html xmlns=”http://www.w3.org/1999/xhtml”>
49
Pharmacogenomics
and
Introduction
The term pharmacogenetics is believed to have first been used in 1959 by Vogel who defined it as “the study of genetically determined variations revealed by the effect of drugs.” 1 Motulsky described the field similarly, but perhaps from the viewpoint of the clinical observer, calling the interaction “drug responses and their modification by hereditary influences.” 2 With the advent of the ability to sequence the entire human genome 3,4 —wherein technology allows one the ability to rapidly and accurately determine billions of pieces of genetic information on a given individual—the term pharmacogenetics has slowly been replaced with pharmacogenomics to denote the consideration of the entire genome. Notwithstanding the term employed, the field is as relevant as ever. It offers the promise of achieving a paradigm of personalized drug therapy based on an individual’s unique genetic makeup.
In reality, personalized care has been practiced for centuries, if one defines personalization as the consideration of all clinical, biologic, and environmental factors that make each patient unique. What changed with the sequencing of the first human genome—and through the simultaneous technological advances of profiling individuals (and, in cancer, tumors) on many levels (DNA, RNA, proteins, epigenetics)—was the expectation that “personalized medicine” would now also mean inclusion of molecular information. Some have termed this type of care precision medicine. 5 Pharmacogenomics, using that historical view, is therefore one of the oldest and most foundational pieces of precision medicine and precision therapeutics. Yet from the standpoint of how much there is still to know and implement, even after 50 years, pharmacogenomics is in its relative infancy.
Pharmacogenomics in Oncology
In cancer therapeutics, pharmacogenomic considerations are particularly relevant, for three reasons. First, many oncology drugs can have severe, undesirable toxicities. If genetic determinants or predictors of such toxicity could be identified, it might be possible to dramatically decrease undesirable harm when prescribing chemotherapy. Second, the stakes in cancer therapeutics are high. Lack of efficacy of a given treatment means loss of time, which in cancer care often is of seminal importance. Finally, pharmacogenomic considerations in oncology are complicated by the fact that there are two genomes in play: the genome of the patient (the germline or inherited DNA) and the genome of the tumor (the somatic or cancer DNA). Pharmacogenomic study has come to encompass the consideration of both, and thus it includes single-nucleotide polymorphisms (SNPs), gene copy number alterations (copy number variants, CNVs), and acquired changes (tumor mutations) as they relate to drug response or toxicity. 6,7 Somatic changes are of clear importance, including several that have led to the development of successful therapeutic interventions against a mutational event. However, for the scope of this chapter, we specifically restrict our considerations to germline genetic changes, because somatic changes reflect disease subcategorization at the molecular level, which is distinct from interindividual variability in drug response. In addition, in contrast to disease genetics, pharmacogenomics focuses specifically on predictive genetic markers of outcome from drug interventions, so we do not discuss DNA changes that potentially confer cancer susceptibility.
Even when considering only germline changes, pharmacogenomic questions have broad implications for oncology therapeutics in three very important ways:
1. In oncology, the concept of the therapeutic index is crucial. Many chemotherapy drugs were initially identified through screens for compounds that killed dividing cells. 8 Such drugs are still the mainstay of most anticancer regimens, but these cytotoxic drugs (and also even newer anticancer drugs that may work differently) are limited by possessing a narrow therapeutic index: the ratio between the dose that results in toxicity to the majority of individuals taking the drug, compared to the dose that results in effect in the majority of individuals taking the drug. It is therefore rational to consider pharmacogenomic factors as potential determinants for drug dosing for chemotherapy drugs, in which relatively small changes in the dose can have dramatic undesirable effects (shifting the therapeutic index toward toxicity). Examples of this are provided later in the chapter.
