Health services research
Michaela A. Dinan PhD Bradford R. Hirsch MD, MBA
Amy Abernethy MD, PhD
Overview
Health services research (HSR) describes a diverse group of research strategies and fields that seek to evaluate the impact of health care on patients and populations and has been a critical component of understanding the use, outcomes, and costs associated with oncology care since its inception over 100 years ago. In modern times, physicians and researchers often tout “bench to bedside” innovations as the goal of biomedical research. However, patients, physicians, and health care systems in the real world are subjected to a host of factors that impact patient care before, during, and after an intervention occurs at the bedside. Although HSR is a complex and evolving concept, a basic understanding of the key principles of HSR is needed to accurately assess, quantify, and optimize the real–world impact of progress in oncology today and in the future.
Introduction—what is health services research?
Health services research (HSR) comprises a diverse group of research strategies and fields that seek to evaluate the impact of health care on patients and populations. While the focus of the included disciplines may differ, there are similarities which allow us to group them together under the umbrella of HSR. In each case the same basic methodology applies: A treatment or intervention is examined in the context of an outcome of interest in order to better understand and guide clinical practice.
According to the Institute of Medicine (IOM), HSR focuses on the investigation of three major aspects of health care including (1) access to care, (2) the quality of care, and (3) the cost of health care in order to inform health care consumers about their best options for medical treatment and/or prevention.1 The IOM has also developed a list of the major issues that health services researchers are studying today (Table 1).
Table 1 IOM list of major health services research topics
|
HSR is truly a multidisciplinary field. Health services researchers, biostatisticians, economists, and clinicians are all examples of critical and necessary players in the HSR arena. In oncology, the clinical expertise of medical, surgical, and radiation oncologists as well as pathologists and radiologist are all needed to develop clinically relevant questions that will lead to improvements in patient care. Close collaboration between methodologists and clinicians is essential to ensure both the scientific validity and clinical significance of HSR studies.
The goals of HSR in oncology are many. While some research focuses on the investigation of diagnostics and treatments to prolong survival, other seeks to improve quality of life (QOL), inform decision making, improve access to care, ensure guideline concordant care, or examine the economic impact of care for cancer patients. In addition to the wide range of these and other potential outcomes, HSR in oncology has several stakeholders with vested interests in the results including patients and their families, physicians and other medical staff, additional providers and payers of health care, industry, and policy makers that must be considered in any effort to make advances in oncology.
The significance of health services research in cancer
HSR in oncology is the principal means by which we frame the scope of cancer and attempt to improve cancer care delivery in the real world. Discoveries made in the controlled environments of laboratories and clinical trials may or may not translate when taken out of the context of a select group of trial participants and applied to the larger population of patients. That is to say, most clinical trials are focused on the efficacy of a specified intervention or the ability of an intervention to provide benefit under tightly controlled circumstances. In contrast, HSR focuses on the effectiveness of these interventions, which describes the ability of an intervention to provide benefit under real-world conditions. The goal of HSR is to improve the effectiveness of interventions as they are disseminated into broader, more diverse populations and promote the health of all members of the population.
HSR in oncology has been used to provide estimates of the scope of cancer on a national scale and as a prediction of how it will change in the future. For example, HSR using the population-based Surveillance, Epidemiology, and End Results (SEER) registry provides the estimate that in 2014, there were an estimated 1,665,540 patients newly diagnosed with cancer and 585,720 people who died from their cancer. As the U.S. population continues to age, this number is expected to increase. For example, in 2010, the total direct estimated cost of cancer care in the United States was $124.5 billion. After only reflecting demographic changes, by 2020, this cost has been projected to increase to $157.8 billion.2 These costs will likely be much higher in reality due to the adoption of expensive innovative therapies, the widespread diffusion of advanced technologies without supporting evidence, inappropriate use (either over or under) of existing treatments, and patient demands and unrealistic expectations are additional areas of concern. HSR provides a framework to consider how to mitigate the impact of this surge in need on current medical resources and infrastructure.
