Abstract
Diagnosing monogenic forms of diabetes provides an opportunity to deliver personalized genetic medicine. The most common monogenic causes of diabetes can be treated with therapeutic options targeted to genetic type that are cheaper and less burdensome than standard diabetes care, improving quality of life and potentially providing cost savings for individual patients. However, the most common forms of monogenic diabetes are rare and have a similar clinical presentation as type 1 and type 2 diabetes. As a result, identifying patients with monogenic diabetes in the population will require testing strategies that acknowledge the population-level costs and health effects of genetic testing. Cost-effectiveness analysis is a health economic method that has shed light on the economic value of routine testing for monogenic diabetes. This chapter reviews recent analyses and remaining questions regarding the cost-effectiveness of testing strategies for monogenic diabetes. Studies of the long-term clinical and economic consequences of monogenic diabetes genetic screening strategies will inform clinical recommendations and coverage decisions in order to translate diabetes genetics knowledge into care that improves health outcomes at a reasonable cost.
Keywords
monogenic diabetes, neonatal diabetes, maturity-onset diabetes of the young (MODY), cost-effectiveness analysis, genetic testing
Cost of diabetes care
Diabetes mellitus is a group of heterogeneous disorders with diverse etiologies, affecting at least 285 million people worldwide. The economic costs are exorbitant, with $376 billion USD spent in 2010, reflecting 12% of health expenditures. In the United States alone, 25.8 million people are affected at an annual cost of $245 billion USD. The high costs of diabetes reflect both the rising costs of routine diabetes care as well as the increasing prevalence of diabetes. In the face of fiscal constraints, the substantial economic burden of diabetes has led to increased scrutiny of new interventions by health care payers. New interventions are systematically subjected to economic evaluations to ensure that the benefits of these interventions are produced at reasonable costs. In this chapter, we will discuss the cost-effectiveness analysis of genetic testing strategies for the diagnosis and classification of monogenic forms of diabetes.
Heterogeneity of diabetes mellitus
To appreciate the role of genetic testing in diabetes, one has to realize that diabetes is not one disease, but many, all characterized by sustained hyperglycemia due to varied underlying pathophysiology. Type 2 diabetes, resulting from a combination of insulin resistance and beta-cell failure and usually driven by obesity, accounts for over 90% of diabetes cases. Five to 10% of diabetes is type 1 diabetes, caused by immune-mediated or idiopathic beta-cell destruction. Other rare causes of diabetes together account for the remaining ∼5% of cases, including monogenic forms of diabetes.
Monogenic diabetes
Monogenic diabetes is due to single gene defects or chromosomal abnormalities that are sufficient to cause diabetes. There are nearly 30 different genes that can cause monogenic diabetes, together accounting for ∼2% of all cases of diabetes. Monogenic diabetes can be divided into two main types: neonatal diabetes and maturity-onset diabetes of the young (MODY), with MODY representing the majority of monogenic diabetes cases. Neonatal diabetes is defined as persistent hyperglycemia requiring treatment, with onset within the first 6 months of life. MODY is classically defined as autosomal dominant, nonketotic, noninsulin dependent diabetes with onset typically before 25 years of age with two to three consecutively affected generations. Patients typically lack significant obesity or metabolic features and do not have pancreatic autoantibodies. Studies show that less than 50% of individuals with a genetic diagnosis of MODY fit the classic description.
Precision medicine in monogenic diabetes
The discovery of monogenic forms of diabetes creates an opportunity for precision medicine. Precision medicine includes the application of genomic information to personalize disease prevention, diagnosis, and treatment in order to improve patient outcomes. Genetic testing to diagnose monogenic diabetes is an example of precision medicine. While monogenic forms of diabetes are rare, the most common genetic causes have specific therapies with proven efficacy that differ from conventional therapy for type 1 and type 2 diabetes. Importantly, these therapies are substantially cheaper and less burdensome than insulin therapy (conventional therapy for type 1 diabetes) and have similar costs as metformin (conventional therapy for type 2 diabetes) with often improved glycemic control, which may translate into lower rates of diabetes complications ( Table 27.1 ). Thus, correct diagnosis of subtypes of monogenic diabetes may be an important source of cost saving at the individual patient level due to genetically driven treatment changes. Diagnosis of monogenic diabetes also aids in identifying at-risk family members for diagnostic or predictive genetic testing, particularly for MODY, which is dominantly inherited.