2. In related fashion, it might be straightforward to understand how germline pharmacogenomic variants can be relevant for predicting the likelihood of toxicity from a drug, because germline variation in genes important for drug metabolism and excretion in the host might directly confer differences in enzyme function within these pathways. Indeed, many of the current, best studied examples of pharmacogenomic findings in oncology relate to toxicity predilection. 9 However, it has been interesting to also find that germline polymorphisms may affect response to treatment in malignancies. We explore ways that this occurs in this chapter. The important point is that germline pharmacogenomics has concrete promise for defining both toxicity and response in cancer therapeutics.
3. Germline pharmacogenomic discoveries have the potential to identify new oncology drug targets. Although this area remains one of ongoing research, germline mutations such as BRCA1 may allow individuals with cancer to be more susceptible to certain types of DNA-damaging agents, such as poly(ADP-ribose)polymerase (PARP) inhibitors. 10,11 While drugs identified in this way remain under development, the number of examples like this is likely to increase, especially as more is learned about inherited changes in DNA structure and variation.
In this chapter we illustrate explicit examples of the first two points. However, we encourage the reader to simultaneously consider how, from the examples provided, opportunities for new drug discovery/identification of new drug targets (point 3) can also arise through the study of pharmacogenomics.
Genotyping and Phenotyping
Pharmacogenomic discovery and clinical implementation require the technical ability to accurately measure genotypes—the DNA sequence variation at specific genetic loci or regions. Most germline DNA variations fall into the class of SNPs, or single base-pair changes that occur with some relative prevalence in the human population (for example, a change from a guanine (G) to adenine (A) in the DNA strand [i.e., G/A polymorphism] in more than 1% of all individuals). Numerous technologies have been developed to perform genotyping and decipher such information at the DNA level. 12 Combining genetic data and associating it with a phenotype—an outward characteristic or trait—is where genomic information becomes valuable. Genotype-to-phenotype associations lie at the heart of pharmacogenomics, wherein all phenotypes of interest relate to drug effects (toxicities, response, drug levels in the blood, or drug effects on other biomarkers of drug action in the body). Clinical examples might include extreme drug phenotypes such as death, intermediate phenotypes such as development of severe hypertension, or more subtle phenotypes such as drug-induced hyperglycemia. The importance of accurate phenotyping also cannot be understated. Pharmacogenomic associations may not be detectable if phenotypes are not carefully defined and measured (ideally prospectively), or if important covariates such as cumulative drug dose are not considered. Similarly, relevant pharmacogenomic effects could be obscured by inappropriately combining phenotypes that are actually distinct. 13
Pharmacogenomic Discovery Approaches
Single-Gene Approaches
Initial pharmacogenomic discovery methods typically consisted of “single-gene” approaches. These approaches presuppose that a given single gene (with purported a priori importance) is relevant to that drug’s metabolism or action. Typically, these candidate genes code for enzymes involved in the mechanistic pathway of a drug’s action or its metabolism. Variants within the candidate gene are then genotyped and analyzed in relation to a given drug phenotype.
The paradigmatic story of irinotecan pharmacogenomics is a classic single-gene example. Irinotecan is an intravenous chemotherapy drug first studied clinically in the late 1980s. 14,15 It is now used widely in the treatment of colorectal cancer. Importantly, irinotecan is a prodrug, requiring activation (through the action of carboxylesterase-2) to SN-38, an inhibitor of topoisomerase I, and then inactivation to SN-38 glucuronide (SN-38G) for elimination. 16,17 The stage for the pharmacogenomic story began with a phase I study conducted at The University of Chicago, which examined irinotecan on a weekly schedule and which suggested that the extent of glucuronidation to SN-38G was inversely correlated with irinotecan’s dose-limiting toxicity. 18 Based on the fact that pharmacogenetic variation had been suggested to be related to toxicity for a few other cancer drugs at the time, 19–21 it was hypothesized that genetic factors resulting in reduced glucuronidation may be a cause of predisposition to irinotecan toxicity. 18 Studies in liver tissue from patients with a genetic deficiency of bilirubin glucuronidation (Crigler-Najjar syndrome) were conducted, and it was found that these liver tissues were unable to form SN-38G. 22 However, transfection with cDNAs encoding the enzyme UDP-glucuronosyltransferase 1A1 (UGT1A1) resulted in SN-38G formation. 22 This result was intriguing, because a genetic polymorphism in UGT1A1 had recently been identified that was associated with variation in glucuronidation. 23,24 The polymorphism is now known to be an insertion/deletion in the UGT1A1 promoter (the “TATA” box) that reduces transcription of the gene, such that the resulting enzyme activity is inversely correlated to the number of TA repeats. 25 Six repeats is considered the wild-type (normal function) genotype, and individuals typically have either 6 or 7 repeats, most commonly (5 and 8 are rare). 26 Using a special pharmacogenomic nomenclature, the 6-repeat genotype is termed ∗1, whereas having 7 repeats is called ∗28.
