Fig. 17.1
Heritability of cancers in different sites based on the information available from twin studies
17.3 Immune Polymorphism
The role of immune system in defense against malignancies was proposed in the early 1990s by Paul Ehrlich [8]. So far this book, page by page, has tried to show the undeniable but complex interactions between the immune system and malignancies. This complex interaction mostly results from the manipulation of the immune system by cancer cells evoluting to prevent self-destruction [8]. Four phenomena contribute to the escape of malignant cells from the immunosurveillance:
1.
Immunoedition: Natural selection of malignant cells which are most successful in deceiving the immune system occurs by the pressure of the immune system itself. This pressure leads a somatic evolution toward variants proficient in immune escape in primary tumor lesions [9, 10].
2.
Tolerance induction and losing immunogenicity: The absence of co-stimulatory molecules, localization in natural environment of healthy cells and therefore absence of danger signals, losing human leukocyte antigen (HLA) class I molecules, and aberrant expression of immunomodulatory non-classical HLA class I antigen (Ag) can all induce tolerance in the immune system [9, 11, 12].
3.
Host immunodeficiency: Any deficiency in the immune status of individuals can predispose them to various malignancies.
In addition, once the immune escape occurred, the immune system can profoundly influence the prognosis, natural history, and response to different therapies either by direct effects on malignant cells or indirect effects on angiogenesis and inflammation [9, 11–13].
The immune system of each individual is subject to variability due to different environments, different diets and nutritional status, and different antigenic exposures and most importantly due to an uncountable number of polymorphisms in genes governing the immune system elements and cells [14, 15].
Genetic polymorphisms are defined as variations in human genome present in at least 1 % of the population [16]. These polymorphisms were beneficiary either in their cross talk with certain environmental factors alone or in combination with their associated polymorphisms, or they were at least neutral enough not to compromise the life of the individual bearing them; therefore they were not erased by the evolutionary pressure [14, 16, 17]. Immune response-associated genes are not an exception, and they have an uncountable number of polymorphisms [14]. For example, HLA region includes the most polymorphic genes in the human genome [14]. This high variety in immune-associated genes is a product of a long interaction with an environment consisting of numerous ever-evolving pathogens [14]. In this context, majority of polymorphisms had the chance to be beneficiary in defense against some pathogens [15, 18, 19].
Single nucleotide polymorphisms (SNP), variable number of tandem repeats (VNTRs) (a repeat unit includes 15–100 nucleotides) and microsatellites are three important types of polymorphisms [20].
SNP is defined as a difference in a single nucleotide in the DNA sequence and is estimated to account for 90 % of the human genome variations. Microsatellites, scattered through the genome with an average density of one in every 2,000 pb, are variable tandem repeats of 2–8 bp, most commonly CA dinucleotide, and their alleles are differentiated by the number of repeats (Fig. 17.2) [20, 21].
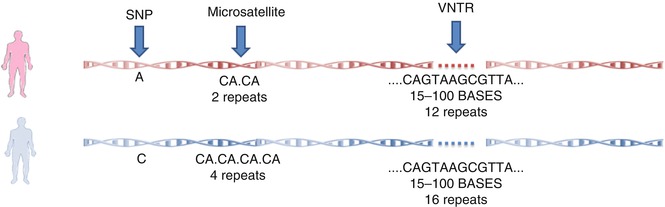
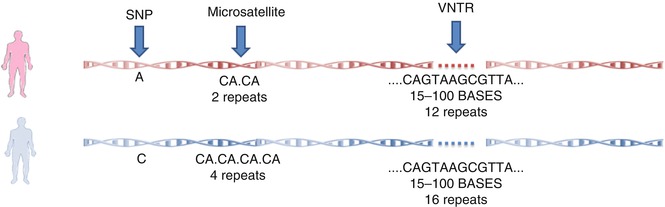
Fig. 17.2
Different types of polymorphisms in the human genome
Polymorphisms are able to change the immune function at several levels from expression patterns to posttranslation modifications:
1.
2.
3.
mRNAs splicing patterns can be modified by polymorphisms as a result of deletion of a splice site, creation of a new splice site, or modification of exon-splicing enhancers and silencers [24].
4.
MicroRNAs (miRNAs) are important elements in gene regulation with various actions. Their binding sites might be disrupted as a result of polymorphisms [24].
7.
8.
Some polymorphisms may change posttranslational modification (PTM) site and consequently influence posttranslational modifications [24].
17.4 Immunogenetics
17.4.1 Background
Immunogenetics, as the meeting point of two exciting fields of immunology and genetics, is a new but rapidly expanding field of science studying this immune polymorphism in order to understand the governance of genetics on the immune system [14, 27, 28].
Although the term “immunogenetics” was used earlier [29], the first milestone in the history of immunogenetics was coincident with the failed study of blood transfusion in 1952 [30]. This failure resulted in the discovery of HLA system [14, 31], which attracted the attention of biomedical researchers to interindividual differences in the immune system. From that point on, for decades, investigators tried to associate different complex diseases with various HLA types using serological methods [32, 33]. However, modern immunogenetics required more than one century of biomedical advances remarked by Mendel’s laws of heredity in 1865 [16, 34], discovery of chromosomes as the cellular basis of heredity in 1902, discovery of DNA double helix as the molecular basis of heredity in 1953 [35], decoding the genetic codes, and last but not least the completion of Human Genome Project in April 2003 [16, 36, 37]. Human Genome Project not only contributed to the discovery of genetic polymorphisms but also provided a infrastructure for other large-scale projects like International HapMap Project and “1,000 Genomes Project” [38]. Discovery of approximately 25–35 % of estimated nine to ten million SNPs is just one of the uncountable achievements of such projects [14, 37–39]. Genetic polymorphisms in the immune system contribute to a large part of the interindividual variation in immune response and today, immunogenetic studies have provided a vast knowledge of the effects of immune polymorphism on the host defense. However, just the estimation that there is one SNP per every 290 bp shows that there is much more to be brought to light [38, 39].
17.4.2 Immunogenetic Tools
Along with the concert of conceptual advancements, tools employed in this field have changed in order to gather immunogenetic information more accurately, in less time and less cost [14]. Twin studies recruit twins in order to remark the importance of genetic component in susceptibility to traits and diseases [16, 40]. The result of such studies provides a rough estimation of genetic contribution to interindividual differences in immune system by comparison of concordance rates of immune traits between monozygotic and dizygotic twins [16, 33, 40]. The higher the concordance difference is, the greater the heritability [7, 16].
Upon introduction of immune polymorphism, several association studies tried to show the contribution of specific genes using the candidate gene approach or hypothesis-driven approach [16, 41]. This approach includes looking into the differences between patients and controls in allele frequencies of SNPs in genes selected based on the known pathophysiologic pathways of the disease. These studies at first employed restriction enzymes to identify specific SNPs called restriction fragment length polymorphisms (RFLPs) in the restriction site of the enzyme [42]. This approach is also known as a reductionist approach, since studies employing this approach investigate only a few genes and polymorphisms at a time [16, 41, 43].
In the early 1990s, discovery of hundreds of informative microsatellites provided the possibility of a dramatic change in the approach of immunogenetic studies from a hypothesis-driven approach to positional approach [4, 16, 44]. In this approach, studies known as genome-wide association studies (GWAS) mainly aim to identify the genome regions bearing disease-associated genes and to localize causal genetic variants of disease as accurately as possible [44, 45]. Therefore, in this approach, new hypothesis are generated after making thousands of unbiased observations [4, 33, 41, 44]. They are especially helpful in order to find unexpected genes as representatives of unknown disease-related pathway [4, 16]. In mid-1990s, early GWASs employed informative microsatellite markers distributed evenly in the 23 chromosomes and investigated their aggregation in multi-case families and large pedigrees identified major susceptibility loci for complex diseases [4, 42, 44].
