17.1
Introduction
This chapter was developed to assist readers with critical evaluation of published research studies, choice of study design in addressing a research question, and understanding of basic epidemiologic terms and principles, and appreciation of some reasons why studies examining similar research questions may report discrepant results.
Epidemiology is the study of the occurrence, distribution, and determinants of diseases and other health-related conditions in populations, and the application of this study to control health problems. It is used for many purposes. One objective is to describe the magnitude and impact of diseases or other conditions in populations. This data are used to set priorities for investigation and control of disease, formulate hypotheses about relationships between exposure and disease, decide where preventive efforts should be focused, and implement community interventions to prevent and reduce the impact of health problems. For instance, data on current hip fracture incidence rates in various parts of the world and projected large increases in the numbers of elderly in developing countries suggest that the public health impact of hip fractures will increase markedly throughout the world and that there is a pressing need to develop strategies for the prevention and treatment, particularly in the developing countries . Accordingly, identifying risk factors for hip fracture in developing regions of the world becomes high priority for analytical epidemiologic studies that test hypotheses about the relationship between an exposure or risk factor and a health outcome, such as hip fracture. Experimental epidemiologic studies, such as randomized placebo-controlled clinical trials evaluating the efficacy and safety of pharmacologic agents in preventing fracture, provide the strongest evidence about the causality between an exposure (e.g., drug treatment) and risk of disease outcome (e.g., fracture). The focus of this chapter is to review the application of these epidemiologic methods in studies of osteoporosis and related fractures.
17.2
Descriptive and analytic studies
17.2.1
Descriptive epidemiology
Descriptive epidemiologic studies provide information on patterns of disease occurrence in populations according to characteristics such as age, gender, race, ethnicity, marital status, social class, occupation, geographic area, and time of occurrence. While descriptive studies may use data collected primarily for research purposes such as a population-based general health survey, they often utilize data from sources not primarily designed for research (e.g., patient registries, hospital discharge records, or death certificates) to indicate the magnitude of a problem or to provide preliminary hypotheses about disease etiology. For example, descriptive studies have documented worldwide variation in hip fracture incidence rates with the highest hip fracture rates in Northern Europe and the United States, intermediate rates in Asian countries, and lowest rates in Latin America and Africa. These studies have generated research examining potential reasons underlying this variation, including population demographics (with more elderly living in countries with higher incidence rates), latitude, genetic factors, and environmental factors.
Correlations between a putative risk factor (e.g., diet or lifestyle behavior) and a disease according to geographic region provide weak evidence that the factor causes the disease. There are many differences in lifestyles and other characteristics of people living in different geographic areas that singling out one factor as being the reason for the difference in incidence rates is usually impossible. Countries with higher hip fracture incidence rates compared to those with lower incidence rates have many differences in diet, as well as different levels of urbanization, physical activity, smoking patterns, neuromuscular functioning, race/ethnicity/genetic variation, medication use, and life expectancy. Accordingly, analytic epidemiologic studies designed specifically to test hypotheses about the association between an exposure and a given disease outcome are conducted to provide more definitive information.
17.2.2
Analytic epidemiology
Analytic studies are designed to test hypotheses that have been generated from descriptive epidemiology, clinical observations, laboratory studies, or other analytic studies. While descriptive epidemiology describes how a disease or characteristic such as bone mineral density (BMD) is distributed in a population, analytic epidemiology tries to explain why. Because analytic studies often require the collection of new data, they tend to be more expensive than descriptive studies, but, if designed and executed properly, generally allow more definitive conclusions to be reached about associations.
Most epidemiologic studies are observational, that is, the investigator observes what is occurring in the study populations of interest and does not interfere with what he or she observes. For instance, an investigator could observe existing physical activity levels among individuals and relate those activity levels to bone mass or fracture occurrence. In contrast, in an experimental epidemiologic study, the investigator intervenes and assigns members of the study population to one exposure (e.g., treatment group) or another, as in a randomized clinical trial. In a randomized trial evaluating the efficacy of physical activity in reducing bone loss and fractures in older adults, an investigator would randomly assign older individuals to programs with varying levels of physical activity and monitor changes in bone mass and occurrence of fractures following the randomization visit.
Analytical epidemiologic studies, including case–control studies, cross-sectional studies, cohort studies (prospective, retrospective, nested case–control, and case–cohort studies), and randomized clinical trials, will be presented in the next section.
17.3
Study designs in analytical epidemiologic studies
17.3.1
Case–control studies
Case–control studies are those in which the investigator selects persons with a given disease (the cases) and without the given disease (the controls) for study. Usually, the cases enter the study as they are diagnosed over time, accompanied by a control that also enters the study over the same time period. The proportion of cases and controls with certain characteristics or past exposure to possible risk or protective factors is then determined and compared ( Fig. 17.1 ). For example, a case–control study of fractures using the UK General Practice Research Database found that 5.7% of participants with a femur fracture and 9.2% of controls were current users of β-blockers, suggesting that β-blocker may be a protective factor associated with lower fracture risk. For a measurement with a continuous distribution, such as weight, the mean level of the characteristic of interest in the cases is compared to the mean level of the characteristic in the controls. In a case–control study in Utah the mean body mass index (BMI) [weight (kg)/height 2 (m 2 )] was 24.1 in women with hip fracture (cases) and 26.5 in female controls, suggesting an increased risk for fracture among those women with lower BMI.
