Summary of Key Points
- •
This chapter provides a review of some of the most promising recent studies of diagnostic biomarkers in lung cancer.
- •
We discuss the challenges and the importance of biomarker validation. Current guidelines recommend a study design to include prospective collections of specimens and retrospective blinded evaluation.
- •
A novel multiomics approach to biomarker discovery has greatly advanced the field of early lung cancer detection.
- •
Noninvasive biomarkers in the blood, sputum, airway epithelium, or exhaled breath can be combined with imaging to detect early stage lung cancer and improve mortality.
Acknowledgment
This work was supported in part by NCI EDRN-sponsored Clinical Validation Center CA086137 (W.N.R.) and by NCI EDRN-sponsored Clinical Validation Center CA152662 (P.P.M.).
Lung cancer is the leading cause of cancer deaths in the United States and worldwide. This statistic is largely due to the persistent poor survival of patients diagnosed with lung cancer. In the United States as of 2009, the overall 5-year survival for nonsmall cell lung cancer (NSCLC) remained at only 16.6%. However, if the cancer is detected at an early stage, the 5-year survival exceeds 50%. For this reason, in the last decade, the quest for an effective means of early diagnosis has intensified. In 2011, the results of the randomized multicenter National Lung Screening Trial (NLST) were published, confirming that early diagnosis of lung cancer can improve survival. Screening for lung cancer in the high-risk group studied in the NLST now has the support of the US Preventive Services Task Force (grade B recommendation). However, low-dose computed tomography (CT) of the chest for lung cancer screening has significant drawbacks, including cost, radiation exposure, high false-positive rates, and a risk of overdiagnosis of indolent cancers. Thus the results of NLST have sparked even greater interest in developing more practical and more specific means of early detection of lung cancer, using noninvasive biomarkers of early disease.
Biomarkers for lung cancer have several potential clinical uses in addition to early detection ( Fig. 8.1 ). They may be used for risk stratification, optimal treatment selection, prognostication, and monitoring for recurrence. Markers of risk can help identify a population to be screened. At this preclinical stage, the marker identifies individuals without disease but with factors that may predispose them to lung cancer. Given the high false-positive rate with CT screening, a marker that could more clearly define the at-risk population could decrease the number of screening CT scans conducted and also improve the specificity of CT screening, thus decreasing patient anxiety and the need for repeated CT and invasive procedures induced by false-positive nodules.
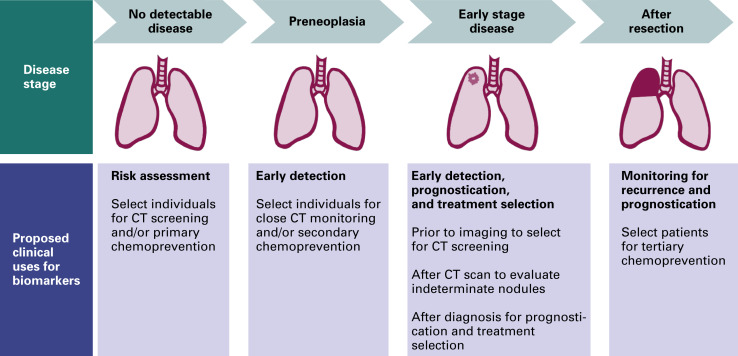
Markers are currently used for treatment selection, prognostication, and monitoring for recurrence in patients with known disease. A variety of markers, reflecting the biology of lung cancer progression from premalignant lesions to invasive lung cancer, may prove to be more useful for each of these roles. In this chapter, we focus on current and potential biomarkers for the early detection of lung cancer. Markers of risk and prognosis are not reviewed.
Early Detection
For the foreseeable future, CT will undoubtedly remain an important part of any program for the early detection of lung cancer. CT can detect the small noncalcified nodules that may represent early lung cancers. However, as a stand-alone screening tool, this technique is problematic. First, it has poor specificity because of the high prevalence of nonspecific benign pulmonary nodules. Second, CT is costly, and the necessity for repeated CT to determine growth rates over time can expose patients to potentially harmful radiation. Lastly, we cannot predict which early lung cancers will progress and which will remain indolent for prolonged periods.
