Fig. 36.1
Schematic representation of the double- and single-channel array technologies. The two methods differ in the sample preparation and labelling procedures. A typical microarray experiment consists in the expression profile comparison of at least two experimental conditions. In the double-channel technology, mRNA extracted from samples is converted in cDNA labelled using a different fluorescent. These molecules are then mixed and used to hybridise a single array. After laser scansion it is possible to associate differentially expressed genes with a starting sample. In the single-channel technology, mRNA from each sample is converted in cRNA (RNA copy) labelled with biotin. Each cRNA is separately hybridised to a single array. After laser scansion it is possible to visualise the hybridisation
Microarray is classified into double-channel and single-channel array, according to the labelling methods. Double-channel array [9] uses cDNA or oligonucleotides spotted on a solid surface. Two different samples, each labelled with a different fluorescent dye (usually Cye3 and Cye5), can be hybridised at the same time on a single array. After laser scansion, it is possible to visualise upregulated and downregulated genes at once (Fig. 36.1).
The surface of a single-channel array is coated by spotted- or “in situ” synthesised oligonucleotides, and one sample is hybridised on a single array (Fig. 36.1). One of the most used single-channel arrays, Affymetrix GeneChip® (www.Affymetrix.com), uses a photolithographic process for a high-density oligonucleotide synthesis, where over 1.4 million probe set are accommodated on a very small surface (11 μm2 features on glass slides). In GeneChip®, multiple short oligonucleotides, perfectly matching to the 3′ ends of transcripts, are used to detect the whole expression level and multiple probe pairs (11–16 probe pairs or probe set) interrogate each transcript. Among the commercial single-channel microarray platforms, Affymetrix has the highest panel of microarray designed for a variety of different organisms and the highest public available datasets (www.ncbi.nlm.nih.gov/geo/). Another single-channel commercial platform is provided by Illumina (www.illumina.com) and is characterised by two interesting features: long oligonucleotides and probe replication. These arrays (Bead Array) are based on randomly arranged beads [10]. A specific oligonucleotide sequence, represented at about 30-fold redundancy, is assigned to each bead type. A series of decoding hybridisations is used to identify each bead, and the high degree of replication makes robust measurements for each bead type.
Microarray Data Analysis
Microarray data analysis is a multistep process in which any step can be performed combining different computational tools. The advantage is that all the necessary tools are implemented in Bioconductor (www.bioconductor.org), an open source and open development project, based on the R statistical programming language.
The main steps in microarray data process are:
1.
Quality control
2.
Data analysis
3.
Differential expression and data annotation
Each step described below can be applied to the different microarray platforms, downloading from the Bioconductor web page all the specific packages. Furthermore, for users with limited experience, it is possible to analyse data by using a graphical interface (OneChannelGUI) [11]. For simplicity, here we refer to GeneChip® 3′IVT array analysis.
1.
Quality controls include (a) hybridisation/construction artefact identification, (b) probe intensity distribution and (c) replicate quality evaluation. This step allows excluding the presence of array artefacts and dishomogeneity of different experimental groups. A function that can be used to identify hybridisation/washing artefacts is the “affyPLM”. It produces probe-level models (PLM) by fitting a linear model to the probe data and enables a model to be fitted with probe-level and chip-level parameters for each probe set. The function generates array pseudo-images very useful for detecting artefacts on arrays. Another PLM method is based on plot of Normalized Unscaled Standard Errors (NUSE) (Fig. 36.2).
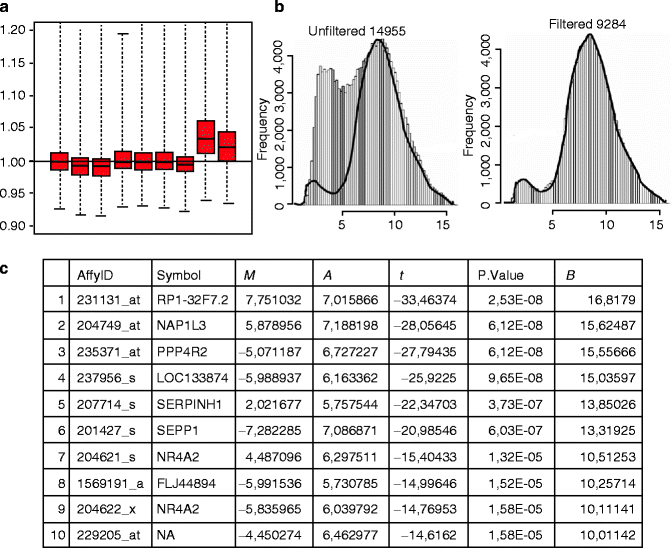
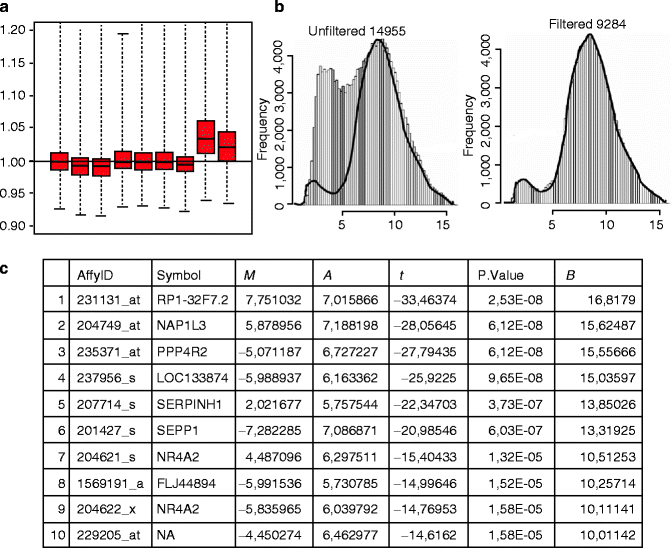
Fig. 36.2
Microarray data analysis, an example of output for the different steps: quality control (a), filtering (b) and annotation (c). (a) The NUSE box plot visualises a standard error from the probe-level model. NUSE plot allows to identify the divergent arrays that usually are not centred at 1.0 and appear spread out in comparison to the other arrays (as the last two box plots). (b) The IQR filtering output allows to filter out nonsignificant transcripts and select transcripts that will subsequently be analysed to detect the differential expression. In this example only genes with an intensity of at least 100 in at least half (50 %) of the arrays were selected. (c) Annotation of differentially expressed genes (top table). The table for each transcript returns different information associated to each probe ID and relative gene symbol. M log 2-fold change, A average intensity, P. Value p-value, T t-statistics, B log-odd statistics
