18.1
Introduction
18.1.1
Genetic diseases
The concept of genetic risk for diseases has substantially evolved in the past decades from traits and diseases that run in families, to the current concept of personalized risk profiles based on DNA analysis of individuals. This is due to new insights into the genetic nature of disease driven by the availability of methodology to identify and characterize genetic factors predisposing to disease. The importance of knowing such genetic risk factors lies in the possibility of making the following advances to (1) determine a personalized DNA “risk profile” at a very early stage, through molecular genetic techniques, even far before the clinical onset of disease; (2) tailor therapeutic intervention strategies on the basis of knowledge about the gene/protein variants involved in drug metabolism and action; and (3) provide early advice on lifestyle changes based on personalized DNA profiles.
An important distinction in this context is that the one between so-called monogenic disease (caused by a mutation in one gene, and apparent in one or more families) and complex genetic disease (caused collectively by common variants in hundreds to thousands of genes interacting with environmental factors, and apparent in an enriched form in the majority of patients at clinical presentation). Much of the advances in the field of genetics are technology-driven and somewhat alien in the field of bone disease, and so we provide a brief glossary of some genetic terms in Table 18.1 .
Allele | One of several alternative forms of a DNA sequence at a specific chromosomal location (locus). At each autosomal chromosomal locus in a cell, two alleles are present—one inherited from the mother and the other from the father |
DNA marker | A polymorphic DNA segment at a known chromosomal location |
Genetic map | The most likely order of DNA segments on the chromosome based on the analysis of cosegregation of DNA markers in pedigrees |
Genome search | The analysis of several hundreds of DNA markers (usually microsatellites) which are more or less evenly spread over all of the chromosomes, in collections of related individuals to look for linkage with a phenotype |
Genotype | The combination of two alleles at a locus in an individual |
GWAS | Genome-wide association study |
Haplotype | A series of alleles found at linked loci on a single chromosome (phase) |
IBD | Identical by descent. The situation where alleles in two or more individuals are identical because of common ancestry |
IBS | Identical by state. The situation where alleles in two or more individuals are identical due to coincidence or to common ancestry |
kbp | kilobase pairs (1×10 3 bp) |
Linkage | The tendency of DNA sequences to be inherited together as a consequence of their close proximity on a chromosome |
LD | Linkage disequilibrium: nonrandom association of alleles at linked loci |
Locus | A unique chromosomal location defining the position of a particular DNA sequence |
LOD score | Logarithm of the odds; measure of statistical likelihood that a genetic marker is associated through physical linkage with a gene causing or contributing to a particular phenotype |
Mbp | Megabase pairs (1.10 6 bp) |
Microsatellite | A locus consisting of tandemly repetitive sequence units the size of which is (arbitrarily) defined as 1–5 bp |
Minisatellite | A locus consisting of tandemly repetitive sequence units the size of which is (arbitrarily) defined as 6 bp or more |
Mutation | An alteration in the DNA sequence |
NGS | Next-generation sequencing |
Physical map | The order of DNA segments on a chromosome as determined by molecular analysis of (large) DNA segments |
Polymorphism | The existence of two or more alleles at a frequency of at least 1% in the population |
QTLs | Quantitative trait loci; a gene that influences quantitative variation in a trait |
RFLP | Restriction fragment length polymorphism |
SNP | Single-nucleotide polymorphism |
Synteny | The location of loci on the same individual chromosome |
UTR | Untranslated region (as in 3′-UTR of a mRNA) |
VNTR | Variable number of tandem repeats; a polymorphic micro- or minisatellite |
WES | Whole-exome sequencing |
18.1.2
Monogenic diseases
Genetic diseases are classically defined as single Mendelian traits usually with an early onset of the disease and relatively fast progression and showing clear Mendelian inheritance patterns in families. Since it was recognized around 1980 that the genetic inheritance patterns of these monogenic diseases could be followed using naturally occurring DNA sequence variations , early molecular genetic technology soon allowed the isolation of the genes responsible for some of these diseases such as Duchenne muscular dystrophy, cystic fibrosis, Huntington’s disease, and several others later on. By now, the chromosomal position and causal mutation are known for more than 5500 disease genes of the estimated 7200 monogenic diseases [see Online Mendelian Inheritance in Man (OMIM); ].
Also, in the area of bone metabolism the accretion of knowledge on the molecular genetic nature of disease had led to important discoveries. Among the cloned disease genes responsible for Mendelian bone disorders are the genes encoding collagen type Iα1 (located on chromosome 17q22) and collagen type Iα2 (7q22.1), responsible for most forms of what is the best known and characterized genetic bone disease: osteogenesis imperfecta (OI) . This inherited brittle-bone disorder predisposes a patient to easy fracturing of bones, even with little trauma, and to skeletal deformity. The condition involves either qualitative or quantitative alterations in type I collagen protein which is the result of a variety of possible small point mutations or small deletions/duplications within the genes that encode the chains of the collagen type I protein and several important enzymes in the pathway. While bone fragility is common to all forms of OI, the clinical phenotypic presentation is remarkably variable, ranging from lethal perinatal forms to only a mild increase in fracture frequency in late-onset forms of the disease. Underlying this range of variation is the so-called locus and allelic heterogeneity, that is, the disease phenotype varies according to which gene (e.g., collagen type Iα1, collagen type Iα2, or other genes) is mutated and according to the type and location of the pathological mutation in that gene.
Apart from these classic genetic bone diseases, a range of other monogenic bone disorders have been elucidated including many osteopetrotic syndromes . These have led to important new insights in bone biology and sometimes also to identification of novel signaling pathways in bone metabolism. Examples include the discovery of the SOST gene and the LRP5 genes as causes of such monogenetic disorders and subsequent insights into the role of Wnt signaling in bone biology . The introduction of next-generation sequencing (NGS) has increased the pace of discovery of such genes. A cost-effective variant of this, so-called whole-exome sequencing (WES; i.e., NGS of all 180,000 exons in a human genome), is applied to search for and identify mutations in pedigrees with Mendelian mutations. NGS has also been applied to bone diseases and has identified several new bone genes and pathways . Because NGS is so specific and requires less family members, it is expected that most of the remaining ~2000 unresolved Mendelian diseases, including several bone diseases, will be solved in the coming 5–10 years by applying NGS.
18.1.3
Complex traits and diseases
The characterization of the molecular genetic basis of OI and other, by current standards, relatively simple genetic disorders, is still changing our concept of bone biology and bone disease. Analysis of such diseases not only illustrates the vast and devastating effects single mutations can have but also generates novel technological tools accelerating the process of gene discovery and mutation detection. Together, this provides the basis to tackle the more challenging problems of the common multifactorial diseases, such as osteoporosis among the musculoskeletal diseases of the locomotor system. Many of the most important medical conditions in the Western world are usually not characterized by simple Mendelian inheritance patterns, with early-onset and straightforward diagnostic criteria. They are in fact multifactorial in origin and presenting much more frequently in the population. See, for example, well-known Mendelian diseases such as cystic fibrosis with an estimated population incidence of 1 in 3000 individuals of European descent or the combined incidence of all forms of OI, which is about 1 in 10,000. This is in sharp contrast with common diseases such as diabetes, hypertension, asthma, manic depression, and osteoporosis occurring in 1 in 2 to 1 in 20 (5%–50%) of the elderly population. These are the diseases that doctors see and treat on a daily basis. In view of the increase in the maximum life expectancy of men and women in our society the prevalence of common diseases will increase even further in their frequency.
In view of the basic importance of DNA and its variation in the cause of disease the search for the responsible genes of these complex disorders is now a priority in medical research. Unlike the relatively straightforward genetics of the monogenic disorders, common diseases have a multifactorial nature (genetic and environmental conditions play a role and they interact), are multigenic (multiple genes are involved, usually hundreds if not thousands of genes), and usually have a late onset with variable clinical manifestations. It is therefore not surprising that these diseases are referred to as “complex diseases.” However, due to the successful application of molecular genetic techniques to monogenic diseases, unraveling of the genetic etiology of complex diseases seems a feasible mission . In the field of bone metabolism the main target common complex disease is of course osteoporosis, but other bone diseases such as Paget’s disease are under similar scrutiny . For many, if not all, of these complex diseases, several intermediate risk factors have been recognized that are also viewed as being caused by multiple gene variants that interact with environmental factors. Examples include bone mineral density (BMD) for osteoporosis, glucose levels for diabetes, blood pressure for cardiovascular disease, among many others. These intermediate “complex traits” (or endophenotypes) usually follow a normal distribution in the human population (and in animal models for that matter) and are also referred to as Quantitative Traits . Genetically mapping the responsible genes to certain loci in the genome for these traits is then referred to as quantitative trait locus (QTL) mapping.
The genetic dissection of complex traits and diseases follows similar analytical strategies for many of the common diseases, including osteoporosis. First, evidence is sought to demonstrate and estimate the heritability of the trait (or one or more of its composite features) and the influence of environmental factors, initially derived from twins and family-based studies, but more recently and reliably from large genome-wide association studies (GWASs). Then, epidemiological studies are needed to quantify the variability of a trait and identify potentially modifying environmental factors. With this knowledge at hand, genetic epidemiological studies in human populations are carried out applying molecular genetic tools that can successfully identify putative candidate genetic variants. Finally, candidate genetic studies will establish the contribution of particular genetic variants in explaining the variation of the trait, also in relation to gene–environment interactions, and, last but not least, investigate the underlying molecular mechanisms. While the first steps of this process are by now almost routine (relatively speaking of course!), the last step of explaining molecular mechanism has been slow. This is not so surprising given the abundance of genetic variants found (comprising thousands of variants for >1800 diseases and traits), the relatively small effect size observed, and the conservative nature of molecular and cell biologists. In these last stages also the clinical implications are being considered for the genetic variants that have been identified. This can involve the discovery of a novel gene or pathway upon which the development of a therapeutic intervention can be based. On the other hand, given a certain number of genetic risk variants identified for a disease, risk modeling can be considered to be applied in clinical settings, while in addition pharmacogenetic studies might be useful for patient stratification purposes in treatment regimens as well as in drug development pipelines. For all complex diseases these scenarios are now being followed, given the success of GWAS in particular in identifying causative genetic variants, and osteoporosis is no exception.
18.1.4
Osteoporosis
Osteoporosis, as discussed in many chapters in this volume, is defined by decreased BMD and degenerative microarchitectural changes of bone tissue, and consequently an increased fracture risk. Naturally, in the absence of molecular insights into the cause of the disease, definitions of it remain vague and descriptive. The main emphasis in this definition is on aspects of bone, while the clinically relevant endpoint in osteoporosis is fracture. Yet, fracture risk is only in part determined by bone characteristics with also other anthropometric and physiological parameters contributing to fracture risk such as cognitive function, body size, and muscle strength (see Fig. 18.1 and other sections in this chapter). Thus the genetic analysis of osteoporosis will include the genetics of bone characteristics, such as BMD, but also needs to address the genetics of cognition, muscle strength, etc. and other factors related to risk of falling and risk of fracture.
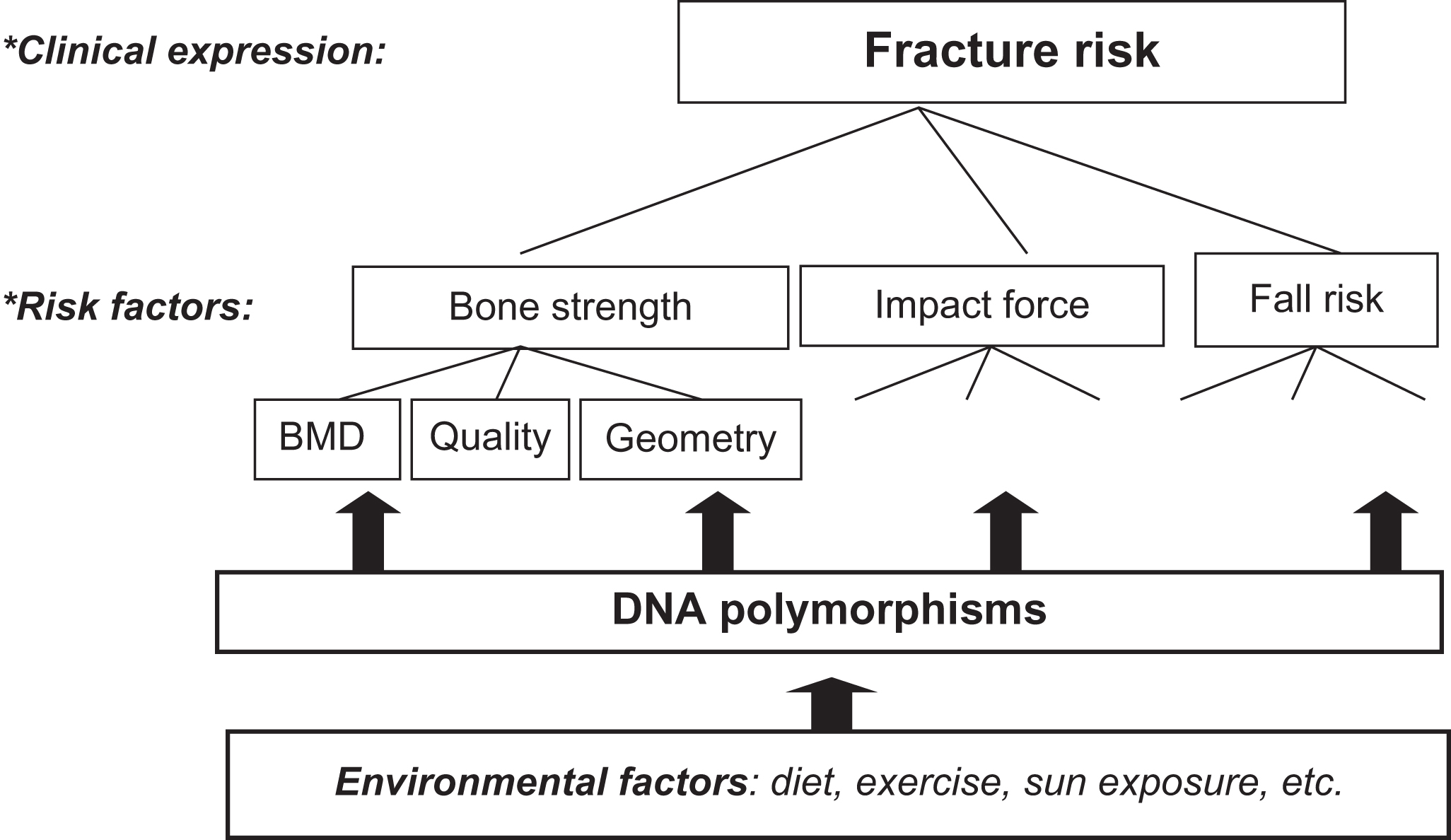
Of particular interest is BMD, which can be considered to be a quantitative trait. That is, in a population BMD can assume a continuous variety of values, while BMD values follow a normal distribution. Although “high” BMD as well as “low” BMD can be defined using particular cutoffs based on, for example, the T -score or the Z -score, such distinctions introduce considerable subjectivity as to what is a sensible threshold to distinguish “cases” from “controls” in scientific research from a more biological perspective. This is in contrast with the more straightforward dichotomous traits such as having a fracture “yes or no.” Yet, these events—while clinically more relevant—tend to become a melting pot of (sub)phenotypes and debates on the best phenotype definitions which capture the disease. This is well-illustrated by the discussion on whether hip fractures, wrist fractures, and vertebral fractures can all be considered as “osteoporotic” fractures. Therefore choosing an appropriate phenotype of interest in genetic studies of osteoporosis is still far from straightforward and open to debate. In this respect the situation is not very different from the field of, for example, osteoarthritis research where similar debates exist on whether the radiographic Kellgren score and/or the surgical joint replacement procedure are appropriate endpoints of the disease to be studied in scientific research, including for genetic studies.
18.1.4.1
Genetics of bone phenotypes
Certain aspects of osteoporosis have been documented to have strong genetic influences. This can be derived, for example, from genetic epidemiological analyses, which showed that, in women, a maternal family history of fracture is positively related to fracture risk . Most evidence, however, has come from twin studies that initially focused on BMD . Monozygous (MZ) and dizygous (DZ) twins share 100% and 50% of their genome, respectively. So, if a trait is strongly influenced by genetic factors, one expects the variance between the two members of an MZ twin pair to be smaller than between members of a DZ twin pair. In simple terms, this difference in variance between the two twin types can be expressed as a “heritability score” indicating whether all phenotypic variance observed is explained for 0%–100% by genetic factors rather than environmental factors. An overview of heritability estimates for osteoporosis-related characteristics is listed in Table 18.2 . While most phenotypes are listed as highly heritable, twin studies can overestimate the heritability of phenotypes. This is because twins tend to share more than just their genes, such as a similar womb environment in the fetal stage, and later on a similar environment and living conditions when growing up. Heritability estimates are not a universal figure, because they can also vary from one study to the next due to the differences between the populations in which they are measured, for environmental factors and genetic context.