By introduction of linkage disequilibrium (LD) defined as the coinheritance of alleles of a block of neighboring SNPs; in 2002, the International HapMap Project, as a global movement, began to identify these blocks (known as haplotypes) and pattern of LD in the human genome [38, 39]. LD results in organization of genetic variation in haplotype blocks with strong LD separated by recombination hotspots [16, 39]. The information from this project provided the immunogenetic scientists with the most suitable SNPs for genotyping in order to indirectly gather as much as information about the genome variation of an individual [16, 46]. These SNPs, which are representative of a block of SNPs, are known as tagSNPs. The extent of LD in a region determines the number of tagSNPs required to cover a region. The lower the LD is in a region, the higher number of tagSNPs are needed and therefore the higher the cost of genotyping the region is [47]. Nowadays, availability of high-throughput gene technologies such as gene chips or microarrays has enabled investigators to genotype cost-effectively, rapidly, and almost effortless hundreds of thousands to millions of SNPs at the same time [4, 33, 41, 44]; therefore this approach is also known as “nonreductionist” approach [4]. These technological advancements were employed in community-based and large-scale GWASs in order to identify trait-associated regions with higher resolution. The results of such studies is a trait-associated SNP (TAS) as a representative of the true casual variant which might be each of the known and unknown variants in whole TAS block. The TAS block is defined as all known and unknown polymorphisms in strong LD with the tagSNP [4, 16, 48]. Therefore, LD along with technological advances turned SNPs, the most common and more importantly the most stable genetic variations in human DNA, into application [49].
However, there are major limitations in GWASs to be overcome.
1.
Generally, the genetic component of complex diseases originates from several major susceptibility loci and a component of as many as a dozen minor susceptibility loci known as polygenes (Fig. 17.3). These polygenes individually have small to medium impact on the overall genetic component; therefore, GWASs require a large study sample with homogenous ethnicity and phenotype to have enough high power to identify these polygenes [4, 21, 48, 50]. This is a major problem in immunogenetic studies of cancers as patients with cancers present with highly variable phenotypes. As a result, the odds ratio for each allele is typically below 1.5, and the P value should be less than 10−6 to show a significant association [6, 51].
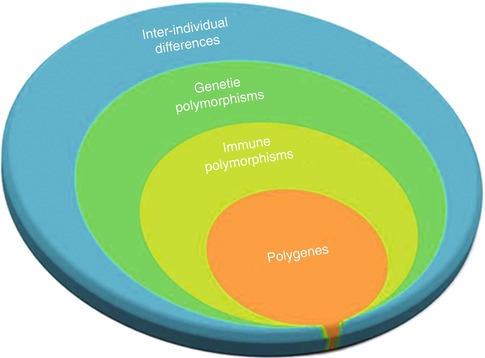
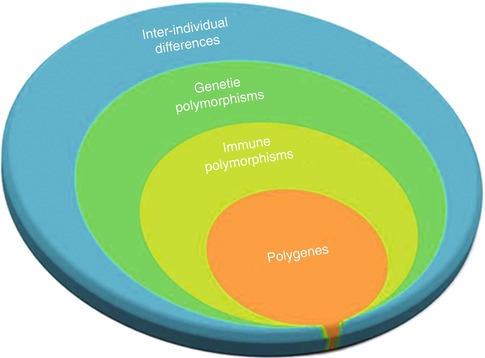
Fig. 17.3
Immune polymorphism component in inter-individual differences
2.
The genetic component and therefore effect of any risk allele decreases by increased exposure of populations to environmental risk factors which is the reason why some results could not be replicated in different populations [6]. For example, increased prevalence of acquired immune deficiency syndrome (AIDS) in some African populations predisposes population to different cancers disregarding their genetic background [52, 53]. This is also the case in regard to some extreme dietary patterns, smoking habits, and other environmental factors [54, 55].
3.
Some cancer susceptibility variants have nonadditive interactions with other genetic and environmental factors. It is possible that the effect of one variant depends on the presence of one or several specific alleles in another locus or even certain environmental risk factors. Therefore, such susceptibility variants can be detected only in GWASs with samples of patients with particular genetic and environmental background [6].
4.
At least 10 % of SNPs within a range of 1 kpb of hotspots are untaggable which means they don’t have any LD with tagSNPs [47]. The presence of these numerous untaggable SNPs always limits the power of GWASs in finding all possible genetic associations [39]. Therefore, GWASs should employ additional sequencing within known recombination hotspots [39].
5.
6.
The different LD, hotspots, and haplotype patterns in different populations might complicate replication studies in different populations [49]. For example, in some population, the causal variant may be separated from the associated TAS block by a hotspot.
7.
Sometimes the associated TAS block does not include a causative allele but an allele beneficiary for the affected individuals with the disease, and therefore the natural selection has selected them instead of those affected individuals without the allele [16].
8.
Population stratification is another source of bias in such studies as the association of the trait and TAS block may be due to an ancient branching of the population bearing both causal trait alleles and the TAS block; however, this bias can be minimized by the careful selection of the control group or by assessing population structure and correcting for it [16, 49, 56].
9.
If certain alleles are associated with a more aggressive disease and lower survival, they are less presented in patients and may not be detected as a susceptibility allele [57].
After identification of associated TAS blocks by GWASs, the actual functional variant in the associated TAS block can be found by further genetic association studies employing more accurate low-throughput technologies and other SNP markers in order to finely map the associated genes and alleles in the associated TAS block [44]. In these studies, allele frequencies of polymorphisms are compared in groups of cases and controls. However, results of such association studies are often contradictory due to the heterogeneous nature of the cancers, numerous gene–gene and gene–environment interactions [58, 59]. In addition, another source of discrepancy between these studies is the limitation in study design. For example, using hospital-based controls can result in a serious selection bias since polymorphisms under investigation might have association with the diseases that hospital-based controls may have [60, 61]. Moreover, some association studies failed to consider other genetic and environmental risk factors such as socioeconomic status, nutritional statues, smoking patterns, etc. [60]. Lacking such information may cause serious confounding bias [62]. Therefore, in order to get the most benefit from results of genetic association studies and to systematize their findings, employing meta-analyses as a powerful statistical method is essential [26, 63]. Meta-analysis by pooling the results of old studies allows us to see the whole picture of the effect of a certain polymorphism [26].
Regardless of interspecies differences, there are similarities in cancer development between humans and rodents, and therefore mouse studies are a complementary tool for genetic association studies within human population [6, 64, 65]. Numerous genetically engineered mouse (GEM) models provide a simplified model of various cancers with controllable genetic and environmental background in which the effects of a unique polymorphism on the malignancy can be studied [6, 66].
Exact mechanism of action of polymorphisms can be identified using different bioinformatic tools and in vitro studies [24]. Numerous bioinformatic online and offline tools are available which can predict the effect of polymorphisms by considering amino acid biophysical properties, active site residues, metal and lipid binding sites of gene product, TFBSs, splice sites and its regulatory motifs, miRNA binding sites, and PTM sites (Table 17.1) [24]. However, bioinformatics is limited by the extent of our knowledge [22, 24].
Table 17.1
A small example of different bioinformatics tool
Title | Address | Description |
---|---|---|
dbSNP | A database for SNP information | |
Ensembl | A database for genome information, comparative genomics, variation, and regulatory data | |
HapMap consortium | A database for haplotype blocks | |
SNPper | Online tool available for SNP analysis | |
SNP3D | Online tool available for functional analysis of SNPs based on structure and sequence analysis | |
SNPeffect | A database for phenotyping human SNPs and for finding information regarding SNPs effect on structure stability functional sites, structural features, and PTM sites | |
MutDB | Online database for human variation data with protein structural information and other functionally relevant information | |
dbSNP | A database for SNP information | |
Ensembl | A database for genome information, comparative genomics, variation, and regulatory data | |
HapMap consortium | A database for haplotype blocks | |
SNPper | Online tool available for SNP analysis | |
SNP3D | Online tool available for functional analysis of SNPs based on structure and sequence analysis | |
SNPeffect | A database for phenotyping human SNPs and for finding information regarding SNPs effect on structure stability functional sites, structural features, and PTM sites | |
MutDB | Online database for human variation data with protein structural information and other functionally relevant information |
Different in vitro methods are developed to identify functional polymorphisms. The most important ones are reporter gene assay and electrophoretic mobility shift assay (EMSA) (Figs. 17.4 and 17.5) [22]. The reporter gene assay employs a reporter gene with a quantifiable product and clones the promoter of interest in its upstream [22, 67, 68]. Therefore, quantification of reporter gene product can provide information about the promoter strength [22, 67, 68]. On the other hand, EMSA can measure the effect of different polymorphisms on the affinity of TFBS sequence for different transcription factors. In these studies, double-stranded oligonucleotide containing the polymorphism of interest is mixed with nuclear extract with various transcription factors [22, 69, 70]. Higher affinity for these factors results in the formation of more protein–DNA complex resulting in retardation of mobility in electrophoresis [22, 69, 70].