Genetic subtype | Established first-line therapy | Cost relative to type 1 diabetes | Cost relative to type 2 diabetes |
---|---|---|---|
NEONATAL DIABETES | |||
KCNJ11 mutations | High doses of sulfonylureas | ↓↓↓ | N/A |
ABCC8 mutations | High doses of sulfonylureas | ↓↓↓ | N/A |
MODY | |||
HNF1A mutations | Low doses of sulfonylureas | ↓↓↓ | ↓/→ |
HNF4A mutations | Low doses of sulfonylureas | ↓↓↓ | ↓/→ |
GCK mutations | No pharmacologic therapy | ↓↓↓↓ | ↓↓ |
Neonatal Diabetes
Neonatal diabetes occurs in 1:90,000−260,000 live births. Approximately half of neonatal diabetes cases are transient and half are permanent. An uncertain fraction of transient cases will have diabetes relapse in later life, typically during adolescence or pregnancy. Transient neonatal and relapsed transient neonatal diabetes may be amenable to treatment with sulfonylureas, but the best treatment approach is not clear.
The most common causes of permanent neonatal diabetes are heterozygous activating mutations in the genes KCNJ11 and ABCC8, which encode the Kir6.2 and SUR1 subunits of the ATP-sensitive potassium (K ATP ) channel. Such mutations abrogate the usual hyperglycemia-induced closure of this channel that allows insulin secretion from beta cells. Sulfonylureas can close this channel in an ATP-independent manner, representing a genetically targeted therapy for patients with diabetes due to K ATP channel mutations. The majority of these patients are able to transition from insulin to oral sulfonylureas, which almost always results in decreased frequency of hypoglycemia and overall better glycemic control, including improved glycated hemoglobin.
MODY
There are at least 13 described MODY genes but mutations in just three, HNF1A , HNF4A , and GCK , together account for >90% of all diagnosed MODY. MODY due to GCK mutations results in mild, nonprogressive hyperglycemia where treatment is not needed, except possibly during pregnancy. Studies have shown that discontinuation of pharmacologic therapy does not alter glycated hemoglobin. HNF1A -MODY and HNF4A -MODY typically are sensitive to low doses of sulfonylureas, showing stable or improved glycemic control as compared to insulin therapy.
Considerations in genetic testing for monogenic diabetes
While there are published practice guidelines for utilizing genetic testing in order to diagnose monogenic forms of diabetes, it is estimated that at least 80% of diagnoses are missed. The importance of genetic testing for monogenic diabetes in infants with persistent hyperglycemia seems to be increasingly accepted among neonatologists and pediatricians. However, screening for MODY is not a routine practice for diabetes classification, and genetic testing is generally pursued only in those with classic features of MODY, which may miss more than 50% of all cases. Reasons for this include varying clinician knowledge of monogenic diabetes, frequent medical insurance denial of coverage for genetic testing, and significant clinical overlap between MODY and type 1 and type 2 diabetes. The lack of clinical distinction makes it difficult to identify individuals who are very likely to have monogenic diabetes and would benefit from genetic testing. The latter deserves special consideration in the discussion of cost-effectiveness of genetic testing for monogenic diabetes.
From an individual patient perspective, diagnosing subtypes of monogenic diabetes is cost-saving due to cheaper therapies as described earlier. Schnyder et al. illustrated how identifying MODY affects clinical care on an individual patient basis. A molecular genetic diagnosis of GCK -MODY in a 5-year-old female initially treated with insulin therapy allowed for discontinuation of treatment and decreased clinic visits from quarterly to annually. They evaluated the cost-effectiveness of genetic testing in their patient by comparing the cost of genetic analysis and treatment of GCK -MODY to the “costs for intensive diabetes control and treatment as recommended for type 1 diabetes.” Their analysis showed that 1,140 euros would be saved in the first year and 1,640 euros annually thereafter. Additionally, the cost of genetic testing for relatives was ∼25% of index patient testing costs, and testing in the patient’s mother revealed that she was heterozygous for the same GCK mutation.