Phenotype | Heritability ( h 2 ) (%) |
---|---|
BMD | 50–80 |
Bone turnover/biochemistry | 40–70 |
Bone geometry | 70–85 |
Quantitative ultrasound | 80 |
Height | 80–90 |
Age at menopause | 60 |
Body mass index | 60–70 |
Fracture risk | |
Hip fracture | 3–68 |
Wrist fracture | 54 |
For BMD in such studies the heritability has been estimated to be high: from 50% up to 80%. Thus although twin studies can overestimate the heritability, a considerable part of the variance in BMD values might be explained by genetic factors, while other parts could be due to environmental factors and interactions between them. Nevertheless, although the precise value of this figure is subject to discussion, it can be concluded that BMD has a strong genetic basis, while this holds also true, for example, for bone loss over time with an estimated heritability of ~50% (measured as a drop in BMD values over time) . More recently, studies assessing the heritability of bone loss have been summarized and the prospects and challenges for identifying underlying genes and variants traced as an intricate enterprise .
In molecular terms the existence of considerable heritability for BMD as a phenotype means that there are so-called bone density genes, whose variants will play a role explaining variation in BMD levels across individuals. Because they influence quantitative variation, these genes are referred to as QTLs. From what we know about how BMD changes over time, these differences can become apparent in different ways, theoretically, from variants that explain differences in peak BMD or differences in the rates of bone loss at an advanced age. Nevertheless, most of the variation in BMD along the lifetime comes from differences in peak bone mass acquisition, rather than from bone loss later in life . In addition, the expression of the genetic influences on BMD can be different, for example, during periods of high bone turnover at puberty and menopause when other factors will be interacting with the genetic predisposition. While it was expected that the genes involved in these different processes during different time periods would also be different to some extent, only a few genetic associations have been identified specific to distinct life periods (age strata), with most having an effect already seen on peak bone mass acquisition .
While these notions have resulted in much attention being paid to the genetics of BMD in the field of osteoporosis, it is likely that this—apparently focused attention—is also due simply to the wide-spread availability of Dual-energy X-ray absorptiometry (DXA) devices to measure BMD for clinical reasons and, thus, many (large) databases with BMD data exist. Similarly, given the rise in (national) biobanks where bone parameters are being measured mostly using more economic measurements such as quantitative ultrasound (QUS) properties of heel bone as measured by relatively cheap machines allowing large-scale data collection, such as in UK Biobank .
Yet, also these other parameters of bone have been found to be heritable such as QUS , femoral neck geometry , and also biochemical indices of bone turnover such as vitamin D and parathyroid metabolism . In addition, other phenotypes, which are (partially) a result of bone phenotypes, such as height being partially driven by bone size characteristics, show strong heritability .
Last but not least, other physiological characteristics with a strong influence on bone properties (as measured by BMD or other characteristics) were found to be heritable such as body mass index , muscle strength , and age at menarche and age at menopause . Since these phenotypes in themselves are complex traits and thus determined by multiple genes in interaction with environmental factors, this highlights the complexity of identifying “osteoporosis genes” and also calls for a more holistic approach, nowadays termed “systems biology.”
The heritability estimates of osteoporosis leave room for a considerable influence of environmental factors that can modify the effect of genetic predisposition. One can think of interactions of genetic factors with dietary habits, exercise patterns, lifestyle in general, and exposure to sunlight. Environmental factors tend to change during the different periods of life which can result in different “expression levels” of the genetic susceptibility. Aging is associated with a general functional decline resulting in, for example, less exercise, less time spent outdoors, and changes in diet. This can result in particular genetic susceptibilities being revealed only later on in life after a period when they went unnoticed due to sufficient exposure to one or more environmental factors, which overcome the potential deficiencies due to genetic predisposition. Alternatively, genetic susceptibilities can only be observed early on in life, or just before the aging process starts (such as is seen for the hip fracture genetic susceptibility). Thus genetic susceptibility is not “a given” to be measured but can become—more or less—apparent in certain situations of stress and at certain moments in a lifetime. Alternatively, this also illustrates how genetic predisposition can perhaps be overcome by changing such detrimental lifestyle factors. This is a major driving force for the current enthusiasm for using one’s genetic profile to provide personalized advice (precision medicine; see later on in this chapter).
18.1.4.2
Genetics of fracture risk
Fracture is the deleterious and most relevant clinical outcome of osteoporosis. Heritability estimates of fracture risk have been—understandably—more limited due to the scarcity of good studies allowing precise estimates. Accumulating large collections of related subjects with accurate standardized fracture data is notoriously difficult in view of the advanced age at which fractures occur. While documenting a fracture event is possible in longitudinal studies, excluding a fracture event in those who report no fracture is more difficult because they could still suffer a fracture later in life. One option to overcome this potential problem might be to take controls that are (much) older. In the case of hip fracture patients (with a mean age of 80 years), this would require control subjects of 90–100 years. It is questionable whether such—very healthy—survivors are proper controls for fracture cases and thus aspects of (genetics of) longevity also have to be considered. For these reasons, most of the genes shown to be associated with fracture risk have been discovered by testing BMD loci identified through GWAS for association with fracture.
Despite the intrinsic difficulties for the discovery of genetic determinants of fracture risk, there is sufficient evidence to pursue their identification. Family history was found to be a strong risk factor for future fracture risk indicating a strong genetic effect on fracture risk. This notion was further strengthened by several twin studies. Andrew et al. studied 6570 white healthy UK female volunteer twins between 18 and 80 years of age and identified and validated 220 nontraumatic wrist fracture cases. They estimated a heritability of 54% for the genetic contribution to liability of wrist fracture in these women. Interestingly, while BMD was also highly heritable, the statistical models showed a very little overlap of shared genes between the two traits in this study. Michaëlsson et al. studied a very large sample of 33,432 Swedish twins (including 6021 twins with any fracture, 3599 with an osteoporotic fracture, and 1055 with a hip fracture after the age of 50 years) and concluded that heritability of hip fracture overall was 48%, but interestingly this was 68% in twins younger than 69 years and decreased to 3% in elderly twins 79 years and older. Indeed, another study from Finland of elderly twins showed very low heritability for risk of fracture . Altogether, this suggests that although fracture risk is genetically determined, at older age other environmental factors are more important in explaining variance in fracture risk.
While it might be difficult to demonstrate that fracture risk is heritable, one can also argue that it follows from simple logical reasoning that aspects of osteoporosis, including fracture risk, must have a genetic influence. We know that DNA is the blueprint of life, that the DNA sequence of an individual (the genotype) is different between individuals, and that phenotypes differ between individuals. Thus the difficulties in demonstrating heritability of fracture risk are probably also due to limitations of our methods and approaches of measuring it.
It is important to realize that (low) BMD is but one of many risk factors for osteoporotic fracture, the clinically most relevant endpoint of the disease (see Fig. 18.1 ). Interestingly, the increased fracture risk associated with a positive family history of fracture persists after adjustment for BMD . This indicates that also the genetic susceptibility to fracture is mediated by additional factors other than only those predisposing to low BMD. One example includes hip axis length (HAL) as a measure of femoral geometry. Twin studies have suggested 80% of the variation in HAL to be explained by genetic factors, independent of BMD , while the same was suggested for ultrasound measurements of bone . Thus bone density and bone architecture will be influenced by shared, but also by separate, genetic factors. Composing a portfolio of genetic risk factors for “osteoporosis,” including bone fracture, will therefore necessitate determining which subphenotype, that is, which particular characteristic of osteoporosis, has the strongest influence and how to weigh the set of interesting factors within the complete set of osteoporosis genetic risk factors. Nevertheless, all loci identified so far that are associated with fracture risk, even by GWAS, constitute known BMD loci . Thus the genetics of any type of osteoporotic fracture in the general population is mediated through the genetic influence on low BMD, a necessary but not sufficient cause of fracture.
To date two GWASs have been performed using vertebral fractures as an endpoint. In the first metaanalysis, one locus on chromosome 16q24 (rs11645938) was associated with the risk of radiographic vertebral fractures, but this failed to replicate across 5720 cases and 21,791 controls . A recent metaanalysis (thus including replication) reported a locus mapping on chromosome 2q13 to be significantly associated with clinical vertebral fractures . The first GWAS on nonvertebral osteoporotic fractures ( N =700) was performed in elderly Chinese individuals and identified one fracture-associated locus within the ALDH7A1 gene . However, this gene failed to replicate in any of the larger European metaanalyses. Trajanoska et al. conducted the largest GWAS on osteoporotic fractures to date comprising 37,857 cases and 227,116 noncases with replication in up to 300,000 individuals (147,200 cases). Altogether, the effort identified 15 fracture loci with modest effects. Interestingly, all identified loci were known BMD loci. Overall, the effect of these single-nucleotide polymorphisms (SNPs) on fracture was smaller than the effect on BMD. Thus the genetics of any type of fractures in the general population is likely mediated through the genetic influence on BMD. This is well characterized by the genetic correlations of fracture risk with BMD. Further, a genetic analysis technique called Mendelian randomization (MR) (reviewed elsewhere ) ( Box 18.1 ) was used to determine if certain clinical risk factors for fracture, for which by now several genetic factors have been determined by GWAS similar as for BMD, for example, are causally related to fracture risk. Among 15 tested clinical factors in this way by MR (including vitamin D levels and milk calcium intake), only BMD was observed to have a major causal effect on fracture, next to a more minor but causal effect of age at menopause . The actual loci and genes identified by this effort as associated with fracture risk are presented later.
Glossary of Mendelian randomisation
Mendelian randomization (MR) is a statistical technique that leverages genetic information in order to provide evidence for a causal relationship between modifiable risk factors and diseases.
Natural experiment
The MR approach uses genetic variants as instrumental variables for the risk factor of interest. Due to the random allocation of alleles during gamete formation the genetic variants are less likely to be associated with any confounders. Importantly, they cannot be affected by reverse causation. Therefore, the MR approach can provide more robust evidence of causal associations compared to the traditional observational studies.
Key assumptions
- 1.
The genetic variants must be associated with the risk factor under investigation.
- 2.
The genetic variants are not associated with any confounder that can bias the association between the risk factor and the outcome.
- 3.
The genetic variants affect the disease under investigation only through the risk factor of interest.
Study design
One sample MR – when both the risk factor and outcome are measured in the study population.
Two sample MR – when the risk factor and outcome are measured in two different study populations. This methodology has been facilitated by the advent of large-scale GWAS that have led to substantial increases in the statistical power of the MR approach.
Limitations
- •
Heterogeneity – Presence of differences in effects estimates between the genetic variants used as instrumental variables for the risk factor under investigation that cannot be explained by sampling variation alone.
- •
Population stratification – Presence of differences in allele frequencies and/or disease prevalence rates between subgroups in the total study population which can confound the association between the risk factor and the disease of interest.
- •
Pleiotropy – When one genetic variant is associated with more than one trait which is a serious violation of the third MR assumption.
- •
Canalization – When the individuals’ response to genetic and environmental influences is attenuated or absent as a result of the presence of so-called “buffering mechanisms” that act against the expected genetic and environmental effects.
- •
Weak instruments – When the genetic variants explain a small proportion of the variation of the risk factors, MR can provide biased causal estimates due to very low statistical power.
We note that so far the genetic factors identified for osteoporosis and fracture are derived from studies using individuals of European background. This renders the results potentially relevant and applicable for such Caucasian populations, but not necessarily for other ethnic groups. From several genome sequencing programs, we know that such ethnic groups will differ in their general genetic background due to number of variants, type, and frequency of genetic variations in the genome and, most importantly, the distinct correlation [linkage disequilibrium (LD)] across variants. As a result, differences in effect size of the particular genetic factors involved in, for example, BMD and fracture in such ethnic groups will also differ to a certain extent from those in Europeans. This will not only offer additional opportunities to discover new biology but also highlights the need for studying other ethnic groups of non-European background for translatability of results from such studies in Europeans. Therefore similar genetic studies with similar power, including GWAS of osteoporosis and fracture, in ethnic groups other than Caucasians, such as Asians and Africans, are therefore eagerly awaited.
18.1.4.3
Genetic effects: large versus small and common versus rare
From the analysis of the tens of thousands of successfully identified genetic risk factors for hundreds of complex traits, it is by now clear that for common/complex disorders in general, the risks associated with each individual genetic variant are generally modest in terms of effect size. These small effect sizes also explain why it has been difficult to identify such risks convincingly, in spite of these genetic variants being so common. Common in this respect means allele frequencies of a genetic risk factor of 5%–50% and modest effect sizes with mean odds ratios (ORs) of 1.01–1.1. Statistical power calculations show that indeed very large study populations of 10,000s–100,000s subjects of case–control collections and/or population-based cohorts need to be studied in order to demonstrate convincingly such small effects by association analysis. Such large study populations are now available and consortia of collaborating investigators have been assembled to address these challenges in a robust manner. This change in scientific culture, instigated by requirements for proper statistics and scientific rigor following revolutionary technological developments, has substantially altered the way we now work collectively on a global scale on the problem of osteoporosis genetics. More or less all groups working on genetics of osteoporosis started to collaborate in the GENOMOS (GENetic Markers for OSteoporosis) consortium since 2003 (see Section 18.2.5.2 ), and since 2008, this has expanded into the GEFOS (GEnetic Factors of OSteoporosis) consortium (see Section 18.3.3 ).
While the risk of disease for a human subject is indeed small for such individual genetic risk variants, because there are so many of these common variants in the human genome (we currently estimate more than 100 million such variants), the combined effect—or genetic load—of these risk variants can be substantial both for the individual as well as for the population. One can speculate that evolution has allowed these common variants associated with age-related disease to float around in the human population because they do not compromise reproductive success (or might even enhance it) and only start to affect fitness of the individual carrying such variants late in life, far after the reproductive period. On the other end of the spectrum, more rare variants will be selected out in evolution because they do affect reproductive success and/or will be private to individuals as newly arisen mutations; but then rare variants that have emerged more recently might also contain some that have escaped selective pressures (i.e., lacking sufficient generations for their frequencies to be shaped by evolutionary pressures).
Overall, the current thought about underlying genetic risk variants of complex diseases such as osteoporosis is that for each complex disease, there will be hundreds to thousands of common variants conferring risk, but any given individual will also carry several genetic variants that are very rare in the population and might have bigger associated genetic risks. As we will see later, we have been successful in identifying hundreds of these more common effects with the smaller effect sizes through GWAS. In addition, by applying NGS techniques in large study populations, several of the much rarer sequence variants have been identified that confer somewhat larger effects. Combined, these genetic factors now explain >35% of the variation in BMD that makes them worthwhile to start using them in a clinical context as in a genetic risk profile.
In the following sections, we will discuss different approaches and some more technical issues, followed by a review of some data obtained thus far in the search for osteoporosis genes.
18.2
Finding risk gene variants for complex traits
Osteoporosis is a multifactorial complex disease where genes interact with environmental factors and so both should be studied for their contribution to variation in disease risk. Yet, it is not surprising that most attention has gone to the study of genes rather than of environmental factors considering that the Human Genome Project has identified nearly all human genes, delineated the complete human genome sequence leading to inventories of millions of DNA sequence variations, and yielded enormous progress in DNA analysis technology, this is referred to as the “genocentric” approach. This approach also has much to do with the difficulty in accurately assessing (the changing) environmental exposure over a lifetime, as opposed to the extremely high detail with which we can determine an individual’s DNA sequence and in a very short time. Once we know which gene variants are involved in a certain complex disease, we will understand which biological pathways are important in explaining interindividual variance. Based on our accumulated biological knowledge about these pathways, we can then better focus on particular environmental factors and gene–environment interactions.
The first step in the molecular dissection of the genetic factors in osteoporosis involves determining the chromosomal location (mapping) of the DNA variants involved and the identification and characterization of the set of genes, variants of which are responsible for contributing to the genetic susceptibility for the different aspects (or subphenotypes) of osteoporosis. Finding the responsible gene for any given monogenic disorders has now become almost a routine exercise for specialized laboratories, especially given the wide-spread application of WES. However, the complex character of osteoporosis (and all other complex traits) makes it quite resistant to the methods of analysis, which in the past decades have worked so well for monogenic diseases. Therefore different approaches have been applied (e.g., see Refs. ), also based on learned experiences with the different approaches over the past years.
