Abstract
Since completion of the Human Genome Project in 2001, dynamics in human genetics have greatly accelerated. Sequencing a human genome used to be a multimillion dollar project but is now reduced to less than $2000 and increasingly becomes routine in research and clinical applications. Disease gene discovery has greatly accelerated and the importance of rare and “private” alleles is evident, which can only be identified by sequencing. Clinical genetic testing is currently going through radical changes, leading to comprehensive, yet still more affordable tests. The optimism is such that the National Institutes of Health (NIH) and other organizations have targeted the identification of possibly all Mendelian disease genes in the coming decade. New challenges have come into focus, including managing very large amounts of data, translation into clinical electronic record systems, genomic data sharing, data security, and others. Regardless, the enthusiasm resulting from decoding the entire human genome is beginning to play out in applications for research and patient care. This chapter will introduce a few key aspects of this, still new, age of genomics.
Keywords
Human Genome Project, genomics, in silico scores, data sharing, GENESIS/GEM.app, $1,000 genome
Overview
Since completion of the Human Genome Project in 2001, dynamics in human genetics have greatly accelerated. Sequencing a human genome used to be a multimillion dollar project but is now reduced to less than $2,000 and increasingly becomes routine in research and clinical applications. Disease gene discovery has greatly accelerated and the importance of rare and “private” alleles is evident, which can only be identified by sequencing. Clinical genetic testing is currently going through radical changes, leading to comprehensive, yet still more affordable tests. The optimism is such that the National Institutes of Health (NIH) and other organizations have targeted the identification of possibly all Mendelian disease genes in the coming decade. New challenges have come into focus, including managing very large amounts of data, translation into clinical electronic record systems, genomic data sharing, data security, and others. Regardless, the enthusiasm resulting from decoding the entire human genome is beginning to play out in applications for research and patient care. This chapter will introduce a few key aspects of this, still new, age of genomics.
From human genetics to genomics
While the terms genetics and genomics are often used interchangeably, they have different meanings. Genetics refers to the science of inheritance, such as Mendelian laws of inheritance, and is generally more focused on chromosomal regions and specific genes. Genomics plays out on the scale of entire genomes and is equally important for classic inheritance traits and so-called complex disorders. The relatively new technical applications of genomics include whole genome sequence, exome sequencing, and genome-wide association studies. Before the availability of whole genome sequencing techniques, geneticists had to invent ingenious methods of mapping and linking smaller portions of the genome to a phenotype of interest. For Mendelian phenotypes, the most successful approach was linkage studies. In brief, several hundred marker alleles are measured across a genome in single or multiple families. A genetic marker allele that cosegregates with the phenotype of interest should be in linkage disequilibrium with the actual disease-causing mutation. If several consecutive markers cosegregate, we speak of a haplotype. This can be statistically formalized and expressed with a LOD score. Once established, a linked genomic region may be followed up in great detail by focused DNA sequencing studies. Until 2009, this was the only broadly applicable method of identifying novel disease genes and had enormous successes. However, the need for large families and homogeneous phenotypes contributed to the limits of this approach. In 2009, the new method of whole exome sequencing was broadly introduced ( Fig. 30.1 ).
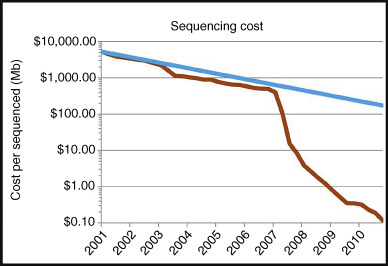
Whole exome sequencing allows sequencing of most of the nucleotides of nearly all protein coding genes in a single individual. With this single base pair resolution of measurement, the actual disease-causing variant, instead of a nearby marker allele, becomes the very target of the search. A formal linkage analysis is not necessary anymore, but may still be performed as supporting evidence. A typical exome yields approximately 20,000 coding changes, with 10,000 being nonsynonymous changes altering protein sequence. Now the task becomes a filtering effort to zoom into the most likely candidate variant and gene. Popular filtering approaches include allele frequency (only very rare or truly novel alleles might be considered), conservation across species (changes of highly conserved nucleotides are more likely to have a biological effect), or segregation studies according to the expected traits (a much smaller number of coding homozygous variants exist in a human genome). Many more considerations may be used and typical annotation software packages provide dozens of annotation criteria for each single nucleotide variant.