The ultimate goal of lung cancer early detection biomarker research is to develop a marker that identifies early stage lung cancer (or even preneoplasia) and prompts a change in clinical practice that saves lives. A more obtainable target may be a marker that can be used in conjunction with chest CT to help distinguish malignant from benign nodules found on CT images or identify aggressive or indolent phenotypes of early lung cancers found by imaging. Depending on the selected size cutoff, 15% to more than 50% of individuals in CT screening programs have nodules. NLST demonstrated that more than 96% of the nodules identified were thought to be benign based on stability on follow-up CT. Of nodules that are ultimately surgically resected, up to 30% are found to have benign pathology. In the NLST, 24% of patients who underwent an invasive diagnostic procedure were found to have nodules of benign etiology. To address the issue of large numbers of false-positive findings on CT, experts have suggested using a larger nodule size cutoff of 7 mm or 8 mm, which would decrease the number of positive CT results to 5% to 7%, or narrowing the definition of high-risk individuals who would be eligible for screening. An effective biomarker would also be an invaluable aid in the management of these indeterminate pulmonary nodules. Depending on their assay performance characteristics, biomarkers could guide the clinician toward reassurance, watchful waiting, or immediate biopsy or resection, and thus decrease the anxiety, cost, and uncertainty of lung cancer screening.
Lung cancer biomarkers may also reduce the problem of overdiagnosis in lung cancer screening. Although the NLST demonstrated that screening can decrease lung cancer mortality, a percentage of cancers diagnosed are likely indolent malignancies that may not progress if disregarded. At the New York University screening program, one-third of the cancers diagnosed were indolent adenocarcinomas, which were followed for a prolonged period before resection and were still stage I at the time of surgery. A biomarker that could a priori identify these indolent cancers may spare older patients or patients with other medical problems unnecessary surgeries.
The Biology of Lung Carcinogenesis
Continued progress in understanding the sequence of molecular changes underlying the progression from preneoplasia to invasive lung cancer has galvanized research into discovery and validation of lung cancer biomarkers for early detection. It has also raised the possibility of personalizing lung cancer treatment using biomarker profiles. The World Health Organization defines the various preneoplastic lesions of the bronchial epithelium as squamous dysplasia and carcinoma in situ, which progresses to squamous cell carcinoma; atypical adenomatous hyperplasia, which may precede adenocarcinoma; and diffuse idiopathic pulmonary neuroendocrine cell hyperplasia, which may progress to carcinoid. Small cell lung cancer (SCLC) is believed to arise from extensively molecularly damaged epithelium without going through recognizable preneoplastic stages.
Alterations in gene expression and chromosome structure known to be associated with malignant transformation have been demonstrated in these preneoplastic lesions, and the changes appear to be sequential; in particular, their frequency and number increase with increasing atypia. Some of the alterations found in preneoplastic lesions include hyperproliferation and loss of cell cycle control; abnormalities in the p53 pathway, the RAS genes, and genes in the genomic region of 3p14.2 and 3q26-29; aberrant gene promoter methylation; increased vascular growth; altered extracellular matrix; decreased retinoic acid and retinoid X receptor expression; and many other genetic and epigenetic changes.
Biomarker Validation
The validation of a biomarker for clinical use is challenging. Any biomarker considered for use in a clinical setting must satisfy a host of criteria related to ease of use and performance. The biomarker must be relatively noninvasive, require only small amounts of material needing a minimum of preparation, be quantifiable and reproducible in multiple populations and laboratories, have a proven clinical use with acceptable sensitivity and specificity for this use, be acceptable to the target population, and be cost-effective and reimbursed by health insurers. No markers have yet made it through these rigorous requirements, although many are in the pipeline. Appropriate study design will be crucial to bringing any of these markers to clinical use.
Guidelines for biomarker study design and statistical evaluation suggest that validation should be conducted using a prospective specimen collection retrospective blinded evaluation design. In this approach, specimens are collected prospectively from a longitudinal cohort that represents the target population. After the outcome status is determined, a nested case–control study can be designed. Cases and controls are selected randomly for biomarker studies, with the investigators blinded to the case–control status. Random sampling of cases and controls from within a well-defined cohort provides validity to the case–control design. An important element of this study design is that the validation population must be representative of the population in which the biomarker will be used, to minimize false positives. In the case of lung cancer, this means that individuals with a history of tobacco use and its related morbidities, including chronic obstructive pulmonary disease, cardiovascular disease, and other malignancies, must be included in the validation cohort. Ideally, the biomarker can be tested in longitudinal samples to ensure its accuracy in detecting early, preclinical disease. Measures of validity include sensitivity, specificity, negative predictive value, and positive predictive value (which can be summarized with a receiver operating characteristic [ROC] curve). The prevalence of the disease influences these measures, thus it is important that the biomarker validation process be applied to all possible populations in which the marker would be used. Lastly, when a potential marker has been validated as effective for early diagnosis, it should be evaluated in a screening trial with lung cancer mortality as the end point to prove that use of the biomarker decreases mortality and the validation studies were not hampered by problems of overdiagnosis, lead-time bias, or length bias. The Early Detection Research Network of the US National Cancer Institute has established guidelines for cancer biomarker development and validation.