18.2.1
DNA sequence variation
All of the analytical approaches to find “osteoporosis genes” are based on the observation that the genomic (and mitochondrial) DNA sequence between two individuals will differ at certain positions. Yet, finding DNA sequence variations between two individuals is not very difficult. This was demonstrated early on already in focused resequencing efforts of certain genes, for example, for the human lipoprotein lipase (LPL) gene , the angiotensin-converting enzyme (ACE) gene , and the vitamin D receptor gene . In addition, coding and regulatory regions of large numbers of genes have been analyzed to find DNA sequence variants. There are different types of DNA sequence variation, but the most prevalent among these are the SNPs . Resequencing efforts across the human genome have revealed a large number of different types of DNA polymorphisms, which are listed in Table 18.3 . All these data are deposited in large databases such as the human SNP database (dbSNP) where by 2019 over 150 million SNPs have been entered . From these approaches, it is estimated that there is on average 1 bp variant within every 30 bp in the human population, with variant (allele) frequencies of >1%. This means that any two random individuals will differ at 1 out of every 100 bp and that two such subjects will differ at about 30 million loci, or 1% of the human genome. In other words, there is an abundant DNA sequence variation between individuals. We distinguish “mutations” from “polymorphisms” purely on the basis of frequency: polymorphisms occur in at least 1% of the population, mutations in less. Yet, this distinction is increasingly shifting toward more rare DNA sequence variations, with population frequencies of <0.01% being referred to as mutations. Therefore the more generic term “DNA sequence variations” or “variations” is more widely used. In addition, while polymorphisms are intrinsic variability components of human diversity, mutations usually carry also the context of disease implications and connote also deleterious consequence on the gene product. From the perspective of the allele(s), these variations can provide information on an individual’s increased or decreased risk of developing a certain condition. So, the clue in genetic analysis of complex traits is therefore to find the DNA variants that matter, in terms of modifying (increasing or decreasing) risk for disease. To find these variations there are—roughly speaking—two approaches: the top-down and the bottom-up ( Fig. 18.2 ).
Type | Number in genome | Genome proportion (% of bp) |
---|---|---|
SNPs | 50–400 million | ~5 |
VNTRs a | 50,000 | ~2 |
CNVs b | 1500 | ~12 |
a Microsatellites, minisatellites, others.
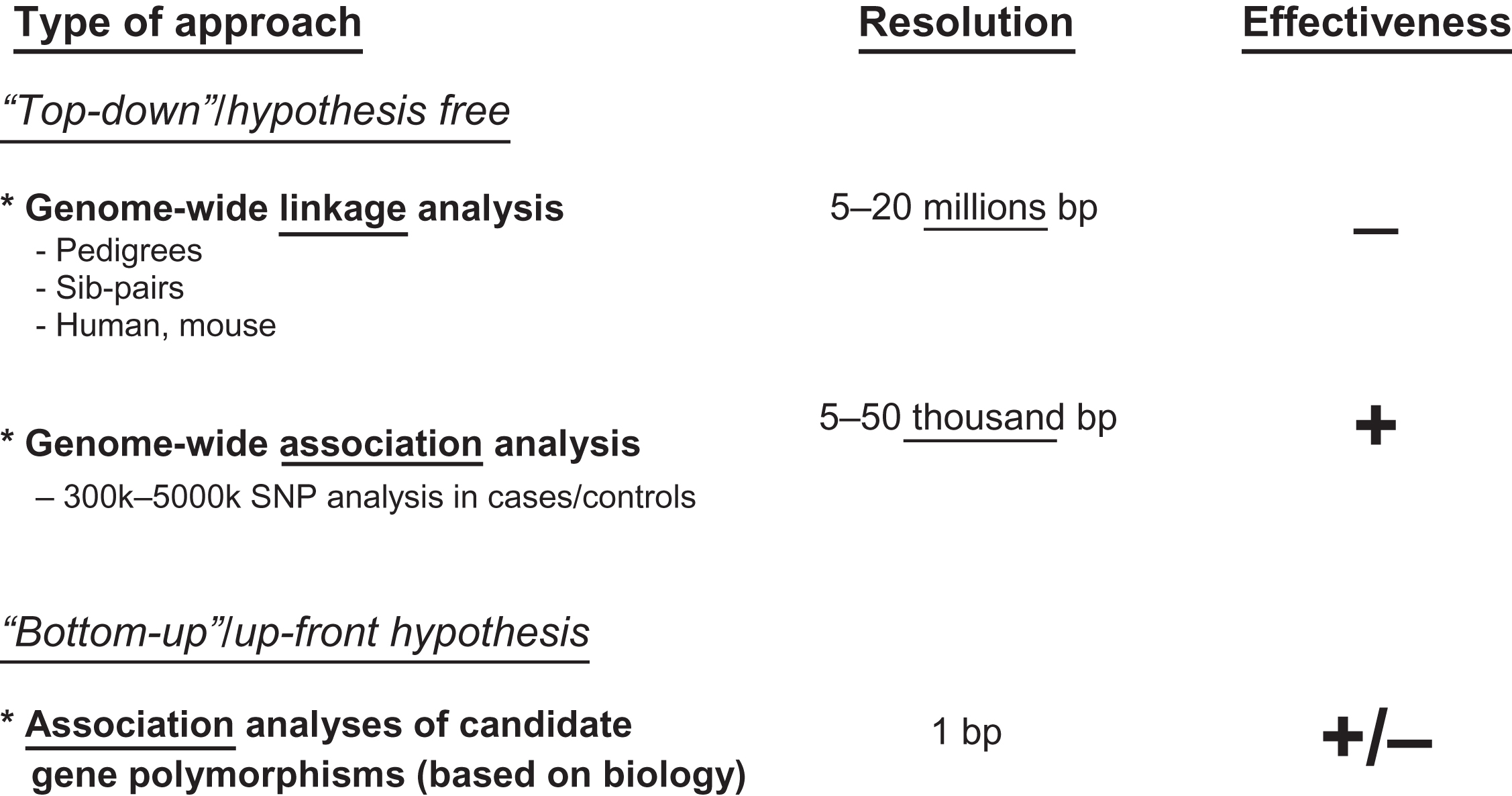
18.2.2
Genome-wide searches and candidate genes: top-down versus bottom-up
In top-down approaches, genome searches are performed that indicate which chromosomal regions might contain DNA sequence variation in or near osteoporosis genes. This approach is known as hypothesis free because it does not make assumptions about which of the many genes are involved in the diseases of interest, in this case osteoporosis. Rather, it simply scans the genome to find regions that show more than expected transmission in linkage analysis of related subjects with the disease or are overrepresented in cases versus controls in association studies.
The genome-wide approach is referred to as genome-wide linkage scans when it is performed in hundreds of relatives (sibs, pedigrees, etc.) with hundreds to thousands of polymorphic DNA markers that are evenly spread over the genome and have a well-known location. Genome-wide linkage searches are based on the assumption that relatives who share a certain phenotype will also share one or more chromosomal areas identical-by-descent (IBD) containing one or more gene variants causing (to a certain extent) the phenotype of interest, for example, low BMD. The disease gene variant is considered then to be physically “linked” with the DNA marker used to “flag” a certain chromosomal region. Upon positive linkage, subsequent research will have to identify which one of the dozens of genes in the chromosomal area is the one involved in bone metabolism causing low BMD and then identify the particular sequence variant giving rise to this aspect of osteoporosis. Although this approach was widely employed in the early phase of complex genetic research, it has not resulted in the identification of risk genes for osteoporosis and in general also not for other complex diseases. This method has therefore been less applied than the more powerful approaches based on association analysis.
The GWAS approach is also based on a genome-wide hypothesis-free scanning but (1) does this at a much higher density of DNA markers and (2) it does not require related subjects to be analyzed. It is preferably used in large sets of cases and controls or in population-based cohorts and uses the classical epidemiological tool of association analysis to establish a correlation between the disease and a DNA marker pointing to a causative gene for the disease. Overall, after more than 10 years of performing GWAS, we can conclude that it has been extremely successful in the identification of risk variants in or near genes for complex diseases. This is mostly due to the wide availability of (longitudinal) epidemiological study populations in which the power of novel genomic technologies can be applied in a setting of very rich phenotyping.
In contrast to the hypothesis-free approaches the bottom-up approach builds upon the known involvement of a particular gene in aspects of osteoporosis, for example, bone metabolism, as established by, for example, hits from a GWAS and cell biological and/or animal experiments. These genes are then considered as candidates to explain the genetic variance of the phenotype of interest, and this approach is referred to as the candidate gene approach. In such a candidate gene, sequence variants will then be tested for association with differences in function of the encoded protein and, thus, with the phenotype of interest by association analyses.
Genome-wide scanning approaches have attracted wide interest because they are expected to be neutral and unbiased and to identify true and major genetic effects (the “low hanging fruit”). In contrast, earlier candidate gene approaches were prone to heavy bias, relied on previous knowledge, and so were not able to provide (much) new biological insight. The results obtained with linkage approaches in sib pairs have been essentially negative for most if not all complex disorders, including osteoporosis. This is mainly due to methodological limitations of linkage analysis as indicated later. In contrast, GWAS has been extremely productive as a genome-wide scanning approach, as discussed below.
The field of genetics of osteoporosis started with the seminal Nature paper by the Eisman group on the contribution of VDR polymorphisms to BMD in 1993 , which was an example of a candidate gene analysis. This observation, corrected some years later with an attenuation of the initially reported, quite substantial, effect size , led to many replication attempts and, because of often conflicting observations, also to confusion in the field. This situation brought several European investigators to start collaborating and bring their data together in a consortium, called GENOMOS (see Section 18.2.5.2 ). Even in the absence of such genome-wide scans, this is a valid candidate gene approach to simply determine their particular contribution to the genetic risk for osteoporosis. Indeed, candidate gene analyses have identified genetic risk factors for osteoporosis, albeit of modest effect size and with a very low success rate in choosing the appropriate candidate genes. Importantly, the outcome of any genome-wide analysis such as by GWAS is the subsequent study of a particular candidate gene, so this approach will remain with us for the coming years in any case.
18.2.3
Biology versus genetics?
It is important to stress that genome-wide approaches to identify risk genes for a complex trait or disease will identify genetic risk factors, irrespective of biological knowledge on the physiological process under study. Gene variants identified through such approaches are simply an indication of the existence within the population of genetic variation that contributes to differences in risk of diseases such as osteoporosis or are associated with variance in the population for a certain trait such as BMD. So, it is much more an evolutionary approach to understand how population genetic variation contributes to disease risk. It will identify those genes in which evolution over the past thousands to hundred thousands of years has allowed variation to occur in the population. Whether or not these genes are important, for example, to bone biology in case of osteoporosis, is a separate issue but sometimes these are confused. Some believe that genes that are important in bone physiology must also be important genetic risk factors for osteoporosis, but this is not necessarily so.
When a new gene has been discovered that is important in understanding bone homeostasis, such as the Wnt signaling pathway through the discovery of the LRP5 gene mutations or the SOST gene mutations, it is often assumed that these genes must also be important genetic risk factors for osteoporosis. Yet, the experiments done to identify these genes in bone biology, that is, cloning from families with a monogenic disease, generation of knockout (KO) mouse models, have only highlighted their crucial role in how bone is formed, remodeled, maintained, etc. This says virtually nothing about the presence or absence of DNA polymorphisms that could contribute to population variance of bone phenotypes. Such new bone genes are more important in understanding which and how certain pathways are involved in bone biology and, thus, could be targets for drug intervention to modulate certain bone phenotypes. On the other hand, GWAS is especially worthwhile because of its hypothesis-free and genome-wide nature, in identifying truly novel biological pathways, which hitherto were not implicated in bone biology. This deepens our knowledge of bone biology and creates vast possibilities for development of novel interventions. In other words, it is capable of rewriting the textbooks (such as this one).
18.2.4
Genome-wide linkage analysis
18.2.4.1
Genome-wide linkage analysis for bone mineral density and osteoporosis
Finding the responsible gene for monogenic disorders (caused by rare mutations in a single gene) has been a straightforward routine exercise for specialized laboratories and involves a genome-wide linkage analysis of some families. This gene finding is based on linkage analysis in pedigrees (in which the disease is segregating according to Mendelian laws), whereby standardized sets of well-characterized DNA markers (mostly SNPs as present on arrays) are analyzed for cosegregation with a phenotypic endpoint.
For linkage analysis, hundreds of relatives (sibs, pedigrees, etc.) are genotyped for hundreds of DNA markers evenly spread over the genome. Most genome searches focused on humans, although mouse genome searches have also been performed for bone-related endpoints. Such genome searches are based on the assumption that relatives who share a certain phenotype will also share one or more chromosomal areas identical-by-descent containing one or more gene variants causing (to a certain extent) the phenotype of interest (e.g., low BMD). The gene is then said “to be linked” with the DNA marker used to “flag” a certain chromosomal region, but this area is usually several million base pairs long. Upon positive linkage, subsequent research will then have to analyze dozens of genes in the chromosomal area to determine which one is (are) the one(s) involved in bone metabolism and then identify the particular sequence variant in that (those) gene(s) giving rise to (aspects of) osteoporosis.
We summarized in the previous editions of this book (2006 and 2012) genome-wide linkage studies done in human populations and in animal studies, but none of these efforts have resulted in identification of a robustly replicated osteoporosis gene in humans. Power calculations have shown that one can expect with a few hundreds of sib pairs in a human linkage study, to be able to detect genes with effects explaining roughly 20%–30% of BMD. We now know through GWAS that these very strong common genetic effects on BMD are not present in the human population, thus explaining why such linkage approaches have failed. We will therefore not discuss these here, and reference to them can be found in the previous editions of this book.
18.2.4.2
Whole-exome sequencing studies of monogenic bone disorders
Nowadays, family analysis of Mendelian diseases is made much more direct by WES of patients and some controls to directly find causative mutations. But still, the net result of such a family/pedigree WES analysis is the identification of a novel bone gene (see Refs. ). This approach is used to discover a gene that plays a role in bone biology, but it does not necessarily mean that common variants in this gene play a role in risk for osteoporosis (see earlier). In other words, linkage analysis in families with severe monogenic bone disorders is a source of novel bone biology and candidate genes that can be scrutinized for their contribution to population variance in risk of disease.
Examples of such an approach is the identification of LRP5 gene mutations being responsible for osteoporosis pseudoglioma as well as for a trait called high bone mass (see later); more recently, the use of WES has facilitated the identification of mutations responsible for early-onset osteoporosis and new forms of OI in WNT1 and for X-linked osteoporosis in PLS3 . Many other examples in the bone field exist and so, for single-gene diseases, this approach works very well to identify “bone genes.” However, from a methodological point of view the complex (and non-Mendelian) character of osteoporosis makes it quite resistant to the methods of analysis that in the past decades have worked so well for the monogenic diseases. Yet, interestingly, with the hundreds of osteoporosis genes having been discovered through GWAS now (see later for more details), investigators have also noticed that there is an overrepresentation of these Mendelian genes among them , as also seen for other complex traits and diseases. At least 15 genes that are found mutated to cause rare Mendelian disorders presenting with skeletal fragility phenotypes also carry more frequent variations in the normal population that contribute to variation in BMD . Some examples include LRP5 , GALNT3 , RIN3 , TNFRSF11A , TNFRSF11B , SOST , SP7 , CLCN7 , among others.
18.2.5
Association analysis of candidate gene polymorphisms
The bottom-up approach to identify genetic risk factors for osteoporosis can build upon previous biological knowledge, that is, the known involvement of a particular gene in aspects of osteoporosis, for example, bone metabolism (see Fig. 18.3 ). Alternatively, once a GWAS hit has been identified one or more of the genes in such an area can be referred to as a candidate gene, where additional functional evidence is required to confirm this an osteoporosis gene. In any case, such a gene is then referred to as a “candidate gene.” The candidacy of such a gene can be established by several lines of evidence including:
- 1.
GWAS identifying a small genomic region containing one or several genes showing a robust association to an osteoporosis endpoint. GWAS does not by itself immediately identify one particular gene.
- 2.
Cell biological and molecular biological experiments indicating, for example, bone cell-specific expression of the gene.
- 3.
Animal models (e.g., mouse or zebrafish organisms) in which a gene has been mutated (e.g., natural mouse mutants), overexpressed (transgenic mice), or deleted (KO mice) and which result in a bone phenotype.
- 4.
Naturally occurring mutations of the human gene resulting in monogenic Mendelian diseases with a bone phenotype, that is, so-called human KOs.