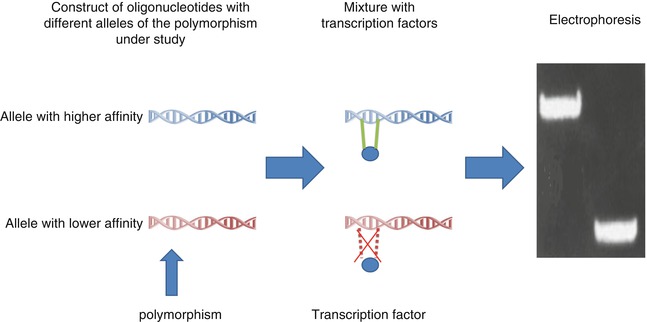
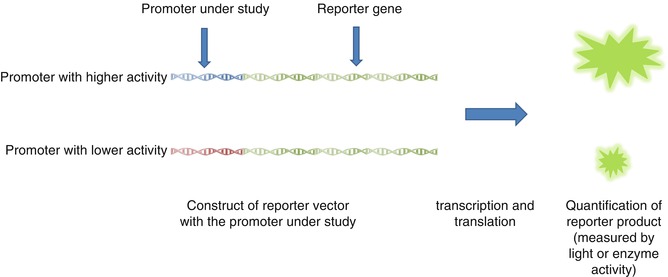
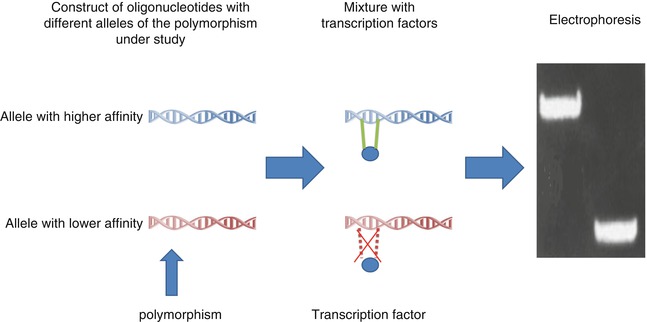
Fig. 17.4
EMSA, an in vitro experiment to measure binding affinities of different TFBS for transcription factors
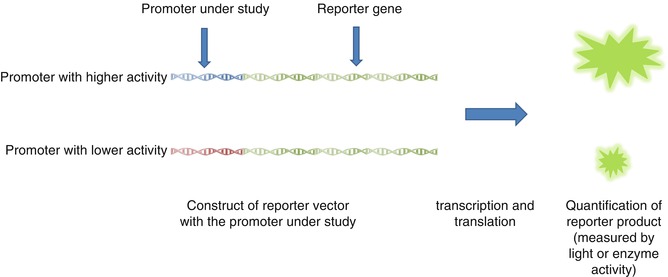
Fig. 17.5
Reporter gene assay, an in vitro tool to measure strength of different promoters
The results from immunogenetic studies should always be interpreted with consideration of information from immunogenomics and immunoproteomics [33]. It should be noted that information from each type of study i.e., GWASs, genetic association studies, in vitro and mouse studies and bioinformatics, are just pieces of the complex puzzle of immunogenetics and cancer. No individual method is precise enough to see the final picture (Fig. 17.6).
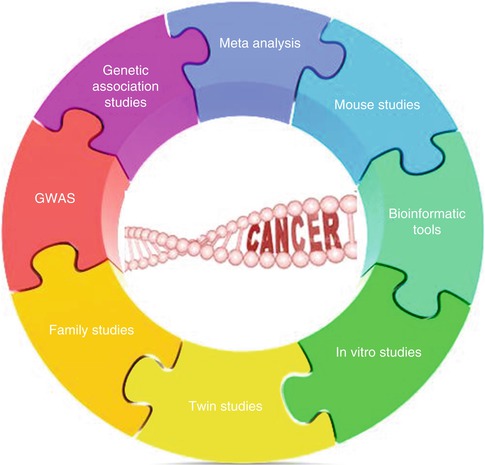
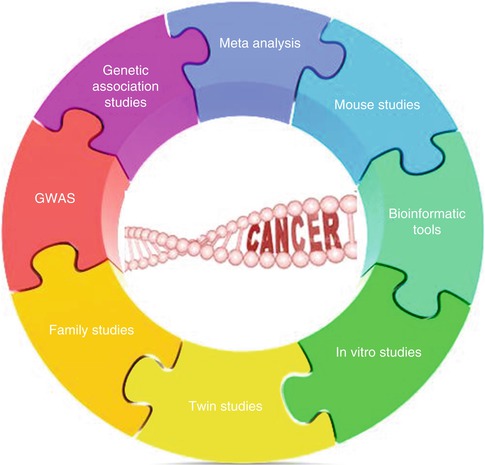
Fig. 17.6
Different methods in immunogenetic studies are pieces of a complex puzzle
17.5 Immunogenetics: A Champion in Fighting the Losing Battle Against Cancer
The application of immunogenetics in cancer is more than promising. Some variations in immune polymorphism reduce the immune capacity in clearing either malignant transformations or cancer-inducing infectious agents and predispose bearing individuals to various cancers as exaggerated in case of most primary immunodeficiency diseases [4, 16, 19, 33]. Although each individual variant has a little informative potential for clinical application, understanding their interactions and therefore their cumulative effect is of high clinical importance [6].
Immunogenetic studies not only can help clinicians in risk assessment of individuals for susceptibility to certain cancers in order to employ preventive strategies but also may open new windows for treatment [4, 16, 19, 33, 48, 71–73]. GWASs might result in the identification of unexpected genes which in turn result in identification of new pathways in pathophysiology of cancers [48]. These new pathways not only provide a broader insight into how and why of the cancers but also may suggest new molecular targets for prevention and immunopharmacology and immunotherapy [4, 16, 33, 42, 48]. Keeping in mind that immune system provides the only antineoplastic reaction completely specific to cancer cells, it is vital to completely understand the genetic factors governing the immune system–cancer interactions and employ this knowledge in eliminating the cancers [4, 74]. In addition, this knowledge might begin a post-genomic era in individualized medicine [4, 33]. The presence of some variants in immune associated genes might affect the success or failure in applying a particular therapy and immunogenetic information provides a way to predict toxicity and clinical effectiveness of different immune-based therapies [4, 14, 20, 33]. Therefore, employing the knowledge from immune polymorphism in prediction of treatment outcome may justify the application of an expensive partly effective treatment option [4, 14, 33, 75].
17.6 Human Leukocyte Antigen
17.6.1 Background
Human leukocyte antigens are specialized elements of the immune system in recognition of self from non-self. HLA is responsible for presenting Ags to T cells and therefore serves as a door to the specific immune system. HLA class 1 Ags are on the surface of almost all nucleated cells and generally present processed endogenous antigens to CD8+ cells [13, 76]. Presentation of abnormal Ags derived from intracellular pathogens or malignant transformations potentially initiate a cytotoxic T lymphocyte (CTL) response and consequently target cell lysis [77]. By their interaction with killer cell immunoglobulin-like receptors (KIRs) on the surface of natural killer (NK) cells, HLA class 1 antigens regulate lytic activity of NK cells. Therefore, any change in either in expression or structure of HLA class 1 profoundly influence T and NK cell-mediated immunity [10].
On the other hand, HLA class 2 Ags are exclusively expressed on the surface of professional antigen-presenting cells (APC) and present processed exogenous Ags to T helper (Th) cells. Following presentation of unfamiliar Ags and in the presence of appropriate costimulatory molecules, Th cells activate effector elements of the immune system [13, 77].
Both classes of Ags comprise an intracellular, transmembrane, and an extracellular part which includes highly polymorphic antigen binding groove. From the evolutionary view, this high variety favors the chance of heterozygosity and consequently Ag presenting potential for each individual along with a significant increase in the general repertoire of the whole specie for Ag presentation [14, 77].
17.6.2 Genes Behind HLA
HLA loci, located in 6p21.3 region, occupy only a small part of major histocompatibility complex (MHC) genetic system which is home to at least 220 genes [78, 79] (Fig. 17.7). MHC is divided into three classes of genes distributed from centromere to telomere. Class 2 with 0.9 mb is the nearest one to the centromere; class I with 1·9 Mb is near telomere, and class 3 with 0·7 Mb lies in between [80]. The first two classes encode for HLA class 1 and 2 and the third class consists of a group of genes encoding some members of the complement system, some cytokines like tumor necrosis factor alpha (TNF-α), heat shock proteins (HSP) and an enzyme called 21-OH hydroxylase [31, 80].