However, from the societal perspective, the individual patient benefit must be balanced against the cost of genetic testing in those who do not have monogenic forms of diabetes. The cost of genetic testing in general is rapidly decreasing and is expected to continue to fall as next-generation sequencing approaches increase efficiency. However, commercial costs for testing a single monogenic diabetes gene still approach $1,000 USD. This cost currently prohibits universal diabetes genetic testing to be incorporated at diabetes diagnosis for correct classification. Thus, precision medicine for monogenic diabetes, and particularly for MODY, is hindered by difficulty in identifying appropriate patients for testing. Despite a number of clinical characteristics, family history and biomarkers that are highly suggestive of an underlying MODY diagnosis, there is no singular feature that can infallibly distinguish all affected individuals from type 1 or type 2 diabetes. Testing criteria based on atypical features for type 1 or type 2 diabetes may identify MODY in 10−25% of the tested population. While this percentage is substantial, it still means that genetic testing in up to 90% will be negative, and this cost must be considered against the benefit of targeted therapy for those with monogenic forms of diabetes. Thus, formal cost-effectiveness analysis is needed to understand how to integrate monogenic diabetes diagnosis into clinical practice.
The role of cost-effectiveness analysis in healthcare
Health economic evaluations have become essential for policymakers and clinical leaders because we live in an era where there are limited healthcare resources and an almost limitless and expanding number of potential healthcare interventions. Because of the mismatch of available resources and potential interventions, we require a conceptual framework to determine how we should allocate our resources to improve health. Each proposed health intervention has the expectation to maximize “the years of healthy life gained for its population in return for a given level of investment.” To compare the value of one intervention to another, we need a way to predict and define the benefits produced and the costs incurred by each intervention. We also need to be able to interpret these benefits and costs in a tangible way that takes the aggregate health outcomes of a population into consideration, and not only the individual. Cost-effectiveness analysis is one of the most well-established methods for assessing the economic value of new diagnostic tests and treatments. Cost-effectiveness analysis “shows the tradeoffs involved in choosing among interventions… giv[ing] decision makers in diverse settings − physician’s office, health maintenance organizations…or state or federal programs − important data for making informed judgments about interventions.” The practice of cost-effectiveness analysis can be summarized as follows: the first step is to calculate the mean costs incurred and the mean benefits gained by each intervention. States of health are assigned a value or utility ranging from 0 (death) to 1 (full health). Health benefits are determined using health utilities for given disease states, accounting for changes produced by the intervention to extend life and improve health. Health benefits are typically expressed as life years or most often as quality-adjusted life years (QALYs). QALYs do not only consider the ability of an intervention to extend life but to improve the quality of life. Next, an incremental cost-effectiveness ratio (ICER) is calculated by dividing the difference in the mean costs over the difference in the mean benefits between the new and the old interventions. The ICER represents the additional costs required by the new intervention to produce one extra unit of benefit over that produced by the old intervention. The ICER is then compared with a threshold value that represents the maximum a decision maker is willing to pay for an additional unit of benefit. If the ICER is lower than this threshold value then the new intervention is deemed to be cost-effective. Established guidelines, such as those developed by the Panel on Cost-Effectiveness in Health and Medicine, have helped to bring uniformity to the conduct of cost-effectiveness analysis.
The cost-effectiveness threshold varies among different healthcare systems. In the Unites States, the threshold for cost-effectiveness is typically set at an ICER of $50,000 per QALY. However, it has been noted that higher values ranging from $109,000 to $297,000 may be more reflective of what US society is willing to pay for healthcare interventions routinely. But, when considering the specific context of diabetes, routine processes of care such as intensive glucose control and statin therapy for hyperlipidemia have ICERs of ∼$41,000 and $52,000, respectively. Thus, it is likely that the conventional benchmark of cost-effectiveness of $50,000 should be applied when considering genetic testing in diabetes.
Cost-effectiveness analysis requires a number of assumptions to be made and the validity of results will be determined by how appropriate assumptions are. Varying values of model assumptions to reflect uncertainty through sensitivity analyses allows models to fully examine the quality of various data sources. The common theme of these techniques is that uncertainty regarding model parameters is made explicit. Cost-effectiveness analyses must also determine which perspective to represent, which impacts which benefits and costs must be considered. For example, the time spent by a patient or caregiver to deliver a health intervention is accounted for when cost-effectiveness analysis is performed from the societal perspective, but this “cost” is not considered in analyses from the health care system perspective.