An example of an innovative approach to the problem of sporadic patients with a suspected dominant trait is the search for de novo changes. De novo mutations arise in every meiosis at the order of less than a dozen variants per genome. The likelihood that such a variant falls on a functionally highly relevant position is very small. By exome sequencing both parents and an offspring with a phenotype (trio-sequencing), one can explore de novo variation systematically and at scale. While it can be daunting to sort out sequencing errors from the exceptionally rare de novo hits, recent studies in autism have been especially successful in applying this approach.
To further improve these approaches, new in silico scores have been developed. Such scores predict consequences of genetic variation on protein function. Examples are SIFT, MutationTaster, and MutationAssessor. In addition, statistical considerations may go into filtering approaches and include new approaches such as the CADD and VAAST score.
As sequencing cost continues to decrease, whole genome sequencing will become the next big wave of exploring individual genomes in 2015 and thereafter. This will lead to the clarification as to what extent noncoding variation in known (and new) disease genes contributes to strong genetic effects. Today, noncoding variation is rarely tested in clinical settings because of limited understanding of the significance. In addition, whole genome sequencing will identify a very large number of small insertions/deletions. As of now, there is no comprehensive knowledge on indels larger than ∼50 bp and smaller than 1,000 bp in the population and in patients in particular. Yet, estimates suggest that this is the most abundant class of structural changes.
To sift through the large number of variations that is detected by any larger-scale genome sequencing study, statistical approaches have been developed. The most successful ones still focus on genes and the protein coding regions. The general idea is to compare the “mutational load” in a given gene in a control sample to a set of cases. These “burden tests” also aim to reduce the background noise of neutral variation by prefiltering variation according to the same conservation and protein prediction algorithms mentioned above. While some studies were successful in identifying strong signals with these methods, it appears that very large sample sizes will be necessary to fully detect genetic signals.
The limits of all these approaches lie in statistical power considerations (sample size), nongenetic contributors in the environment, and also epigenetic factors that are not measured by DNA sequencing. The combination of multiple different “genomic” datasets (whole genome sequencing, RNA sequencing, histone sequencing, proteomics, etc.) will therefore be a promising way forward.
Excess of rare variation in the human genome
Large-scale exome sequencing studies have shown that the human genome, on a population level, contains an enormous number of rare variants. “Rare variants” are not strictly defined, but refer to DNA changes that occur at levels from <1% allele frequency to changes identified only in a single person (“private changes”); such frequency assessments may be confounded by ancestry as some alleles are only rare or frequent in specific populations. While “rare variants” are infrequent individually, they actually provide by far the largest group of DNA variation. In a sample of 6500 exomes of European and African ancestry, the protein-coding regions contained a change every 21 nucleotides. Approximately 86% of all single nucleotide variants in this study were measured in less than 0.5% of alleles and 82% were population specific (European/African ancestry). Estimates have been published that predict that in 1 million exomes, every other coding nucleotide will be a variant compared to the NCBI reference in at least one exome of such a sample. Given that the human species today encompasses more than 7 billion genomes (>14 billion copies of each chromosome), it is safe to assume that every nucleotide will be changed in at least one individual as long as its functional impact is not detrimental to life. This “excess” of rare variation in the human genome represents a rich opportunity to discover disease-associated alleles. On the other hand, it presents a high level of “noise” in large-scale statistical studies of rare variation. Pure statistical approaches will require very large numbers of participants and may still not be able to discern more rare causes of human disease. It will therefore be important to develop innovative approaches to disease allele discovery. Combining different techniques to gather evidence from genetic, expression, in silico pathway modeling, and functional genomics studies is likely the most immediate way forward. More audacious concepts have proposed the large-scale functional mutational analysis in cell-based assays. Enabled by more effective gene editing via CRISPR/Cas9, such assays may explore a large part of the theoretical “mutational space” of the human genome ( Fig. 30.2 ).