Given that there was a candidate polymorphism, an in vitro pharmacogenetic study was next conducted. Liver tissues were again used to test the hypothesis that genetic variability in the UGT1A1 promoter was associated with SN-38G formation (which it was), 27 and then, importantly, with irinotecan toxicity in patients being treated with the drug. 28 The latter was further demonstrated (specifically an association with neutropenia from irinotecan) in a larger study of irinotecan-treated patients (350 mg/m2 every 3 weeks). 29 Severe neutropenia was significantly more common in patients with two copies of the ∗28 variant ( ∗28/∗28; 50% of such patients had severe neutropenia) compared to only 12.5% in those with a ∗1/∗28 genotype, and no patients with severe neutropenia among those having ∗1/∗1 genotypes. The relative risk of severe neutropenia was 9.3 (95% confidence interval 2.4-36.4) for the ∗28/∗28 patients. 29 The authors 29 and, later, others 30 also confirmed the metabolic role of UGT1A1 with supportive pharmacokinetic information.
Based on the data, the FDA drug label for irinotecan was revised in 2005 to include the pharmacogenomic information about UGT1A1. It recommends that patients with the ∗28/∗28 genotype should be treated with a reduced starting dose of irinotecan. 31
A large study including 250 metastatic colorectal cancer patients went on to redemonstrate the pharmacogenomic importance of UGT1A1, finding an odds ratio of risk of approximately 9 for those with the highest risk genotype ( ∗28/∗28) for developing immediate severe neutropenia after irinotecan, although the pharmacogenomic relationship did not persist for subsequent treatment cycles of irinotecan. 32 A meta-analysis of the data was performed and published in 2007. 33 The meta-analysis included nine studies and a total of 821 patients. It confirmed the importance of ∗28 homozygosity. However, it interestingly suggested that the pharmacogenomic effect was most important for patients receiving higher doses of irinotecan (150 mg/m2 or higher). At lower doses (doses that are still in the commonly used range), there was no statistically significant increased risk for patients with the ∗28/∗28 genotype.
