Fig. 13.1
Dexcom G4, Navigator and Medtronic Enlite sensor equipments are shown
The combination of an insulin pump and RT-CGM turns the two devices into a new form of therapy, the so-called sensor-augmented pump therapy (SAP).
13.3 Sensor Accuracy
CGMs are not as reliable as those provided by standard fingerprick blood glucose devices. Some of these differences are physiological, such as the time lag during rapid glucose changes, but at other times sensors deviate from reference glucose because of inaccurate calibration, pressure dropouts, sensor dislodgment, and other artifacts [2].
Although CGM readings are still less accurate than capillary glucose measurements, they provide a broader view of daily glucose fluctuations. A real-time GCM usually provides alarms for all readings below or above a preprogrammed target range in order to allow corrections for hyperglycemic peaks or rapid interventions in the event of a potentially severe hypoglycemia [12]. For these reasons, it is paramount that a sensor reads glucose values as properly as possible; in fact, the effectiveness and safety of CGMs are dependent on their accuracy and reliability.
Evaluating the accuracy of a sensor is a complex process. Evaluating the fluctuations of blood glucose only in an indirect manner, i.e., by measuring actually fluctuations of glucose levels in the interstitial compartment and not directly in the blood, creates a series of problems to face sensitivity, stability, and calibration of the instrument and physiological delay between blood and interstitial glucose [13].
Many numerical approaches have been proposed for the assessment of accuracy. The main parameters are:
RD (relative difference): the difference between the value of blood glucose and the one provided by the sensor (it can have positive or negative value in case of over- or underestimation).
AD (mean absolute difference): the average of the absolute difference between the value of blood glucose and the one provided by the sensor (only in absolute terms, without indication of over- or underreading).
MARD (mean absolute relative difference): the average of the absolute difference between the value of blood glucose and the one provided by the sensor as a percentage.
ISO criteria (International Standard Organization): they require that a blood glucose value by the sensor be within 15 mg/dl compared to the value for blood glucose levels, when blood glucose is less than 100 mg/dl or within 20 % for blood glucose values above 100 mg/dl [14]. By this definition, sensor accuracy is defined as the percentage of values meeting these requirements.
CG-EGA (continuous glucose error grid analysis): In 1987, Clarke first reported a novel method for the assessment of the accuracy of the reading of blood glucose by blood glucose meters (Clarke error grid analysis). This method was innovative because it took into account not only the difference between the system generated and reference blood glucose values but also the clinical significance of this difference [15–17]. In 2004, a method specifically developed for sensors (CG-EGA) was developed [18]. The grid is divided into zones that identify different levels of acceptability of clinical errors. Depending on the relationship between the sensor readings and laboratory determinations, each point lies in areas of the graph indicative of varying degrees of accuracy: zone A = good accuracy, zone B = error without any clinical consequences, and zones C, D, and E in which the error becomes progressively less acceptable. The experiences with existing monitors have provided good results, with percentages of reliefs falling within areas A + B above 95 %.
Suboptimal accuracy and reliability remain one of the biggest obstacles of glucose sensors. The devices on the market can generally reach a MARD 18 % or less [19] (Fig. 13.2); however, both transient (temporary loss of signal or increase of sensitivity of the instrument, mechanical alterations, etc.) and persistent (calibration errors or incorrect storage or transport of the sensors, etc.) deviations of great width may still occur. When a sensor overestimates a glucose level, the persistent alteration poses the greatest risk for closed-loop systems, namely, delivering excessive amounts of insulin increasing the risk of hypoglycemia [20].