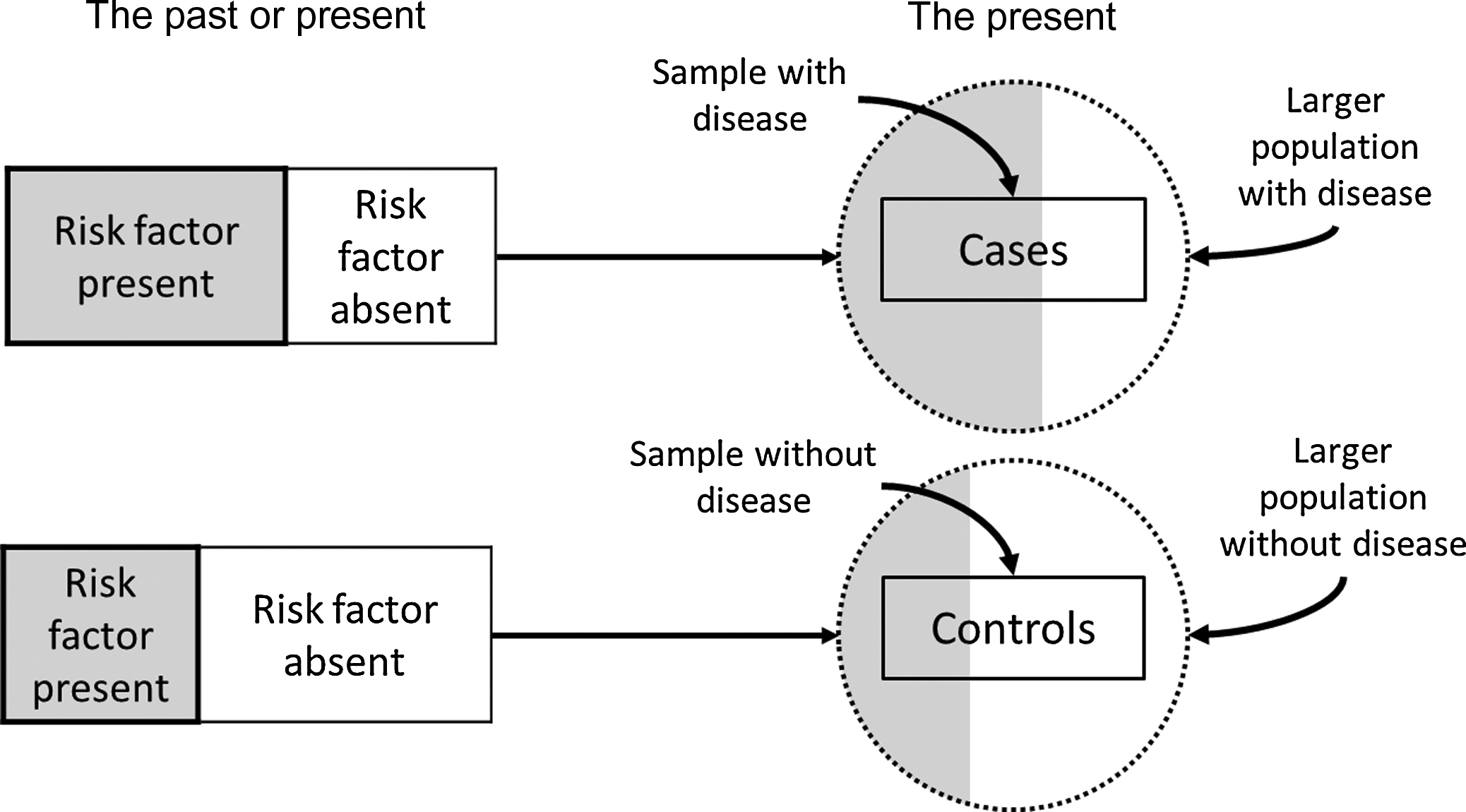
Case–control studies are relatively inexpensive to conduct, useful for generating multiple hypotheses about disease etiology since a given investigation can examine several exposures, and uniquely efficient for studying rare diseases. However, they also have serious limitations. Case–control studies do not provide estimates of the incidence of the disease under investigation because the proportion of subjects with the disease is determined by the number of cases and number of controls the investigators chooses to sample, rather than by their proportion in the population. A major weakness of case–control studies is their susceptibility to bias due to separate sampling of cases and controls and retrospective measurement of exposures (e.g., risk or protective factors).