Advances in Techniques for Biomarker Discovery
Currently, we see a profusion of potential biomarkers for lung cancer. Different histologic types, different stages of disease, and a variety of molecular pathways to transformation contribute to making the process of biomarker discovery for lung cancer complex. New high-throughput technologies allow researchers to look for and validate multiple biomarkers simultaneously. Microarrays are used to evaluate thousands of potential markers concurrently.
For example, circulating DNA (cDNA) microarrays identify thousands of genes that are differentially expressed in lung cancers, preneoplasias, and normal lung; antibody arrays evaluate multiple antigens or antibodies at once; and methylation arrays identify methylation of many different gene promoters simultaneously. Proteomics is the study of protein profiles in tissues and body fluids. Matrix-assisted laser desorption/ionization time-of-flight mass spectrometry (MALDI-TOF MS) and surface-enhanced laser desorption/ionization have been used to describe protein profiles and to identify individual protein markers in lung cancer. The ability to accurately measure quantitative transcriptome in individual cells with relatively small number of sequencing read makes single-cell RNA sequencing a popular technology for biomarker discovery. In recent years, important advances in the development and validation of these and other high-throughput technologies have raised the potential for great strides in biomarker discovery.
Specimen Types
One of the most important criteria for a successful biomarker is that the testing material be easily accessible. Current markers use multiple biologic sources. Tissue-based assays are generally the most invasive, but may be acceptable in some circumstances. The concept of field cancerization supports the theory that surrogate tissues—such as bronchial, buccal, and nasal brushings; endobronchial biopsy specimens; or even exhaled breath—may be used as markers of increased risk for lung cancer. Genetic and epigenetic changes in the bronchial epithelium or perhaps the nasal or buccal epithelium may mirror changes in the lower respiratory tract and suggest that a lesion seen on CT images represents malignancy. Although obtaining the tissue may require bronchoscopy, pairing molecular markers obtained from the airways with a high-risk profile and a lesion on CT images may increase the specificity of lung cancer screening. The potential use of tissue-based biomarkers is highly dependent on the accessibility of the specimens and the robustness of the assay offered. It may take additional time to refine airway epithelium-based biomarkers because banked samples are not as readily available as they are for tumor tissues or blood. Blood-based assays are attractive due to the ease of acquisition. This simplicity aids in the discovery process, the validation process, and the acceptance into clinical practice. Altered or methylated DNA, overexpressed messenger RNA (mRNA), microRNA (miRNA), proteins, peptides, metabolites, and even circulating tumor cells (CTCs) can all be detected in the circulating blood; however, there are significant challenges as well. Blood is a dynamic medium, which reflects various physiologic and pathologic states that can overwhelm the detection of an early stage, preclinical cancer.
Other biofluids—exhaled breath condensate, sputum, and urine—are also easily accessible samples for biomarker analysis. Each type of sample has its own appeal and its challenges. Exhaled breath is easily and painlessly obtained, and large volumes can be collected without detriment to the patient. Theoretically, the use of exhaled breath analysis may allow for a more specific lung cancer diagnosis. However, only volatile compounds can be detected and genetic material is sparse or absent. Sputum has the advantage of perhaps giving results specific to lung cancer, as it contains both bronchial epithelial cells and other secretions reflecting the local milieu of the lung. However, it is difficult to obtain adequate sputum samples from the lower airways, and samples are frequently exclusively saliva. Urine is an easily accessible biofluid, but it may be less specific to lung cancer. Lung cancer biomarker research using urine as the biologic sample is still in its infancy.