Given these nuances and concerns about the predictive power of irinotecan pharmacogenomics during initial dosing, some have argued that UGT1A1 genotyping cannot be justified as a mandatory test in every patient for the purpose of initial dosage adjustment. 34 Indeed, UGT1A1 ∗28 does not meet the typical standards for clinical specificity and sensitivity required of most diagnostic tests (one analysis suggested that its median positive predictive value was 50% [range 15% to 57%] and median negative predictive value was 85% [range 79% to 96%]). 26 Moreover, the ∗28 genotype alone is not sufficient to describe risk in individuals of some ethnic backgrounds, since the ∗6 allele also results in decreased UGT1A1 activity. 26,35 Nonetheless, the test clearly has potential clinical value in ethnic groups with a high prevalence of the ∗28 genotype and when high doses of irinotecan are planned, but these latter considerations emphasize the need for ongoing study of ways to improve the understanding of toxicity risk with irinotecan administration. Including other risk alleles within UGT1A in a haplotype-based analysis may increase the predictive value, because several other variants in these genes have now also been shown to alter enzymatic activity and affect irinotecan-related outcomes. 36
Pathway Approaches
Pathway approaches use the rationale that a single gene may be of limited lone importance from a pharmacogenomic standpoint, because few drugs are metabolized by or have mechanisms involving only one gene product. In other words, most drugs are likely to be under multigenic control. 37 Therefore, pathway approaches hypothesize that a set of genes defining a specific metabolic or mechanistic pathway might sensibly be interrogated as a composite set, for pharmacogenomic relevance. Typically, investigators will select various polymorphisms of interest that have potential biologic relevance in the genes of interest (e.g., exon SNPs that change the coding sequence, or SNPs in promoter regions)—or, less specifically, they will select SNPs that “tag” regions across the genes of interest. Despite the reasonable rationale, to our knowledge this approach to pharmacogenomics has yet to yield any salient, clinically important results. Such studies often suffer from the problem that multiple SNPs are being tested, meaning that there is limited power for demonstrating statistical significance of any one SNP in small sample sizes (see further discussion of this later).
Genome-Wide Approaches
Single-gene approaches like those described earlier have the advantage that the analyses are conducted on very focused areas of the genome, areas having potentially the highest relevance. Typically, some evidence already exists that the gene is important on a functional level, usually through studies showing that the gene product is under differential expression or that the gene product is key to determining the pharmacokinetics of a drug. When only a single gene or even a few genes (as in pathway approaches) are being considered, only a limited amount of genomic information must be tested and assimilated using bioinformatic techniques.
In contrast, genome-wide association studies (GWAS) take an unbiased approach to pharmacogenomic discovery. These are open to the possibility that any gene is important (unbiased) and they can identify new genetic targets (i.e., they are hypothesis generating). The limitations of GWAS include a need for vast computational ability, the possibility of false discovery (explained further later), and, typically, a requirement for genetic information on a large number of individuals to identify compelling associations.
One well-executed example of the power of this approach is illustrated by the work of Yang and colleagues. 38 The authors set out to identify germline genetic factors that might predict therapy response in pediatric acute lymphoblastic leukemia (ALL). They and others had observed that significant interindividual heterogeneity existed for treatment response in ALL, and that most prior work had focused on tumor-related factors which might explain such variation. The authors hypothesized that unidentified germline (host) factors might also be important. Indeed, one pharmacogenomic factor had already been well described for a drug commonly used to treat ALL and other leukemias—6-mercaptopurine—although the pharmacogenomic relationship was that of the role of TPMT (thiopurine methyltransferase) activity in predicting severe toxicity (bone marrow suppression) from that drug, 39,40 not treatment response. The story of TPMT/6-mercaptopurine (and, relatedly, TPMT/thioguanine) toxicity pharmacogenetics is one of the earliest and best-characterized examples of the potential utility of pharmacogenomics. The FDA labels for 6-mercaptopurine and thioguanine have been revised to include TPMT pharmacogenetic information, 41 and some hospitals have implemented routine ordering of TPMT testing before administration of these agents. 42 This pharmacogenomic relationship had been identified through a candidate gene approach.