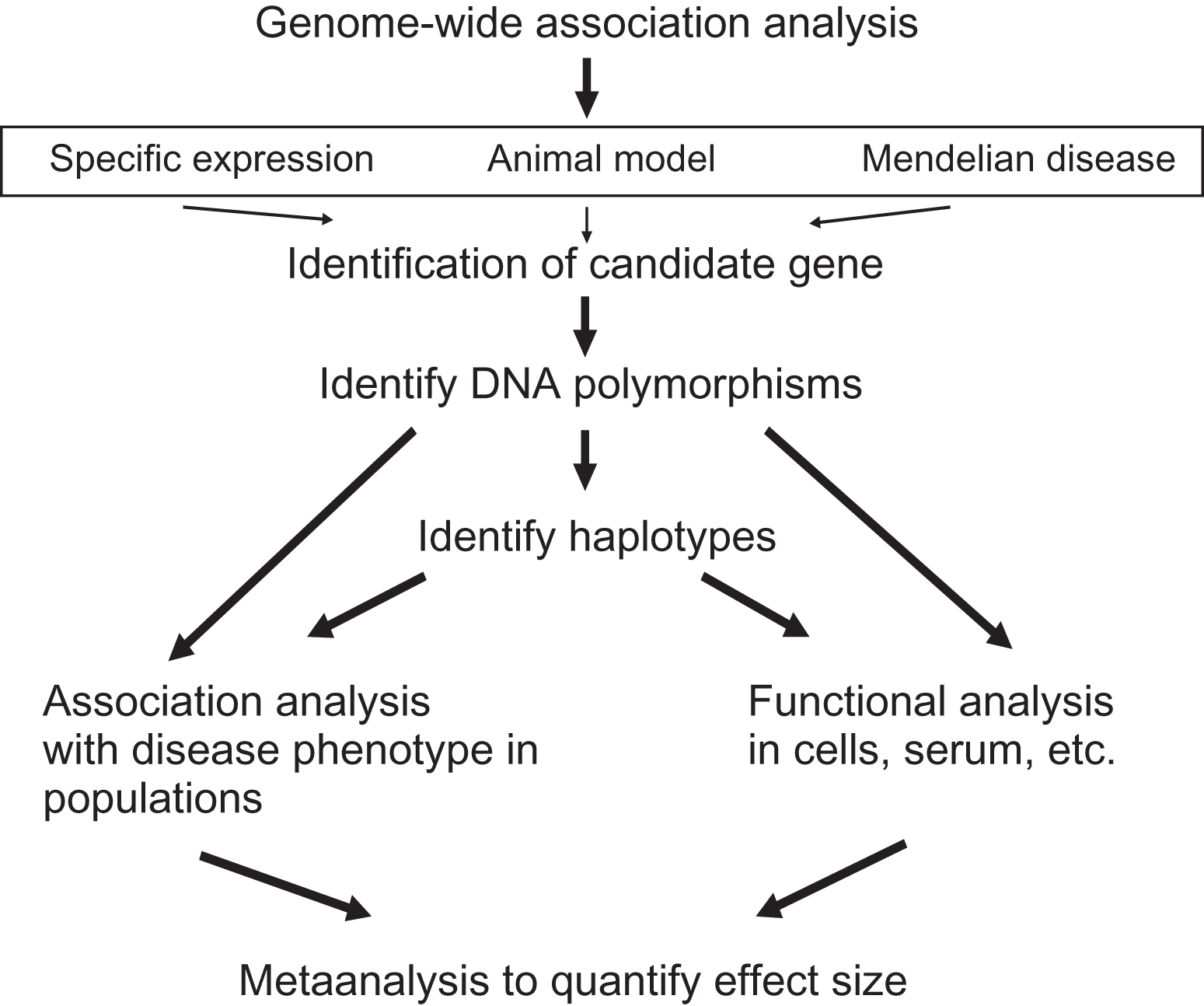
Subsequently, DNA sequence variations have to be identified in the candidate gene that leads to subtle differences in level and/or function of the encoded protein. Such sequence variants are widely documented in several databases (e.g., NCBI, dbSNP, HapMap, 1000 Genomes, and several more specialized databases). The scrutiny of all of these DNA sequence variations is still underway. The picture that now emerges is that per gene region dozens of such DNA sequence variations will have consequences for the level and/or activity of the protein encoded (functional polymorphisms). These can include, for example, sequence variations leading to alterations in the amino acid composition of the protein, changes in the 5′-promoter region leading to differences in mRNA expression, and/or polymorphisms in the 3′-region leading to differences in mRNA degradation. Clearly, it depends on the gene how many and what kind of polymorphisms will be present in the population. Some genes have been, for example, under more evolutionary pressure and will not display much variation. Other genes, however, might be part of a pathway with sufficient redundancy to allow for more genetic variation to occur whereby subtle changes in level/function of one protein can be compensated for by another protein in the same pathway.
Polymorphisms of interest are usually further tested in population-based and/or case–control “association studies,” to evaluate their contribution to the phenotype of interest at the population level. However, association studies do not establish cause and effect; they just show correlation or cooccurrence of one with the other. Cause and effect of such DNA sequence variations have to be established in truly functional cellular and molecular biological experiments involving, for example, transfection of cell lines with allelic constructs and testing activities of the different alleles. This can occur at different levels of organization (see Fig. 18.4 ) and depends on the type of protein analyzed, for example, enzymes versus matrix molecules versus transcription factors. Acknowledging these complexities, it will remain a challenge, once an association has been observed, to identify the correct test of functionality. And vice versa once functionality has been established, to identify the correct endpoint/phenotype in an epidemiological study.
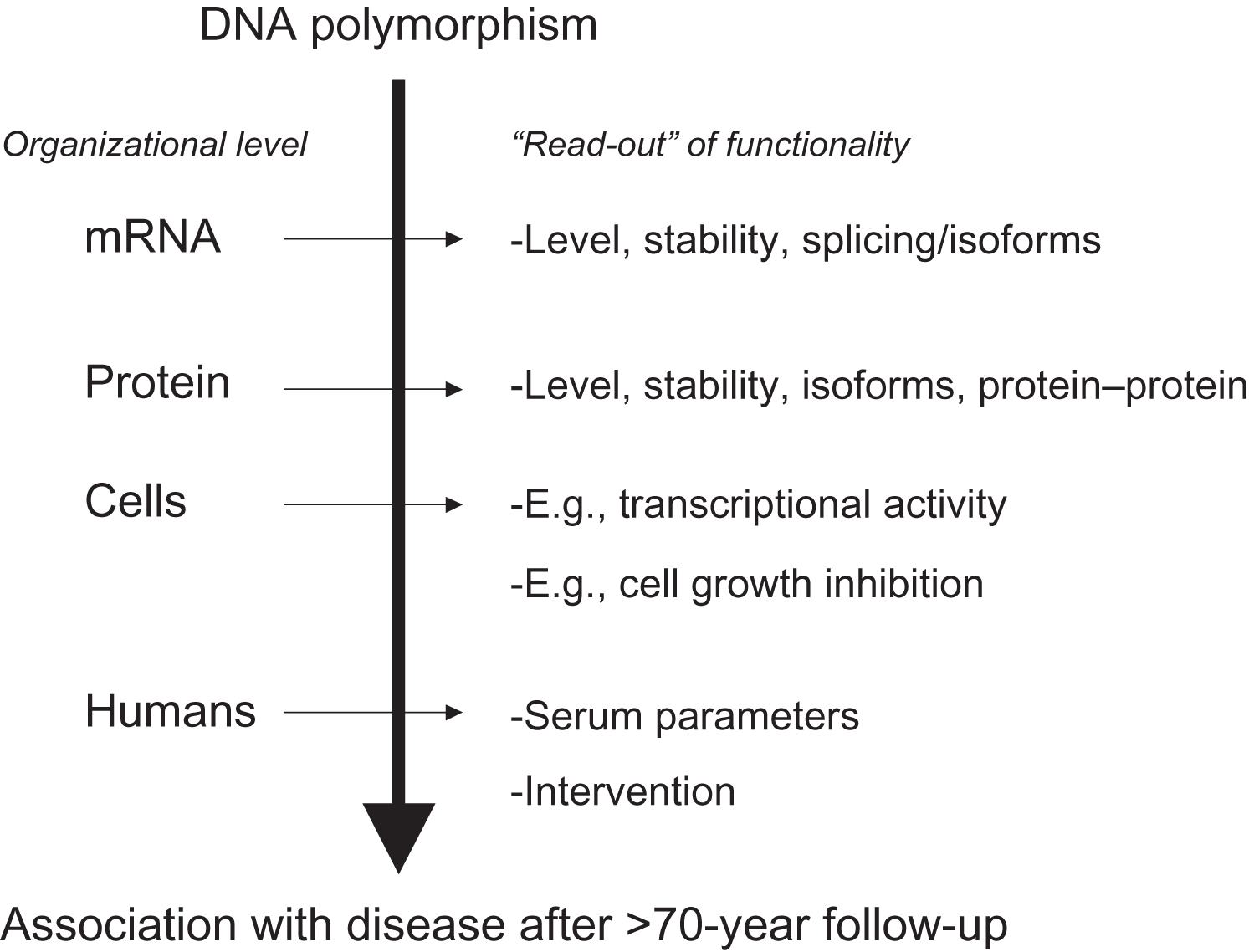
Because functional polymorphisms lead to meaningful biological differences in function of the encoded “osteoporosis” protein, this also makes the interpretation of association analyses using these variants quite straightforward. For example, for functional polymorphisms, it is expected that the same allele will be associated with the same direction of effect on the same phenotype in different populations. This can even be extended to similar associations being present in different ethnic groups, although allele frequencies can of course differ by ethnicity, as well as the genetic context (i.e., the collection of other DNA sequence variations in such genomes of other ethnic origin that might interact with the variation of interest) within such ethnic groups will likely be quite different . Thus complicating factors in such cross-ethnic comparisons are the genetic background in which such a variant is, which can enhance or diminish the genetic effect of that particular variant. So, although it is true that certain genetic variants can show their effect no matter what circumstance or background (so-called universal or cosmopolitan variants), there are also circumstances in which this will not be the case.
Due to the very nature of GWAS the current focus in genetic studies of osteoporosis is quite strongly on common variants that explain a certain proportion of population variance, due to their frequency in the population (5%–50%) and modest individual effect sizes. However, also more rare variants (<5% or even less frequent variants below 0.5%) will present somewhat stronger effects but with limited contribution to population variance. In addition, such less frequent variants might be important in certain populations but not in others, as is also illustrated by the so-called GWAS catalog documenting by now >150,000 GWAS associations for more than 4000 traits and diseases.
18.2.5.1
Haplotype blocks and topological associated domains
From resequencing studies for the dbSNP database, it has become evident that, on average, 1 out of every 100 bp is varying in the population. Given an average size of 50–100 kb of a gene, this means there are hundreds to thousands of polymorphisms in a given gene. Thus candidate gene analyses will have to focus on which of the many variant nucleotides are the ones that actually matter. That is which sequence variation is functionally relevant by changing expression levels, changing codons, etc. Given the average size of a gene and the relatively young age of human populations, it can be predicted that several sequence variations “that matter” will coexist in a gene in a given number of subjects from a study population. A major challenge of fundamental research will therefore be to unravel the functionality of these variations and how they interact with each other within the gene to determine gene function.
It has become clear that neighboring polymorphisms are not independent of each other in genetic terms, that is to say they are in LD meaning they tend to “travel together” in so-called haplotypes . Haplotypes are strings of coupled or linked variants, which occur, on average, over a distance of 10–30 kb on a chromosome. With polymorphisms occurring roughly 1 out of 100 bp, this means there will be dozens of polymorphisms within these “haplotype blocks.” This information has been compiled into a catalog of common variation within the HapMap project where millions of polymorphisms are genotyped in several thousand subjects of diverse ethnic origin to document their interrelationship within different ethnic groups. The catalog has been expanded to include less frequent and rare variation derived from whole-genome sequencing (WGS) on even more diverse reference populations part of the 1000 Genomes project .
An important aspect of association analyses in this respect is then to establish which common haplotype alleles (rather than individual DNA sequence variants) are occurring in the candidate gene, which has two important practical consequences:
- 1.
If association is found of a particular allele of an individual polymorphism with a certain phenotype/disease, this can also be explained by (an) adjacent polymorphism(s) within the haplotype block. Thus one can never be sure what causes the association until the haplotype structure at that position within the gene has been resolved.
- 2.
When, for example, 20 polymorphisms are located within a haplotype block only a fraction (typically only one-third) has to be genotyped to identify the haplotype alleles. This saves on time and money to perform the association analyses while obtaining maximal information relevant for point 1. This aspect has been crucial in the design and success of the so-called SNP arrays with which to genotype DNA for hundred thousands of DNA polymorphisms.
A typical gene can have one or several haplotype blocks covering the promoter region, another block covering the coding region and yet another block covering regulatory regions 3′ of the gene. Similarly, for the functioning of a complete gene in a given cell of a given subject, it is then important to know which combination of haplotype alleles is present in that subject. In Fig. 18.5 , a hypothetical example is given of the functional relevance of gene-wide combinations of genotypes (based on single SNPs or on haplotypes). The figure describes the situation when two subjects have identical genotypes for three adjacent polymorphic sites when analyzed independently. Yet, they differ in their combination of alleles on one chromosome, and this will result in different effects at the cellular level. This example illustrates that the effects of single polymorphisms might be difficult to interpret when ignoring the polymorphisms in the rest of the haplotype block and the other haplotype blocks in the gene. As can be seen for the VDR gene, this is not a trivial phenomenon with so many polymorphisms per gene.
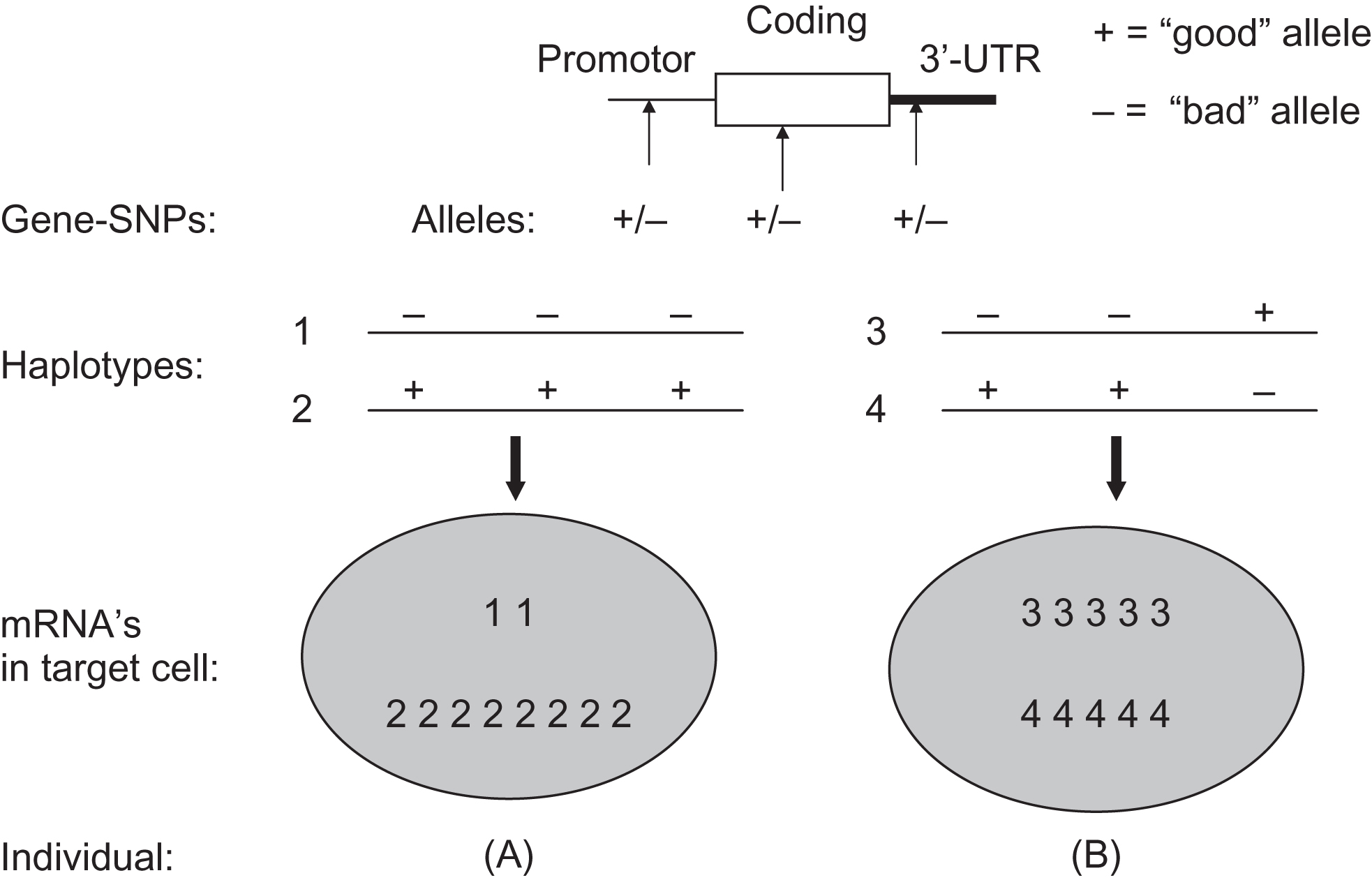
More recently, the concept of haplotype blocks derived from a lineal approximation to the structure and organization of the genome, which has been the rule along the last decade is progressively starting to be replaced. New types of relationships between genetic variants have emerged from considering three-dimensional chromosome structures in the form of topological associated domains (TADs) consisting of a self-interacting genomic region . By definition, variants within a TAD physically interact with each other more frequently than with those outside the TAD, constituting regions harboring important elements of the gene regulation landscape .