Sampling in a case–control study begins with cases, who may be persons seeking medical care for the disease. To be more certain that the risk factor preceded the disease rather than being a consequence of the disease so that cases with rapidly fatal conditions or conditions of short duration are appropriately represented in the study, only newly diagnosed persons are ideally included as cases. For instance, fracture cases tend to change their physical activity patterns following a fracture. If cases with a previous fracture are included, it is more difficult to differentiate the physical activity pattern that preceded the fracture from the physical activity pattern that resulted from the fracture. Numerous case–control studies have been undertaken to identify potential risk factors for hip fracture. Because patients with hip fracture are almost always hospitalized, virtually all cases can be identified from hospital sources. For fractures of many other common skeletal sites, such as distal forearm fractures, only a select portion of all cases would be evaluated in a hospital setting, so the representativeness of cases selected from hospital settings to the entire population of individuals who sustain distal forearm fracture would be highly questionable. Efforts should be made to identify distal forearm fracture cases identified and treated in the outpatient setting as well, a considerably more extensive undertaking.
Choice of an appropriate control group is more difficult and one of the most controversial aspects of designing a case–control study . Ideally, the controls should be selected in an unbiased manner from the population of individuals who would have been selected as cases if they had developed the disease under study. The choice of which control group to use generally depends on the source of the cases, the relative costs of obtaining the various types of controls, and the facilities and resources available to the investigator. If cases consist of all individuals developing the disease of interest in a defined population, the single best control group would generally be a random sample of individuals (in the same age range and of the same sex, for instance) from the same source population who have not developed the disease. Matching ensures that cases and controls are comparable with respect to major factors related to the disease (e.g., age and sex), but not of interest to the investigator. On the other hand, one limitation of matching is the inability to examine the effect of the matching factor (e.g., age) on the likelihood of the disease in question. If cases are identified at certain hospitals that do not cover a defined geographic area, it is usually impossible to specify the source population from which the cases arose. In this situation, controls are often chosen from among other patients admitted to similar services of the same hospitals as the cases, but with a different diagnosis as one wants to obtain a source of controls subject to the same selective factors as the cases. If this approach is used, it is important to exclude potential controls that have had their specific disease for a long time because, like the cases, the presence of their disease may have influenced exposure of control subjects to possible risk factors. For example, exposures such as physical activity, diet, weight, and medication use may change as a result of having many different diseases.
Controls in case–control studies of hip fracture have included people from the same retirement community as the cases , people from the same prepaid health-care plan as the cases , people sampled from the same general population as the cases , and patients seen at the same hospitals as the cases for other conditions . In countries or other geographic units with population registries, controls might consist of a random sample of persons from the same geographic area as the cases in the appropriate age groups, as listed in the register .
The various types of control groups have their own strengths and weaknesses. If controls from hospitals are used for case–control studies of hip fracture, the controls by definition are different from the cases in that they have another disease condition(s) for which they have sought medical care. If smoking is the putative risk factor, for instance, there may be concern that hospital controls include a disproportionate share of smokers, as smoking is associated with many diseases in addition to hip fracture that require hospitalization. A major concern in using controls from some groups is that a substantial proportion of potential controls (typically 30%–40% in otherwise well-executed studies) may decline to participate, and it is possible that participants and nonparticipants controls differ in ways that affect study results. Cases and controls from prepaid health-care plans or from retirement communities are generally more likely to participate in studies, thus giving higher response rates. A case–control study conducted using a population-based general practice database is not subject to this selection bias, but these databases do not contain information on putative risk factors such as physical activity level. In some situations when no single control group is obviously best, it may be helpful to have two or more control groups with which to compare the cases .
Certain cases and controls may be excluded from a study, such as those with other disorders that affect bone metabolism and that are not of interest to the study being conducted. Although excluding cases and controls may limit generalizability, the validity of the comparison between cases and controls must take higher priority. The general principle that the same exclusion criteria should be applied to cases and controls should be maintained whenever possible. If cases are restricted to a certain sex or age range, controls should be similarly restricted. If cases with certain medical conditions are excluded, then controls with those conditions should also be excluded. While equal application of exclusion criteria may sound reasonable and easy, in practice this may be difficult to implement. Undiagnosed diseases of bone metabolism such as Paget’s disease may exist among controls in a case–control study of hip fracture, as the controls may not have had a similar diagnostic evaluation as the cases. Inequitable access to health care between cases and controls can exacerbate this problem.
Bias due to measurement error caused by the retrospective approach to measuring exposures, especially when it occurs to a different extent in cases as compared with controls, is a key limitation of case–control studies. Exposure information may be obtained in several ways. Risk factor data are obtained most commonly by means of questionnaires administered by trained interviewers to cases and controls. For instance, a practical way to find out about a person’s smoking habits is to ask the person using standardized questions. Existing records may sometimes be used to find out about exposures such as medication use or laboratory measurements prior to outcome occurrence. Use of this information is less susceptible to bias, especially if the abstractor is unaware of or blinded to case–control status. If physical measurements or laboratory tests on sera or other tissue are drawn or collected from cases and controls after the occurrence of the outcome (e.g., fracture), one must keep in mind that measurements of characteristics including bone density, biomarkers (e.g., markers of bone turnover or 25-hydroxyvitamin D), or biopsy material made after a fracture has occurred may differ from the values of these characteristics before the fracture occurred. Whichever methods are used, ensuring that ascertainment of exposure status is comparable in cases and in controls is of the utmost importance.