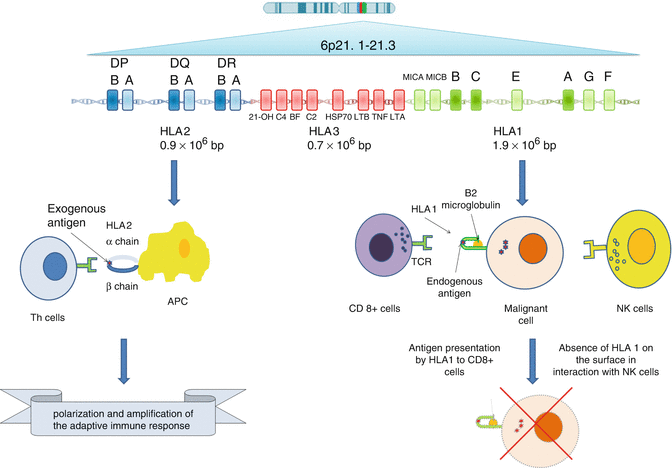
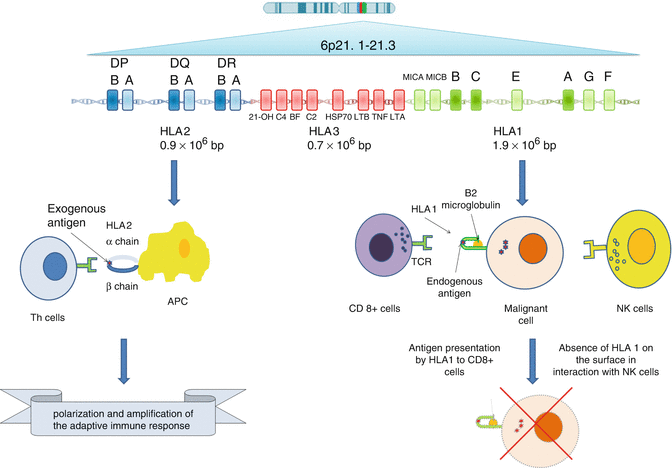
Fig. 17.7
HLA as the gate of adaptive immunity from genes to function
In class 1, there are three highly polymorphic classic genes known as HLA-A, HLA-B and HLA-C, while there are numbers of nonclassical genes known as HLA-E, HLA-F and HLA-G [81, 82]. Class 1 genes encode the highly polymorphic heavy chain of HLA class 1 (45 kDa) which later joins the non-polymorphic B2 microglobulin encoded by chromosome 15 [81, 82]. Classic genes consist of eight exons, but the most important exons are exons 2 and 3 encoding for peptide binding groove. Other exons encode for transmembrane region and cytoplasmic tail [31, 83]. Beside these highly polymorphic classic HLA class 1 genes, there are three other HLA genes in class 1 known as HLA-E, HLA-F and HLA-G which are more conserved. Most probably, they are not involved in Ag presentation but in interaction with more conserved parts of the immune system. For example, HLA-E, which is minimally polymorphic, regulates cytotoxic activity of NK cells by interacting with CD94/NKG2 lectin-like receptors. The conservation within this gene guarantees that there is a constant protection for healthy cells in most people and provides a minimum safeguard for autoimmunity [32, 84, 85]. Some of them like HLA-G are expressed on trophoblastic cells and placental chorionic endothelium and induce immune tolerance during pregnancy [81, 86–90].
Class 2 consists of classic genes called DP, DQ and DR and nonclassic genes known as DM and DO. Classic genes encode for one highly polymorphic beta chain (26–28 kDa) and a less polymorphic alpha chain (33–35 kDa) [80]. Therefore, there are six classic D genes in this region. Genes for alpha chain consist of five exons, while beta chains are encoded by six exons. The exons 2 and 3 in both set of genes are responsible for encoding peptide binding domains [31].
HLA class 1 and 2 genes are the most polymorphic genes in the human genome with 2,365, 3,005 and 1,848 alleles for HLA-A, HLA-B and HLA-C, respectively, and 2,156 alleles for class 2 genes (based on IMGT/HLA database, release 3.13 on July 2013) [91]. This high polymorphism is mostly clustered in several hypervariable blocks in exons 2 and 3 which are responsible for encoding antigen binding groove. Therefore, a unique combination of sequence motifs in these hypervariable regions determine each allele [13]. This genetic structure is accompanied by high LD not only between HLA genes but also non-HLA genes constituting extended haplotypes [92]. The majority of polymorphisms in hypervariable regions result in amino acid substitutions in peptidebinding grooves, which in turn dramatically changes Ag binding affinity of the final product [13]; on the other hand, variants in noncoding regions, influence transcription, translation, and splicing and thereby expression levels [77].
Nowadays, with a few exceptions, HLA alleles are named by six or even eight digits. The first two digits are representative of the serological family the allele belongs to, while the third and fourth digits distinguish between different sequences affecting amino acid sequences. The next two digits are identifiers of synonymous polymorphisms, and seventh and eighth digits are used to distinguish intronic polymorphisms or ones located into untranslated regions [93].
17.6.3 From Polymorphisms to Clinic
HLAs are involved in cancer immunity and therefore in susceptibility and prognosis mainly by presenting certain Ags known as tumor-associated antigens (TAA). TAA are the first contact of malignant cells with adaptive immunity. Since introduction of the first TAA in melanoma patients in 1991, a broad heterogeneous group of Ags were discovered and associated with different malignancies. This heterogeneous group can be divided in to four classes of Ags [8, 94]:
1.
Cancer–testis Ags are a result of epigenetic alterations leading to reactivation of silence genes. One of the famous examples is Ags from MAGE family. These Ags are not exclusive to just one type of cancer. The reason for this naming is that they are normally expressed in MHC-negative testicular germ cells and placental trophoblasts.
2.
Differentiation Ags are normally expressed in the tissue of origin of the tumor, like Melan-A, and tyrosinase in melanomas
3.
Unique tumor Ags are products of mutated tumor suppressor genes and oncogenes like abnormal product of RAS or p53. Fusion proteins as a result of chromosomal aberrations are also included in this group.
4.
Infectious tumor Ags are expressed by oncogenic viruses associated with some malignancies. The examples are latent membrane proteins 1 and 2 (LMP-1 and LMP-2) in Epstein-Barr Virus (EBV)-associated Hodgkin lymphoma (HL) and E6 and E7 associated with human papillomavirus (HPV)-associated cervical cancer.
Nowadays, hundreds of HLA association studies prove that HLA alleles are important elements in predisposition to cancer. Seven mechanisms are suggested for complex relationship of HLA genotypes and susceptibility, prognosis, recurrence, and clinical response to immunotherapy and tumor vaccines:
1.
Efficiency in TAA presentation: One of the major factors in Ag presenting ability of different HLA is the affinity of their Ag binding grooves to different epitopes. This affinity is highly dependent on the amino acid sequence in the hypervariable regions. Even one change in this sequence due to polymorphisms profoundly influences binding affinities to TAAs and Ags used in tumor vaccines and therefore susceptibility prognosis and response to tumor vaccines [32, 82, 95–97]. For instance, HLA-A*0207 is associated with susceptibility to EBV-associated lymphoma in East Asian population, while HLA-A*0201 is a protective factor; however, this huge difference at the clinical level is a result of a single amino acid change (Y99 to C) at the protein level [98, 99].
2.
3.
Efficiency in inducing immune response to infectious agents: Antigen binding abilities of different HLA alleles influence immune reaction to infectious agents associated with malignant transformation. For example, EBV is frequently emphasized as an important environmental factor in the pathogenesis of HL and nasopharyngeal carcinoma (NPC) [101]. Latent membrane protein-1 (LMP-1) and Epstein-Barr virus nuclear antigen (EBNA-4 and EBNA-6) proteins produced during latent infection by EBV, are efficiently presented by A*0201 and A*1101 respectively [83]. Therefore, these alleles can induce a strong immune response which consequently results in resolving the infection and lower chance of malignant transformation. Another example is the protective effect of DQB1*0301 allele on hepatitis C virus (HCV) infection, HCV-associated liver cirrhosis, and HCV-associated Hepatocellular carcinoma (HCC). This allele can efficiently present majority of immunodominant epitopes of HCV [102].
4.
Change in HLA expression patterns: In some malignancies like melanoma, Burkitt’s lymphoma, and carcinoma of the cervix and lung, HLA expression and Ag processing machinery are disturbed in order to prevent TAA presentation and consequently immune recognition of malignant cells. This mechanism is one of the major pathways for the immune escape of tumoral cells [10]. Some polymorphisms within the noncoding regions can influence expression levels [32]. In addition, some HLA alleles are specifically lost during malignant transformation [103]. Loss of HLA-A2 in colorectal cancers, breast cancer, and cervical cancer or lower expression levels of HLA-DR4 and HLA-DR6 in melanoma is a good example for these phenomena [104, 105]. On the contrary, some alleles like HLA-B*4405 are not dependent on some elements of the regular Ag processing machinery like transporter associated with Ag presentation (TAP) and therefore, can present antigens without susceptibility to viral-induced diminished TAP function [106].
