology (◘ Fig. 63.1).
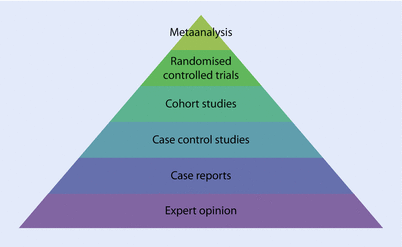
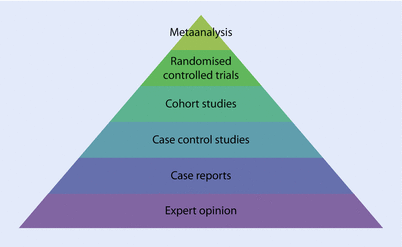
Fig. 63.1
Hierarchy of research evidence
The quality of the design and the quality control of these trials are of the utmost importance to warrant the safety, efficacy and reproducibility of the data and conclusions drawn from them. Surgical trials however have a number of challenges when compared to non-surgical trials. Choosing a homogenous patient population and an equivalent control group is challenging. Surgery, unlike a pill, is not a standardised, reproducible entity, but rather a unique product whose details are defined by variables, which include the skill of the surgeon. The skill level will not only vary among surgeons, but will increase for the same surgeon whilst he/she gains experience (surgical procedures have a learning curve) [19]. Furthermore, surgeons with a specific interest in a procedure will perform better [20]. These surgeons are also well disposed to develop new techniques in their own centre and subsequently analyse their series. This is one of the reasons why so many informative non-randomised hospital or personal series are published. RCTs in surgical oncology are therefore less common than cohort studies. Surgical versus non-surgical comparative trials also suffer from problems of lack of equipoise both on the part of the surgeon and the patient as the differences between treatments are often extreme. It is also difficult to blind the patient and surgeon to the intervention which may introduce bias.
There are some examples of well-designed trials comparing two surgical modalities or surgical versus non-surgical interventions. Trials such as those conducted by pioneering surgeons Umberto Veronesi in Europe and Bernard Fisher in the USA, comparing mastectomy versus breast-conserving therapy several decades ago, are excellent examples which lead to a massive change in practice in the field of breast cancer care [10, 11]. Trials that have successfully compared surgical and non-surgical options have also been conducted in breast care. An innovative, highly impactful and controversial trial compared standard axillary completion clearance with no further surgery in the ACOZOG 0011 trial [21]. This trial, whilst methodologically imperfect, has again changed global practices concerning axillary clearance in patients with low-risk clinically positive lymph nodes. Similarly the AMAROS trial, in which patients with T1–2 primary breast cancer and no palpable lymphadenopathy were randomised to receive either axillary lymph node dissection or axillary radiotherapy in cases with a positive sentinel node, concluded that radiotherapy gave excellent oncological results with less axillary morbidity [12]. As with trials in other surgical disciplines, breast surgery trials have also sometimes included a learning curve phase to ensure technical competency in the new technique. A good example of this was the ALMANAC trial of SLNB in breast cancer [1, 2, 18, 22]. There have been many advances in trial methodology in the past decade to ensure that data generated is valid and may be compared between studies. These have been formalised into trial guidelines such as the CONSORT statement for RCTs [23] and the PRISMA standards [24] for systematic reviews and meta-analyses. There are numerous other quality standards in action. It is essential that breast surgeons have a good understanding of how to assess the quality of research evidence so they can decide what is worthy of clinical adoption. There are a number of excellent overviews of how to critically assess the quality of research, a skill that should be an integral part of an oncologists’ training [25–28].
In addition to randomised trials and meta-analyses, there are valid reasons why some research questions cannot be answered using this methodology. In the field of breast cancer, there are many such examples of where a cohort methodology is advantageous or the only feasible option.
63.4.2 Observational Studies (Cohort, Case Control)
Data from observational studies are increasingly used to fill knowledge gaps [29]. However, several challenges exist in the use of observational data: bias due to confounding by indication is one of the major obstacles. This could potentially be tackled by using comparative effectiveness research if careful design and analysis is applied. For those research questions where randomised controlled trials are unethical, impractical or simply too lengthy for timely decisions, observational research may be used. The main problem with observational studies is treatment allocation bias which is difficult to fully adjust for in analysis. Patients who received a certain treatment typically differ from patients in whom that treatment is omitted. Excellent examples are patients treated non-surgically with primary endocrine therapy for operable cancer due to age, frailty or comorbidity will have higher morbidity and mortality rates than the fitter cohort who undergo the surgical option. Although it may be possible to adjust for factors that were measured, there will always remain certain factors that were unmeasured, so-called residual confounders [30]. The best example is frailty which is rarely formally assessed in studies but has a profound impact on treatment allocation and outcomes. Direct comparison of treatments can result in overestimation of treatment efficacy, and it is very likely that this problem occurs in most studies that have used this methodology. Although randomisation does not guarantee that treatment groups are equal across all possible confounding factors, it does guarantee that residual differences between groups are due to chance [30].