Potential problems need to be carefully considered before deciding whether a case–control study is appropriate in a given situation . Among the most common concerns are that (1) information on potential risk factors may not be available either from records or the participants’ recall, (2) information on other relevant variables may not be available either from records or from the participants’ recall, (3) cases may search for a cause for their disease and thereby be more likely to report an exposure than controls, (4) the investigator may be unable to determine with certainty whether the agent was likely to have caused the disease or whether the occurrence of the disease was likely to have caused the person to be exposed to the agent, (5) identifying and assembling a case group representative of all cases may be unduly difficult, (6) identifying and assembling an appropriate control group may be unduly difficult, and (7) participation rates may be low.
Because of these potential limitations, the case–control study is considered by some to be a hypothesis-generating study that provides leads to be followed up by more definitive cohort studies. However, decisions as to whether preventive actions should be taken must sometimes be reached on the basis of information obtained from case–control studies. Each case–control study should be evaluated individually, as some studies are affected by error and bias, while others are not.
In summary, case–control studies can provide much useful information about risk factors for diseases, including hip fracture and other fractures, in settings where fractures can be ascertained readily. Case–control studies are the more frequently undertaken type of analytic epidemiologic study, particularly when there are diseases with categorical outcomes such as the presence or absence of a fracture. They can generally be carried out in a much shorter period of time than cohort studies, do not require nearly so large a sample size, and, consequently, are less expensive. For a rare disease, case–control studies are usually the only practical approach to identifying risk factors.
17.3.2
Cross-sectional studies
In a cross-sectional study, variables, including exposure to a hypothesized risk factor or characteristic of interest and the occurrence of a disease, are measured at a single point in time or within a short period of time in a study population, with no follow-up period. This study design is well suited to the goal of describing variables and their distribution in a population. The prevalence of disease (e.g., number of cases of existing disease or condition per population at risk at a given point in time) is compared in those with and without the exposure or characteristic of interest. For a continuous variable such as BMD, the mean values of BMD in the exposed and nonexposed groups may be compared.
For example, a cross-sectional study using data from the Third National Health and Nutrition Examination Survey (NHANES) 2005–10 estimated the prevalence of osteoporosis and low bone mass (i.e., osteopenia) according to categories based on sex, age group, and race/ethnicity . Based on the overall adjusted prevalence rates from NHANES and US Census 2010 population counts, the study determined that 10.2 million US adults aged 50 years and older had osteoporosis (8.2 million women and 2.0 million men) and 43.4 million had osteopenia (27.3 million women and 16.1 million men). In cross-sectional studies the choice of which variables to label as predictors and which to designate as outcomes depends on the cause-and-effect hypotheses of the investigator, rather than on the study design. While the designation of predictor and outcome variablesis based on biologic plausibility and information from other sources, it is impossible to differentiate cause and effect. Thus findings from cross-sectional studies yield weaker evidence for causality than cohort studies, where the measurement of the predictor variables precedes the occurrence of the outcome. Interpretation of findings from cross-sectional studies is generally clear only for potential risk factors that will not change as a result of the disease, such as genotype, and even then can be difficult if the risk factor impacts survival as discussed next.
Cross-sectional studies include all cases of disease, new and old. Therefore another limitation of cross-sectional studies is that the case group tends to be weighted toward individuals with disease of long duration, as the chances for cases of long duration to be included are greater than those for cases who recover or die quickly. Thus any associations found between an exposure and a disease may be more applicable to survivorship with disease rather than development of disease. For cross-sectional studies to be useful in describing the prevalence of a disease or condition in the population, it is important that the subjects to be representative of the population to which the results are to be generalized. In the NHANES the sample is designed to represent the US population. Thus findings from NHANES provide a major source of information about health and habits of the US population. However, even analyses using NHANES data may be subject to bias due to nonresponse rates in the survey or examination phase. Thus analyses using NHANES data should use a weighted approach to ensure that the sample is representative of the US civilian noninstitutionalized Census population .
Unlike longitudinal cohort studies that can be used to estimate incidence (proportion of the population who develops the disease or condition over time), cross-sectional studies provide information only about prevalence (the proportion of the population who have the disease or condition at one point in time). However, a cross-sectional study can be included as the first step in a cohort study or experimental study at little cost. Cross-sectional studies are impractical for the study of uncommon diseases if the design involves collecting data from a sample of people from the general population. However, a major strength of cross-sectional studies is that they are inexpensive and can be performed quickly.