Lung Cancer Biomarkers for Early Detection
Given the many different genetic and epigenetic changes involved in malignant transformation in lung cancer, it is not surprising that innumerable potential biomarkers exist. With progress in understanding the biology of lung carcinogenesis, the development of high-throughput techniques for biomarker discovery, and increased focus on early detection of lung cancer, the field of lung cancer biomarker research has expanded at a phenomenal rate. As yet, no biomarker has been shown to have adequate sensitivity, specificity, reproducibility, and ease of use to be validated as a biomarker for the early detection of lung cancer. However, many studies of biomarkers for the early diagnosis of lung cancer have shown promising results ( Table 8.1 ).
Author (y) | Type of Marker | Type of Specimen | Marker(s) | No. of Markers | Platform | No. in Training Set | No. in Test Set | Sensitivity a (%) | Specificity a (%) | AUC a |
---|---|---|---|---|---|---|---|---|---|---|
Cytology | ||||||||||
Varella-Garcia et al. (2004) | Chromosomal aneusomy and cytology | Sputum | Multitarget DNA FISH assay and cytology | 2 | FISH | 33 | NR | 83 | 80 | NR |
Xin et al. (2005) | Sputum cytometry | Sputum | DNA content and cytologic malignancy grade | 2 | Automated DNA image cytometry | 2461 | NR | 80 | 93 | 0.87 |
Kemp et al. (2007) | Sputum cytometry | Sputum | Lung sign: Cell nuclear features (DNA content, chromatin distribution) | 13 features | Automated DNA image cytometry | 1123 | NR | 40 | 91 | 0.69 |
Roy et al. (2010) | Nanoarchitectural alterations | Buccal epithelium | Disorder strength of cell nanoarchitecture L (d) | 1 | Partial wave spectroscopic microscopy | 207 | 46 | 78 | 78 | 0.84 |
Noncoding RNAs | ||||||||||
Xing et al. (2010) | MicroRNA | Sputum | miR-205, miR-210, miR-708 (squamous) | 3 | qRT-PCR | 96 | 122 | 73 | 96 | 0.87 |
Xie et al. (2010) | MicroRNA | Sputum | miR-21 | 1 | qRT-PCR | 50 | NR | 70 | 100 | 0.90 |
Yu et al. (2010) | MicroRNA | Sputum | miRNA signature for adenocarcinoma | 7 | qRT-PCR | 72 | 122 | 81 | 92 | 0.90 |
Bianchi et al. (2011) | MicroRNA | Serum | miRNA signature | 34 | qRT-PCR | 64 | 64 | 71 | 90 | 0.89 |
Boeri et al. (2011) | MicroRNA | Plasma | miRNA signature | 15 | miRNA array and qRT-PCR | 20 | 15 | 80 | 90 | 0.85 |