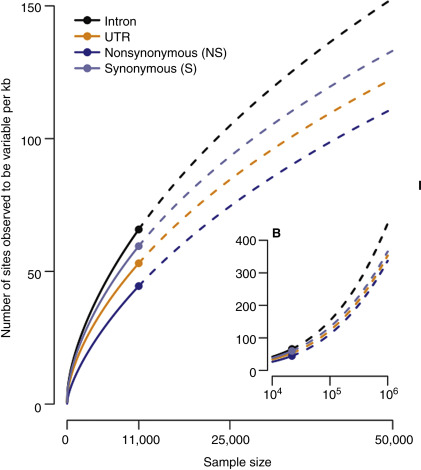
Data sharing becomes essential
Genome sequence data is increasingly recognized as a value beyond individual studies. Individual genomes may be used across different studies as controls or to search for broader phenotypic expressions of disease genes. Further, as rare variation is becoming the driving force for clinical applications, more genomes from different ancestral backgrounds are available for comparison to specific alleles. The idea of data sharing on a global level is quickly catching up. NIH has begun to enforce DNA sequence data to be deposited on dbGAP. The ClinVar and HumanVariome initiatives are collecting clinically relevant variation at a large scale and in a transparent fashion. The latter two initiatives place a strong focus on connecting phenotypes to alleles.
Researchers working on the identification of novel disease genes often do not have access to the necessary number of patients and extended families. With increasingly only small families available and new genes generally being very rare, the problem of finding “a second pedigree” with supporting evidence for a new and rare disease gene is increasingly recognized and has spurred several initiatives to address this issue. The underlying concept is that a rare disease gene still needs significant genetic evidence and should ideally be supported by more than one pedigree. Otherwise, the literature will soon be dominated by single patient/single-family gene reports of uncertain significance.
Over the past four years, the Zuchner lab at the University of Miami has developed a tool that is designed around data sharing. This Genome Management Application (GENESIS/GEM.app) holds all variant data in a cloud-computing environment. This allows for worldwide access via any web browser, any internet-connected device, and response times comparable to a Google search. Individual investigators or large consortia are able to deposit genome-level data. The uploading investigator/organization stays in full control of their data; yet, easy sharing controls allow data access for other investigators with a few mouse clicks. The data never leave the database, making it possible also to revoke access rights and to create truly ad hoc collaborations. With now over 6,000 exomes and genomes from well-defined patients with over 100 different diseases, more than 500 researchers from 38 countries have already registered. This system has allowed for the discovery of over 60 novel disease genes in the past 30 months. The flexibility and ease of use offers a complementary approach to the often more rigid rules of formal consortia.
Since a small but growing number of tools and variant databases exist, the need for data searches across these resources grows. A new initiative supported by the Global Alliance for Genomics and Health is the Genomic Matchmaker Exchange ( http://matchmakerexchange.org ). This effort is creating a standard computer interface (API) that will allow for querying across databases in a secure fashion. Every database that contains phenotype or genotype information will be able to implement this API and become part of a global virtual genomics network ( Fig. 30.3 ).
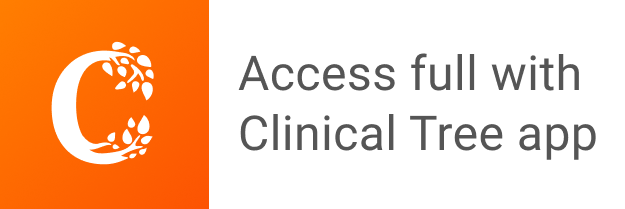