17.3.3
Cohort studies
All cohort studies involve following groups of participants over time. The hallmark of a cohort study is the need to define a group of individuals at the beginning of a period of follow-up. The study population should resemble the population to which the results will generalizable, and the number of subjects should provide adequate power (see Section 17.7 ). Because cohort studies typically analyze associations between exposures (e.g., predictors) and disease or conditions (e.g., outcomes), they are classified as analytic epidemiologic studies, but they also provide descriptive data by characterizing the occurrence of outcomes over time.
The quality of the cohort study depends on the representativeness of the cohort and the accuracy and precision of the measurements of predictor and outcome variables. If levels of predictor variables are likely to change during follow-up, these measurements should be updated. Assessment of outcomes should be performed using standardized procedures and should be blinded to predictor status.
Compared with studies using a randomized trial design, cohort and other observational studies are limited in their ability to make causal inferences and interpretation of results is often clouded by the influences of confounding (see Section 17.4.1 ) variables. The ability to draw inferences concerning cause and effect is dependent on the degree to which the investigator has identified and measured potential confounders and sources of effect modification (see Section 17.4.2 ).
17.3.3.1
Prospective cohort study
In the classic prospective cohort study the investigator selects a sample from the underlying population of interest. Persons free of the disease or condition under investigation at the time of entry into the study are classified according to whether they are exposed to the potential risk or protective factors. After defining the study sample (e.g., cohort) and measuring the characteristics (e.g., predictor variables) in study participants, the cohort is then followed for a period of time (which may be many years), and the incidence rates (number of new cases of disease per population at risk per unit time) in those exposed versus those not exposed are compared ( Fig. 17.2 ). A prospective cohort study may also involve measuring exposure status at the beginning of a study and determining how this relates to changes in a characteristic or outcome (e.g., BMD) over time.
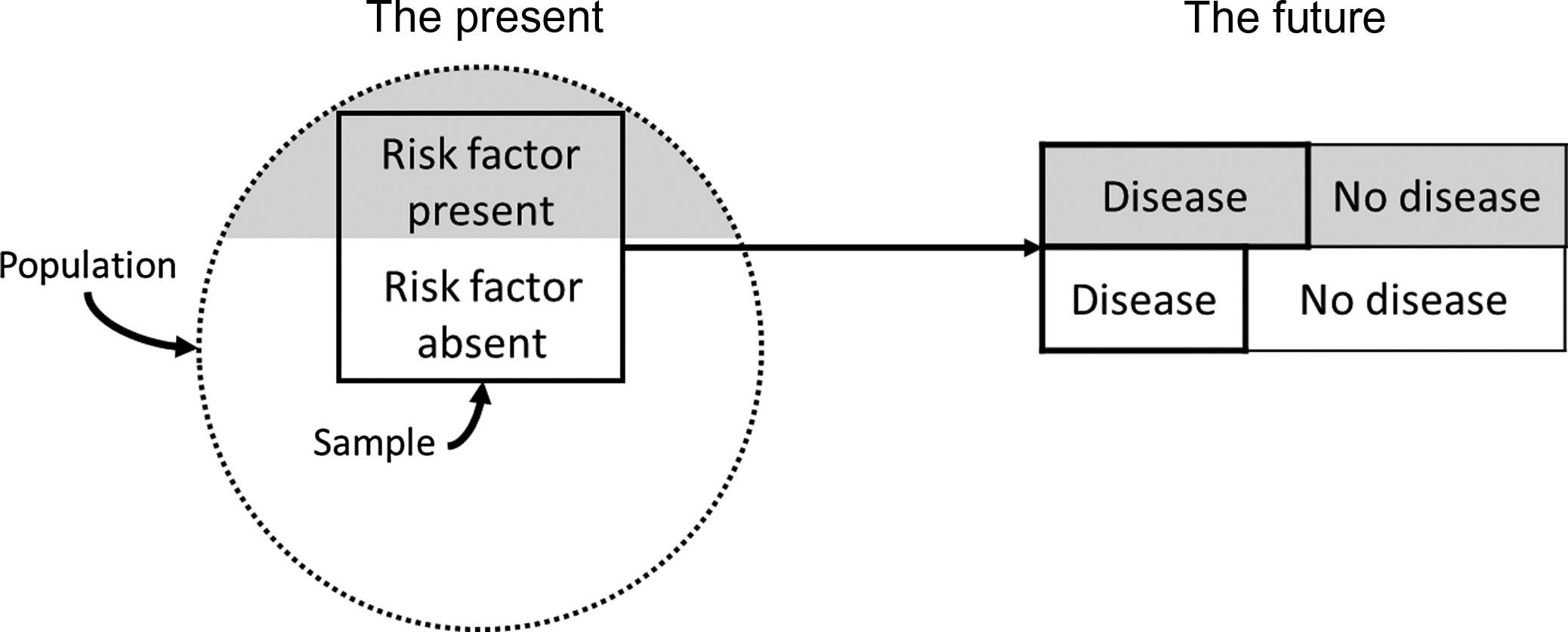
For example, the prospective cohort study, the Study of Osteoporotic Fractures (SOF) , has examined the incidence and risk factors for osteoporosis, fractures, and other age-related conditions in older women. The investigators originally enrolled a total of 9704 Caucasian women aged 65 years and older from four geographic regions in the United States (Minneapolis, Minnesota; Monongahela Valley near Pittsburgh, Pennsylvania; Baltimore, Maryland; and Portland, Oregon) between 1986 and 1988 and then expanded the total cohort to 10,366 women with the addition of 662 African-American women in 1997–98. Participants in SOF attended nine examinations and completed triannual contacts (over 95% complete during 25 years of follow-up) providing a powerful comprehensive archive of measurements (e.g., predictor variables). SOF prospectively validated outcomes of fracture, breast cancer, other age-related diseases, and mortality during follow-up. Prior to SOF, most previous studies examining risk factors for