Disciplines within health services research
In practice, HSR is often separated into multiple distinct fields of discipline—health economics, epidemiology, qualitative research, implementation research. The distinctions have developed over time based on the data requirements, methodologies, and expertise needed to answer questions meaningfully. Despite these potential differences, there is considerable overlap and a common goal of inferring causality and informing practice. Outcomes research revolves around the identification of a treatment or exposure of interest and a relevant outcome (i.e., the impact of a new treatment on survival). Health economists focus on health care costs and resource utilization, epidemiologists on naturally occurring exposures and patterns, and health services researchers on questions that examine exposures or treatments within the health care system—either directly or indirectly.
An overview of health services research study designs
HSR can be conducted with a variety of different study designs. They may analyze primary data (collected prospectively as part of a clinical study) or secondary data (collected for some other purpose, such as for hospital billing, and are then repurposed). Clinical trial study designs are more familiar, where a specific intervention is imposed on a study group. Randomized Clinical Trials (RCTs) are considered the gold standard of clinical research because the intervention or exposure of interest is randomly designated across study participants. RCTs are often blinded, where the physician is not aware of a subjects assignment to a given study arm, or even double blinded, where neither the physician nor the subject is aware of the assignment. These design elements help to avoid any biases that may occur by ensuring the random distribution of interventions across subjects.
Observational analyses do not assign interventions among subjects and are most often retrospective in nature. Instead, researchers examine the relationship between exposures or events of interest that occurred and the associated effects or outcomes. The studies can be descriptive or analytic in their design. Descriptive studies include case reports or case series, ecologic studies, and cross-sectional studies, while analytic studies include longitudinal cohort and case–control studies. Descriptive analyses are used to generate hypotheses, whereas analytic analyses are used to test specific hypotheses about the association between a specific exposure and outcome.
Types of secondary data sources relevant to HSR in oncology
A common understanding among health services researchers is that the study is only as good as the data. There are limitations to every data source so health services researchers must use multiple approaches to be able to address a question of interest. Primary data quality will depend largely on the instrument design and any logistic constraints involved in data collection. Secondary data are often limited by the purpose for which they were originally collected. Billing data (aka claims data), are collected as documentation of services provided and payments received; studies using these data must account for the inherent limitations when designing an analytic plan.
There are a few commonly encountered types of oncology databases available for secondary analyses in HSR which include clinical registries, administrative datasets, clinical trial databases, and more recently an increasing body of data from aggregated electronic medical records (EMRs) (described later in the chapter). A thorough understanding of the strengths and limitations of the dataset is critical for one to conduct a valid analysis (Table 2). Clinical registries may collect relevant data on patients with an incident cancer diagnosis within a specific population, health care system, network, or region. Strengths of clinical registries include high-quality short-term exposure and outcome data, clinically rich data specific to a disease of interest, collection of potential confounders, and the potential for large sample sizes. The principal weakness of registry data are a lack of randomization to key exposures of interest, poor intermediate and long-term follow-up and outcomes, and lack of data regarding unrelated disease states such as cardiovascular disease.
Table 2 Strengths and weaknesses of commonly encountered large secondary databases
Data Source | Examples | Strengths | Weaknesses |
Disease registries | SEER NCDB EMR data-generated |
|
|
Administrative data | CMS (Medicare) VA (Veterans Affairs) Kaiser Permanente |
|
|
Clinical trials | Collaborative group studies Industry trials Institutional trials |
|
|
Administrative datasets consist of information that is routinely collected within the operations of a given health care entity, such as an insurer (i.e, Medicare), integrated health care systems (i.e., Veterans Affairs Hospitals), or large health care organizations (i.e., Kaiser Permanente). The strength of administrative data lie in their broad coverage of tens or even hundreds of millions of lives, the efficiency of data availability, and the long-term follow-up available through the use of patient identifiers. In addition, administrative data are representative of the population at large, while data collected within a clinical trial are clinically rich but limited to a carefully pre-selected, smaller population because of cost and scientific considerations. The weaknesses of administrative data stem from their collection for purposes of billing and tracking health care resource utilization, often limiting the granularity of available information. Information such as dates of claims and types of procedures performed are highly accurate. However, information that is not critical to billing, such as cancer stage, are not always captured.
Different types of data can be linked together to help offset limitations of any single data set. For example, registry and clinical trial data, which often suffer from a lack of long-term follow-up and incompleteness can be supplemented with administrative claims data (such as Medicare) to augment the available follow-up and survival data.