18.2.5.2
Metaanalyses of single polymorphisms (GENetic Markers for OSteoporosis consortium)
Numerous osteoporosis candidate genes have emerged out of the four lines of evidence mentioned above. From these the GWAS approach is the most robust and prolific source of identified genes as has been proven in the past few years, but those will be discussed below. The candidate genes from the other—biologically experimental—sources include “classical” candidate genes expected to play pivotal roles in bone biology, such as collagen type I , the vitamin D receptor , and the estrogen receptors among other bone-active genes. Yet, also Mendelian “bone” genes identified in the past decade through linkage analysis in pedigrees, such as LRP5 and SOST , have become candidate genes because of their newly established involvement in bone biology and since recently constituting novel drug targets for the treatment of osteoporosis .
With this plethora of candidate genes, it is difficult to decide where to start scrutinizing certain candidate genes. Initially, in the early 1990s, this happened somewhat randomly driven by personal preferences of individual scientists and led to much controversy in the field of genetics of osteoporosis. This was because of various reasons listed in Table 18.4 with pitfalls in the analytical process that have played (and sometimes still play) a role in the association analyses of candidate osteoporosis genes. Apart from these considerations, also somewhat seemingly more trivial factors can play a role. For example, the effect size, that is, the actual difference in a certain measured endpoint (e.g., BMD or number of fractures) between genotypes, should not be confused with reliability of the conclusions (the confidence intervals around the point estimate) or their significance (the P -value). Big effects (usually in small samples) that do not reach significance do not indicate that there is no relationship. It should instigate the investigator to increase the sample size because the current number does not allow a straightforward conclusion. The first line of defense against critique on this point usually includes power calculations. However, power calculations are frequently used in cases where there are small sample sizes (e.g., n <300) to demonstrate enough power (>80%) to detect unrealistic big differences such as 1 SD (standard deviation) in BMD or more, in population analyses of BMD by genotype.
Epidemiological | |
---|---|
1. Sample size is too small leading to chance findings | |
2. Population is biased due to selection, admixture, inbreeding, etc. | |
3. Environmental factors differ between populations | |
Genetic | |
1. Allelic heterogeneity: different alleles are associated in different populations | |
2. Locus heterogeneity: gene effects differ between populations due to genetic drift and founder effect | |
3. Linkage disequilibrium: one or more adjacent polymorphisms are the true susceptibility loci instead of the polymorphism being tested | |
Molecular Genetic | |
1. Low genetic resolution: unjustified grouping of alleles due to insufficient methodological discriminatory power (especially with VNTRs) | |
2. Anonymous polymorphisms: there is no known functional effect of the polymorphism to provide a direct biological explanation of the association | |
Problem | Solution ? |
Small sample size | Combine study populations (across Europe, globally): metaanalysis |
Ill-defined choice of polymorphisms | Rationalize choice of polymorphisms: functionality, haplotypes |
Lack of standardized genotyping | Standardize genotyping methods: reference DNA plate |
Lack of standardized phenotype data | Standardize phenotypes across populations: metaanalysis individual-level data |
Publication bias | Run prospective metaanalyses in consortia |
From the previous paragraphs, we can also see some solutions to commonly encountered problems in association analyses. Thus association studies are best done with functional polymorphisms in large populations. Intuitively, it is clear that small differences require a large sample size to be able to detect them. It therefore is quite useless to reiterate association analyses (i.e., same polymorphism, same endpoint but in different populations) in samples of about the same size or even smaller than the original study population. In addition, analyzing other—but still anonymous—polymorphisms will only add to the confusion rather than solve it. It is more informative to analyze functional polymorphisms and look at the relationship of this polymorphism with nearby SNPs such as they occur in haplotypes of alleles of adjacent SNPs in a region of genomic DNA. Finally, it is now established that prospective metaanalyses are the best way forward in establishing the contribution of a certain polymorphism to the risk of complex diseases, and osteoporosis is no exception to this.
So, if investigators were to embark on an association study of a candidate gene to identify genetic markers for osteoporosis, what would be the crucial issues to address? A few suggestions:
- 1
Take a large population.
Bigger is better to make your initial observations statistically robust.
- 2
Identify proper endpoints upfront.
Fractures are clinically the most relevant but you need substantial numbers to make your finding statistically robust. BMD is only one of the risk factors but it is a continuous trait and gives more statistical power. Population-based studies have the advantage of being able to switch phenotypes during analysis very easily, for case controls, this possibility is very limited.
- 3
Cover all relevant genetic variation within the gene.
Focus on functionally relevant variants within a gene. A clear-cut functional variant can be analyzed in isolation, ignoring the rest of the genetic variation in the gene. However, determine the haplotype structure to understand how the complete gene is functioning.
- 4
P -values: rather seek replication of your finding.
Simple adjustment for multiple testing is regarded as not appropriate (where to start and stop counting?). Rather, formulate a proper a priori hypothesis and seek replication(s) of the observed association in similar populations.
- 5
Perform a metaanalysis to quantify effect size and assess heterogeneity.
Join consortia with your population and datasets to standardize genotype and phenotype definition and estimate effect size of polymorphisms, preferably by prospective metaanalysis rather than metaanalysis of published data.
It will therefore be necessary to put all these genetic association data in perspective by performing metaanalyses of the individual studies. Metaanalysis can quantify the results of various studies on the same topic and estimate and explain their diversity. A systematic metaanalysis approach can estimate population-wide effects of genetic risk factors for human disease and large studies are more conservative in these estimates and should preferably be used . An analysis of 301 studies on genetic associations (on many different diseases) concluded that there are many common variants in the human genome with modest but real effects on common disease risk and that studies using large samples will be able to convincingly identify such variants .
We have tackled these uncertainties in the field of osteoporosis, by setting up the EU-funded GENOMOS consortium , which performed such studies using standardized methods of genotyping and phenotyping. GENOMOS also paved the way for subsequent GWAS metaanalyses performed within the (EU-funded) GEFOS consortium. The GENOMOS project involved the large-scale study of several “classical” candidate gene polymorphisms in relation to osteoporosis-related outcomes in subjects drawn from several study populations around the globe. Its main phenotypic outcomes included fracture risk and femoral neck and lumbar spine BMD, and design details are described in the first metaanalysis of individual-level data on the ESR1 gene , and in the subsequent metaanalyses of the COLIA1 gene , the VDR gene , the TGF-beta gene , and the LRP5 and 6 genes .
In Fig. 18.6 an overview is presented of the gene structure for four of the genes analyzed in the setting off the GENOMOS consortium, including the position and nature of the polymorphisms studied per gene. In Table 18.5 an overview of the results obtained with the GENOMOS metaanalysis is presented. The metaanalysis of three polymorphisms in the ESR1 gene [intron 1 polymorphisms XbaI (rs9340799; “rs” stands for reference SNP ID number to be found in the dbSNP database ) and PvuII (rs2234693) and the promoter (TA) variable number of tandem repeats microsatellite] and haplotypes thereof, among 18,917 individuals across eight European centers, demonstrated no effects on BMD but a modest effect on fracture risk (19%–35% risk reduction for XbaI homozygotes), independent of BMD . For the COLIA1 Sp1 polymorphism there was a 0.15 SD reduction in BMD for the thymine-thymine (TT) homozygotes . While no association with overall fracture risk was observed, there was a trend toward a 10% increase in vertebral fracture risk per T-allele resulting in a 33% increased risk in TT homozygotes. For the 5 VDR polymorphisms tested (Cdx2, FokI, BsmI, ApaI, TaqI), no association with either BMD or with overall fracture risk was observed , but a trend was observed toward an increased vertebral fracture risk, in this case for Cdx2 A-allele carriers. For none of the five tested TGF-beta polymorphisms an effect was observed , while GENOMOS did demonstrate an effect for the LRP5 coding polymorphism on BMD as well as on fracture risk . While the LRP5 polymorphism was heralded as the first GWAS finding in osteoporosis (see later), it was in fact already demonstrated in GENOMOS to play a role and also with better statistical power thereby providing better estimates of the effect size.
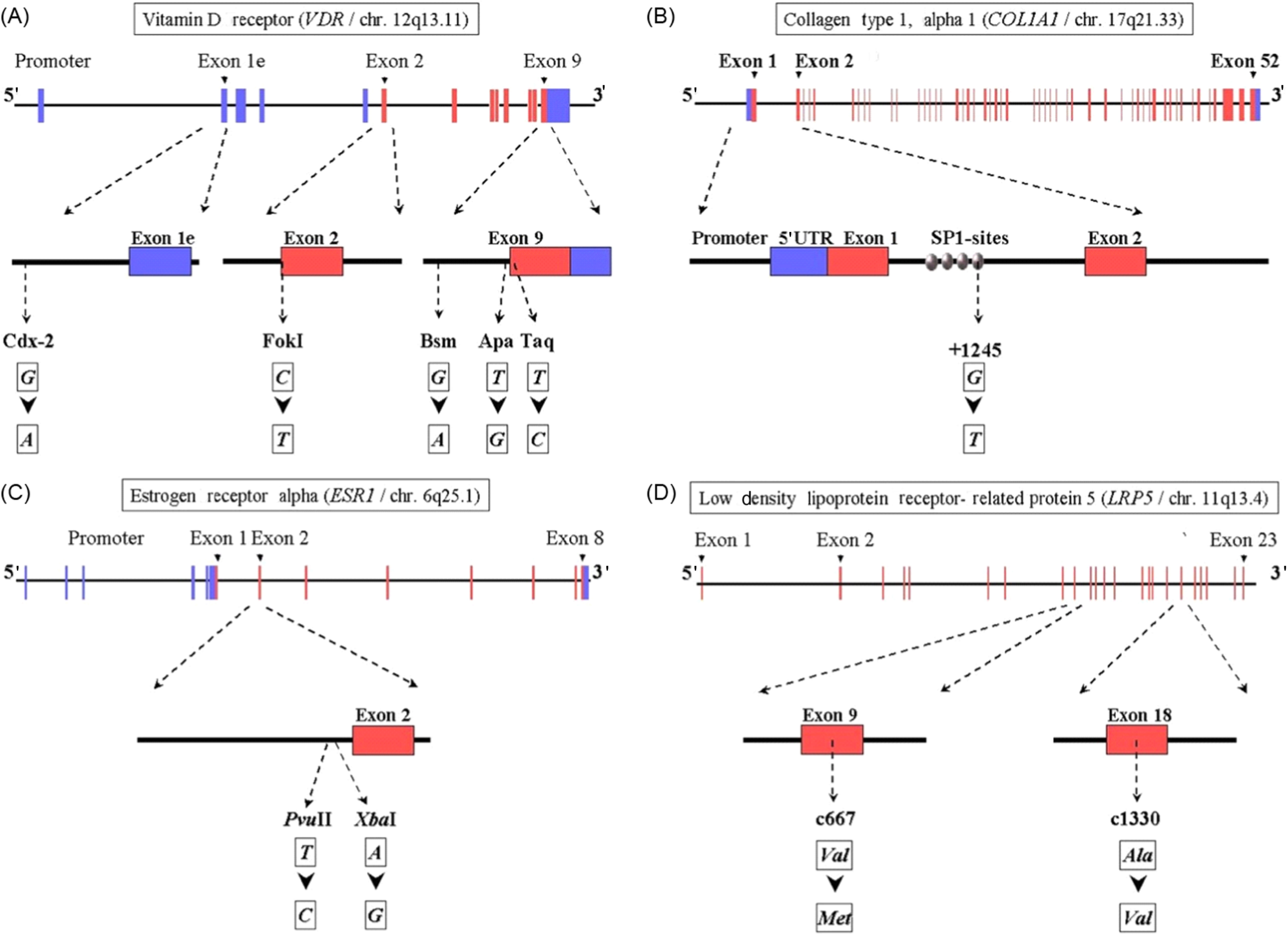
BMD | Fracture risk | ||||||
---|---|---|---|---|---|---|---|
Gene | SNPs ( n ) | Sample size ( n ) | Femoral neck | Lumbar spine | Vertebral fx (%) | Nonvertebral fx (%) | Reference |
ESR1 | 3 | 18,917 | – | – | 20–30 | 10–20 | |
COLIA1 | 1 | 20,786 | 0.15 SD | 0.15 SD | 10 (Sp1) | – | |
VDR | 5 | 26,242 | – | – | 10 (Cdx2) | – | |
TGFb | 5 | 28,924 | – | – | – | – | |
LRP5 | 2 | 37,760 | 0.15 SD | 0.15 SD | 12–26 | 6–14 | |
LRP6 | 1 | 37,760 | – | – | – | – |
Altogether metaanalyses of the GENOMOS consortium were shown to be an effective screening mechanism to determine the true contribution of particular genetic variants to some important parameters of osteoporosis: BMD and fracture risk. It has provided a high degree of evidence of involvement across what was then highly regarded risk alleles; some were shown effectively contributing to explain population variance in risk for osteoporosis but this has been shown to be quite modest at best (e.g., for LRP5 ) if at all (like VDR and COLIA1 ). Indeed, none of the classical candidate genes, such as VDR , TGF-beta , and COLIA1 , have been identified in the GWAS metaanalyses of GEFOS so far as associated with osteoporosis traits (see later). In view of experience with other complex diseases this comes as no surprise, but it was an important take-home lesson for the genetics community working on osteoporosis at that time, about the need for well-powered studies and the essential need for replication of the identified genetic associations.
Apart from it being a very large study of the genetics of complex disease within the latest analysis >45,000 subjects included (and which has grown to include >150,000 subjects), an important aspect of this study is its prospective multicenter design. This means the combined association analysis is done only after the genotype data were generated per center, thereby rendering it immune to possible publication bias. The targets of the study are polymorphisms for which some a priori evidence for involvement in osteoporosis is already present; it is not designed to be a risk gene discovery tool and therefore cannot, for example, assess all genetic diversity across a gene. This role, however, as mentioned previously has been taken up by the GEFOS consortium applying the GWAS approach (see further details in section 18.2.6 ). With such a diverse set of populations included in the GENOMOS consortium, possible population stratification could be a problem and should be controlled for in analyses, by accurately detailing ethnic background or as it is possible now by using genetic SNP array data.
Importantly, some functional SNPs can show similar effects across different ethnic groups in spite of different genetic background of the ethnic groups. In this respect, it has been demonstrated that genetic markers for proposed gene-disease associations can vary in frequency across populations, but their biological impact on the risk for common diseases may usually be consistent across traditional “ethnic” boundaries of LD blocks . Yet, it is also conceivable that some genetic variants will have particular “local” effects, either due to particular environmental factors and/or due to differences in genetic background. Such factors could mask or enhance the effect of the particular polymorphism of interest.
Thus such a metaanalysis approach used by the GENOMOS and GEFOS consortia will identify individual genetic risk factors, but it will probably also be instrumental in estimating the presence and effect size of genetic interactions (gene–gene) and gene–environment interactions. This approach will be followed for genes in a certain pathway, for which we know that interaction is likely, and can be extended to explore unexpected interactions. However, even with large studies of, for example, 150,000 subjects, this might be difficult to convincingly demonstrate. This stresses the need for even larger studies and consortia such as advocated by the HuGE network of investigator’s networks . Within GENOMOS and now in GEFOS such analyses have indeed been executed in collaboration with large (national) biobank collections with genotypes and relevant phenotypes.
18.2.5.3
Pleiotropic effects
When we consider risk factors for osteoporosis and fracture, factors other than characteristics of bone, such as BMD and bone architecture, have to be considered. These include the fall frequency of individuals, body height, body mass index, their cognitive abilities, their muscle strength, among many other factors. For comparisons of studies on “genetics of osteoporosis,” it is therefore important to first define what the endpoint of the analysis is. Bone is, of course, a major target tissue in the genetic analysis of osteoporosis. Yet, many, if not all, of the genes considered in the genetic analysis of bone density are expressed not exclusively in bone but in several other tissues. For example, collagen type Iα1 is the most abundant bone matrix protein but is also present in vessel walls, the skin, and in other matrices where they (also) play important biological roles. This phenomenon is referred to as pleiotropy, which is the involvement of a gene product (RNA or protein) in more than one metabolic pathway. Thus genetic variations in pleiotropic genes will have influences on more than one endpoint and their effect could be missed and/or could be influencing the outcome if one is analyzing only one particular endpoint. Furthermore, proteins can be part of metabolic pathways that can be active at different levels and under different control at certain time periods during development, the adult stage and during aging. Finally, the hierarchical position of the gene is of relevance, to determine if there is redundancy or if they are upstream or downstream genes within a physiological cascade or pathway. Upstream genes will tend to be master-control genes (e.g., genes from the steroid receptor family encoding transcription factors), variations in which will have a cascade of effects in several pathways. Downstream genes will be expected to have a more limited repertoire of effects in view of their more specialized nature.