5.
Increased susceptibility to chronic infections or autoimmunity: Some HLA haplotypes and alleles are associated with various chronic inflammatory diseases which in turn predispose individuals to various cancers [75, 107]. Excess growth factors and prolonged proliferation in the background of chronic destruction increase the risk of malignant transformation [107].
In addition, chronic immune stimulation of B cells and prolonged and repeated DNA double-strand breaks associated with somatic hypermutation (SHM) and class switch recombination (CSR) significantly increase the chance of malignant transformation, and therefore, autoimmunity and chronic infection are important risk factors for some hematological malignancies like non-Hodgkin lymphoma (NHL) [107]. In these cases, HLA alleles can affect the extent of immune reaction and stimulation of B cells [107]. For instance, HLA–DRB1*0301, HLA–B*0801 HLA–DRB1*0101, and HLA–DRB1*0401, the susceptibility alleles of NHL is associated with autoimmune diseases such as systemic lupus erythematosus (SLE), rheumatoid arthritis (RA), Sjögren’s syndrome, and celiac disease [97, 102, 108]. The more prominent example is the paradoxical relationship of DQB1*0301 with HCV infection and HCV-related B-cell lymphoma. While DQB1*0301 is associated with a better immunologic control of HCV and a self-limiting infection, it is a susceptibility factor for HCV-related NHL. In this case, efficient presentation of viral antigens by DQB1*0301 in the context of persistent HCV infection results in CD4+-dependent chronic stimulation of B cells [102].
6.
Sensitivity to mutation: It is suggested that some HLA alleles are more susceptible to mutations like rearrangements of the DNA material and crossover. Such dramatic alterations might influence the function of oncogenes or tumor suppressors in the proximity of HLA genes. An example of such an oncogene is Waf1/p21 gene, located in 6p21.1 [100].
7.
Linkage disequilibrium: LD with non-HLA genes of class 3 or even nonclassical HLA in the form of extended haplotypes can justify some of the founded associations. Some classical genes are in LD with certain HLA-G and HLA-E alleles which are both involved in suppression of NK cell-mediated immunity against tumors [73]. LD with non-HLA genes like TNF-α, in context with extended haplotype, can influence the relationship between toxicity of immunotherapy and HLA alleles. For example, high TNF-α increases the IL-2 toxicity in patients with melanoma [109, 110].
17.6.4 HLA Typing and HLA Association Studies: Lessons from the Past
HLA has a history as long as immmunogenetics itself. An observation of transfusion failures in 1952 paved the road to the discovery of the first HLA allele by Jean Dausset in 1958 [111]. Since 1958, there was a continuous international effort in order to share experimental data and HLA typing technologies, identify new HLA alleles and serotypes, and uncover the role of HLA system in pathogenesis of numerous diseases [31]. The result of such effort was the identification of over 9,500 alleles for HLA class 1 and 2 over a short period of four decades [31]. Along with the discovery of new alleles, the first nomenclature committee was held in 1987 followed by several nomenclature committees to unify the nomenclature and classification [31].
Early studies employed low-resolution serological methods which detected HLA on T cells or B cells [112]. Although these serological methods were subject to huge development in detection methods from complement-dependent cytotoxicity test to ELISA method, flow cytometry, and Luminex technique, the real breakthrough in HLA association studies was the introduction of PCR and high resolution DNA-based typing methods [31]. This technology allowed not only detection of high HLA polymorphisms with higher sensitivity and specificity but also the detection of new alleles with more flexibility by simply adding new probes to the old panels [113]. Nowadays, the old DNA-based method employing PCR-RFLP has been replaced by more rapid tests [113]. Generally, they either identify PCR products containing hypervariable regions by hybridization with sequence-specific probes (SSO) or employ sequence-specific primers (SSP) to identify variants as part of PCR process itself [13, 31, 114]. The latter was extensively used back in mid-1990 [13, 31, 114]. Even though aberrant typing as a sign of new allele can be followed by direct DNA sequencing, both methods are ineffective in case there is a new allele [13]. Later this limitation was overcome by polymerase chain reaction-sequence-based typing which can directly detect the sequence of alleles. In this method which is based on dye terminator chemistry, dye bounded 2,3 dideoxynucleotides are used as substrates for PCR process. Randomly addition of labeled dideoxynucleotides, and consequently, a stop in elongation of DNA chain result in the development of numerous DNA fragments with different sizes. These DNA fragments can easily be separated by capillary electrophoresis, and the ending dideoxynucleotides can be identified by specific fluorescence emitted from the related dye.
In parallel, huge efforts were made to understand the role of these alleles in etiology and natural history of several diseases. In oncology, the first association was found in HL in 1967 [32]. This finding triggered a series of HLA association studies on different cancers worldwide. The fruit of this global movement was finding association between HLA alleles and susceptibility to several hematological malignancy including HL, NHL, childhood acute lymphoblastic leukemia, Kaposi’s sarcoma, chronic myeloid leukemia (CML), and also non-hematological malignancies including nasopharyngeal carcinoma, thyroid cancer, renal cell carcinoma (RCC), cervical cancer, and both melanoma and non-melanoma skin cancers [13, 115]. Moreover, investigations on natural history of cancers showed relationship of several alleles from both classes with mortality in ovarian cancer, non-small cell lung carcinoma, head and neck squamous carcinoma, and local recurrence in melanoma [73, 96, 100]. Several studies showed importance of HLA context in the outcome of immunotherapy and tumor vaccines in melanoma, RCC, cervical carcinoma and CML [73, 95, 110, 116].
Although the result of such studies was inconsistent in some cases, most studies pointed to the undeniable role of HLA polymorphism in susceptibility, prognosis, natural history, and response to immunotherapy in different cancers [32]. These past experiences emphasize that a prestigious HLA association study is a complex art rather than a simple case-control study and several factors should be considered in interpreting their results. In this regard, results of meta-analysis of these association studies are more reliable (Table 17.2).