2.
Data analysis allows to eliminate nonsignificant transcript. It includes (a) background subtraction, (b) data normalisation, (c) transcript intensity summary and (d) filtering of nonsignificant transcripts.
Normalisation and background correction procedures ensure that differences in intensities are really due to differential expression. The “affy” Bioconductor package uses intensities scaled so that each array has the same average value used for constant normalisation. If a non-linear relationship between arrays exists, it is more accurate to apply the quantile normalisation method [12] that makes the distribution of probe intensities for each set of arrays the same. This method is based on the concept that a quantile-quantile plot will show the same distribution of two data vectors if the plot is a straight diagonal line, but not if it is other than diagonal. Of course it is possible to have up to “n” dimensions if more than two arrays are available.
Genes estimated to be not expressed, according to user-defined specific parameters, are removed from the dataset by filtering (Fig. 36.2). IQR (interquartile range) is a filtering approach [13] implemented in the “genefilter” package which eliminates genes with no sufficient variation in expression across all samples, because of their weak discriminatory power.
Statistical validation methods are finally applied to identify differentially expressed genes and to obtain biological information from microarray data (data annotation). However, microarray experiments are conducted on a very limited number of biological replicates that is the exact opposite for an ideal statistical test. So many efforts have been made to optimise the statistical tests for microarray data. Furthermore, due to the reduced number of samples, the resulting differential expressed genes must be biologically validated using different approaches, such as real-time PCR or TMA assays. Several well-known statistical tests (e.g. t-test, ANOVA, linear model analysis) have been subjected to sophisticated optimisation to secure a good estimate of inter-/intra-sample variance despite the limited number of replicates. All methods assume that the transcription expression level of one gene is independent from that of the others. Best results are obtained with multiple statistical methods sharing a core of very robust differentially expressed genes that are always identified. The central issue is to find the best condition to obtain the highest number of only true signals [14].
In performing multiple statistical tests, two errors are possible: Type I error or false positive (a gene is identified as differentially expressed when it is not) and Type II error or false negative (a failure to identify a real differentially expressed gene). The Biocon-ductor package “multtest” implements widely applicable resampling- based single-step and stepwise Multiple Testing Procedures (MTP) that control a broad class of Type I error rates, null hypotheses and test statistics.
3.
The ultimate goal in gene expression analyses is to identify differentially expressed genes and to understand their biological significance in the specific context.
An important issue is the specific association of probe set identifiers with genome-annotated transcripts (Fig. 36.2). A critical point is how the annotation has been produced. Generally, commercial arrays define probes retrieving transcript sequences from the last release Unigene (www.ncbi.nlm.nih.gov/entrez/query.fcgi?db=unigene), the major repository of sequences belonging to transcriptomes for all known organisms. To associate a probe set with a specific gene, Bioconductor uses a specific annotation library (AnnBuilder) which creates Bioconductor annotation libraries or XML annotation files starting from the association “probe set identifier”/“GenBank accession number” (i.e. the primary target for probes design).
The biological knowledge extraction from the candidate gene list represents the last step of the data analysis procedure. The assignment of functional categories to a gene list is the main challenge in understanding the biological question. Furthermore, it is generally accepted that genes sharing a similar expression profile might have similar biological functions.
Different public databases, as Gene Ontology (http://www.geneontology.org/), KEGG (http://www.genome.ad.jp/kegg/) and GSEA (www.broadinstitute.org/gsea/index.jsp), allow performing a functional analysis.