Data is universally important across all study designs but the type of data is highly specific to the type of study being conducted. Further discussion of specific datasets and approaches will be presented subsequently in the context of different methdologies. However, two cancer-specific registries, SEER and the National Cancer Data Base (NCDB) that are particularly important to know as they play central roles in HSR in oncology are worth describing in detail.
Surveillance, Epidemiology, and End Results
The SEER tumor registry program collects detailed clinical and pathological information on 28% of cancer patients in the United States, aggregating it from participating registries that are representative of the national patient population. Limitations of these data include a lack of detailed information on treatments, providers, and cost. To address these needs, linkages were pursued to generate a richer set of variables. This resulted in a collaboration between the National Cancer Institute (NCI) and the Centers for Medicare and Medicaid Services (CMS) to make a SEER-Medicare dataset available that adds Medicare administrative data and health care claims. As CMS provides health insurance to over 97% of Americans aged 65 and older, this allows a detailed assessment of health care utilization and costs among SEER patients. The SEER-Medicare data have been used to examine cancer care quality around issues of racial disparities, physician and hospital characteristics, screening, treatment choices (i.e, surgery, chemotherapy, radiation), complications, costs, and mortality.3 While powerful, a core limitation of the SEER-Medicare dataset is that it only includes those 65 years of age and older.
National Cancer Data Base (NCDB)
Another oncology-specific dataset which is widely used is the NCDB which is a joint project of the American Cancer Society and the American College of Surgeons’ Commission on Cancer (CoC). The NCDB was established in 1989 as a nationwide, hospital-based, comprehensive clinical surveillance data set. The NCDB obtains data from more than 1,500 CoC-accredited facilities which captures 30 million patient records and 70% of all newly diagnosed cancer cases.4 The key strength of the NCDB is its sheer size and capture of the majority of cancers diagnosed within the United States. It is well posed to study nationwide patterns of care, adoption of novel surgical procedures, and the approach to rare cancers. As a surgical data set, it contains excellent data on the details of a patient’s surgery and survival. However, some aspects of the data are not reliably coded; there are limited details regarding chemotherapy, radiation or noncancer related health; and there is no information on relapse, recurrence, or subsequent treatments.
Statistical analyses in health services research
An in depth review of statistics is well beyond the scope of this chapter, so we will focus on commonly used techniques that encompass the vast majority of HSR. In HSR, statistical analyses are used to analyze data from a population of interest in order to either describe or learn something (i.e., make an inference) about the general state of affairs in that population. Descriptive statistics simply describe or summarize a set of data, by providing means, frequencies, counts, plots, or other depictions. Inferential or analytic statistics use more complex methodologies to attempt to draw generalizable inferences from a set of data, such as whether or not two groups differ from one another by more than what would reasonably be expected by chance.
Multivariable versus univariate analyses
In HSR, one of the most common distinctions made in practice is the use of statistics to investigate single variables vs. multiple variables. Single variable or “univariate” analyses are used to summarize or describe the properties of a single variable, such as what percent of a population has ever smoked. Multivariable or multivariate analyses (in practice these are used interchangeably) are more complex and seek to explain the relationship between a single variable of interest and multiple other variables at the same time.
Often univariate versus multivariable analyses are referred to as “unadjusted” and “adjusted,” as the multivariable approach “adjusts” for potential confounding by control variables. Such control variables might include clinically relevant variables associated with the outcome of interest such as age, stage, or grade and must include any variables that might be associated with both the exposure and outcome of interest in order to fully control for potential confounding. In a study that performs both “unadjusted” and “adjusted” analyses, the results from the adjusted (or multivariable) analyses are more likely to reflect the true relationship of interest, as these models have at least attempted to adjust for factors that might otherwise explain the observed relationship. Variables are often inter-related in HSR and a failure to account for these relationships can result in erroneous conclusions. The key question that HSR seeks to answer is often how a certain treatment, condition, or exposure impacts a specific outcome of interest after controlling for all other relevant factors.