hip fractures were case–control studies that were limited by selection and recall biases . Identification of risk factors for hip fracture was a major aim of SOF. The study examined risk factors for hip fracture over the short term (4 years) ( Table 17.1 ) and long term (10 years) ( Table 17.2 ) . The investigators found that baseline characteristics, including older age, maternal history of fracture after age 50, increased height at age 25, any fracture after age 50, decreased body size (increased weight loss since age 25 or lower BMI), and lower BMD were independent predictors of risk of first incident hip fracture over the short and long term. More recently, SOF investigators reported that a single assessment of femoral neck BMD and prior fracture history predicted risks of nonvertebral and hip fractures over the subsequent 20–25 years with little degradation over time .
Relative risk (95% confidence interval) | ||
---|---|---|
Measure (comparison or unit) | Without BMD | With BMD |
Age (per 5 years) | 1.5 (1.3–1.7) | 1.4 (1.2–1.6) |
History of maternal hip fracture (vs none) | 2.0 (1.4–2.9) | 1.8 (1.2–2.7) |
Increase in weight since age 25 (per 20%) | 0.6 (0.5–0.7) | 0.8 (0.6–0.9) |
Height at age 25 (per 6 cm) | 1.2 (1.1–1.4) | 1.3 (1.1–1.5) |
Self-rated health (per 1-point decrease) b | 1.7 (1.3–2.2) | 1.6 (1.2–2.1) |
Previous hyperthyroidism (vs none) | 1.8 (1.2–2.6) | 1.7 (1.2–2.5) |
Current use of long-acting benzodiazepines (vs no current use) | 1.6 (1.1–2.4) | 1.6 (1.1–2.4) |
Current use of anticonvulsant drugs (vs no current use) | 2.8 (1.2–6.3) | 2.0 (0.8–4.9) |
Current caffeine intake (per 190 mg/day) | 1.3 (1.0–1.5) | 1.2 (1.0–1.5) |
Walking for exercise (vs not walking for exercise) | 0.7 (0.5–0.9) | 0.7 (0.5–1.0) |
On feet ≤4 h/day (vs >4 h/day) | 1.7 (1.2–2.4) | 1.7 (1.2–2.4) |
Inability to rise from chair (vs no inability) | 2.1 (1.3–3.2) | 1.7 (1.1–2.7) |
Lowest quartile for distant depth perception (vs other three) | 1.5 (1.1–2.0) | 1.4 (1.0–1.9) |
Low-frequency contrast sensitivity (per 1 SD decrease) | 1.2 (1.0–1.5) | 1.2 (1.0–1.5) |
Resting pulse rate >80 beats/min (vs ≤80 beats/min) | 1.8 (1.3–2.5) | 1.7 (1.2–2.4) |
Any fracture since age of 50 (vs more) | – | 1.5 (1.1–2.0) |
Calcaneal bone density (per 1 SD decrease) | – | 1.6 (1.3–1.9) |
a From Cummings, Nevitt, Browner, et al. by permission of the Massachusetts Medical Society.
b Health was rated as poor (1 pt); fair (2 pts); good to excellent (3 pts).
Measure (comparison or unit) | Without BMD | With BMD |
---|---|---|
Total hip BMD (−0.13 g/cm 2 ) | NA | 1.84 (1.66–2.05) |
Age (+5 years) | 1.44 (1.33–1.85) | 1.35 (1.25–1.24) |
Any previous fracture since age 50 (yes/no) | 1.57 (1.34–1.85) | 1.35 (1.14–1.58) |
History of maternal hip fracture after age 50 (yes/no) | 1.51 (1.20–1.89) | 1.43 (1.14–1.80) |
Parkinson’s disease (yes/no) | 2.21 (1.09–4.45) | 1.81 (0.89–3.65) |
Type II diabetes mellitus (yes/no) | 1.68 (1.23–2.30) | 1.83 (1.34–2.50) |
Lowest quartile for distant depth perception (yes/no) | 1.38 (1.16–1.65) | 1.34 (1.13–1.60) |
BMI using current weight vs age 25 height (−4.5 kg/m 2 ) | 1.48 (1.34–1.63) | 1.10 (0.99–1.23) |
Height at age 25 (6 cm) | 1.14 (1.05–1.23) | 1.20 (1.11–1.31) |
Nulliparous (yes/no) | 1.25 (1.04–1.51) | 1.28 (1.06–1.55) |
Walking speed (−0.22 m/s) | 1.25 (1.14–1.37) | 1.17 (1.07–1.28) |
Digit symbol test number completed (−12) | 1.20 (1.09–1.31) | 1.19 (1.08–1.30) |
a From Taylor, Schreiner, Stone by permission of Blackwell Publishing.