One of the more appropriate alternative methods that can be used to study treatment effectiveness in observational data is the use of instrumental variables. The instrumental variable is a factor that is associated with the allocation of a certain treatment, but is not directly associated with the outcome. For example, if two countries with comparable patients have a very different treatment strategy, the country may be used as the instrumental variable. Instead of comparing the outcome of patients with and without a certain treatment, differences in outcome between the two countries are compared, which eliminates bias due to confounding by indication [30]. There are three conditions that must be fulfilled for the use of an instrumental variable:
- 1.
The instrumental variable must be related to the probability of receiving the treatment under investigation.
- 2.
It should not be related to the prognosis of the patient.
- 3.
It should not affect the outcome in any other way than through the treatment given [31].
The use of an instrumental variable is particularly useful in populations where randomisation is not feasible or not ethical [31], but it can be challenging to identify a proper instrumental variable that fulfils all three criteria. Still, when a good instrumental variable is available, it is considered to be the best method that can be used to study treatment effects in observational research [30].
Excellent examples of where observation studies have value are in areas where there are high levels of patient variability such that stratification with a randomised trial would be unfeasible. Research into the elderly is an excellent example of where observational data may be of value. Randomised trial inclusion of patients over the age of 70 is very limited with only 1–5% of the included patients older than 70 years, and there are a number of valid reasons for this. Not all older patients are suitable for standard treatments administrated to younger patients, and heterogeneity of the older population may complicate inclusion criteria [32]. Furthermore, early mortality – directly resulting from comorbid conditions – could reduce the apparent effectiveness, and shorter follow-up time will decrease statistical power to detect differences between treatment and control arms. Besides these methodological barriers, the participation and preferences of older patients and the willingness of otherwise of their clinicians may be considered another barrier. For all of these reasons, observational studies with appropriate adjustment for patient characteristics may be the only avenue to determine best practice in this age group.
63.4.3 Case Reports
For exceptionally rare conditions, running trials or even large observational studies may be impossible, and case reports may be the appropriate level of evidence to support best practice. In the field of breast cancer surgery, publication of case reports may ultimately lead to better understanding of rare associations, for example, the link between angiosarcoma and radiotherapy or the newly described but exceptionally rare breast implant-associated anaplastic large cell lymphoma (BIA-ALCL) (► Chap. 29, breast implants).
63.5 Clinical Trials and Bias
A full understanding of bias and how it influences study results is essential for the practice of evidence-based medicine. Bias is defined as any factor of influence which prevents objective consideration of a question. Bias can occur at any phase of a study, including study design or data collection, as well as in the process of data analysis and publication. Breast surgeons must be trained to critically interpret study results and must also evaluate chosen endpoints, study design (patient population/controls) and identify study biases.
In clinical trials some forms of bias are always present; the issue is to what degree bias influences a favourable outcome for the test group (◘ Table 63.3) [33–35].
Table 63.3
Different types of bias which may affect clinical research
Trial period | bias | Definition | How to avoid |
---|---|---|---|
Planning the trial | Flawed study design | Many errors can occur in study design. Sample size should meet the power. Study groups should be equal, control treatment as equal as possible, endpoints adequate to answer to the hypothesis, time point adequate to test the hypothesis, etc. | Meticulous study design, power analysis, publish clinical trial study design, register trials, medical ethical committee approval before starting the trial |
Selection bias [33] | Comparing two different groups | Prospective design with unknown outcome reduces the likelihood of selection bias. Clear defined study population with inclusion and exclusion criteria for patients most at risk | |
Randomisation or allocation bias | Use blinded treatment allocation | Researchers should not have a hand in allocation of treatments preferably by using computerised external randomisation | |
Bias during the trial execution | Interviewer bias | Questions to be asked should be standardised to avoid any suggestion of interviewers | Use blinding for group/outcome. Use validated questionnaires to avoid ‘researcher’s influence’ |
Recall bias | Influence of the question in the recall of events happened in the past | Only use validated tools (questionnaires); use objective interviewers blinded to group and outcome | |
Chronology bias | Historical data as control | Prospective design consecutive patients | |
Performance bias | Variation in performance can influence outcome results | Standardise surgical techniques to minimise variation between surgeons | |
Bias from misclassification | Misclassification of results or outcome due to variation in interpretation to classify results | Blinding for outcome, standardisation of data collection and outcome | |
Transfer bias | Missing information due to subjects lost to follow-up | Reduce lost to follow-up as much as possible | |
After the trial | Publication bias [34] | The tendency of investigators to submit or the reviewers and editors to accept manuscripts based on the direction or strength of the study results | Reviewers and editors’ responsibility to accept all decent quality research regardless of positive or negative findings |
Citation bias | Tendency of negative results not to be published and positive results to be published | Also publish negative results of studies Preregister trials | |
Confounders | Any factor that correlates with dependent and independent variables | Control for confounders in the study design. Use stratification. Use double blinding and randomisation | |
Internal vs external validity [35]
![]() Stay updated, free articles. Join our Telegram channel![]() Full access? Get Clinical Tree![]() ![]() ![]() |