Gene Ontology (GO) contains a controlled vocabulary of terms (ontologies) describing gene product characteristics and gene product annotation. Three structured ontologies describe gene products in terms of their associated molecular functions, the biological processes they act and the cellular components they belong to. GO is organised as a graph, where each term is a node and the relationships between the terms are arcs between the nodes.
KEGG is another database based on hierarchal graphs representing the various KEGG objects, from the molecular level to the more complex levels. The structure contains graphs (each graph is a set of nodes or KEGG objects) and edges (that are the biological relationships).
GSEA is a tool that functionally associates pathways and differential expression by performing a “per-gene” statistics across genes. In this way, it is possible to determine if a priori defined set of genes shows statistically significant differences between two biological states and to identify either small changes in many genes or large changes in few genes [15].
Different commercial tools are also available to extract biological meaning from a candidate gene list, as IPA (www.ingenuity.com) and Pathway Studio (www.ariadnegenomics.com/products/databases/). IPA is a web-based software application, which enables to analyse and integrates gene expression data in which information is manually curated by experts in order to ensure accurate and detailed content.
Pathway Studio bases its core technology on MedScan® technology, a software able to extract biological information from multiple sources of public information (text, journals) and from different datasets.
Both software use databases derived from primary literature sources and the users can always link back to the original findings in the original source article.
NGS Technology
The completion of human genome sequence has represented the first step for the development of new sequencing methods to rapidly assemble and sequence whole genomes. NGS represents the latest methods that allow to studying structural genome variants and methylation patterns over gene expressions.
Three main platforms are used in NGS application: the 454 Genome Sequencer (Roche, www.454.com), the Genome Analyzer (Illumina, www.illumina.com) and the SOLiD (Life Technologies, www.appliedbiosystems.com). The other two available platforms, the Polonator G.007 and the HeliScope (Helicosbio), will not be described here.
NGS technologies are based on the clonal amplification of the sample to analyse, followed by sequencing and imaging procedures. The template preparation consists in a random fragmentation of DNA needed to obtain a library of fragments to amplify. These fragments are then ligated to adaptors that can bind universal primers in the amplification reaction.
454 and SOLiD realise the template amplification in a cell-free system, through an emulsion PCR (ePCR). Single-stranded DNA binding beads are encapsulated in micro-reactors by vigorous vortexing of the aqueous micelles containing PCR reactants and PCR oil. The amplified beads are purified and then deposited in the micro-wells of the slide (Roche) or onto the glass slide surface (Applied) where the sequencing reaction takes place (Fig. 36.3).
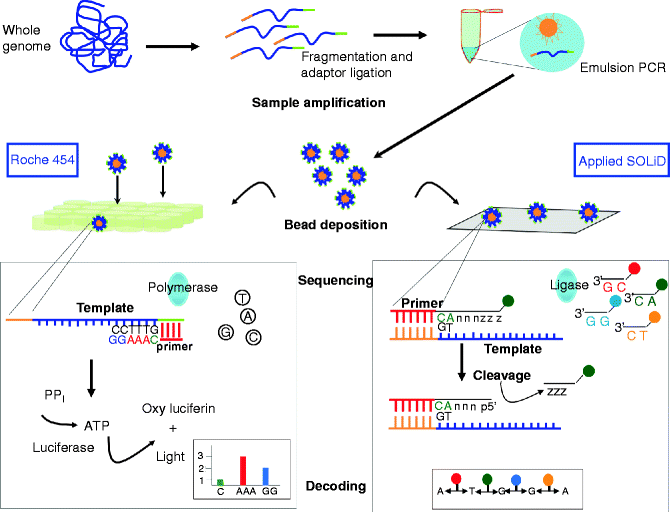
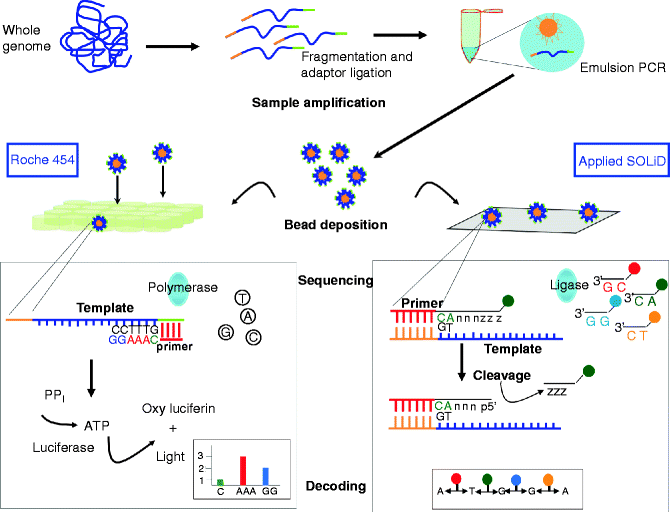
Fig. 36.3
NGS sample amplification and sequencing procedures for 454 and SOLiD platform. Samples to sequence are clonally amplified by emulsion PCR after fragmentation and ligation of adapter to the fragments. After amplification the bead-containing fragments deposited into picotiter-plate wells with sequencing enzymes (454) or onto a glass slide (SOLiD). Sequencing in 454 platforms is based on pyrosequencing. A nucleotide incorporation results in pyrophosphate (PPi) release that is then converted into light. The order and the intensity of the light peaks are recorded as a fluorogram revealing the DNA sequence. The light signal generated by complementary nucleotides will be proportional to the number of nucleotides incorporated. The SOLiD system uses sequencing-by-ligation method and a colour-space decoding. Sixteen different dinucleotides, corresponding to all possible 2-base combinations, are linked to six degenerate bases (nnnzzz) and to four different fluorescent labels at 5′ end. The template binds the complementary dinucleotide, and after ligation the degenerated nucleotides are cleaved and the fluorescence is recorded. The cycle is then repeated and completed after ten ligases and five different primers. The colour-base reads are converted into base-space reads based on a two-colour method, in which each base corresponds to different and fixed colour combination
Illumina amplifies the DNA, by a solid amplification. The glass slides contain forward and reverse primers covalently attached to the surface. The fragmented DNA is linked to the adaptors that can bind the primers on the glass slide. Then clonally amplified clusters from each fragment are produced (Fig. 36.4).