Yang and associates, however, wanted to determine whether there were germline factors predictive of treatment response in ALL. 28 Rather than restricting their analysis to any one candidate gene, they performed a GWAS of more than 470,000 germline SNPs to identify genotypes that associated with increased risk of minimal residual disease (MRD) in two independent cohorts (318 children and 169 children) with newly diagnosed ALL. They identified 102 SNPs associated with MRD in both cohorts. 38 Twenty-one of these SNPs were also associated with antileukemic drug disposition, strengthening a plausible link between MRD eradication and greater drug exposure. Many SNPs were in regions never previously studied as potential pharmacogenomic loci. Interestingly, five of the top associated SNPs were located in the interleukin 15 gene (IL-15) gene. 38 IL-15 was previously shown to protect lymphoid tumors from glucocorticoid-induced apoptosis in vitro, 43 and IL-15 expression in ALL blasts had been linked to central nervous system (CNS) involvement at diagnosis and an increased risk of CNS relapse. 44 Therefore, the link between germline SNPs in IL-15 and worse outcomes, for several possible reasons, seemed strong. Because the authors did not (for obvious reasons) have an untreated control group in their study, it was impossible to say whether these IL-15 germline SNPs were truly pharmacogenomic SNPs (predictive of residual disease through their action on antileukemic drug action) or prognostic SNPs that conferred a worse outcome, regardless of treatment. This points to how GWAS approaches can, simultaneously, yield powerful results and be hypothesis-generating, encouraging further follow-up of the role of IL-15 in this disease and its treatment. This point notwithstanding, at least some of the top 102 SNPs in this study were linked to antileukemic drug disposition and can therefore be characterized as pharmacogenomic, showing the power of this approach as a pharmacogenomic discovery tool.
Whole-Genome Sequencing (WGS)
Very recently, WGS methods have begun to become more commonplace. 45 This approach has the advantage of theoretically identifying every piece of genetic information (and also variation) across the entire genome, including every base pair, deletion/insertion, and copy number change. GWAS approaches, in contrast, classically cover only a limited number of such variants across the entire genome. Concrete examples of applications of WGS to pharmacogenomics are still currently awaited.
Bioinformatic Considerations
Last, it is important to describe the potential for false discovery in pharmacogenomic analyses. This is the inherent possibility that a variant will be found to be statistically associated with a phenotype by chance alone. 46–48 When one is assimilating millions or even billions of pieces of genetic information at once (as in GWAS and WGS methods), the sheer number of association tests being performed is so large that one must adjust the threshold of statistical significance that defines a positive result. 46 As an additional measure of sorting true-positive findings from false positives, it is typically accepted that high-quality pharmacogenomic results are those for which the association can be replicated in an independent population. 49 Here again, assurance that the phenotypes match and were collected in similar fashion is an important methodological step.
Clinical Relevance of Pharmacogenomic Findings During Implementation
A number of oncology drugs have been studied to uncover pharmacogenomic effects, 9,34 and other well-validated oncology drug-gene interactions exist (TPMT/6-mercaptopurine; 5-fluorouracil/DPYD). An even greater number of promising associations have been described for many other drugs that await further characterization and validation.
What remains to be further elucidated for many of these associations is the degree to which individual associations have an effect on a clinical outcome when assimilated within the larger context of all variables that affect a therapeutic decision. By this we mean: when a clinician makes a prescribing choice, he or she already is used to—and is accomplished at—incorporating many factors to make the best drug choice. These include all of the elements of “traditional” personalized care: clinical factors about comorbidities, organ function, allergies, and drug-drug interactions; biologic factors such as disease subtype, age, and gender; and environmental factors such as habits, social environment, and compliance. The question is whether a specific pharmacogenomic association for a drug’s toxicity or response likelihood—which is probabilistic by its very nature and is usually identified in a large cohort showing an overall effect—will matter for an individual patient when the clinician has to assimilate the pharmacogenomic information along with all other treatment factors, including potentially other important genetic factors. In many respects, it is a question that resolves around the effect size of the pharmacogenomic effect. The UGT1A1 example—where the dose of the drug being administered (irinotecan) seems to matter as to whether the pharmacogenomic effect is observable 33 —may be one example of this dynamic and delicate interdependence between genetic effects and other clinical effects (such as dose) interacting (or one predominating) to determine the clinical phenotype.