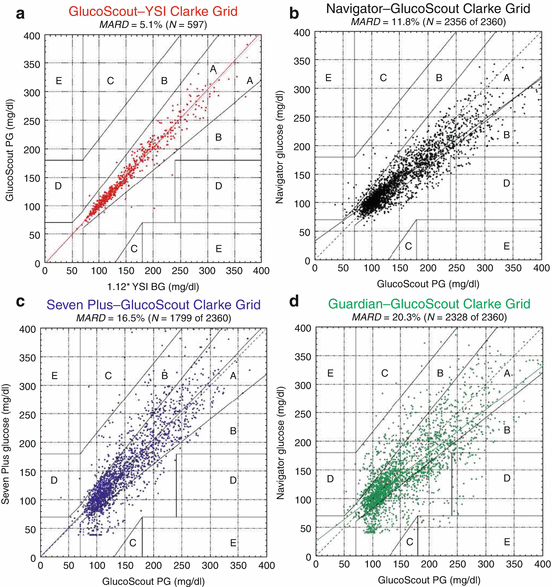
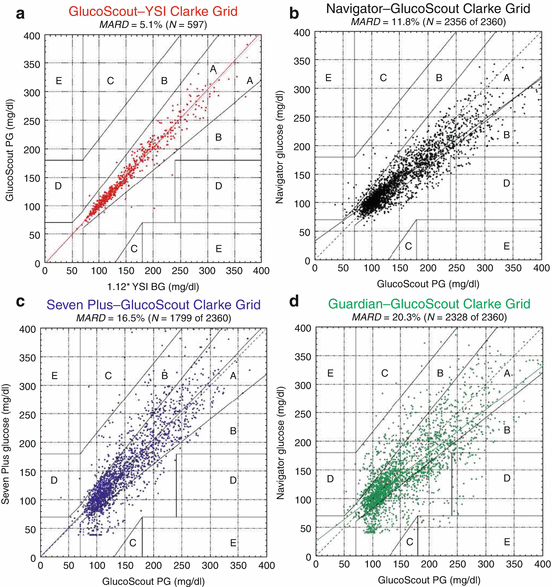
Fig. 13.2
Clarke error grid analyses and MARDs of venous plasma glucose (PG) measured by GlucoScout (a), with venous BG measured by the YSI designated as the reference and CGMS measured by the Navigator (b), the Seven Plus (c), and the Guardian (d), with venous PG measured by the GlucoScout designated as the reference (From Ref. [19])
13.3.1 Possible Reasons of Errors
All models of sensors (with the exception of FreeStyle Libre) still require a period of initial calibration (usually 2 h) and daily calibrations (depending on the model) based on capillary blood glucose checks. The initial calibration phase is particularly delicate because that could depend on the quality of the sensor performance during the entire monitoring. In fact, although the positioning of the sensor takes place in an almost atraumatic way for the subject, the insertion of the catheter into the subcutaneous space also determines a local reaction that requires a waiting period so that the signal of the sensor is stabilized. Daily calibrations have to be performed every 8–12 h to maintain the accuracy and precision of reading. All calibrations should be carried out in periods of relative stability of blood glucose in order to avoid errors in sensor reading due to the time lag between blood and interstitial glucose concentration. Other reasons that could influence the sensor performance are represented by interactions with cells and substances in the subcutaneous space, the presence of proteolytic enzymes and free radicals, and skin bleeding during the connection.
Local pressure on the sensor, occurring, for example, during sleep, may provoke a local change in hydrostatic pressure, therefore preventing glucose to move toward the interstitial fluid: the measured glucose value may result falsely low [21].