The Study of Women’s Health Across the Nation (SWAN) is another example of a prospective cohort study designed to examine the health of women during their middle years. Between 1996 and 1997, SWAN enrolled 3302 women aged 42–52 years representing five racial/ethnic groups from seven geographic regions in the United States for participation in a baseline examination, including hormone levels and BMD . Subsequently, 12 annual follow-up examinations, including these same measurements, have been performed. Using this data, investigators examined the association between hormone levels (e.g., estradiol, follicle-stimulating hormone, and androgens) and changes in BMD during three phases (pretransmenopausal, transmenopausal, and postmenopausal) of the menopausal transition . The investigators found that higher levels of follicle-stimulating hormone were associated with faster rates of spine bone loss during pretransmenopausal and transmenopausal phases, while lower levels of estradiol were associated with faster rates of spine bone loss in the later postmenopausal phase.
Prospective cohort studies provide valuable information about disease incidence. They have a major advantage over case–control studies in that exposures or characteristics of interest are measured prior to disease development (or before changes in an attribute take place). This design feature allows the investigator to measure predictor variables more accurately and completely than is possible using retrospective design and prevents the predictor measurements from being influenced (e.g., biased) by knowledge of outcome status. However, prospective cohort studies generally require large sample sizes, long-term follow-up of study subjects, large monetary expense, and complex administrative and organizational arrangements. The strength of the prospective cohort design can be undermined by incomplete follow-up of study participants. To minimize loss to follow-up, investigators use a number of strategies, including exclusion of potential subjects who are not likely to be available for follow-up, collecting information at baseline that facilitates tracking of participants, regular communication with participants during follow-up to keep contact information up to date, and providing information or education to participants to promote their commitment to research. Finally, the outcome of interest in a prospective cohort study must be relatively common, or prohibitively large numbers of participants will be required to ensure adequate numbers of subjects experiencing that outcome.
17.3.3.2
Retrospective cohort study
A retrospective cohort study (e.g., historical cohort study) differs from a prospective one in that the assembly of the study cohort, baseline measurements, and follow-up have all occurred in the past. In this design, investigators assemble a cohort by reviewing records to identify exposures (e.g., risk factors or predictor variables) in the past (often decades ago). Based on recorded exposure histories, cohort members are divided into exposed and nonexposed groups or according to level of exposure. The investigator then reconstructs their subsequent disease experience up to some defined point in the more recent past or up to the present time.
For instance, to estimate fracture risk among unselected community men with prostate cancer and systematically assess associations with androgen deprivation therapy and other risk factors for fracture, investigators used data from the Rochester Epidemiology Project database (a unique medical records-linkage system that encompasses the care delivered to residents of Rochester and Olmsted County, Minnesota) to identify all men with prostate cancer first diagnosed in 1990–99, allowing for a decade of more of subsequent follow-up . Olmsted County is well suited for retrospective cohort studies because comprehensive medical records for the residents are available for review, and the pertinent records can be identified through a centralized index to diagnoses made by essentially all medical-care providers used by the local population . Using community medical records, the men with prostate cancer were followed forward in time until death or the most recent clinical contact. The primary analysis compared the fractures observed at each skeletal site (based on the first fracture of a given type per person) with the number expected in this cohort during their follow-up in the community. Compared to the expected rate, overall fracture risk was elevated 1.9-fold in men with prostate cancer, with an absolute increase in risk of 9%. Fracture risk was increased even among men not on androgen deprivation therapy but was elevated a further 1.7-fold among androgen deprivation therapy–treated compared with untreated men with prostate cancer.
Retrospective cohort studies have many of the same strengths of prospective cohort studies but can be completed in a much more timely fashion and are therefore much less expensive. However, the investigator has limited control of the nature and quality of the predictor variables. Only when the necessary information on past exposure and other characteristics of interest has been accurately and reliably recorded can a retrospective cohort study be reasonably undertaken. In addition, the investigator may have limited control over the approach to sampling the population. It must be feasible to trace a large proportion of the cohort members in order to determine whether they, in fact, experienced the outcome of interest.