Table 17.2
Significant results from published meta-analysis of HLA associations with cancers
Alleles | Cancer site | Total number of cases | Total number of controls | OR ± 95 % CI | Population included | Reference |
---|---|---|---|---|---|---|
DQB1*03 | Hepatocellular carcinoma | 398 | 593 | 0.65 (0.48–0.89) | China, Italy, Spain, Egypt | Xin et al. [117] |
DQB 1*02 | Hepatocellular carcinoma | 398 | 593 | 1.78 (1.05–3.03) | China, Italy, Spain, Egypt | Xin et al. [117] |
DQB1*0502 | Hepatocellular carcinoma | 257 | 349 | 1.82 (1.14–2.92) | China, Spain | Xin et al. [117] |
DQB1*0602 | Hepatocellular carcinoma | 173 | 226 | 0.58 (0.36–0.95) | China, Spain | Xin et al. [117] |
HLA-DRB1*07 | Hepatocellular carcinoma | 281 | 466 | 1.65 (1.08–2.51) | China, Italy, Spain, Egypt | Lin et al. [118] |
156 | 224 | 2.1 (1.06–4.14) | China | Lin et al. [118] | ||
125 | 242 | 1.41 (0.83–2.42) | Italy, Spain, Egypt | Lin et al. [118] | ||
HLA-DRB1*12 | Hepatocellular carcinoma | 281 | 516 | 1.59 (1.09–2.32) | China, Italy, Spain, Thailand | Lin et al. [118] |
206 | 324 | 1.73 (1.17–2.57) | China, Taiwan | Lin et al. [118] | ||
75 | 192 | 0.3 (0.04–2.47) | Spain, Italy | Lin et al. [118] | ||
HLA-DRB1*15 | Hepatocellular carcinoma | 281 | 466 | 1.7 (0.8–3.59) | China, Italy, Spain, Egypt | Lin et al. [118] |
156 | 224 | 3.22 (1.63–6.37) | China | Lin et al. [118] | ||
125 | 242 | 0.8 (0.34–1.89) | Spain, Egypt, Italy | Lin et al. [118] | ||
HLA-DRB1* 0701 | Cervical squamous cell carcinoma | 1,445 | 2,206 | 1.59 (1.09–2.35) | Iran, USA, England, Sweden, France, Brazil | Yang et al. [119] |
1,083 | 1,248 | 1.29 (1.02–1.63) | Caucasians | Yang et al. [119] | ||
HLA-DRB1* 1301 | Cervical squamous cell carcinoma | 2,743 | 3,904 | 0.63 (0.52–0.78) | Iran, USA, England, Sweden, France, Brazil | Yang et al. [119] |
2,013 | 2,360 | 0.61 (0.48–0.77) | Caucasians | Yang et al. [119] | ||
HLA-DRB1* 1302 | Cervical squamous cell carcinoma | 1,877 | 2,966 | 0.49 (0.36–0.68) | Iran, USA, England, Sweden, France, Brazil | Yang et al. [119] |
2,013 | 2,360 | 0.75 (0.57–0.98) | Caucasians | Yang et al. [119] | ||
HLA-DRB1* 1501 | Cervical squamous cell carcinoma | 1,915 | 2,628 | 1.42 (1.23–1.65) | Iran, USA, England, Sweden, France, Brazil | Yang et al. [119] |
2,191 | 2,628 | 1.22 (1.01–1.47) | Yang et al. [119] | |||
HLA-DRB1* 1502 | Cervical squamous cell carcinoma | 1,424 | 2,184 | 1.87 (1.08–3.26) | Iran, USA, England, Sweden, France, Brazil | Yang et al. [119] |
HLA-DRB1* 1503 | Cervical squamous cell carcinoma | 432 | 894 | 3.4 (1.69–6.87) | Iran, USA, England, Sweden, France, Brazil | Yang et al. [119] |
HLA-DRB1* 1602 | Cervical squamous cell carcinoma | 1,314 | 2,234 | 0.61 (0.38–0.98) | Iran, USA, England, Sweden, France, Brazil | Yang et al. [119] |
HLA-DRB1* 0403 | Cervical squamous cell carcinoma | 1,796 | 2,050 | 2.05 (1.02–4.12) | Caucasians | Yang et al. [119] |
HLA-DRB1* 0405 | Cervical squamous cell carcinoma | 1,496 | 1,700 | 6.13 (1.03–36.33) | Caucasians | Yang et al. [119] |
HLA-DRB1* 0407 | Cervical squamous cell carcinoma | 1,796 | 2,050 | 2.71 (1.11–6.61) | Caucasians | Yang et al. [119] |
HLA-DRB1* 0901 | Cervical squamous cell carcinoma | 1,796 | 2,050 | 0.58 (0.34–0.99) | Caucasians | Yang et al. [119] |
17.6.5 Typing Methods
Indeed, immunogenetic studies are deeply influenced by technological advances. Low-resolution serologic HLA typing was one of the major limitations in early studies [83]. Serologic typing is only enabled to identify the family of alleles. This family often comprises a heterogeneous group of alleles with different affinities and different potential for Ag presentation. Since distribution of alleles belonging to the same serotype is different in various populations, such studies often obtained conflicting results in different populations. One of the best historical examples is HLA association studies in nasopharyngeal carcinoma (NPC).
NPC, as an epithelial carcinoma of the head and neck origin, was one of the main focuses of early HLA association studies. Early serological studies showed an association between HLA-A2 and NPC-in Chinese population, while studies in Caucasians found HLA-A2 as a protective allele for both NPC and EBV-associated HLA [106, 120–124]. Later, higher-resolution studies showed HLA-A*02:07, a common allele in Chinese population but rare among Caucasians, as the main risk factor, while HLA-A*02:01, a common allele in Caucasians, was shown to be the actual protective factor in this population [125, 126]. It is possible that future studies employing higher-resolution methods reveal even new causal variants within the current associations.
17.6.6 Environmental Factors
Various environmental and genetic factors play roles behind scenario of cancer, and malignant transformation is the result of a complex interaction between these factors. It is often the case that certain genetic factors need certain environmental factors to play their role in pathogenesis of cancer. The role of environmental factors in HLA association studies is more prominent in virus-associated malignancies like HL, NPC and cervical cancer. Each virus has different strains with different Ags and the prevalence of these strains is not the same in different populations. Each strain is best presented by certain HLA alleles. Therefore, one HLA allele efficient for presenting Ags of one population’s prevalent strain may not present Ags of another population’s prevalent strain efficiently [83]. Such a phenomenon might be extended to other environmental factors like virus prevalence, viral load, diet, cigarette smoking, and socioeconomic status, all of which are highly dependent on the population under study [74, 127]. For instance, pathogenesis of cervical cancer is dependent on persistent infection with high-risk human papillomavirus (HPV) and this risk factor itself is highly related to socioeconomic status, sexual relationship, and prevalence of high risk variants in the region [127, 128].
17.6.7 Linkage Disequilibrium
MHC region is home to more than 200 genes beside classic HLA genes. Due to the low recombination rates, these genes are often in strong linkage disequilibrium together [78]. This strong LD can complicate finding the actual causal allele. The problem gets worse when the causal allele is an unknown allele in strong LD with the associated allele. This limitation can be overcome by whole genome sequencing (WGS) of the region in close proximity of the associated allele [101]. One example is the association of NPC with HLA-A*0207 and HLA-B*4601 which are in strong LD. In this case, either allele, both of them, or even a third allele in LD with both of them might influence the pathogenesis of NPC [126].
Some studies reported extraordinary LD in MHC region between alleles from one class and alleles of other classes and even non-HLA genes. This extraordinary haplotypes are known as extended haplotypes [83]. Thus, in interpreting results of HLA association studies or design of one, non-HLA genes such as the transporter associated with Ag processing (TAP) MHC class I chain-related A (MIC-A), heat shock proteins (HSP), and TNF-α which are located nearby or within the classic HLA genes should be considered [78, 83]. These extended haplotypes are especially of importance in immunogenetic studies of cancers, since numerous elements of the immune system are in the front line of defense against cancer.
For instance, the ancestral haplotype 8.1 (AH 8.1: HLA-A*01-B*08-Cw*07-DRB1*03-TNF-G308A), in which HLA alleles are in LD with TNF-α, is the most frequent extended MHC haplotype in Caucasian populations [109]. Primarily, this extended haplotype was associated with clinical course of NHL [75, 109]; however, later studies showed that polymorphism in TNF-α gene has a more prominent effect in this association compared to Cw*07 and DRB1*03 alleles [8, 75]. In this case, polymorphisms in TNF-α promoter influence TNF-α expression levels. TNF-α level consequently affects the extent of immune activation upon tumor challenge. In addition, increased TNF-α impairs Ag presentation potential of APCs and by its effect on cytokine profile results in a bias toward Th2 immune responses [75]. All these factors can contribute to the exacerbation of systemic symptoms, anemia, hypoalbuminemia, and poor outcome [8].
Another example is the association of HLA-A*03 and chronic myeloid leukemia (CML) [78]. A translocation between t(9;22)(q34;q11) creating a truncated chromosome 22 known as Philadelphia chromosome is present in majority of patients with CML [129]. Depending on the precise location of the fusion, different fusion proteins are encoded. Keeping this in mind and the absence of costimulatory molecules on CML cells, it is improbable that the association of HLA-A*03 is due to its efficiency in presenting fusion proteins and its ability to induce an effective immune response [78]. However, this allele is in with the C282Y mutation of the hemochromatosis gene, a susceptibility marker for CML [78].
In some cases, an optimal immune response is dependent on optimal Ag presentation by both HLA classes and the presence of certain alleles in non-HLA genes. An absence of one of these optimal alleles may result in anergy and immune escape. In some populations, these alleles might be in LD in form of an unknown extended haplotype, while in other populations this haplotype might be absent [57]. One of such associations has been reported between cervical squamous cell carcinoma and multi-locus haplotype of B*4402-Cw*0501-DRB1*0401-DQB1*0301 [57].
17.7 The Cytokine Network
17.7.1 Background
Cytokines are a group of soluble regulatory factors by which the immune system controls and modulates different activities of its cells. Each cytokine triggers certain cascade of events in their target cells by binding to their receptors and activating intracellular signal transduction pathway [14, 20]. Cytokine network is responsible for coordination of effector actions of different elements of the immune system, as well as the differentiation and proliferation of different immune cells. In addition, secretion of antibodies and inflammation is tightly regulated by complex interaction between these cytokines [13, 23, 26].