Boeri et al. (2011) | MicroRNA | Plasma | miRNA signature | 13 | miRNA array and qRT-PCR | 19 | 16 | 75 | 100 | 0.88 |
Shen et al. (2011) | MicroRNA | Plasma | miR-21, miR-126, miR-210, miR-486-5p | 4 | qRT-PCR | 28 | 87 | 86 | 97 | 0.93 |
Shen et al. (2011) | MicroRNA | Plasma | miR-21, miR-210, miR-486-5p | 3 | qRT-PCR | 94 | 156 | 75 | 85 | 0.86 |
Chen et al. (2012) 179 | MicroRNA | Serum | miRNA signature | 10 | qRT-PCR | 310 | 310 | 93 | 90 | 0.97 |
Hennessey (2012) | MicroRNA | Serum | miR-15b and miR-27b | 2 | qRT-PCR | 50 | 130 | 100 | 84 | 0.98 |
Patnaik et al. (2012) | MicroRNA | Whole blood | miRNA signature | 96 | Locked nucleic acid microarrays | 45 | NR | 88 | 89 | 0.94 |
Liao et al. (2010) | Small nucleolar RNA | Plasma | snoRD33, snoRD66, and snoRD76 | 3 | qRT-PCR | 85 | NR | 81 | 96 | 0.88 |
Genetic Changes and Gene Expression | ||||||||||
Miura et al. (2006) | mRNA | Serum | Human telomerase catalytic component and epidermal growth factor receptor | 2 | qRT-PCR | 192 | NR | 89 | 73 | NR |
Li et al. (2007) | Genetic deletions | Sputum | FHIT and HYAL2 | 2 | FISH | 74 | NR | 76 | 92 | NR |
Spira et al. (2007) | mRNA | Airway epithelium | Gene expression signature | 80 | Affymetrix array (Santa Clara, CA, USA) | 77 | 52 | 80 | 84 | NR |
Blomquist et al. (2009) | Gene expression | Bronchial epithelium | Antioxidant, DNA repair, and transcription factor genes | 14 | Standardized RT-PCR | 49 | 40 | 82 | 80 | 0.87 |
Showe et al. (2009) | Gene expression | PBMC | Gene signature | 29 | Illumina human whole genome bead array | 228 | NR | 91 | 80 | NR |
Zander et al. (2011) | Gene expression | Whole blood | Gene expression profile | 484 | Illumina human whole genome bead array | 77 | 156 | 97 | 89 | 0.97 |
DNA Methylation | ||||||||||
Palmisano et al. (2000) | DNA methylation | Sputum | P16, O 6 -MGMT | 2 | PCR | 144 | NR | 100 | n/a | NR |
Kim et al. (2004) | DNA methylation | Bronchoalveolar lavage | p16, RARβ, H-cadherin, RASSF1A | 4 | MS-PCR | 212 | NR | 68 | NR | NR |
Grote et al. (2004) | DNA methylation | Bronchial aspirates | APC | 1 | qMS-PCR | 222 | NR | 39 | 99 | NR |
Grote et al. (2005) | DNA methylation | Bronchial aspirates | p16(INK4a), RARB2 | 2 | qMS-PCR | 139 | NR | 69 | 87 | NR |
Belinsky et al. (2006) | DNA methylation | Sputum | p16, MGMT, DAPK, RASSF1A, PAX5β, GATA5 | 6 | Nested MS-PCR | 190 | NR | 64 | 64 | NR |