17.3.3.3
Nested case–control study
A nested case–control design has a case–control study “nested” within a cohort study. It is an excellent study design for predictor variables that are expensive to measure and can be assessed at the end of the study (e.g., biomarker measurement using banked baseline sera specimens) on subjects who develop the outcome of interest during the study (cases) and on a random sample of subjects who do not (controls). The investigator can increase power by selecting two or three controls for each case and by matching on constitutional risk factors for the outcome such as age and sex. The investigator begins with identification of a suitable cohort (e.g., one with banked specimens, images, or information) that has enough cases by the end of follow-up to provide adequate power to address the research question. Cases at the end of follow-up are identified first and then controls are selected. Power can be increased by selecting two or three controls per case and by matching cases and controls on constitutional determinants of the outcome such as age and sex. Once cases have been identified and controls have been selected, the investigator retrieves the specimens, images, or records that were collected before the outcome occurred and measures the predictor variable in cases and controls.
For example, a case–control study nested within the population of 205,466 community-dwelling women 68 years and older living in Ontario, Canada initiating oral bisphosphonates between April 1, 2002 and March 31, 2008 was conducted to determine whether prolonged bisphosphonate treatment is associated with an increased risk of subtrochanteric or femoral shaft fracture. Cases were women hospitalized with a subtrochanteric or femoral shaft fracture ( n =716), and each case was matched to up to five controls with no such fracture. Study participants were followed up until March 31, 2009. Compared with transient bisphosphonate use, treatment for 5 years or longer was associated with a 2.7-fold higher risk of subtrochanteric or femoral shaft fracture. Among the women with at least 5 years of bisphosphonate treatment, a subtrochanteric or femoral shaft fracture occurred in 0.13% during the subsequent year and 0.22% within 2 years. The investigators concluded that bisphosphonate treatment for more than 5 years in older women was associated with a higher risk of subtrochanteric or femoral shaft fractures, although the absolute risk of these fractures was low.
Nested case–control studies have the advantages of cohort studies that are due to collection of predictor variables prior to occurrence of the outcome and avoid potential biases of traditional case–control studies that select cases and controls from different populations. They are especially suitable for expensive measures on serum or radiographic imaging studies that have been archived at the beginning of the cohort study and preserved for future analyses. Thus in addition to cost savings resulting from not making predictor measurements on the entire cohort, the design allows investigators to perform novel predictor measurements that were not available at the onset of the study. However, many research hypotheses are not amenable to the strategy of storing data for later analyses on a sample of study subjects. If predictor measurements can be easily and efficiently performed on the entire study cohort, nothing is gained by using a nested case–control design. In addition, since characteristics (e.g., age) of controls may be matched to that of cases, rates of disease events cannot be estimated.
17.3.3.4
Nested case–cohort design
A nested case–cohort study is similar to a nested case–control study and is another method of increasing efficiency compared to a traditional retrospective or prospective cohort study. Again, the investigator selects a cohort with banked specimens, images, or information and then identifies study participants who developed the outcome during follow-up (cases). However, the controls are a random sample from the entire cohort (e.g., subcohort), regardless of outcome status. This means that there will be some cases among the subcohort, and these subjects are analyzed as cases in the analyses (removing them from the subcohort for the purpose of analyses is a negligible problem provided that the outcome is uncommon in the subcohort).
A case–cohort design is particularly useful when the associations between a serologic marker or other predictor variable and several disease outcomes are of interest. For example, using the case–cohort design nested within the prospective Osteoporotic Fractures in Men study of older community-dwelling men, investigators have used a single set of 25-hydroxyvitamin D levels from the baseline examination measured in a random sample of the cohort to determine the association between 25-hydroxyvitamin D and risk of hip and nonvertebral fractures in one analysis and risks of frailty and mortality in other analyses. In addition, the subcohort is representative of the entire cohort and therefore provides a basis for examining the estimating incidence and prevalence of disease outcome or condition in the population from which it was drawn.
17.3.4
Randomized clinical trial
In general, the strongest evidence that a given exposure is a cause of a disease is produced from experimental studies. In a clinical trial, the outcome is compared in groups of participants that receive different interventions. This design typically includes a group(s) that receive(s) an intervention(s) to be tested and a control group that receives either no intervention or a comparison treatment. In a randomized placebo-controlled blinded clinical trial of a medication, the investigator selects a sample from the population, measures baseline characteristics, randomly assigns study participants to an active treatment group(s) receiving the medication(s) or control group that receives identical appearing placebo and then follows the subjects through time to measure outcome variable during follow-up blinded to treatment assignment ( Fig. 17.3 ). Randomly assigning the intervention can eliminate the influence of confounding variables, and blinding the administration of the treatment can eliminate the likelihood that the observed effects of the intervention are due to differential use of other treatments in the active treatment and placebo group or biased ascertainment of the outcome.
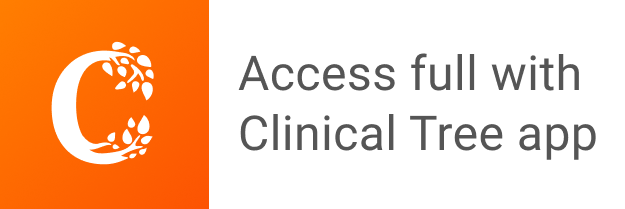