Multivariable analyses
One of the most widely used methods in multivariable analysis in HSR is that of multivariable regression analysis. The term “regression” was original coined in the late 1800s by Francis Galton to describe the tendency of tall or short pea plants (outliers) to produce offspring that were more similar to or “regressed” towards the overall population height.5 In its simplest form, this is essentially drawing a simple straight line through a plot of two variables that shows the mean or average of one variable as a function of the other. Significant mathematical and computational progress since has allowed for the routine use of far more complicated models that are able to predict the value of a so-called “dependent” variable whose value is predicted depending on the values of multiple “independent” variables. Such models come in many forms and have been refined and developed to be able to predict, model, or describe any combination of different variable types. Most often in HSR, continuous data are modeled using linear regression, dichotomous data using logistic regression (from its use of binary logic values, i.e., zero or one to describe the dependent variable), and survival data using cox proportional hazard models (survival variables include an event such as death or relapse vs. no death or relapse combined with the time to that event). Linear regressions will predict the mean value of the dependent variable as a function of each independent variable (i.e., average weight as a function of sex and height), logistic regressions will estimate odds ratios (OR) associated with a particular exposure, and cox proportional hazards models yield hazard ratios (HR). Other frequently used multivariable statistical analyses include variations of the χ2 test (chi-squared), such as the Cochran–Mantel–Haenszel test,6, 7 which essentially performs the χ2 test for an association between two categorical variables while controlling for other potentially confounding variables.
One of the benefits of multivariable regression is the ability to capture “interaction effects,” or an interaction between two independent or explanatory variables on the dependent variable of interest. Interaction effects can capture cases where a variable might only impact the outcome of treatment within a subset of patients. For example, a meta-analysis of three randomized controlled trials in nonsmall cell lung cancer found a significant interaction between squamous tumor histology, receipt of pemetrexed, and a lack of treatment response.8 This meta-analysis confirmed that the drug pemetrexed is associated with improved outcomes, but only in patients with nonsquamous cell histology. As a result, pemetrexed is now the drug of choice in nonsquamous NSCLC.
Bias and confounders
Perhaps the single most important concept in HSR is the idea of bias or confounding. A confounder is anything that confuses, obscures, or otherwise mixes the effect of the characteristic of interest with others. The word “confound” comes from the latin for “pour together,”9 and so literally describes a situation where the observed association is mixed or confused by a confounder. An example of confounding can be demonstrated using a hypothetical retrospective study of patients with metastatic disease, in which patients who have less aggressive disease and receive surgery for their metastatic disease are observed to live longer. In this case, the observed association of surgery with prolonged survival is being confused with, or “confounded” by, the association of nonaggressive disease with both surgery and survival.
Bias is also used to describe confounding, but can be more descriptive by additionally specifying the direction of the observed erroneous association. Bias is a related term from the old French biais, meaning “slant,”9 and describes the situation where the observed association is biased, or unfairly slanted, to yield either a false positive (can bias the estimate of an association upward or downward) or false negative (biased towards the null hypothesis) association between two variables.
One specific example of bias in the HSR oncology literature comes from analyses of survival in patients with nonsmall cell lung cancer (NSCLC). Several studies had observed that receipt of PET scans are associated with improved survival, and erroneously concluded that receipt of PET improves survival. However, upon closer examination, we know that PET scans are generally administered to patients with early stage disease who are candidates for curative surgery and definitive treatment. The use of PET scans is not indicated for patients with obvious advanced or metastatic disease. In this case, one would say that the association of PET with survival is confounded by selection bias, or selective administration of PET scans to patients with less advanced disease. Another way to describe this would be to say that the association of PET with survival is being confounded by selective administration of PET to lower risk patients.10, 11
There are several commonly encountered types of bias that are important in the accurate interpretation of observational HSR studies. Omitted variable bias is one of the principal forms of bias in observational studies and is nearly always present to some extent in HSR. It will occur in any situation where (1) a variable exists that is associated with both the outcome and exposure of interest and (2) is omitted as a control. For example, in the PET-NSCLC scenario, disease stage was correlated with both PET and survival, but was not included in the model.