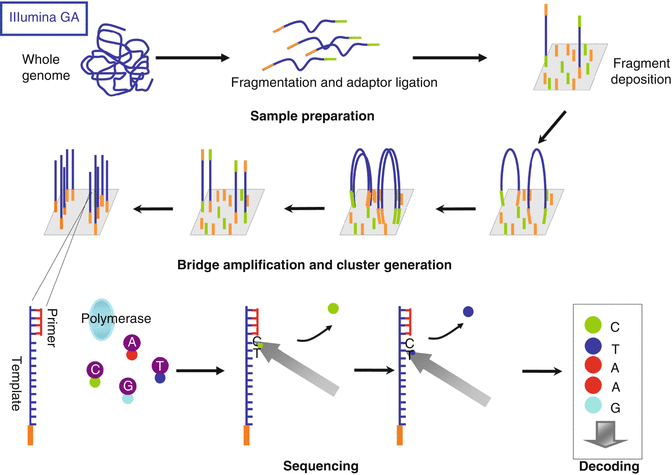
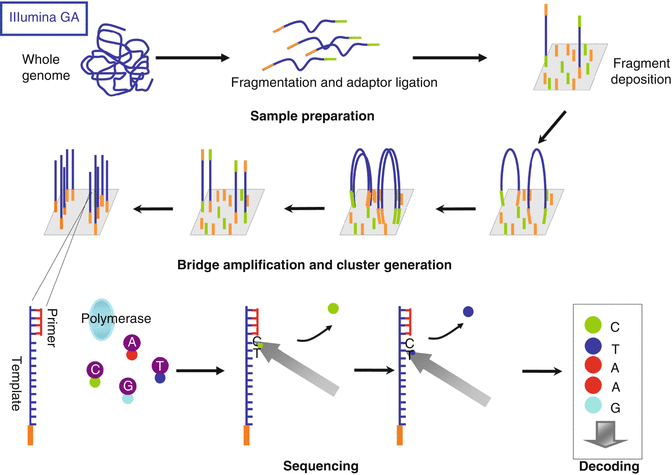
Fig. 36.4
Illumina Genome Analyzer sequencing with reversible terminators. Samples to sequence are fragmented and ligated to adapters. Single-stranded DNA fragments are added to glass surface where they bind complementary primers. Fragments are clonally amplified by bridge amplification generating clusters. Clusters are then denatured and sequencing starts with the addition of primer, polymerase and 4-colour reversible dye terminators. Fluorescence is recorded at each nucleotide incorporation. After imaging fluorescent dye is removed and the cycle restarts until the template is sequenced
The sequencing reactions in the Roche GS FLX 454 Genome Sequencer is based on sequencing-by-synthesis method, or pyrosequencing developed by Ronaghi [16], in which a chemiluminescent signal is produced when a nucleotide complementary to the template is inserted. The nucleotide incorporation is connected to inorganic pyrophosphate release that is measured by converting it into a light signal through the ATP that converts luciferin in oxyluciferin and light (Fig. 36.3). The 454 Sequencer produces an average read length of 400 bp, and its more powerful application is in the de novo genome assembly. The latest model (GS FLX+) can produce up to 750 bp per read with Sanger-like read length generating 500 Mbp (mega base pairs) of mappable sequences per run.
The sequencing method in SOLiD platform is based on sequencing by ligation, with two-base encoding probes and four colours. The beads containing the template fragments are deposited on the slide and are ligated to specific 8-mer primers, fluorescently labelled in the 4 and 5 positions. The reads produced are in colour space and each ligation step is followed by fluorescence detection. A specific two-base encoding scheme permits to associate the four dinucleotides with one colour (Fig. 36.3). The sequence is then retrieved through the alignment with a colour-space reference sequence. The advantage of this method is that each template is interrogated twice. A complete round of ten ligations and five different primers sequence 50 base fragments and produce 80–100 Gbp of mappable sequences per run. A new recent model (5500xl) is able to generate over 2.4 billion reads per run and 200 Gbp.