13.4 Evidences from the Literature
The first wide (large) study focused on the utility of CGM use in children, adolescents, and adults with T1DM was sponsored by JDRF and published in 2008 [22]: CGM can be associated with improved glycemic control, but the benefit was strongly related to age. In fact, HbA1c levels decreased about −0.53 % in adults, −0.08 % in adolescent, and −0.13 % in children after the 26-week follow-up. The authors partially tried to explain these results considering the frequency of sensor use: whereas 83 % of adults wore the sensor for at least 6 days/week, only 30 % who were 15–24 years of age and 50 % who were 8–14 years of age did the same. Several studies [23–25] reported a lack of improvement in metabolic control with the use of GMC hypothesizing the reason in a progressively decrease of its use during everyday life in children and adolescents with T1DM. Nevertheless, parental satisfaction with CGM is high, because of the rapid detections of blood glucose fluctuations (hypoglycemic episodes and hyperglycemic peaks) [24]. Hypoglycemia and the fear of it remain the main barriers to a strict adherence to insulin treatment. The utility in the detection of asymptomatic hypoglycemia (especially during nighttime) had been already highlighted in some studies using retrospective systems of CGM [9, 26]. Several studies affirm that glucose sensors can detect more hypoglycemic episodes compared to self-monitoring blood glucose (SMBG) allowing more frequent and precise insulin adjustments [27–29]. Although the use of GCM did not resolve the problem of severe hypoglycemic episodes and its relatively high frequency, the sensor ability of early and promptly signaling hypoglycemia has potentially the benefit of allowing intervention in order to avoid or prevent it. Even well-controlled T1DM subjects can benefit of the use of CGM, maintaining HbA1c levels in target range [30]. Pickup et al. [31] published a meta-analysis collecting six randomized controlled trials comparing glycemic control and hypoglycemia in subjects with T1DM on intensive insulin therapy where either RT-CGM or self-monitoring of blood glucose was used throughout the study for at least 2 months. They reported that CGM was associated with a reduction in HbA1c levels (−0.3 %), greatest in those with the highest HbA1c at baseline and who most frequently used the sensors (Fig. 13.3). Exposure to hypoglycemia was also reduced during the monitoring. A Cochrane review on CGM was published in 2012 [32] including 22 RC trials meeting the inclusion criteria: the authors confirmed that the largest improvements in HbA1c were seen in patients with poorly controlled diabetes. The authors found that HbA1c levels 6 months after baseline were lower for CGM users compared to SMBG users (−0.2 %) but even lower than for patients starting using an insulin pump and CGM at the same time. No significant increasing of severe hypoglycemia or ketoacidosis for CGM users. Higher compliance of wearing the CGM device can help in improving metabolic control. A further meta-analysis [33] established that concerning glycemic control RT-CGM is superior to SMBG and that SAP is superior to MDI associated with SMBG without increasing the risk for hypoglycemia.
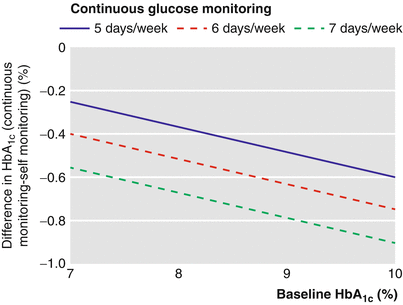
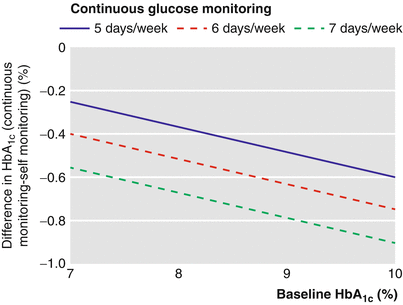
Fig. 13.3
Model estimated difference in HbA1c using CGM compared with SMBG to baseline HbA1c percentage and sensor usage in an example 40-year-old participant with T1DM (From Ref. [31])
As far as the use of SAP is concerned, several studies demonstrated the superior effectiveness of the integrated systems compared to insulin pump or MDI in achieving metabolic control goals.
In 2010, the STAR 3 study [34] affirmed that in poorly controlled adults and children with T1DM, SAP therapy resulted in significant improvement in HbA1c levels, as compared with injection therapy. The SWITCH study demonstrated that SAP therapy was associated with a reduction of HbA1c levels (the mean difference in HbA1c was −0.43 % in favor of the SAP arm vs. insulin pump) and time spent in hypoglycemia compared to subjects using CSII alone. The authors concluded that allowing more frequent insulin, self-adjustments could have contributed to these results [35].
The onset study [36] aimed to evaluate the management of children with T1DM using a SAP therapy from diagnosis and found that after 12 months SAP was associated with better metabolic control (HbA1c −0.5 % in regular-sensor users vs. poor-sensor users), less decline in fasting C-peptide particularly in older children, and less glycemic variability.
A Cochrane review [32] indicated a benefit of CGM for patients starting on SAP therapy compared to patients using multiple daily injections and standard monitoring blood glucose; after 6 months, there was a significant larger reduction in HbA1c level for RT-CGM users starting insulin pump therapy compared to patients using MDI and SMBG in change in HbA1c level −0.7 %.