Chronic inflammation, by inducing chronic tissue damage and compensatory cell proliferation, is a considered a major promoter of malignant transformations. As an example, nitric oxide, produced during inflammation, might damage DNA structure in different tumor suppressor genes and oncogenes [130]. Therefore, any dysregulation in cytokine network can result in excessive production of tumor-inducing factors, DNA damage, angiogenesis, and dysplasia and consequent development of various inflammatory diseases including different cancers [26, 131]. Cytokine network is a determinant factor in the development of metastasis and natural history of cancers [26]. In some cancers, malignant cells can manipulate cytokine network in order to escape immunosurveillance or promote their own proliferation [130, 132]. In addition, cytokine network can influence the outcome and toxicity of different immunotherapy methods [13, 20, 133]. Several cancers including hepatocellular carcinoma (HCC), oral squamous cell carcinoma, melanoma, the gastric, pancreatic, and prostate cancer were associated with high levels of certain proinflammatory or antiinflammatory cytokines [26].
Cytokine levels are not the same in all individuals. Interindividual differences in cytokine levels in both baseline and stimulated phases are a result of both genetic and environmental factors [133]. Since there is no an intracellular storage for cytokines, their secretion is dependent on the transcriptional and translational rates of their genes [14, 26]. Not surprisingly, genes responsible for encoding cytokines and their receptors are relatively polymorphic [13, 20, 23]. Several polymorphisms in their gene can affect their expression, structure, and activity [20, 23, 26, 130, 134]. Most of these polymorphisms are in non-coding regions including promoter or intronic sequences and exonic regions are usually highly conserved [13, 14]. So far, numerous genetic association studies have been suggested as associations of these SNPs with various cancers in different populations. However, results of such studies were often inconsistent, and the reported associations varied not only in different populations but also in different cancers and even in their different subtypes [131]. Therefore, a meta-analysis of these studies can show some more conclusive evidence of these associations.
In addition to polymorphisms of cytokine genes, there are other polymorphic elements such as various transcription factors and cytokine-specific receptors which are involved in actions of cytokine network [20, 26]. For instance, polymorphisms in the NF-κB nuclear factor-kappa B gene, one of the most important transcription factors, can result in extensive changes in the cytokine network by altering transcription of TNF-a, IL-1, IL-6, and IL-8 [20]. Although the exact roles of these polymorphisms in tumor immunology are less clear, the relevance of this role is becoming more and more apparent in recent years [20].
17.7.2 Interleukin-1 Superfamily
IL-1α and IL-1β and their antagonist IL-1Ra are members of this superfamily with pleiotropic effects on inflammation, immunity, and hemopoietic system. High levels of IL-1 are found in tumor sites, however IL-1 family plays an ambivalent role in tumor immunity. IL-1 induces cytokine secretion from T cells to potentiate the differentiation and function of immunosurveillance cells. On the other hand, IL-1 induces the expression of adhesion molecules, matrix metalloproteinases, growth factors, and angiogenic factors and promotes invasiveness and metastasis of malignant cells [135, 136].
17.7.2.1 Interleukin-1 α
IL-1α is encoded by seven exons of a gene located in 2q14. Variant−889 C>T (rs1800587) is one of the common promoter variants of IL-1α gene (Table 17.3). Although, the promoter containing T allele has been shown to result in a marginally higher level of expression, at the protein level, T allele was associated with significantly increased IL-1α levels which could not be justified by only different expression patterns. Further studies showed that this SNP has high LD with an exonic SNP in +4845 G>T (rs17561) resulting in substitution of alanine with serine at the position of 114 which results in more efficient process of pre- IL-1α comparing to Ala114 and consequently higher release of IL-1α [23].
17.7.2.2 Interleukin-1 β
High levels of IL-1β have been shown to be associated with increased risk of most human cancers and also poor prognosis in cancer patients [130, 132, 139]. IL-1β is encoded by a 7.5 kb gene with seven exons located on 2q14. Its expression is regulated by two distal and proximal promoter elements [140, 141]. So far, several polymorphisms have been identified in this gene. −598T>C (rs16944) and −31 C>T (rs1143627) are two common variants in the promoter region, and +3954 C>T (rs1143634) is a common synonymous polymorphism in coding region of IL-1β gene (Table 17.4) [26].
In northern and western European ancestry (CEU), −598T>C (rs16944) and −31 C>T (rs1143627) had strong LD (r 2 = 0.94) [26, 132]. In vivo, −598C/−31T haplotype has been associated with higher IL-1β levels in the lungs and gastric mucosa. It is suggested that −31 C>T (rs1143627) is the causal variant of this haplotype [23, 141]. In the same line, in vitro studies like luciferase reporter assay showed higher expression of luciferase gene with promoter containing T allele in −31 C>T (rs1143627) [23]. Results of EMSA studies suggested that this higher expression is a result of higher affinity for several transcription factors as a result of a change in a TATA-box motif [23].
T allele in rs1143634 was associated with increased IL-1β secretion and several inflammatory diseases [132]. However, no evidence on the functionality of +3954 C>T (rs1143634) is available, and it seems that +3954 C>T (rs1143634) is just a marker for a functional polymorphism such as −31 T>C (rs1143627) [23, 26].
One recent meta-analysis of 81 case-control studies with 19,547 patients with HCC, gastric, lung, blood, cervical, esophageal, prostate, breast, and skin cancers and 23,935 controls showed that, overall, −598T>C (rs16944) has no significant association with cancers [132], while another meta-analysis of 26 studies with 8,083 patients with cancer and 9,183 controls showed a significant association of +3954 C>T (rs1143634) with increased risk of cancers in a dominant model which is in accordance with the results of another metaanalysis of 33 studies (Table 17.5) [132, 145].
Table 17.5
Significant results from published meta-analysis of associations of IL-1β polymorphisms with cancers
Alleles | Cancer site | Total number of cases | Total number of controls | Analysis type | OR ± 95 % CI | Population included | Reference |
---|---|---|---|---|---|---|---|
rs16944 | Gastric cancer | 2,041 | 2,441 | TT + CT vs. CC | 1.23 (1.09–1.37) | Italy, Japan, China, Korea, Portugal, UK, mixed Asian | Vincenzi et al. [142] |
Cervical cancer | 836 | 980 | TT vs. CC | 1.74 (1.28–2.36) | Egypt, Korea, India, China | Xu et al. [132] | |
CT vs. CC | 1.71 (1.32–2.23) | ||||||
TT + CT vs. CC | 1.74 (1.35–2.23) | ||||||
Hepatocellular carcinoma | 890 | 821 | CT vs. CC | 0.75 (0.60–0.94) | Japan, Taiwan, Thailand | Xu et al. [132] | |
TT + CT vs. CC | 0.68 (0.47–0.99) | ||||||
Blood cancers | 3,839 | 3,762 | CC + CT vs. TT | 1.19 (1.04–1.37) | Italy, Spain, Germany, USA, Canada, Greece | Xu et al. [132] | |
rs1143627 | Lung cancer | 3,435 | 4,719 | TT + TC vs. CC | 1.23 (1.06–1.43) | China, Italy, mixed European, Denmark | Peng et al. [143] |
Gastric cancer | 1,535 | 2,585 | TT + TC vs. CC | 1.16 (1.01–1.33) | Korea, Mexico, China, Brazil, Italy, USA | Vincenzi et al. [142] | |
Hepatocellular carcinoma | 1,039 | 1,588 | CC + CT vs. TT | 1.31 (1.09–1.57) | Japan, Taiwan, Morocco | Jin et al. [144] | |
rs1143634 | Malignancy | 8,083 | 9,183 | TT + CT vs. CC | 1.15 (1.01–1.30) | Sweden, Poland, China, UK, Germany, Tunisia, Costa Rica, Oman, USA, Greece, Netherlands, Norway, Japan | Xu et al. [132] |
Gastric cancer | 2,359 | 3,613 | CT vs. CC | 1.16 (1.03–1.32) | USA, China, UK, Germany, Italy, Japan, India, Sweden, Oman | Zhang et al. [145] | |
Oral cancer | 346 | 417 | CT vs. CC | 0.65 (0.45–0.94) | Greece, China | Zhang et al. [145] | |
TT + CT vs. CC | 0.69 (0.49–0.98) |
A meta-analysis of studies on associations between IL-1β gene polymorphisms and gastric cancer published from January 2000 to December 2009 (including 18 studies with 4,111 controls and 3,295 cases for −598T>C (rs16944), 21 studies with 5,883 controls and 3,786 cases for −31 T>C (rs1143627) polymorphism, 10 studies with 3,610 controls and 1,559 cases for +3954 C>T (rs1143634)) showed significantly increased risk of cancer in individuals with IL-1β −598T allele. In stratified analysis for different ethnicities, such an association was present in Caucasians but not in Asians or in Hispanics. This study also showed such an association for intestinal-subtype and noncardia gastric cancer [146, 147]. However, this study didn’t show any significant associations between gastric cancer risk and −31 T>C (rs1143627) and +3954 C>T (rs1143634) [146]. Older studies conducted on 2005 and 2007 more or less showed such pattern for this SNP [142, 147]. However, a meta-analysis of five studies published up to September 2008 showed association of +3954 C>T (rs1143634) and gastric cancer risk in Chinese and Japanese population [148].