The Illumina Genome Analyzer uses a sequencing method based on the use of reversible terminators in a cyclic three-step way: nucleotide incorporation, fluorescence imaging and cleavage. The clonally amplified molecules located on the slide are detected using a bridge amplification that extends cluster strands with all four nucleotides, each labelled with a different fluorescent dye. Each nucleotide is identified after the incorporation (Fig. 36.4). The cycle is repeated until the imaging reveals the sequence corresponding to each amplified template (50–75 bp). The latest Illumina sequencer (HiSeq 2000 Genome Analyzer) generates about 200 Gbp of short sequences per run.
Illumina and SOLiD platforms both represent the ideal solution for genome resequencing or for exomes and transcriptome assays, where reference sequences are available.
NGS Data Analysis
NGS assay produces large datasets of sequence reads in a massively parallel format. The reads must be aligned to reference data in order to obtain quantitative information about any parameter (position, variation or frequency) and relative to read differences from the reference sequence.
There are three main steps in NGS data management and analysis:
Primary analysis or alignment
Secondary analysis or assembly
Tertiary analysis
The first two steps allow mapping the reads to the right genomic location before assembling them into longer contiguous sequences. The primary analysis is the transformation of image data in sequence data. The reads obtained can be in sequence or in colour space depending on the platform used to generate data. This step also ranks the quality value of each nucleotide. Sequences obtained from the primary analysis are aligned to reference sequence in the secondary analysis. The reference is specifically selected based on the application. We can select a complete genome or a genome subset if we are analysing only the expressed genes. The tertiary analysis is a multistep procedure used for the extraction of biological information from the obtained data (Fig. 36.5).
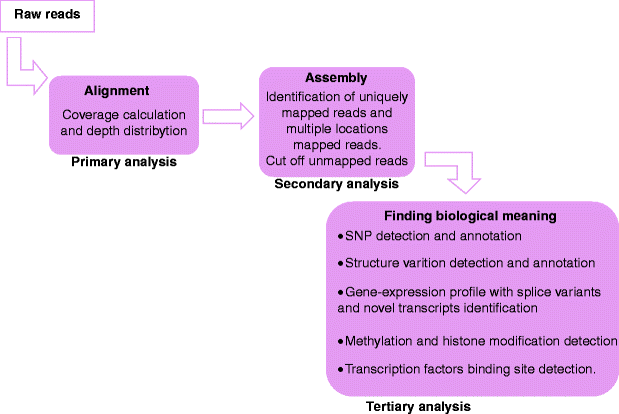
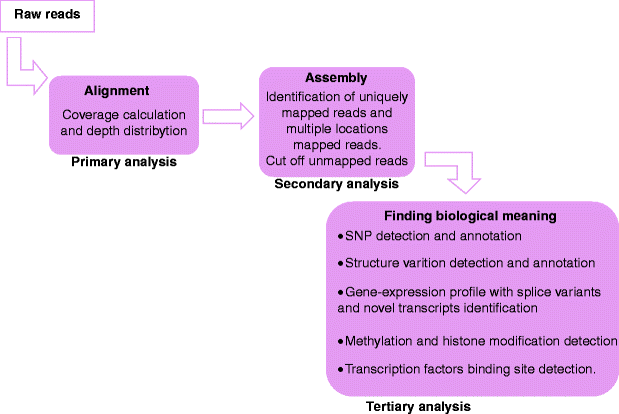
Fig. 36.5
NGS data analysis. Pipeline for NGS data analysis
1.
The alignment to a reference sequence is fundamental to establish the failure or the success of an NGS experiment. Different algorithms can be used for alignment and assembly depending on the sequencing platform used. The two fundamental alignment algorithms are the hash-based method [17] and the Burrows-Wheeler transform method [18].
The hash-based methods generate a lookup table that is designed to efficiently store noncontiguous keys (account numbers, part numbers, etc.) that may have wide gaps in their alphabetic and numeric sequences. In order to map a sequence, the program identifies in the reference sequence small strings where it is probable to find the best mapping. This step is then followed by a more accurate alignment algorithm, such as the Smith-Waterman [19]; it is used to determine the exact position of the reads on the genome reference sequence. This approach is used by different tools that can build the hash table either from input reads (MAQ, ZOOM, SHRiMP or ELAND that is part of the Illumina Suite) or from reference genome (SOAP, BFAST and MOSAIK).
The Burrows-Wheeler transform method is an algorithm originally created for text compression (zip), which uses suffix arrays for rapid exact subsequence search. The method first generates an index of the reference genome, which is used for a rapid positioning of the reads on the genome. This method can be easily improved resulting in a faster processivity without loss in sensitivity. BOWTIE, BWA and SOAP2 are examples of tools that use BWT method.