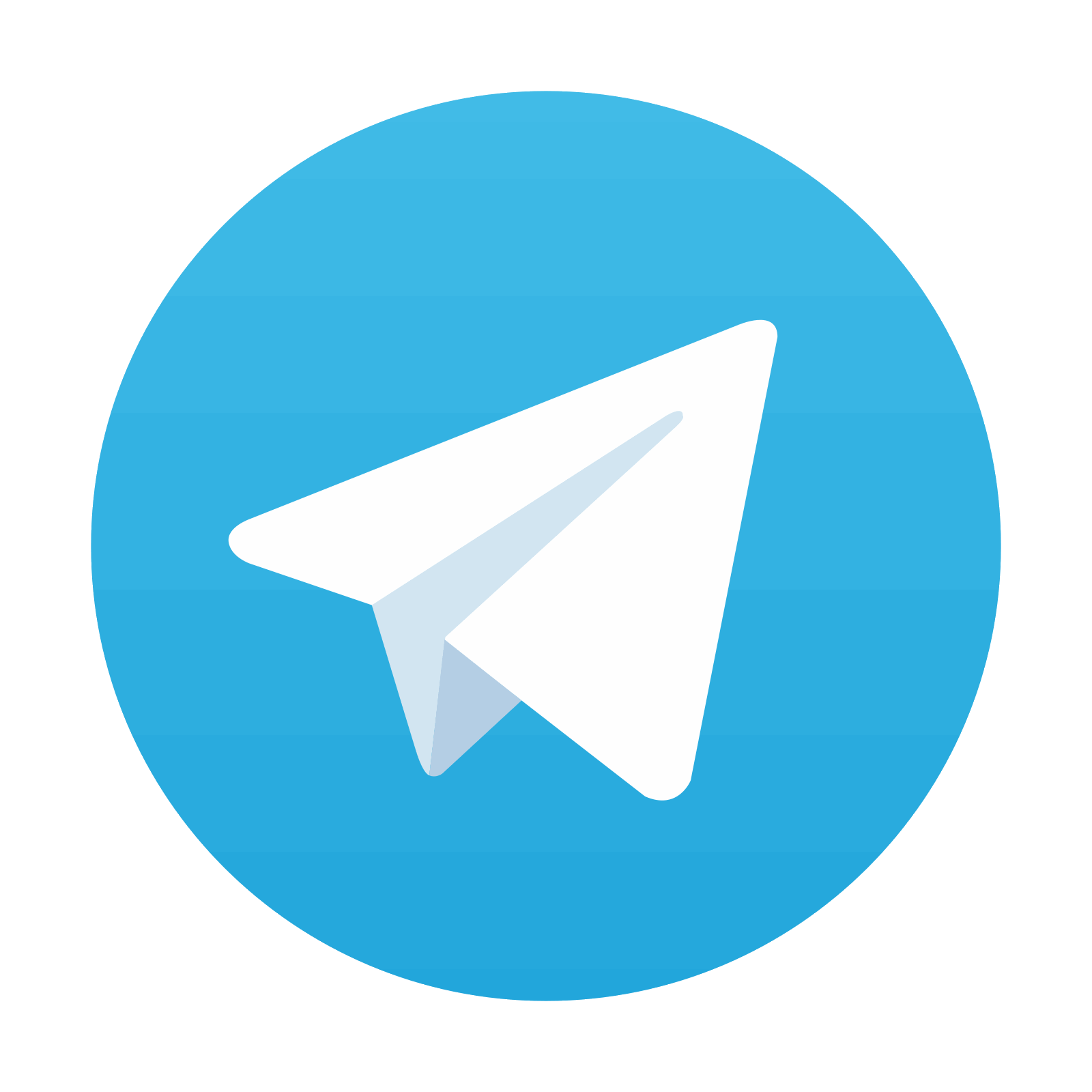
Stay updated, free articles. Join our Telegram channel

Full access? Get Clinical Tree
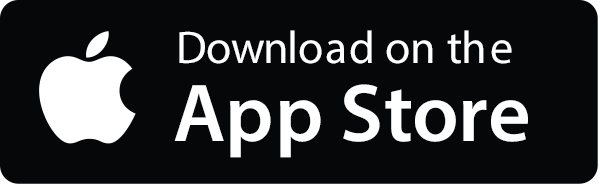