Selection bias is used to describe when a group or exposure is not randomly applied to patients, or in the example case above, where PET scans were selectively applied to patients with less advanced disease. Recall bias refers to the situation where one population is more likely to recall an event than another. For example, patients with a rare form of cancer might be more likely to recall exposure to any number of environmental stimuli that have been forgotten or would have been overlooked by patients without cancer who had the identical exposure. Bias due to loss to follow-up can occur if patients who remain in the study are systematically different from those who do not. Nonresponse bias
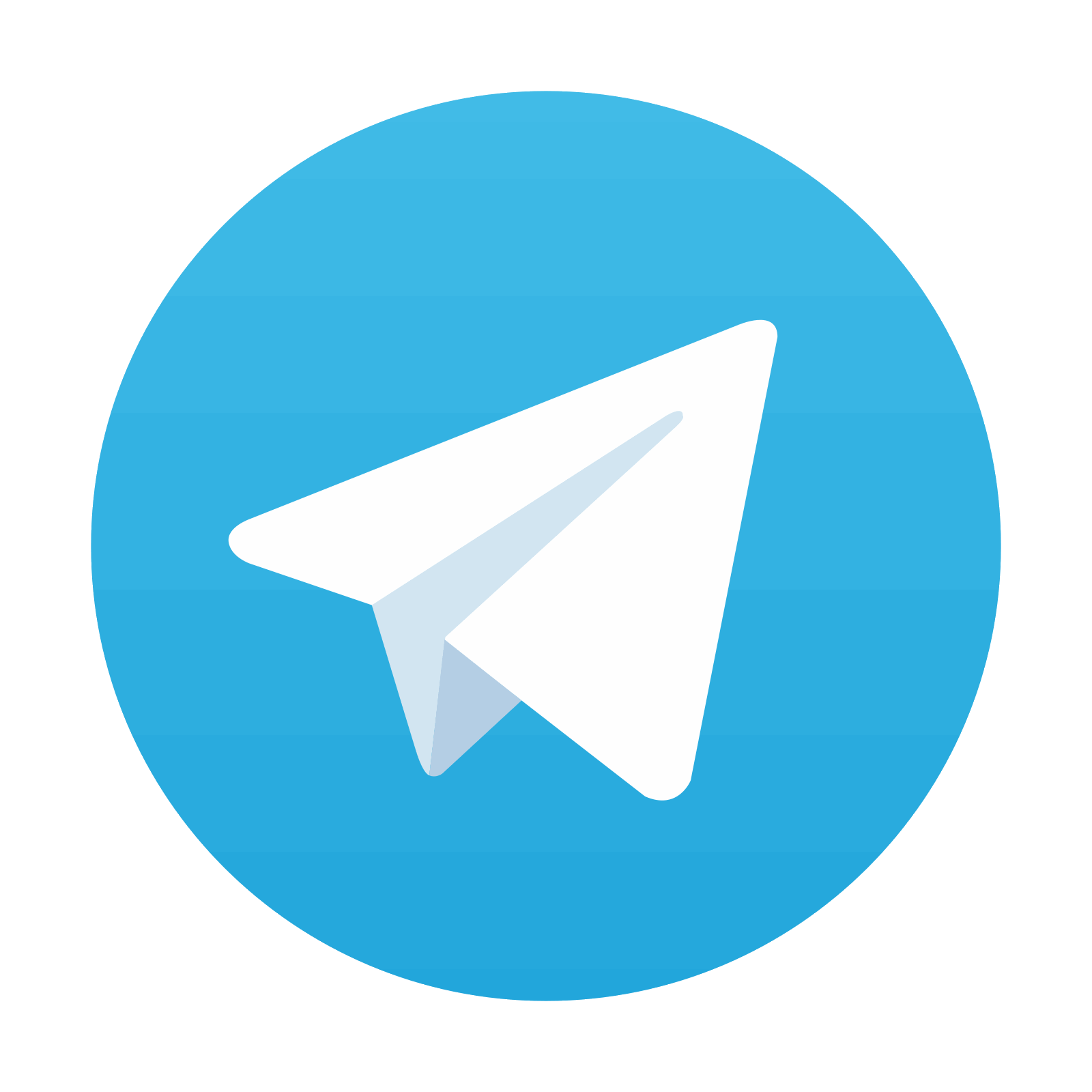
Stay updated, free articles. Join our Telegram channel

Full access? Get Clinical Tree
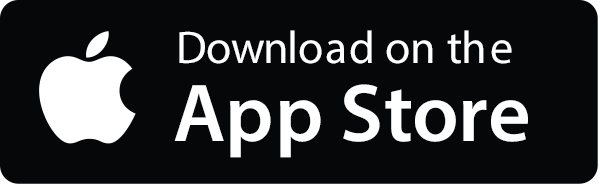