Another systematic review evaluating associations of HCC with polymorphisms of IL-1 gene (reported up to September 2010) and a meta-analysis of 1,279 patients with lung cancer and 2,248 controls failed to support any significant increased risk for −598T>C (rs16944) and −31 C>T (rs1143627) [143, 149].
17.7.2.3 Interleukin-1Ra (IL-1Ra)
IL-1RA has antiinflammatory properties by competing with IL-1 cytokines in binding to their receptors. This cytokine is encoded by IL-1RN gene located on 2q14.2. Its transcript may contain six, five, or four exons [23, 130]. There is an 86-bp variable number tandem repeat (VNTR) in intron 2 of this gene [23]. The short allele of this VNTR contain only two repeats (IL-1RN*2), while long alleles may have three to six repeats (IL-1RN L) [58, 146]. The more prevalent allele containing four repeats is named IL1RN*1 [150]. In vitro and in vivo studies have shown extensive associations of this variant with the members of IL-1 superfamily. IL-1RN*2 was associated with not only higher IL-1RA levels but also enhanced IL-1β production and decreased IL-1α production [151]. However, the final result of IL-1RN*2 was decreased IL-1RA/IL-1β ratio, followed by prolonged proinflammatory immune response [23]. Although, intronic VNTR contains potential binding sites for an interferon-α silencer, an interferon-β silencer, and an acute-phase response element, all leading to its functional importance, these associations are suggested to be a result of LD with other variants [140, 152]. Some authors suggested that the enhancing effect of IL-1RN*2 on IL-1RA levels is dependent on the presence of −511T allele or the absence of +3954T in IL-1β [23].
A meta-analysis of 71 case-control studies (including 37 studies on gastric cancer, six studies on HCC, four on cervical cancer, four on breast cancer, four on lung cancer, and 16 studies on other cancers) with 14,854 cases and 19,337 controls showed that overall carriers of IL-1RN*2 are significantly more susceptible to cancer (Table 17.6) [145].
Table 17.6
Significant results from published meta-analysis of associations of IL-1RN VNTR with cancers
Cancer site | Total number of cases | Total number of controls | Analysis type | OR ± 95 % CI | Population included | Reference |
---|---|---|---|---|---|---|
Malignancy | 14,854 | 19,337 | 22 vs. LL | 1.37 (1.07–1.75) | 40 studies of Asian descendents, 29 of Caucasian descendents, and two with mixed ethnicity | Zhang et al. [145] |
2 L vs. LL | 1.19 (1.07–1.32) | |||||
22 + 2 L vs. LL | 1.25 (1.12–1.41) | |||||
2 vs. L | 1.23 (1.10–1.38) | |||||
Breast cancer | 1,145 | 1,102 | 2 L vs. LL | 0.74 (0.58–0.93) | Japan, Germany, Korea, India | Zhang et al. [145] |
22 + 2 L vs. LL | 0.78 (0.62–0.97) | |||||
Gastric cancer | 3,209 | 4,856 | 2 L vs. LL | 1.22 (1.05–1.41) | Portugal, China, Germany, Brazil, Taiwan, Thailand, UK, Italy | Zhang et al. [145] |
22 + 2 L vs. LL | 1.25 (1.09–1.43) | |||||
2 vs. L | 1.20 (1.05–1.38) | |||||
3,418 | 5,789 | 22 + 2 L vs. LL | 1.26 (1.06–1.51) | Arab, Brazil, Netherland, Korea, USA, China, Italy, Mexico, South Korea, Germany, Taiwan, Portugal, Poland | Xue et al. [146] |
17.7.3 Interleukin-4
Interleukin-4 (IL-4) is a pleiotropic cytokine with major roles in regulation of humoral immunity by its various effects on production of several other cytokines and dedifferentiation of B cells and promoting expression of class II MHC Ags [26, 130]. It also has potent antitumor activity against various tumors by its inhibitory effect on the growth of tumor cells and its growth stimulatory effect on lymphocytes [153, 154].
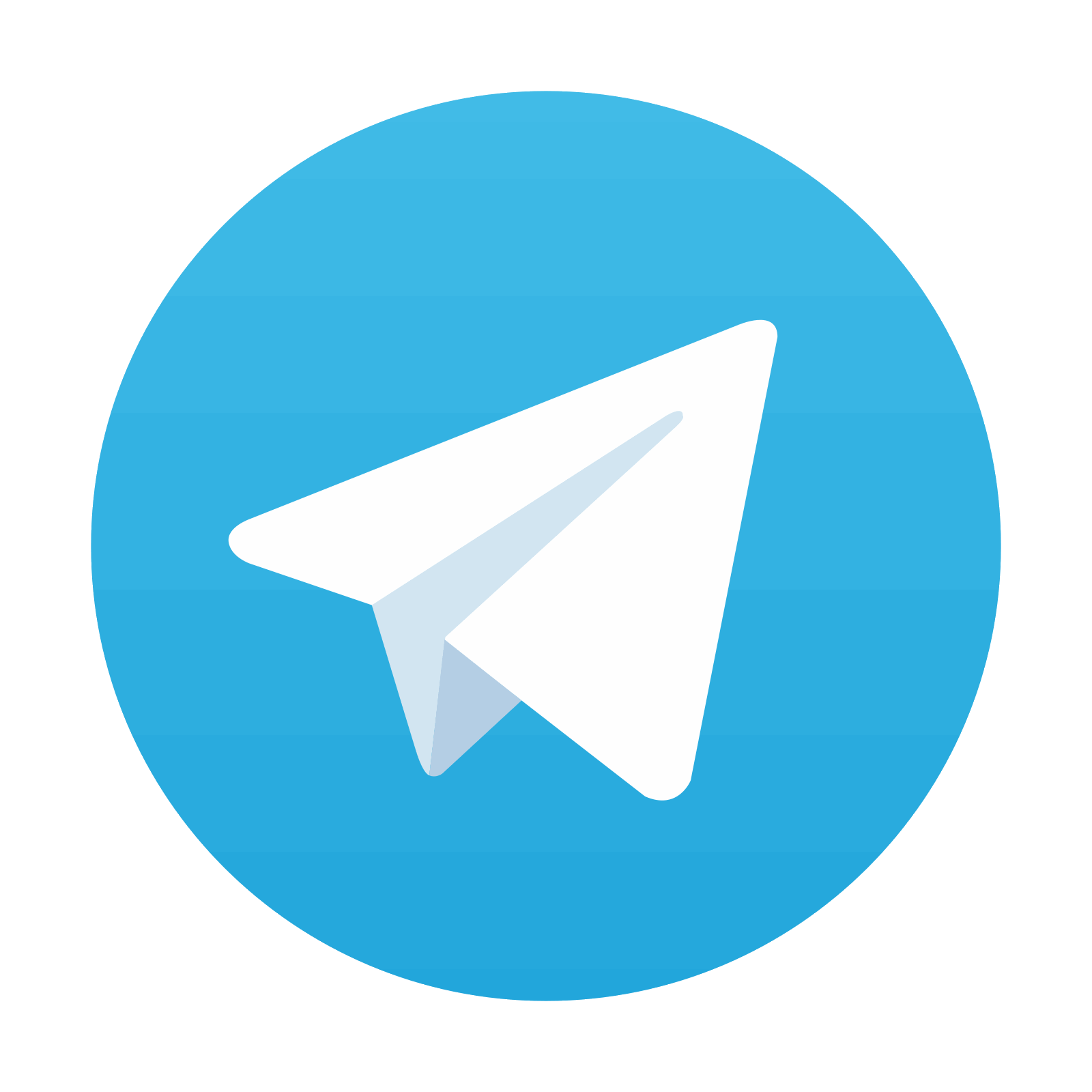
Stay updated, free articles. Join our Telegram channel

Full access? Get Clinical Tree
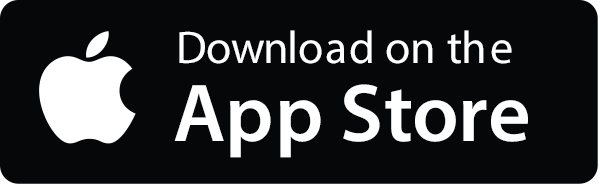