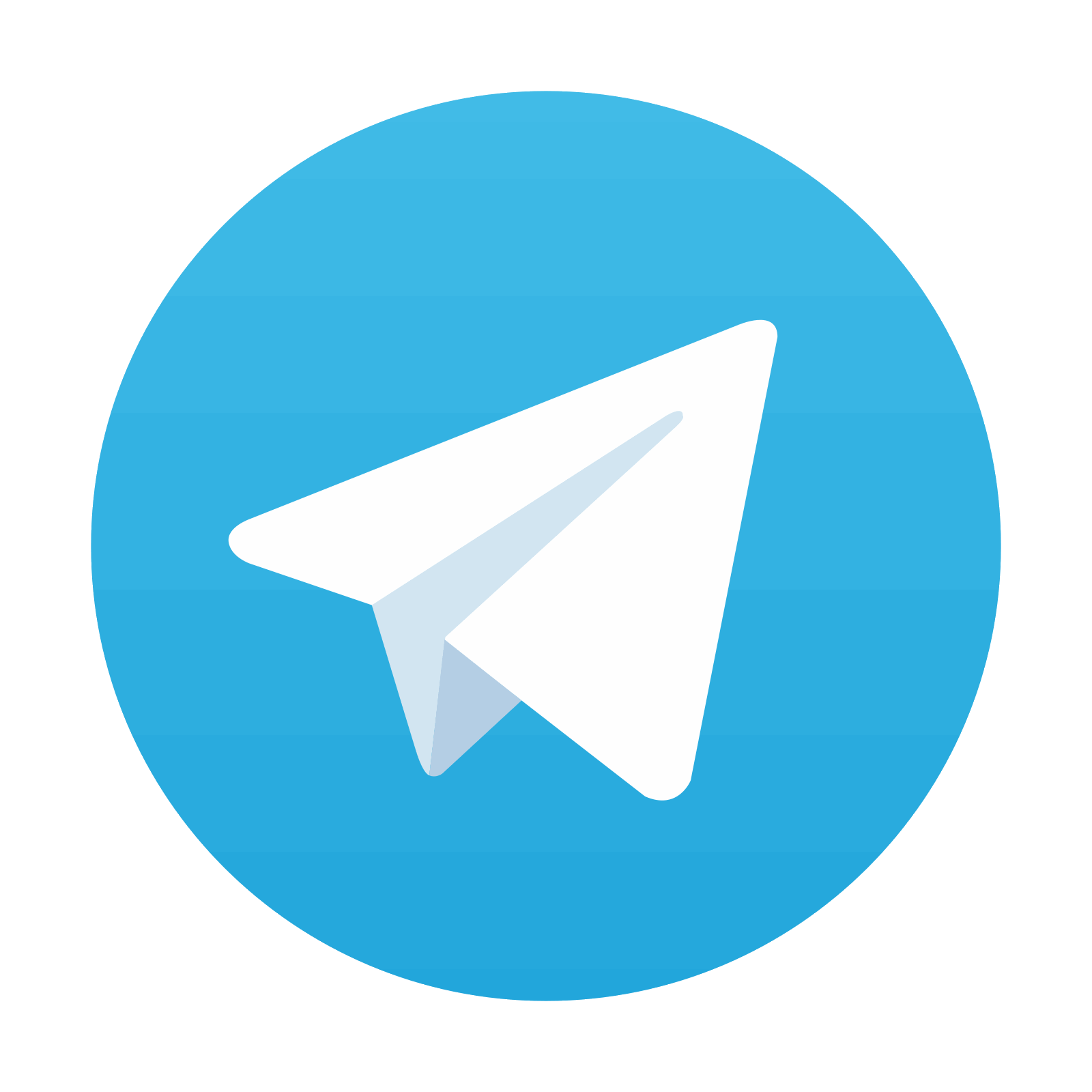
Stay updated, free articles. Join our Telegram channel

Full access? Get Clinical Tree
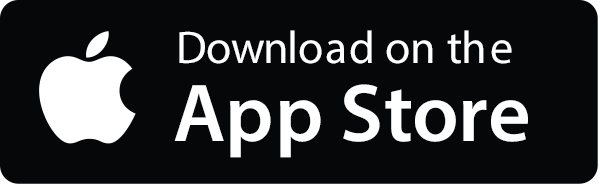