2.
Assembly has a more important role in analysing organisms for which a reference genome is not available. It is a procedure that allows having large continuous regions of DNA sequence. The algorithms used to read the long sequences (800 bp) obtained from gel-capillary technologies were based on the assembly of overlapping sequences. Because of the short read lengths (25–50 bp) and large volumes of data generated by NGS assays, it is complex to assemble short reads using the classical shotgun method [20]. In fact it is difficult to distinguish repetitive sequences derived from adjacent genomic regions or from highly polymorphic loci. The NGS technology overcomes this limitation by oversampling the target genome with short reads from random positions, by using specific software.
Assembly methods are based on “graphs”, a concept used in computer science composed by sets of nodes connected by sets of edges. The graph can be visualised as balls in space connected by arrows, with edges directionally connected from a node to another. Different paths can be viewed from different edges and it is possible to have intersecting paths. So an overlap graph might represent the reads and their overlaps [21]. Furthermore, the graph is able to discriminate in the 5′ and 3′ ends’ reads, their length and the overlap length and type. Finally, paths between graphs represent contiguous parts and they can be converted in sequence.
The “de Bruijn graph” is a compact representation based on short words (k-mers). In the graph (k-mer graph), the nodes are all possible fixed-strength sequences (of symbols from the DNA alphabet) and the edges indicate the potentially overlapping sequences. The structure is based on very small sequences of fixed length k (k-mers), not reads; thus, high redundancy is naturally handled by the graph without affecting the number of nodes. Non-repetitive sequences induce formation of a single path, while repeats induce convergent and divergent paths [22]. Many assembler tools are based on the “de Bruijn graph” (SHARCGS, VCAKE, VELVET, EULER-SR, EDENA, AbySS and ALLPATHS).
3.
The complexity of the tertiary analysis is tightly linked to the assay. While pattern variation analysis is easy, digital gene expression assays are very complex. In fact expression measurements need to be normalised between datasets and statistical comparisons are critical in order to assess differences. Various bioinformatic approaches are available according to the application, as de novo sequencing, resequencing, and transcriptome (RNA-seq) or chromatin immunoprecipitation (ChIP-seq) sequencing analyses. Given the complexity of the final step in NGS data analysis, we will not further discuss in detail the methods available.
Tertiary analysis can be done using different packages from Bioconductor (www.bioconductor.org) and several commercial tools such as Golden Helix (www.goldenhelix.com) software and SeqSolve (www.integromics.com/ngs) that provide a global analysis starting from mapped reads. Golden Helix was specifically designed for genotype analysis, while SeqSolve performs RNA-seq or ChIP-seq analyses on any annotated genome.
Applications in Melanoma
Melanoma Biology
Melanoma originates from the pigment-producing melanocytes that are positioned at the epidermal-dermal junction and are interspersed among every five to ten basal keratinocytes. Despite the dynamic nature of epidermal shedding, involving constant growth, differentiation and vertical migration of keratinocytes, proliferation of melanocytes is strictly controlled and rarely observed under physiologic conditions, albeit melanocytes maintain a lifelong proliferation potential.
The developmental process of melanoma is characterised by a dramatic change of the cellular homeostasis due to the deregulated expression of many genes [4, 23].
The Clark model of melanoma genesis and progression has provided a basic and relatively straightforward platform for the hypothesised stepwise transformation of normal human epidermal melanocytes (NHEM) to melanoma, describing five distinct stages of progression based on histological criteria (Fig. 36.6) [24]. According to this model, melanocytes can proliferate and spread, leading to a formation of a naevus or common mole. Based on the localisation of proliferating melanocytes, naevi can be classified as junctional, dermal or compound. Some naevi are dysplastic with morphologically atypical melanocytes. In the most cases, naevi are benign but can progress to the radial growth phase (RGP) melanoma, which consists of an intra-epidermal lesion, which could involve some local invasion of dermis. RGP cells can progress to vertical growth phase (VGP) primary cutaneous melanoma (PCM). This represents a more dangerous stage, in which the cells have potential metastatic properties, with nodules or nests of cells invading the dermis. Not all melanomas pass through each of these individual phases. RGP or VGP primary melanoma can develop directly from both isolated melanocytes and naevi, and both can progress directly to metastatic melanoma (MM).