The meta-analysis led in 2012 reported that sensor-augmented insulin pump use resulted in a statistically and clinically significant greater reduction in HbA1c levels than with MDI or SMBG in persons with type 1 diabetes mellitus [33]. Norgaard et al. [37] established the effectiveness and the feasibility of SAP in a real-life setting: the average sensor use for 12 months was 30 % (wide range, 0–94 %) and sensor use decreased with time. This study confirmed its effectiveness, especially in subjects with poor control (HbA1c >7 %), older age, and more frequent sensor use.
13.5 Evidences on Accuracy
No official recommendations currently exist for selecting a particular sensor system.
Some studies were recently published focused on the effectiveness and accuracy of different CGM systems.
Damiano et al. [38] compared three GCM devices: Abbott Navigator, Dexcom G4 platinum, and Medtronic Enlite. The comprehensive head-to-head-to-head comparison revealed marked differences in both accuracy and precision. The Navigator and G4 Platinum (G4) had the best overall accuracy than Enlite, with an aggregate MARD of 12.3 ± 12.1 %, 10.8 ± 9.9 %, and 17.9 ± 15.8 %, respectively (Fig. 13.3). Matuleviciene et al. [39] aimed to compare two CGM systems (Dexcom G4 to Enlite sensor) in 38 adult subjects with T1DM wearing the sensor for 4 up to 6 days. They found that Dexcom was associated with greater overall accuracy than Enlite sensor, with a MARD index of 13.9 % and 17.8 %, respectively. Moreover, patients referred a more positive experience with Dexcom G4. Preliminary data indicate that the new Dexcom G5 is the first sensor with a MARD below 10 %.
Another study developed in a population with T1DM and type 2 diabetes reported a MARD of 13.8 % for Enlite sensor [40].
In the hypoglycemic range, maintaining a good accuracy of CGM is challenging, and the performance of all CGM systems is markedly worse when compared with BG results [41]. Other recent studies on hypoglycemia reported that Dexcom G4 Platinum is one of the most accurate CGMs even for hypoglycemic values [42].
The Abbott FreeStyle Libre is a recent glucose sensor sold directly online to the patients, with a longer duration (14 days) and a cheaper price (approximately half) compared to the other sensors. It does not require calibration, and it is not equipped with a transmitter: glucose data are stored up to 8 h and are visualized via a 1-s scan by the specific reader. The company reports a MARD of 13.4 and 83.5 % of the values within the A area of the consensus grid, with nonsignificant improvement (12.7 %) after calibration.
13.6 Psychosocial Aspects
Young subjects with T1DM seldom wear a CGM system as much as recommended. The positive and/or negative psychosocial effects of CGM use could influence patients’ frequency and persistence of using the device [43].
The positive psychological and behavioral effects could be represented by reinforcement of diabetes education, decreased anxiety about asymptomatic hypoglycemia, increased flexibility in daily living, and enhanced motivation for improved glycemic control. The negative aspects could be the increasing overall burden of diabetes management, generating unrealistic expectations about its benefits, managing an overwhelming amount of glucose data, and wearing the sensor in particular social settings such as at school [44]. The most common perceived benefits of RT-CGMS are prevention of hypoglycemia and decrease in hypoglycemia-related anxiety [45]. The results of this pilot study suggest that benefits of CGM extend beyond reductions in HbA1c to reductions in fear of hypoglycemia and improvements in quality of life [46]. However, the psychological impact of CGM still remains unclear, and some authors reported different psychological impacts of CGM use on youth, their parents, and adults [47]. The SWITCH showed that adding CGM improved short-term treatment satisfaction in adults and that the introduction of a new technology does not have a significant negative impact on children’s quality of life [48].