From the one discussed previously, it is clear that often a single sequence variation in a single gene will not have a single effect. This makes the association analysis of sequence variations more troublesome but at the same time also more realistic. Naturally, the discovery of pleiotropic effects is driven by the availability of the polymorphisms that have been described in one of the genes under study. However, they also reflect the inherent complexity of biological (disease) processes; in that, a single protein is involved in multiple metabolic pathways. One example is the MTHFR gene that has been previously implicated in atherosclerosis through the homocysteine metabolism pathway but was also found to be associated with differences in BMD in population of postmenopausal Japanese women . The latter finding could reflect the involvement of MTHFR in the homocysteine metabolism possibly affecting collagen cross-linking. Such a pleiotropic effect could then reflect the involvement of this enzyme in both atherosclerosis as well as in bone metabolism. These biological processes share certain metabolic pathways encompassing matrix components, calcium-deposition, among other biological processes. This notion has been much strengthened by the discovery of homocysteine as a risk factor for osteoporotic fracture .
Thus for genetic analyses, the existence of pleiotropic effects could also be a reason to study the involvement of a particular gene variant that has been implicated in a particular disease process, in another metabolic pathway. At a different level, pleiotropic effects can also be considered relevant for the aging process, given the fact that
- 1.
many of the complex traits show an onset at advanced age and have an increased prevalence with age,
- 2.
aging has been shown to be associated with a functional decline reflected in many different pathways such as hormone metabolism, and
- 3.
many complex diseases show interrelationships.
Examples of such interrelationships in the field of bone and aging include the association of low BMD with stroke , the inverse relationship of plasma 25(OH)vitamin D with myocardial infarction , and the inverse relationship of osteoporosis and osteoarthritis .
18.2.6
Genome-wide associations studies
The HapMap project established that the human genome is organized following a haplotype block structure . This knowledge together with the emergence of high-throughput genotyping opened a novel approach to search the genome for genetic markers of disease: the GWA analysis (reviewed in Refs. ). In this approach, many hundreds of thousand SNPs are analyzed in sets of (usually) a few hundred unrelated cases and unrelated controls. The first successful use of a GWAS analysis using such high numbers of SNPs was reported by Ozaki et al. who, by means of a nowadays considered small-scale, case ( n =94)–control ( n =658) association study using 92,788 gene-based SNPs, identified significant associations between myocardial infarction and 2 SNPs in LTA (encoding lymphotoxin-alpha): one SNP changed an amino acid residue from threonine to asparagine (Thr26Asn) while another SNP in intron 1 influenced transcription level of LTA .
Klein et al. reported a GWAS of 96 cases and 50 controls for polymorphisms associated with age-related macular degeneration (AMD), a major cause of blindness in the elderly. Among 116,204 SNPs genotyped (using Affymetrix 100k chips), a tyrosine-histidine change at amino acid position 402 (T402H) in the complement factor H gene ( CFH ) was strongly associated with AMD. This polymorphism is in a region of CFH that binds heparin and C-reactive protein. The CFH gene is located on chromosome 1 in a region repeatedly linked to AMD in family-based studies. This indicates that the effect size of this SNP was rather large as was already indicated by the low number of cases and controls required to obtain genome-wide significance.
The relatively low density of SNPs used in these first studies in combination with the limited genetic complexity (of AMD in particular with such a strong genetic effect as CFH ) could explain why only a few associated regions were observed in these studies, while one would expect (many) more gene regions to show up. Indeed, later studies using higher density arrays have identified a much higher number of significant associations. The GWAS approach has since then been applied in diverse areas of complex disease genetics and seen great success in identifying genetic risk factors . These results from GWAS show the great potential they have for elucidating complex disease and osteoporosis is no exception. Indeed, the scientists working on genetics of osteoporosis were among the first in the ever-growing field of complex genetics to adopt and embrace this novel approach of GWAS to find genetic markers for a disease and related phenotypes.
Although GWAS has been very successful, some limitations should be mentioned here as well. Initially, GWASs were quite expensive because of the costs of the array and the many samples need to find significant results. Although there are exceptions, most GWAS results involve SNPs with modest-to-very-modest individual effect sizes and which are located in genome regions without annotation. This limits their immediate use as genetic risk factor for prediction and their use to identify drug targets. Another requirement for successful GWAS is a collaboration with other groups (for sample size and replication) which—initially—was seen as a limitation hindering fast progress in discoveries and individual careers, also given the sometimes very large list of contributing authors. Technically, GWAS is limited to detect associated genetic variants that are relatively common, for example, with a minor allele frequency (MAF) of (initially) >5%. While because of better imputation panels and larger sample sizes, this MAF is now lowering to >0.1%, GWAS cannot detect rare to ultrarare variants where LD mapping is not feasible due to limited amount of correlation between rare variants. Finally, also some of the shortcomings that are well-known in classical epidemiology also apply to GWAS. For example, because of its hypothesis-free nature, one can subject any given collected phenotype, including more exotic ones of “behavioral” or “social” parameters such as household-income, wine preference, or entrepreneurship, to a GWAS and find some hits if sample sizes are large enough. Immediately, interpreting the mechanism behind such a relationship then remains quite challenging.
While GWAS arrays were initially quite expensive, costing roughly between 400 and 1000 euros per DNA sample (depending on which array type is chosen), the price has drastically decreased in time to now fall under 30 euros, enabling thousands to millions of samples to be genotyped in recent times. Yet, the same statistical requirements apply as in a given case–control or population-based study of a candidate gene polymorphism. Luckily, such drop in price has facilitated the study of complex traits, allowing to bring together data from hundreds of thousands of samples. From current evidence after 10 years of GWAS the dissection of complex traits has been a successful venture bringing genetic discoveries each time closer to clinical application.
Given the cumulative initial high cost of genotyping DNA samples with SNP arrays and the relatively modest predictive value of SNPs, some critics have stated that GWAS has been a waste of time and money. This is not the place to go into this complex argument deeply that involves issues on which research in general to fund with what amount of money. But, suffice to say that GWAS has led to a number of important findings including:
- 1.
Opening up our molecular understanding of complex diseases by convincingly identifying—for the first time—some of the genetic factors involved, which include elucidating a role for known drug targets.
- 2.
Bringing to light of abundant novel biology (~50% of GWAS hits across different phenotypes and diseases concern genomic regions with hitherto unknown function) which will simply take time to unravel and appreciate, and are likely to include new drug targets.
- 3.
Being the “top of the iceberg” of scientific insights, because GWAS genotyping has often been applied to cohort studies with literally hundreds of phenotypes of which only a fraction have been scrutinized by GWAS. Such cohort studies have, in addition, seen a big “bang for the buck” given that the same dataset is used in GWAS for many different phenotypes.
- 4.
Constituting the jumpstart of success in the field of complex traits (osteoporosis included) with clear successes after 10 years, after more than two decades of unfruitful attempts.
- 5.
Having a relative high success rate considering that only 0.3% of the human genome nucleotides have been assessed. Already there is arising an understanding of abundant new biology, while an explained variance of >50% has been obtained for multiple phenotypes (as compared to 0% for genetic markers for complex disease before GWAS).
- 6.
Giving a very large impetus to changes in the way good scientific research is done in human genetics and beyond, that is, in establishing collaboration groups and by replicating experiments and findings.
18.2.6.1
Microarray technology and quality control
With no doubt the advent of GWAS has been facilitated by the availability of microarray technology. A microarray (also called DNA chip) is a small glass slide with short DNA probes attached to it over which fragmented DNA is washed. Pieces of these DNA fragments hybridize to the chip producing luminosity from the reaction. The intensity can then be detected and quantified by a high-resolution laser and scanning software in order to produce genotype calls. In this manner, hundreds of thousands to millions of genotype calls can be obtained in one sample. The GWAS microarray market has evolved rapidly and two main vendors, Affymetrix and Illumina, dominate the market. Historically, Affymetrix has offered chips with SNPs based on XbaI/HindIII and Nsp/StyI digestion that are more or less evenly spread across the genome. Densities have increased over time from 10,000 (10k) to 100k (actually 2×50k) to 500k and now to 1.8 million markers. The Perlegen Company offered an in-house service using Affymetrix chip technology containing 200k–1000k haplotype tagging SNPs but these are not used anymore. Illumina Infinium is using glass arrays that are spotted at high density with very selected haplotype tagging SNPs, such as coding SNPs (100k), or the HumanHap 300k–550k SNPs. A new set of arrays with specific marker content (OMNI family) has constituted a new generation of arrays summing to 700k, 2.5M, and 5M series, where the latter two are enriched with 1000 genomes project content. More recently, the market has been governed by the Global Screening Array from Illumina and the Axiom Precision Medicine Research Array of Affymetrix, which hold very comprehensive genomic coverage and their affordable price (less than $30) have made very large-scale studies a reality.
Although microarray technology has now evolved sufficiently to be considered as “robust,” important aspects of quality control are required to preserve a successful study design. This is particularly true, considering that GWAS focuses on searching for variants with modest effects in what usually constitutes very large epidemiological studies. Conscientious quality control is required to limit potential sources of error, which can generate biases that can easily be translated into false-positive findings or which will hamper the power of a study. The main controls rely on checks over batch effects, marker quality, sample genotyping efficiency, sample identity/relatedness, and population substructure (discussed below).
Batch effects refer to the partitioning of samples for processing in units, which most frequently constitute genotyping plates, encompassing 48, 96, or 384 wells with DNA samples in it. Systematic differences in genotyping affecting plate accuracy and efficiency will be reflected in the association analysis as batch effects.
Therefore the composition of individuals in a batch should be random and not reflect an enrichment of subgroups used in the analyses (i.e., the case–control ratio should be ~1 across multiwell plates containing DNA samples, or at least they should be randomly distributed across plates). Several aspects of marker quality also need to be considered. Marker call rate will be an indicator to identify those SNPs with poor genotyping performance that should be excluded from analysis, and commonly used thresholds include exclusion of marker genotyped (call rates) in less than 95%–98% of individuals. The per sample genotyping efficiency or call rate refers to the number of markers successfully called in one given sample and common inclusion thresholds of 95%–98% per individual are usually applied to exclude what usually arises from problems in DNA quality. In addition, determining the amount of heterozygous calls in a given sample will allow identifying sources of DNA contamination detected by excess of heterozygosity. Using the X-chromosome and/or genome-wide data can help detecting problems of sample identity that typically arise from sample handling errors. Checking the reported sex of each individual against that predicted using the genetic data (i.e., X-chromosome heterozygosity rates) can detect sample swaps. Despite that this check will be fairly sensitive, it will not identify the same-sex swaps in the study and can be misled by long homozygocity stretches of the X-chromosome or chromosomal alterations of the X-chromosome (i.e., Klinefelter syndrome XXY karyotype in males or Turner syndrome XO karyotype in females). Genome-wide data can also be used for the purpose of tracking samples by means of measuring the extent of genomic data sharing across individuals. Pairwise identity-by-state and identity-by-descent estimations allow identifying duplicates in the data and/or determining cryptic family relatedness between individuals.
18.2.6.2
Genomic control for population stratification
Population stratification is an omnipresent threat to the validity of genetic association studies and GWAS are not immune to it. Fortunately, the use of genome-wide genotyping data allows employing different strategies to avoid this kind of bias either by correction of the test statistics using the genomic control procedure or by correction of the effect estimates by employing genetic principal components as covariates in the statistical models. Population stratification occurs in the presence of undetected population structure whereby study samples comprising sets of individuals differ systematically in both genetic ancestry and the phenotype under investigation. Instead of identifying true association of alleles to disease phenotypes, spurious associations arise which are fully explained by differences in ancestry.
The most straightforward way to avoid population stratification biases is ensuring that the study sample is derived from a relatively genetically homogenous population. Of course, this is not always possible and is the reason why statistical methodology needs to be applied to detecting and adjusting for population stratification. Genomic control aims to control for population stratification by first estimating the degree of inflation of the test statistics by comparing the median distribution of the test statistics for association as compared to that under the null (no association) distribution. Inflation of the test statistic can be the result of population stratification, cryptic relatedness between the samples, genotyping error, or be due to true association. The inflation is quantified in the form of the genomic inflation factor ( λ ), which is used to correct downward the test statistics by this factor under the assumption that the test statistics are equally inflated at each locus across the genome, which is not usually the case. A genomic inflation factor close to unity reflects no evidence of inflation, while values up to 1.10 are generally considered acceptable for GWAS. Another (preferred) method utilizes large samples and thousands of markers throughout the genome to estimate pairwise allele sharing between individuals (described above) and use the IBS matrix for all individuals to obtain a given number of principal components to adjust the effect estimates for population structure. Mixed models that allow including the pairwise relationship matrix in the models to correct for population stratification and cryptic family relatedness are currently the preferred approach, despite being much more computationally demanding.
18.2.6.3
Genotype imputation and metaanalysis
Once the genotyping has been subject to quality control and the degree of population stratification has been assessed (and controlled for), SNP-phenotype associations can be performed. Nevertheless, the evidence for association will usually remain far from conclusive in a given study (due to small effect size per SNP). This is the reason why sharing results for a larger suitably powered metaanalysis, usually in the context of consortia, is the next step forward.
As discussed previously, there are different genotyping platforms, each with different types of arrays differing in marker content. In order to be able to metaanalyze the data, genotype imputation needs to be performed to obtain a standard set of markers across studies and which is usually arising from a public reference [i.e., the HapMap panel containing Caucasian (CEU), Asian (JPT/CHB), and African (YRI) samples] but now being replaced by large sequenced reference samples including the 1000 Genomes project (1 kGP) and the Haplotype Reference Consortium (HRC) panels. Before genotype imputation, the genotyped data undergoes all the quality control procedures described above plus some strict marker pruning whereby SNPs with MAF <0.01, SNP call rate <0.98, and Hardy–Weinberg P -value <1×10 −7 are excluded. This will enhance the performance of imputation algorithms under the assumption that low-quality genotyped SNPs can be recovered by imputation. Imputation is the solution to a problem of missing information. In a nutshell, genotypes generated by a given platform are phased to haplotypes for each sample (i.e., determine the adjacent SNP alleles on a chromosome), aligned to the phased haplotypes of the reference panel with a much richer SNP density (such as HapMap or 1 kGP), and allowing specific mathematic algorithms to infer the missing genotypes in the form of genotype probabilities which are frequently converted to allelic dosages ranging between 0 and 2 in the association analyses. The result is that independent of which genotyping platform has been used (and the contained SNP sets therein) genotype dosages for the same set of markers in the reference (~2.5 million in CEU samples for HapMap v2, and now including 14 million variants with HRC) can be metaanalyzed across studies for evidence of association with the phenotypes of interest.
The GWAS metaanalysis will procure the identification of markers associated with traits at a genome-wide significant level, which under current standards with Bonferroni correction remains at P <5×10 −8 . This significance level is assumed to correct for multiple testing considering the number of independent tests across the genome (determined by the LD blocks of common variants in the human genome). While the number of genetic variants in the imputation reference panels has expanded substantially over the past decade, this significance threshold has remained stable because it is based on the estimated number of LD blocks in the human genome. However, once less frequent to rare variation is analyzed, this LD-block model needs reconsideration and more stringent thresholds (e.g., P <1×10 −9 ) will be more appropriate. Similar considerations are valid when other ethnicities are analyzed by GWAS, given that the LD structure of, for example, the African genome is more fragmented than the Caucasian genome.
The preferred approach in GWAS metaanalysis is to use a fixed-effects metaanalysis while reporting the degree of heterogeneity in the results. Such heterogeneity is assessed by the significance of the Q-Statistic and quantified by estimation of the I -square ( I 2 ) (%) metric. In general, values 25%< I 2 <50% are said to represent a moderate source of heterogeneity, while I 2 estimates >50% are said to indicate that a high source of heterogeneity is present in the SNP-phenotype association. In the latter case a metaanalysis with random effects is indicated, with further study to identify the potential sources of high heterogeneity.