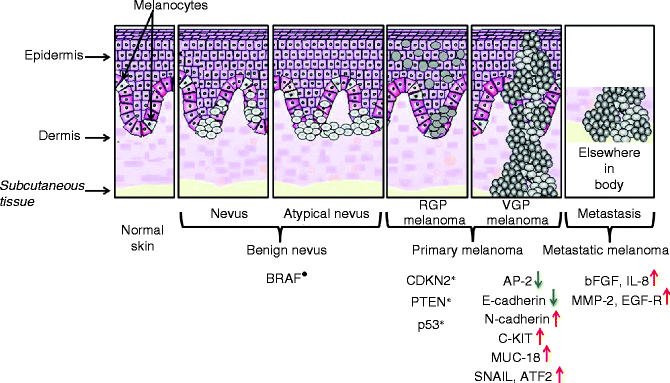
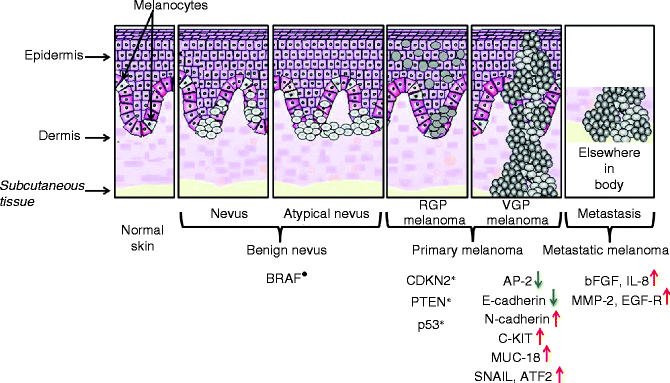
Fig. 36.6
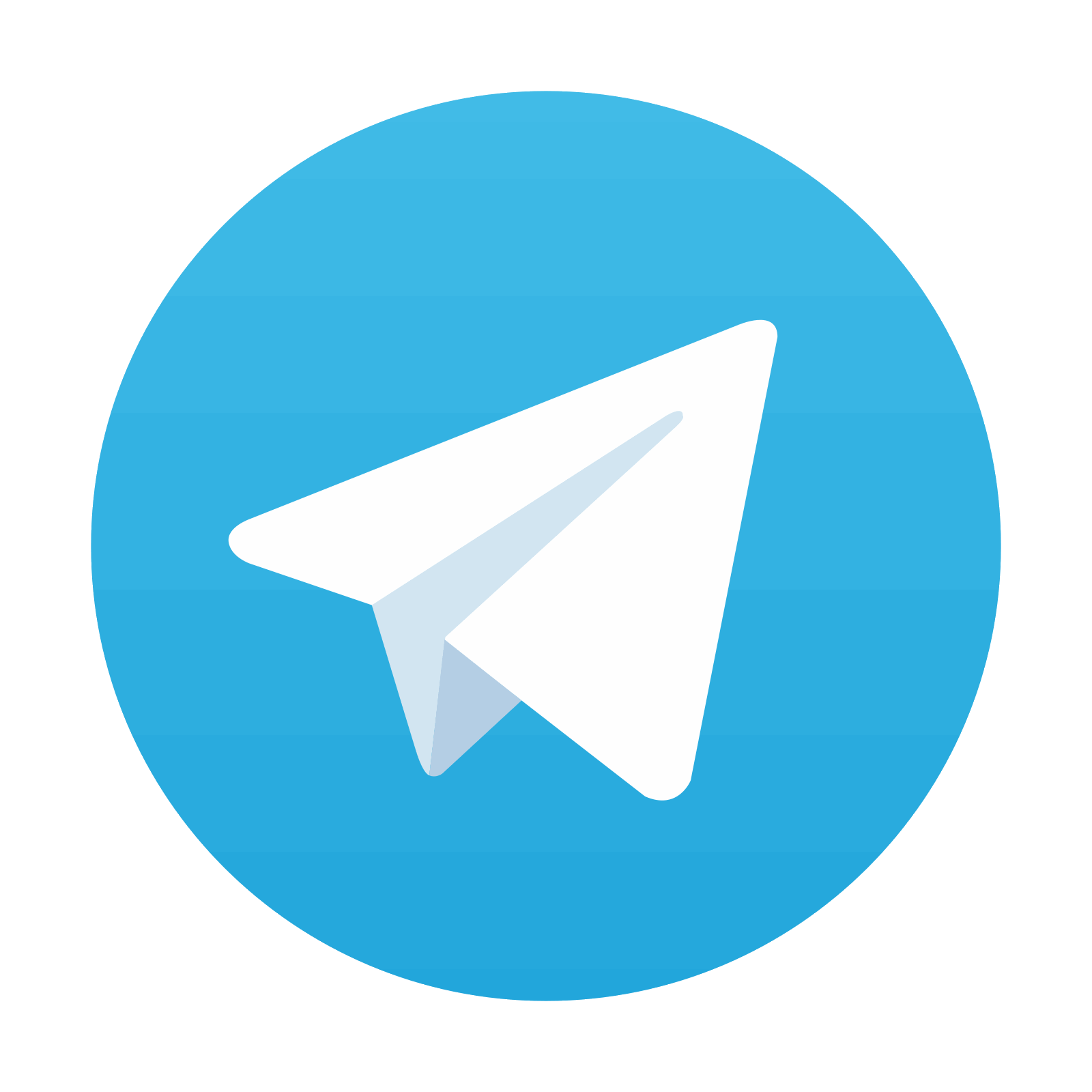
Stepwise changes involved in melanoma genesis. Five distinct stages associated with the evolution of melanoma, from naevus to metastatic melanoma, are represented. Molecular events associated with melanoma progression are also reported. Constitutive activation was