Grote et al. (2006) | DNA methylation | Bronchial aspirates | RASSF1A | 1 | qMS-PCR | 203 | NR | 46 | 100 | NR |
Ostrow et al. (2010) | DNA methylation | Plasma | DCC, Kif1a, NISCH, Rarb | 4 | qRT-PCR | 37 | 183 | 73 | 71 | 0.64 |
Schmidt et al. (2010) 180 | DNA methylation | Bronchial aspirates | SHOX2 | 1 | PCR | n/a | 523 | 68 | 95 | 0.86 |
Begum et al. (2011) | DNA methylation | Serum | APC, CDH1, MGMT, DCC, RASSF1A, AIM | 6 | qPCR | 401 | 106 | 84 | 57 | NR |
Kneip et al. (2011) 181 | DNA methylation | Plasma | SHOX2 | 1 | qPCR | 40 | 371 | 60 | 90 | 0.78 |
Richards et al. (2011) 182 | DNA methylation | Lung tissues | TCF21 | 1 | PCR | 42 | 63 | 76 | 98 | NR |
Protein and Proteomic Markers | ||||||||||
Khan et al. (2004) | Protein | Serum | Serum amyloid A | 1 | ELISA | 50 | NR | 60 | 64 | NR |
Rahman et al. (2005) 183 | Proteomic profile | Bronchial biopsies | TMLS4, ACBP, CSTA, cytoC, MIF, ubiquitin, ACBP, Des-ubiquitin | 8 | MALDI-MS | 51 | 60 | 66 | 88 | 0.77 |
Patz et al. (2007) | Protein panel | Serum | CEA, RBP, α1-antitrypsin, SCCA | 4 | ELISA | 100 | 97 | 78 | 75 | NR |
Yildiz et al. (2007) | Proteomic profile | Serum | Proteomic signature | 7 features | MALDI-MS | 185 | 106 | 58 | 86 | 0.82 |
Farlow et al. (2010) | Protein panel | Serum | TNFα, CYFRA 21-1, IL-1ra, MMP-2, MCP-1, and sE selectin | 6 | Luminex (Austin, TX, USA) and ELISA | 133 | 88 | 99 | 95 | 0.98 |
Gessner et al. (2010) | Proteins (cytokines) | Exhaled breath condensate | VEGF, bFGF, angiogenin | 3 | Multiplex bead-based immunoassay | 75 | NR | 100 | 95 | 0.99 |
Ostroff et al. (2010) | Aptamers | Serum | Aptamer signature | 12 | Aptamers | 985 | 341 | 89 | 83 | 0.90 |
Joseph et al. (2012) | Protein | Plasma | Osteopontin velocity | 1 | ELISA | 43 | NR | 80 | 88 | 0.88 |
Lee et al. (2012) 184 | Proteomics | Serum | AIAT, CYFRA 21-1, IGF-1, RANTES, AFP | 5 | Luminex | 347 | 49 | 80.3 | 99.3 | 0.99 |
Higgins et al. (2012) | Protein | Plasma | Variant Ciz1 | 1 | Western blot | 170 | 160 | 95 | 74 | 0.90 |
Ajona et al. (2013) | Complement fragment | Plasma | C4d | 1 | Immunocytochemistry | 190 | NR | NR | NR | 0.73 |
Patz et al. (2013) | Protein panel, clinical features | Serum | CEA, α1-antitrypsin, SCCA, nodule size | 4 | ELISA | 509 | 399 | 80 | 89 | NR |
Li et al. (2013) | Protein panel | Serum | Protein panel | 13 | Multiple reaction monitoring mass spectrometry | 143 | 104 | 71 | 44 | NR |