18.3
Identifying osteoporosis risk gene variants
18.3.1
Gene finding methods
Whereas the top-down approach, as discussed previously, encompasses hypothesis-free genome-wide searches which will identify one or more chromosomal regions containing such candidate genes, the bottom-up approach involves the a priori choice of a particular, known gene to be searched for polymorphisms that might contribute to population variance of one or more risk factors for osteoporosis, nowadays directed to assess the functionality of SNPs in the region. The choice of such a candidate gene is guided by considerations that revolve around the involvement in bone biology. Several lines of evidence can be followed to establish such a role in bone biology of a particular gene product. For example, mutations in the gene-of-interest lead to a known Mendelian disease of bone or, vice versa, the gene responsible for a Mendelian bone disorder will be of interest to screen for polymorphisms and to be evaluated in association analysis.
The involvement in bone biology can also be established when the gene-of-interest is knocked-out in mice and a bone phenotype occurs, or simply when the gene product occurs specifically in bone tissue. Thus an osteoporosis gene product will have characteristics all more or less in line with at least one of these considerations. In view of this rather wide definition, it can be expected that there are many potential osteoporosis candidate genes. Examples will be discussed later of the approaches and considerations that can lead to the choice of osteoporosis candidate genes to be studied further. In addition, a few particular candidate genes will be discussed in more detail because of the scrutiny they have already undergone.
18.3.1.1
Linkage analysis of monogenic bone metabolism syndromes
Conventional linkage analysis in families in which a, usually rare, metabolic bone disorder or skeletal dysplasia is segregating as a Mendelian monogenic trait can lead to the discovery of genes playing a role in bone metabolism. Most often mutations in the coding sequence of such genes lead to the severe phenotypes, characteristic of the syndrome. Whether polymorphic variants of such genes have possibly milder effects and are important for aspects of osteoporosis at the population level have to be determined by association analysis in large-scale epidemiological studies. Over a hundred skeletal dysplasias have been described for which the responsible genes are known. A well-known example is OI which is caused, among others, by mutations in the bone matrix protein components collagen type Iα1 and Iα2 (see Section 18.1.1 ). Consequently, these genes have been searched for polymorphisms to be associated with osteoporosis.
In addition, genome searches are applied in “single-gene” Mendelian bone disorders to discover the responsible genetic defect. Examples include human osteopetrosis, also known as Albers–Schönberg disease which describes a group of hereditary disorders characterized by abnormal bone resorption . Three clinical forms exist which have been mapped to different locations: autosomal recessive or infantile osteopetrosis (11q13), autosomal dominant osteopetrosis (1p21) and osteopetrosis with renal tubular acidosis which is known to be caused by mutations in the carbonic anhydrase gene (8q22). All these are osteoporosis candidate genes that can be searched for polymorphisms to contribute to explaining population variance in, for example, BMD. Yet, by now it is more efficient to do “lookups” of these genes in large GWAS datasets (preferably from metaanalyses such as in GEFOS) to determine how high they rank in that respect.
A localization of interest in this respect includes the mapping on chromosome 11q12–q13 of a locus for high BMD, containing the putative high bone mass (HBM) gene, in an American Caucasian pedigree , for autosomal recessive osteopetrosis in two Bedouin pedigrees , and for the low BMD/osteoporosis pseudoglioma syndrome , all with high LOD (logarithm of the odds) scores to the same DNA marker (D11S987). This locus is now known to contain the LRP5 gene, mutations in which have been shown to underlie both HBM and the osteoporosis pseudoglioma syndrome. Subsequently, large-scale association analysis in the GENOMOS consortium has shown that some polymorphisms in this gene (in particular the Val 1330 variant of the Ala1330Val polymorphism) are associated with lower BMD and with increased fracture risk in some populations . While this gene has opened up research into the Wnt signaling pathway in relation to bone biology, the contribution to population variance in risk for osteoporosis seems modest, and similar to what has been found, for example, for the ESR1 gene in the GENOMOS metaanalysis (see Table 18.4 ). This gene has indeed popped up in large GWAS metaanalyses of GEFOS, indicating that evolution has tolerated polymorphisms to exist in this gene in the population, and which are associated with osteoporosis.
Another example of the mapping of interesting monogenic bone disorder gene is the localization of the van Buchem disease/sclerosteosis gene, which is associated with systemic increased bone formation, to the 17q12–q21 area . We now know this is the SOST gene, and also here some polymorphisms have been found to influence BMD in some populations , and this has later on been confirmed in a much larger dataset in the GEFOS metaanalysis (see later).
Further examples include the gene for absorptive hypercalciuria with bone loss, which is associated with decreased BMD, to 1q24 , and the mapping of one of the Paget’s disease genes and the familial expansile osteolysis gene to 18q21–q22 . The responsible gene in this latter area was shown to be the TNFRSF11A or RANK gene by demonstrating mutations in patients of 4 families, in the signal peptide of this protein, which is essential in osteoclast formation . Indeed, later on, Paget’s disease itself has been subject to subsequent GWAS identifying several responsible loci . All of the responsible genes for these disorders will play a role in bone metabolism and, thus, be of interest to be searched for polymorphisms and analyzed for association with aspects of osteoporosis. Since GWAS is now the leading approach to identify osteoporosis candidate genes, the preferred approach is then to do “lookups” of polymorphisms in these genes and determine their contribution to explaining population variance in BMD and/or other osteoporosis characteristics. Alternatively, if a GWAS locus is identified, and one of these genes responsible for a monogenic disorder is present in this area, this gene becomes a prime suspect to explain the GWAS signal, given its high annotation as a bone metabolism gene .
18.3.1.2
Mouse models
Another prolific source of osteoporosis candidate genes involves animal models in which one or more gene mutations are present giving rise to bone phenotypes. A number of animal models, usually mouse models, have been described that mimic certain aspects of osteoporosis but mainly osteopetrosis . The models can be induced by surgery (ovariectomy), or result from spontaneously arisen mutant strains, or are based on genetically engineered strains such as transgenes or KO models. Characterization of the underlying genetic defects will ultimately result in candidate genes, the human homolog of which can be analyzed in linkage and/or association studies to evaluate the contribution to differences in BMD and/or risk for osteoporosis. Indeed, several examples of such convergence of research approaches have been described.
Several spontaneous mutations have occurred in mouse strains resulting in models of osteopetrosis such as the op/op mouse, which is due to a mutation in the M-CSF1 gene and the osteosclerotic mouse oc/oc . The latter model is of particular interest because the underlying mutation was shown to be a 1.6 kb deletion in the promotor region of the osteoclast-specific vacuolar proton pump ATPase subunit . This genotype–phenotype relation is further supported by knocking out of this gene in −/− Atp6i-deficient mice which also show an osteopetrosis phenotype . Further osteopetrotic or osteosclerotic mouse models that were developed include KO models for the c-src proto-oncogene , the c-fos gene , the NF-kB1 and NF-kB2 genes , the β3 integrin gene , and the cathepsin K gene . The latter human gene does not carry polymorphisms to explain population variance of BMD (as is clear from the lack of it showing up in GWAS metaanalyses), while it has led to the development of novel osteoporosis therapeutic regiments.
Mouse models that mimic osteoporosis are still rather scarce. One exception is a KO-mouse model that was described for the c-Abl gene that leads to an osteoporotic phenotype . One frequently used approach to induce osteoporosis in mice is by ovariectomy but this has so far not been very helpful to identify osteoporosis candidate genes. A set of spontaneous mutant mouse strains that develop osteoporosis are the so-called senescence-accelerated mouse (SAM) strains. Especially, the SAMP6 strain exhibits a lower BMD that is thought to be due to a number of genetic variations . It was therefore used in crosses with high BMD strains with the hope to identify BMD genes in genome searches. Another example of a spontaneous osteoporosis mouse is the autosomal recessive Unhip (Unh) mouse, homozygotes of which develop bone mineralization defects leading to fractures. A genome scan has identified mouse chromosome 14;2 (corresponding to human chromosome 3p14) to harbor the mutated gene .
One of the first genetically engineered mouse models of osteoporosis was based on a transgene with increased expression of interleukin 4 . Intriguingly, analysis of human sib pairs showed linkage of the human IL4 gene (5q31) to differences in serum IgE production , whereas it is known that osteoporosis is a common complication in patients with the hyperIgE syndrome. Another early example of a genetically engineered osteoporosis mouse is the biglycan-deficient KO mouse . Mouse deficient for this extracellular matrix proteoglycan is normal at birth but develops low bone mass which becomes more obvious with age.
By insertional mutagenesis of a novel mouse gene, called “ klotho ” (kl), a mouse model for aging was generated, including the development of osteopenia . Although the accelerated aging phenotype is similar to the SAM mouse models the underlying defects are different. The klotho mouse mutation is a single-gene variation, while the gene shares sequence similarity with β-glucosidase enzymes and has a human homolog on chromosome 13q12 . Mouse models of osteoprotegerin (OPG) have been generated thereby providing strong evidence to implicate this gene in the regulation of bone mass. Whereas OPG −/− mice develop osteoporosis and increased incidence of fractures , transgenic mice overexpressing OPG develop osteopetrosis . This gene has popped up in the GEFOS GWAS metaanalyses as one of the highest ranking genes associated with BMD, while this gene has also led to development of novel osteoporosis therapeutic regiments.
Mouse studies like the ones cited here are valuable because they can give molecular insight in the contribution of one or more genes to certain pathways in bone biology and to determining BMD and/or to risk differences for fracture. Together with the existence of a human disease in which the genes of interest are mutated, the existence of osteoporotic or osteopetrotic mouse models makes the genes involved very likely to be prime candidate human osteoporosis genes. Although they can be supposed to be implicated in determining BMD variation in a population, very few of these have actually shown a strong signal in GWAS. This indicates that they are biologically important, not tolerating much genetic variation (during evolution) compatible with life and/or reproductive success. With the International Mouse Phenotyping Consortium seeking to screen KO models for all genes and across a vast array of phenotypes and the Origins of Bone and Cartilage Disease (OBCD) Consortium high-throughput assessment of skeletal traits , interaction with groups generating the human genetic discoveries is warranted.
18.3.1.3
Zebrafish models
However, testing large numbers of gene candidates in mouse models outside large-scale programs is neither efficient nor cost effective. There are advantages from pursuing high-throughput screening in the zebrafish to systematically assess the function of a large number of potential candidate genes. A growing number of studies suggest that homology between zebrafish and mammals, is deeply conserved . For instance, zebrafish possess the physiochemical (e.g., collagen, hydroxyapatite) and cellular (osteoblasts, osteoclasts, and osteocytes) hallmarks of mammalian bone; they express core genes mediating mammalian osteogenesis and respond to known osteoactive compounds. While the skeletons of zebrafish and mammals differ in several aspects, it has been established that zebrafish and humans share the same mechanism of bone formation and remodeling during development and throughout life. While the skeletons of mammals and fishes differ in several aspects (e.g., lack of hematopoietic marrow, diminished participation in calcium homeostasis and a reduced role in resisting gravitational loading), conditions such as osteoporosis, kyphosis, and muscle weakness are observed even in small fish models . The zebrafish is amenable to CRISPR:Cas9 genetic editing as simple injection into single-cell embryos, leading to efficient generation of mosaic founders with a high percentage of germline transmission . Many genes show similar phenotypic outcomes when disrupted, demonstrating the potential for zebrafish mutants to predict the effect of genes in regulating musculoskeletal abnormalities . Therefore zebrafish models, and perhaps more in general laboratory fish models, hold unique potential to open powerful avenues for skeletal research that are challenging in other vertebrate systems.
18.3.1.4
Humans or animal models
Several of the approaches discussed previously have also been attempted in mice as a model for humans. Especially, transgenic and KO mice have provided very interesting clues regarding bone biology and, thus, have been a source of candidate genes to pursue human studies of genetic variation contributing to risk of osteoporosis. Yet, the obvious drawback of this approach is that humans are not mice and, thus, biology can be very different and that—in the end—we always have to turn to analyzing humans. Indeed, there are examples of KO mouse models that did not result in a clear bone phenotype, whereas the human Mendelian counterpart did result in clear bone phenotypes. For example, the carbonic anhydrase II (CAII) null mice do not show the prominent osteopetrotic phenotype that is seen in human Mendelian CAII mutants . In addition, such approaches in mouse models show us what genes are important in bone biology, but they do not tell us what genes have a relevant functional genetic variation in the human population that contribute to osteoporosis.
In this respect, it could be more informative to analyze many different mouse strains for genetic differences that contribute to variation in bone phenotypes in mice. Or even better, run a GWAS (with dense mouse SNP arrays) on thousands of mice collected in the wild and measured for bone phenotypes. With respect to genome-wide linkage analysis, again, many examples of linkage peaks were reported for the linkage approaches in mice, but—so far no actual genes have been identified. Only, in an elegant but cumbersome combination of approaches, Klein et al. identified the lipoxygenase gene Alox15 as a negative regulator of peak BMD in mice. Crossbreeding experiments with Alox15 KO mice confirmed that 12/15-lipoxygenase plays a role in skeletal development whereas pharmacologic inhibitors of Alox15 improved bone density and strength in two rodent models of osteoporosis. In humans, however, the situation is somewhat unclear which out of the three Alox genes is important in bone metabolism. Ichikawa et al. analyzed some polymorphisms in a human homologous gene, ALOX12 which is in fact not the human homolog of the mouse QTL but a functionally related gene, but found only modest evidence of association with BMD which so far has not been replicated elsewhere while the gene is also not showing up in the GEFOS metaanalyses .
Nevertheless, it is fair to say that mice are used extensively and usefully to study skeletal biology; and while differences between human and mouse bone physiology remain, there are other processes with remarkable homology. Key molecules that regulate cartilage (e.g., Wnt/beta-catenin, Ihh, PTHrP, Sox9, and FGFR3) and bone (e.g., Wnt/beta-catenin, Runx2, FGFR1, osteocalcin, osterix, OPG, RANKL, Triiodothyronine Receptor Auxiliary Protein [TRAP], cathepsin K, and Tumor necrosis factor [TNF]) in mice have the same functions in man, and human genetic disorders causing abnormalities of cartilage and bone are recapitulated in genetically modified mice. Similarly, endocrine and metabolic control of bone and cartilage are faithfully preserved in mice and humans. Such is also the case for zebrafish models. A growing number of studies suggest that genetic homology between zebrafish and mammals is deeply conserved (as discussed previously). However, the use of fish models for bone biomedical research has yet to be widely established.
Taken together and given the amount of effort and time involved in generating animal models, and the substantial progress in knowledge of the human genome and its variation, it remains questionable whether this source of candidate genes coming from mouse and zebrafish models will deliver many novel osteoporosis risk genes of relevance for humans. Rather, imminent progress is expected from animal models now frequently used as follow-up of GWAS findings to test candidate genes in a certain GWAS area for causing skeletal phenotypes. Here, multidisciplinary international collaboration will be the cornerstone to successfully achieve this purpose.
18.3.2
Pre–genome-wide association study genes
Before the advent of GWAS in the field of osteoporosis genetics, a multitude of candidate genes was studied with variable success. It was not until the GENOMOS consortium was erected in 2003 that the most widely studied “classical” osteoporosis candidate genes were put to the test in very large, well-powered prospective metaanalysis.
Later we present a brief overview of the four most widely known candidate genes from that era. See Fig. 18.6A–D for a schematic overview of the gene structure and position of the polymorphisms studied.
18.3.2.1
The vitamin D receptor gene
Association studies
The candidate gene that actually initiated the “molecular genetics of osteoporosis” is the vitamin D receptor gene. Three adjacent restriction fragment length polymorphisms (RFLPs) for BsmI, ApaI, and TaqI, respectively, in intron 8/exon 9 at the 3′ end of the gene, were most frequently studied ( Fig. 18.6A ). Morrison et al. first reported that the BsmI RFLP in the last intron of the VDR gene was related to serum osteocalcin concentration and subsequently to BMD in a twin study and in postmenopausal women . Although the initial observations on the twin study have been withdrawn due to a technical error in the genotyping process , in the following years dozens of papers were published analyzing the same RFLPs in relation to BMD. Some of these confirmed the observation, while others could not find an association or found another allele associated. In the meanwhile the VDR gene has been sequenced in high detail and many polymorphisms have been documented . The GENOMOS metaanalysis ( Table 18.5 ) could not find any relationship between 5 VDR polymorphisms (including the original BsmI, ApaI, and TaqI) and BMD or fracture risk , making it very unlikely that these polymorphisms have any general or universal effect on BMD or fracture risk in humans.
Pleiotropic effects
The vitamin D endocrine system has been shown to be involved in a number of endocrine pathways related to calcium metabolism, immune-modulation, regulation of cell growth and differentiation (of keratinocytes, osteoblasts, cancer cells, T-cells), etc. (for a review, see Ref. ). Thus for a pleiotropic “master” gene such as the VDR one can expect to find associations of this gene with multiple traits and disease phenotypes. Indeed, the VDR gene has been found associated with a number of different phenotypes, but most of these lack the rigor of GENOMOS. GENOMOS has apparently clarified the minimal (if any) role of the VDR gene in the genetics of osteoporosis. Subsequent consortia around these diseases, collecting equally large samples, have to be awaited to evaluate the contribution of VDR polymorphisms to these other phenotypes, as compared to the VDR possible relationship to osteoporosis. In addition, the potential confounding effect, which arises from VDR pleiotropy, can influence the associations observed. For example, VDR gene variants can influence calcium metabolism through differential absorption in the intestine and, at the same time, influence bone turnover, while also influencing the occurrence of osteophytosis, together these effects can result in a net effect on BMD, measured at a certain site, at a certain age and in a subject with a certain diet.