indicated with the symbol (•), while inactivation by mutation, deletion or epigenetic alterations was indicated with the symbol (*). Upregulated (↑) and downregulated (↓) genes were reported
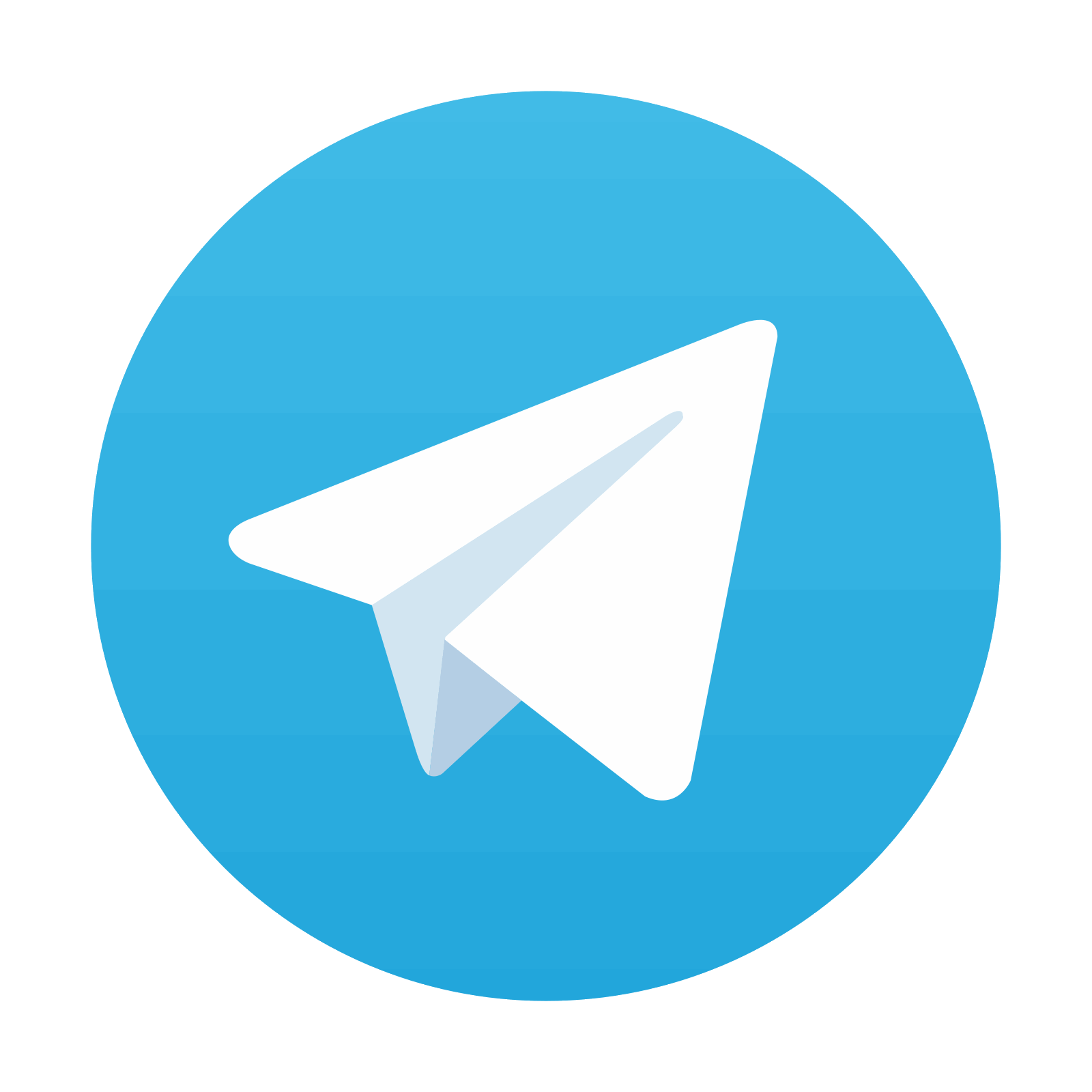
Stay updated, free articles. Join our Telegram channel

Full access? Get Clinical Tree
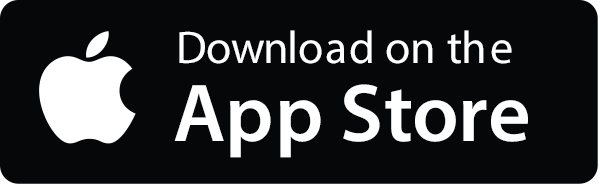

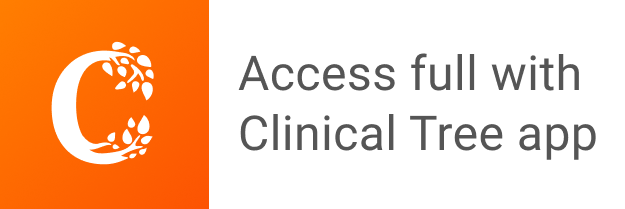