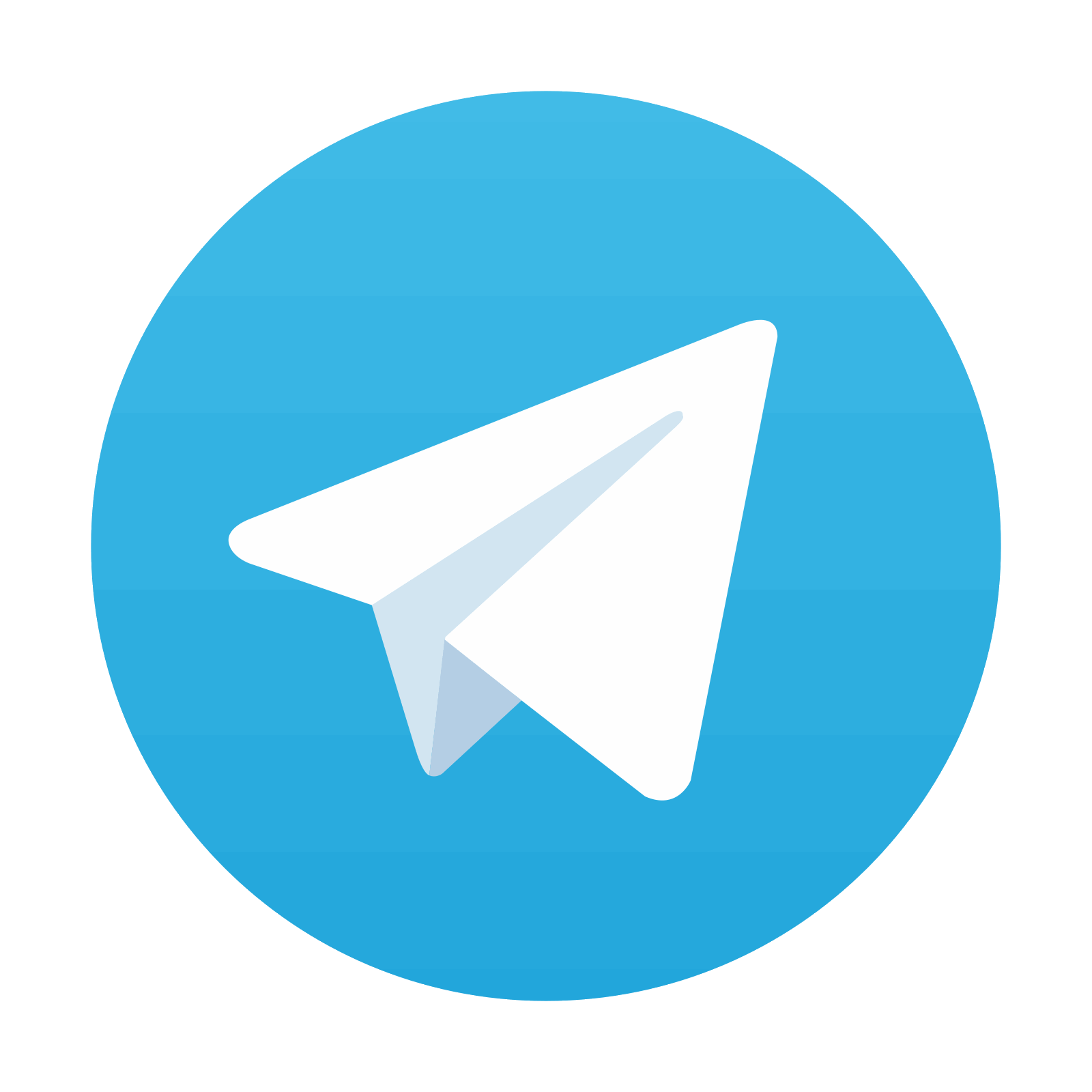
Stay updated, free articles. Join our Telegram channel

Full access? Get Clinical Tree
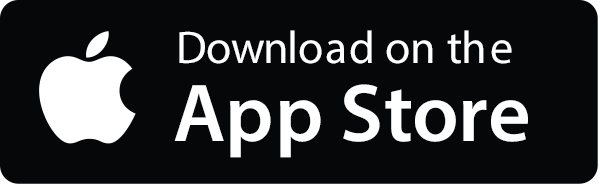