Functional studies
The interpretation of the VDR gene association studies is severely hindered by the fact that most of the studies have used polymorphisms that are “anonymous,” that is, have no known function. The likely explanation for any observed association is then to assume the presence of a truly functional sequence variation elsewhere in the gene which is—to a certain extent—in linkage with an allele of the anonymous polymorphism used. Although the identification of these functional polymorphisms in the VDR gene has been made possible , several investigators have—nevertheless—analyzed multiple bioresponse parameters using the anonymous polymorphisms, including the BsmI, and Bsm–Apa–Taq haplotypes, and a polyA tract in the 3′–untranslated region (UTR). These studies include in vitro cell biological and molecular biological studies, and in vivo measurements of biochemical markers and response to treatments with vitamin D, calcium, and even hormone replacement therapy or bisphosphonates. In view of what has been discussed previously, it is not very surprising that these studies have not shown one allele being consistently associated with all of the different parameters. Major caveats of these cell biological studies are (1) the use of the anonymous rather than functional polymorphisms to group subjects and cells by genotype and (2) the use of different types of bioresponses and different cell types and cell culture conditions in which the vitamin D response might not be evident.
Taken together, all these data indicate that multiple polymorphic variations exist in the VDR gene which could each have different types of consequences. Thus 5′-promotor variations will affect mRNA expression patterns and levels, while 3′-UTR sequence variations will affect the mRNA stability. In combination, these genotypic differences are likely to affect the VDR protein levels and/or function, depending on the cell type, developmental stage, and activation status. Thus the phenotypic variability as observed in action of the vitamin D endocrine system is likely to involve not only the VDR but also other proteins. Hence, polymorphic variations in the genes encoding such proteins will also contribute to genotype–phenotype relationships concerning the VDR genotype associations and might also contribute to heterogeneity between studies.
In summary, although VDR gene variants have been reported to influence biological endpoints, it must be noted that none of the other associated diseases/phenotypes have undergone the scrutiny that osteoporosis/BMD has seen with the GENOMOS consortium. We must therefore be very cautious in claiming any biological phenotype being associated with VDR variants. Testing truly functional sequence variants that matter, establishing the phase of alleles across the entire VDR gene in different populations and defining haplotype patterns, is therefore required to better understand these VDR gene associations. The GWAS results so far have not seen the VDR gene to pop up, indicating that the genetic effect of this gene is very small compared to all the GWAS top hits for BMD.
18.3.2.2
The collagen type I α 1 gene
Association studies
Mutations in the genes encoding collagen type Iα1 and collagen type Iα2 cause the Mendelian disease OI Thus these genes have been early on considered as candidate genes for osteoporosis. While no frequent allelic variants could be found in the coding region of these genes , Grant et al. found a G to T substitution in intron 1 of the COLIA1 gene at a potential binding site for the Sp1 transcription factor ( Fig. 18.6B ). They observed the binding site indeed to bind the Sp1 transcription factor and the “T” allele to have a population frequency of about 18% making this a polymorphism of potential functional significance. In an analysis of 205 predominantly postmenopausal British women, they reported decreased BMD for carriers of the T-allele and an increased fracture risk. In a larger cohort of 1778 Dutch Caucasian elderly women the associations of the T-allele with decreased BMD and increased fracture risk could be confirmed with evidence for a gene dose effect .
Also, this COLIA1 Sp1 polymorphism has undergone the scrutiny of the GENOMOS metaanalysis ( Table 18.5 ) and a 0.15 SD reduction in BMD was observed but only for the TT homozygotes. While no association with overall fracture risk was observed, there was a trend toward a 10% increase in vertebral fracture risk per T-allele resulting in a 33% increased risk in TT homozygotes. So, there is some confirmation in the genetic effect of this polymorphism, but the effect seems limited to the TT homozygotes following a recessive model, and much smaller than originally observed. In the much larger GEFOS GWAS metaanalyses this gene has not been identified to contribute to explain population variance in BMD and/or fracture risk at genome-wide significant levels (see later).
Pleiotropic effects
Considering that collagen I alpha 1 is the major collagen of skin, tendon, and bone tissue, it is expected that the disorders resulting from alterations in this gene are confined to these tissues. This has been documented for several monogenic disorders, for example, Caffey disease (a lethal form of prenatal cortical hyperostosis), several forms of OI, and Ehlers–Danlos syndrome, while COLIA1 mutations are also associated with dermatofibrosarcoma protuberans, a particular type of skin cancer. Whether polymorphisms in this gene contribute to other phenotypes remains to be evaluated in consortia around these diseases, using the same rigor as GENOMOS/GEFOS.
Functional studies
There is evidence to suggest that the COLIA1 “T” allele has direct biological effects and is functional. The first report on the polymorphism demonstrated the putative Sp1 binding site containing the G to T polymorphism to bind the Sp1 transcription factor protein . Subsequent preliminary reports suggested the “T” allele to bind the Sp1 protein twofold stronger and to be associated with a threefold higher COLIα1 mRNA and protein level . In cultured osteoblasts such differences lead to altered COLIα1/COLIα2 protein ratios, very similar to what is seen for null mutations (allelic “KOs”) in OI patients but to a much milder extent. On the basis of these so-called null-mutations in OI patients, it can be speculated that an increased proportion of the COLIA1 homotrimer, such as could be the case in GT and TT subjects, would lead to a more fragile bone. This notion is strongly supported by the observation that the “T” allele was found associated with decreased bone strength in that the yield strength of bone taken from the femoral neck was about half in “GT (guanine-thymine)” heterozygotes compared to that of “GG (guanine-guanine)” homozygotes . This explanation of the COLIA1 Sp1 genotype effect is further supported by what is seen in the oim/oim mouse. In this naturally occurring mutant mouse strain a COLIA1 homotrimer is produced due to a nonsense mutation in the COLIA2 gene. The phenotype of homozygous oim mice includes skeletal fractures, generalized osteopenia and small body size , aspects of osteoporosis which are also observed in human TT homozygotes.
Thus, in summary, this polymorphism does not seem to be associated with appreciable effects on BMD as is clear from the GENOMOS analysis . For any effect on fracture, this does also not seem to be the case as is clear from the GWAS on fracture . If the Sp1 sequence variation is the only frequent functional polymorphism in this gene still remains to be established. The GWAS results so far have not seen the COLIA1 gene to pop up, indicating that the genetic effect of this gene is very small compared to all the GWAS top hits for BMD and/or is not adequately tagged/imputed by the polymorphisms contained by the GWAS platforms.
18.3.2.3
The estrogen receptor alpha gene
Association studies
The estrogen receptor type 1 gene ( ESR1 ) maps to 6q25.1. ESR1 ( Fig. 18.6C ) is a very large gene, spanning 473 kb, and is composed of eight coding exons and nine noncoding 5′-UTR exons (A, B, C, D, E1, E2, F, T1, and T2). Historically, ESR1 has been a strong candidate for genetic regulation of bone mass. Three main polymorphisms have been thoroughly studied in many candidate gene studies, including a TA microsatellite repeat in the promoter region, the PvuII (IVS2–397T>C), and XbaI (IVS2–351A>G) RFLPs. In the prospective large-scale individual-level metaanalysis of the GENOMOS consortium involving 18,917 individuals of European background ( Table 18.5 ), none of these polymorphisms were associated with BMD variation, but the PvuII and XbaI polymorphisms did show a significant association with a vertebral fracture that was independent of BMD . The first GWAS postulating variants in the ESR1 locus as associated at genome-wide significant level with BMD variation in humans was performed in Icelandic individuals with replication on other North-western European populations . This study showed signals mapping to the neighboring gene C6orf97 , while this and other independent variants (not in LD) mapping within ESR1 were subsequently replicated in larger GWAS (see later).
Pleiotropic effects
The estrogen receptor is essential for sexual development and reproductive function. In addition to the role on bone tissue described here, the estrogen receptor is also involved in pathological processes affecting other tissues, such as breast cancer, endometrial cancer, and cardiovascular disease, Interestingly, the ESR1 locus not only displays multiple signals (allelic heterogeneity) for BMD but also for other phenotypes assessed by GWAS, including breast cancer risk and body height .
Functional studies
The estrogen receptor protein is ubiquitously expressed, for which several splice variants exist, differing primarily in their 5′-UTR. These splice variants form dimers with the “wild-type” form and can affect the function of the receptor. Estrogen receptor isoforms are expressed in osteoblasts, osteoclasts, and bone marrow stromal cells as well as in many other tissues.
18.3.2.4
The low-density lipoprotein receptor-related protein 5–like (LRP5) gene
Association studies
The low-density lipoprotein receptor-related protein 5–like ( LRP5 ) gene is located on chromosome 11q13.2 spanning 137 kb and composed of 23 exons ( Fig. 18.6D ). The gene has been known to play an important role in bone biology already for decades. Its role as a critical regulator of bone mass was first established by linkage studies in monogenetic family studies (see Section 18.3.1.1 ). Even though several early candidate gene association studies showed that common variants in the LRP5 gene underlie variation of BMD in the general population , it was only within (the largest study run to date from) the GENOMOS consortium including ~45,000 individuals that two nonsynonymous coding variants (Exon9 Val667Met and Exon 18 Ala1330Val) were robustly associated with BMD, at a level of significance surpassing the current stringent standards of genome-wide level ( Table 18.5 ). An association with the risk of fracture was also observed in that study and has been recently confirmed in the GEFOS consortium (see later).
Pleiotropic effects
LRP5 was originally cloned on basis of its association with type 1 diabetes in humans, while mutations in this gene have also been shown to cause familial eye disorders (exudative vitreoretinopathy), on top of the described skeletal syndromes.
Functional studies
Many common LRP5 variants have been studied in association studies, but the most likely functional candidates are a valine to methionine variant in exon 9 at codon 667 and the alanine to valine substitution at position 1330 in exon 18 described previously ( Fig. 18.6D ). These variants are also precisely tagged by the GWAS signals mapping to this locus. LRP5 protein binds and internalizes ligands in the process of receptor-mediated endocytosis. Within the Wnt pathway, it serves as coreceptor together with the Frizzled (receptor) transducing canonical signals. The LRP5 promoter contains RUNX2, KLF15, and SP1 binding sites and the gene is expressed in osteoblasts. LRP5 is also a target for the inhibitory effects of Dickkopf ( DKK1 ). In mouse models the gene has been shown to affect bone mass accrual during growth. Homozygous mutants show variable bone loss, decreased osteoblast proliferation, impaired glucose tolerance, hyperlipidemia on high-fat diet, and persistent embryonic eye vascularization
18.3.2.5
Other genes
Although the VDR , COLIA1 , and LRP5–6 polymorphisms have received the greatest attention so far, polymorphisms in several other candidate genes have also been studied. Mostly anonymous polymorphisms were studied in genes including steroid receptor genes, cytokine genes, bone matrix proteins, and more exotic osteoporosis candidate genes such as the Apolipoprotein E gene and the HLA gene complex. Although some of these found associations with low BMD, increased fracture risk, or other skeletal phenotypes, the associations were never replicated in additional, preferably larger populations such as in GENOMOS, to undergo the same scrutiny as applied for the VDR , COLIA1 , and LRP5 gene polymorphisms. In addition, identification of functional polymorphisms and description of the LD and haplotypes across the gene can clarify which SNP(s) contribute in what way to a particular phenotypic endpoint of interest. Yet, it is also clear that the candidate gene approach as such will not be very effective even to identify the strongest genetic risk factors, the “low hanging fruit.” For that, we have applied the GWAS approach within GEFOS and the UK Biobank, where now hundreds of such common variants have been identified.
18.3.3
Genome-wide association studies in osteoporosis
18.3.3.1
Timeline of discoveries
As in other human complex diseases, the studies on the genetics of osteoporosis have entered a new era of discoveries with the advent of GWASs . GWASs were made possible by the knowledge provided by the HapMap project (on the organization of common variation in the genome) and the introduction of high-throughput genotyping technology (allowing assessing hundreds of thousands of DNA polymorphisms in adequately powered settings, including tens of thousands to millions of individuals). Before the GWAS era, the literature about the genetics of osteoporosis and fracture had been confined to a very large number of “genome-wide linkage” and “candidate gene association” studies. In retrospect, and with few exceptions, the majority were small, inadequately powered studies generating controversial and frequently nonreproducible reports.
In an effort to summarize all possible candidate gene studies within the framework of GEFOS, variants in about 150 candidate gene regions for osteoporosis according to HuGeNet were tested in the GEFOS-I dataset of ~19,000 subjects with data on osteoporosis and with GWAS genotypes . Within the candidate gene areas, dozens to hundreds of polymorphisms were tested for association using a Bonferroni multiple testing threshold. Although a few polymorphisms were indeed identified as being associated with either BMD or fracture (such as for LRP5 ) none of them reached P <5×10 −8 , the current standard for declaring genome-wide significance. This indicates that the genetic effects of these candidate genes are (much) smaller than the genome-wide significant loci identified by the hypothesis-free GWAS approach.
A historic timeline of discoveries from the GWAS on osteoporosis is presented in Fig. 18.7 and discussed later in more detail. The first GWAS in the field of osteoporosis was published by Kiel et al. . The limited sample size ( n =1141) and sparse SNP content (100k) of the study resulted in no loci found associated at a genome-wide significant level. In Richards et al. , variants in LRP5 and TNFRSF11B ( OPG ) were reported as associated with lumbar spine and femoral neck BMD in 8557 UK and Dutch individuals. Almost simultaneously, Styrkarsdottir et al. published a report on 5861 Icelandic individuals, with replication in an additional 7925 European individuals , which identified variants mapping also to TNFRSF11B ( OPG ), together with additional ones mapping to the TNFSF11 ( RANKL ), ESR1 , ZBTB40 , and the MHC loci. A subsequent report from this group published in early 2009 was based on an extended set including 6865 Icelandic individuals, with replication in other 8510 European individuals . They identified variants mapping to TNFRSF11A ( RANK ), SOST , MARK3 , and SP7 ( osterix ). Shortly afterward, variants in the osterix gene were also identified by Timpson et al. in an effort based on 1518 UK children, followed by replication in adults, including an “extremes truncate selection” of 132 Australian individuals with high or low BMD, and in 3692 individuals of European descent . During mid-2009, Cho et al. published a study examining ultrasound of the radius, tibia, and the heel in 8842 Korean individuals (with replication in additional 7861 individuals) postulating FAM3C and SFRP4 as new BMD loci . Although speed of sound (SOS) ultrasound does not directly measure BMD, it is associated with fracture risk. Notably, it was estimated BMD (eBMD) from heel ultrasound as the phenotype used in the UK Biobank to screen bone health in close to 500,000 individuals (described later). At the end of 2009 the GEFOS consortium reported the first large-scale metaanalysis of most GWAS done by that time involving a discovery dataset of ~20,000 GWAS samples resulting in a large leap in the discoveries as described in Rivadeneira et al. . Within the collaborative setting of the GEFOS consortium , variants were identified in 13 additional loci (on top of the seven already known at the time) in individuals of Northern European origin. These 13 loci reached genome-wide significance for the first time and included WLS (formerly known as GPR177 ), SPTBN1 , CTNNB1 (β-catenin) , MEPE/SPP1/IBSP , MEF2C , STARD3NL , SHFM1 , LRP4 , SOX6 , DCDC5 , FOXC2 , CRGR1 , and HDAC5 . After that, two different GWAS of BMD, using extreme-ascertainment designs based on 800 Chinese and on 1955 Australian individuals, provided evidence for additional loci after replication in nearly 20,000 individuals (18,898 and 20,898, respectively). In the first study, Kung et al. identified variants in JAG1 associated at a genome-wide significant level of P <5×10 −8 . In the second study, Duncan et al. identified genome-wide significant variants mapping to GALNT3 and RSPO3 , while variants in SOX4, LTBP3 , and CLNC7 were suggestive of association.
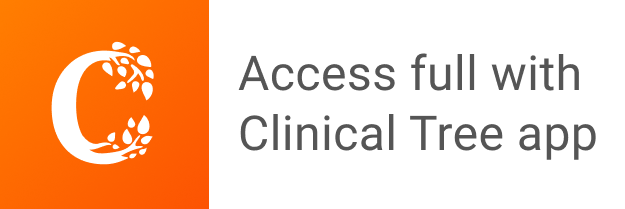