Accurately quantifying the frequency of disease is important for measuring the scope of the problem (i.e., how many people are affected by the disease) and for allowing comparison between different groups (i.e., those with and without a particular risk factor of interest). The most commonly used measures of disease frequency are prevalence and incidence.
PREVALENCE
Prevalence is defined as the proportion of people with disease at a given point in time (e.g., the proportion of hospitalized patients who have an HAI). This may also be referred to as the “point prevalence.”

Prevalence, which is a proportion and has no units, depends on both the incidence (i.e., the number of new episodes that develop) and the disease duration (i.e., how long a disease lasts once it has developed). The greater the incidence and duration of disease, the higher the resultant prevalence. Prevalence is useful for measuring the burden of disease (i.e., the overall proportion of persons affected by the disease). Because all populations are dynamic, the prevalence may vary depending on when it is measured. If a dynamic population is at steady state (i.e., cases leaving are equal to cases entering), the prevalence will be constant over time. Surveillance systems in Western European countries have more commonly measured the prevalence rather than the incidence of HAIs at the country and hospital levels (see
Chapter 6).
INCIDENCE
Incidence is defined as the number of new episodes of disease occurring in a specified period of time. Incidence may be described in several ways. Cumulative incidence is defined as the number of new episodes of disease in a particular time period divided by the total number of disease-free individuals at risk of the disease at the beginning of the time period (e.g., the proportion of patients who develop an HAI during hospitalization).
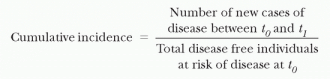
The cumulative incidence, like prevalence, is a proportion and thus has no units. To calculate the cumulative incidence, one must have a complete follow-up on all individuals so that their final disposition with regard to the outcome is known.
Although this measure describes the total proportion of new episodes occurring in a time period, it does not describe when in the time period they occurred. For the cumulative incidence of HAIs, the period implied is the beginning of hospitalization until a first event or until discharge. However, patients do not stay in the hospital and remain at risk for exactly the same period of time. Thus, comparing the cumulative incidence of HAI among patient groups with differing lengths of stay may be very misleading.
The incidence rate (or incidence density) is defined as the number of new episodes of disease in a specified quantity of person-time of observation among individuals at risk (e.g., the number of HAIs per 1,000 hospital days).
The value of this measure can be seen when comparing infection rates in groups that differ in their time at risk (e.g., short-stay patients vs. long-stay patients). When the time at risk in one group is much longer than in another, the incidence rate is the most convenient way to correct for time. This allows one to separate the effect of time (duration of exposure) from the effect of daily risk. Incidence rate is usually restricted to first events (e.g., the first episode of HAI). It is standard to consider only first events because second events are not statistically independent from first events in the same individuals.
Unlike cumulative incidence, the incidence rate does not assume a complete follow-up of subjects. However, even when the follow-up is complete (and thus cumulative incidence could be calculated), reporting the incidence rate may still be preferable. Cumulative incidence reports only the overall number of new episodes occurring during the period (regardless of whether they occur early or late in the time period). By comparison, the incidence rate, by incorporating the time at risk, accounts for potential difference in time to occurrence of the event.
The assumption in the incidence rate is that all time at risk is equal (e.g., the likelihood of developing an HAI in the first 3 days after hospital admission is the same as the likelihood of developing an infection during days four through six of hospitalization). If all time periods are not equivalent, the incidence rate may be misleading. The Centers for Disease Control and Prevention’s (CDC’s) National Healthcare Safety Network (NHSN) predominantly uses incidence rate calculations rather than prevalence rates (see
Chapter 5).