Autoantibodies and Tumor-Associated Antigens | ||||||||||
Zhong et al. (2005) | Autoantibodies | Plasma | Phage peptides | 5 | Fluorescent protein microarray | 41 | 40 | 90 | 95 | 0.98 |
Zhong et al. (2006) | Autoantibodies | Serum | Phage peptides | 5 | ELISA | 46 | 56 | 91 | 91 | 0.99 |
Qiu et al. (2008) | Autoantibodies | Serum | Annexin I, 14-3-3 theta, LAMR1 | 3 | Protein array | NR | 170 | 51 | 82 | 0.73 |
Rom et al. (2010) | Tumor-associated antigens | Serum | Panel of tumor-associated antigens | 10 | ELISA | 194 | NR | 81 | 97 | 0.90 |
Wu et al. (2010) | Autoantibodies | Serum | Phage peptide clones | 6 | ELISA | 20 | 180 | 92 | 92 | 0.96 |
Boyle et al. (2011) | Autoantibodies | Serum | p53, NY-ESO-1, CAGE, GBU4-5, annexin 1, SOX2 | 6 | ELISA | 241 | 255 | 32 | 91 | 0.64 |
Lam et al. (2011) | Autoantibodies | Serum | p53, NY-ESO-1, CAGE, GBU4-5, annexin 1, SOX2 | 6 | ELISA | NR | 1376 | 39 | 87 | NR |
Chapman et al. (2012) | Autoantibodies | Serum | p53, NY-ESO-1, CAGE, GBU4-5, SOX2, HuD, and MAGE A4 | 7 | ELISA | 501 | 836 | 41 | 93 | NR |
Pedchenko et al. (2013) | Autoantibodies | Serum | Single-chain fragment variable antibodies to IgM autoantibodies | 6 | Fluorometric microvolume and homogeneous bridging MESA SCALE DISCOVERY | 30 | 43 | 80 | 87 | 0.88 |
Volatile Organic Compounds | ||||||||||
Phillips et al. (1999) | VOC | Exhaled breath | VOC profile | 22 | GC/MS | 108 | 100 | 81 | NR | |
Philips et al. (2003) | VOC | Exhaled breath | VOC profile | 9 | GC/MS | 178 | 108 | 85 | 80 | NR |
Poli et al. (2005) | VOC | Exhaled breath | VOC profile | 13 | GC/MS | 146 | 72 | 93 | NR | |
Mazzone et al. (2007) | VOC | Exhaled breath | VOC pattern | 36 sensors | Colorimetric sensor array | 100 | 43 | 73 | 72 | NR |
Bajtarevic et al. (2009) | VOC | Exhaled breath | VOC profile | 21 | Proton transfer reaction MS/solid-phase microextraction, GC/MS | 96 | NR | 71 | 100 | NR |
Ligor et al. (2009) | VOC | Exhaled breath | VOC profile | 8 | Solid-phase microextraction, GC/MS | 96 | NR | 51 | 100 | NR |
Fuchs et al. (2010) | VOC | Exhaled breath | Aldehydes: pentanal, hexanal, octanal, and nonanal | 4 | GC/MS | 36 | NR | 75 | 96 | NR |
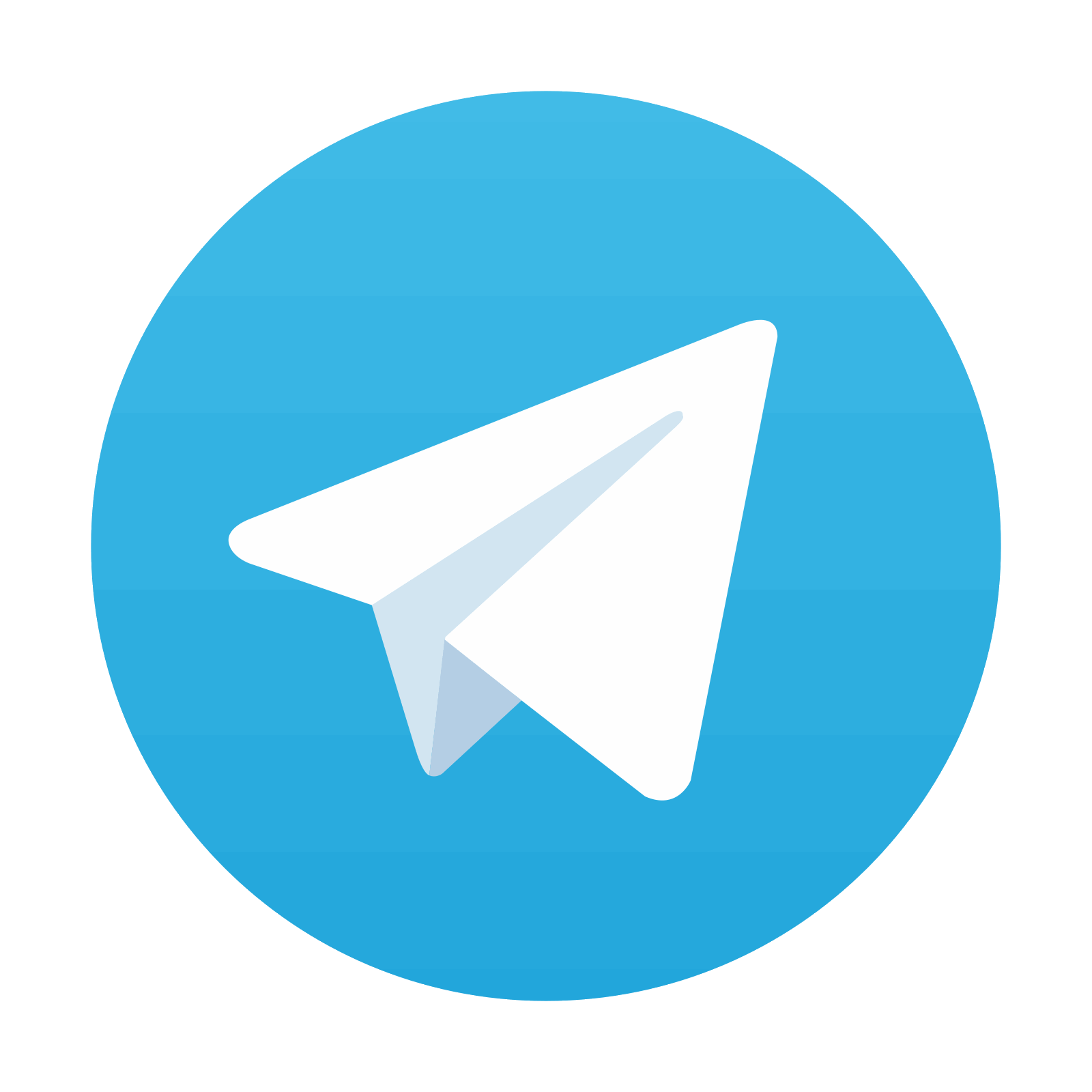
Stay updated, free articles. Join our Telegram channel

Full access? Get Clinical Tree
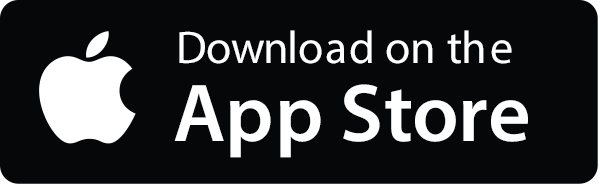

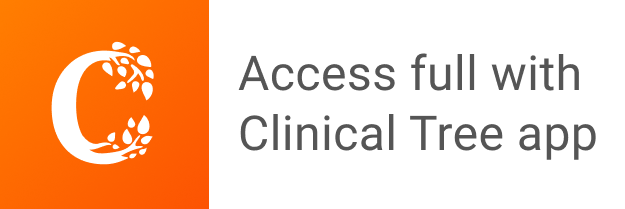