Breast cancer is a heterogeneous disease associated with variable clinical outcomes and response to therapy. Classic clinicopathologic factors associated with outcome include anatomic features associated with prognosis (eg, tumor size, number of positive regional lymph nodes) and biologic features associated with prognosis and/or predictive of response to specific therapies, usually by evaluating protein expression by immunohistochemistry (eg, estrogen and/or progesterone receptors) or amplification of a single gene (eg, HER2/neu). Gene expression profiling evaluating thousands of genes is now feasible, and has facilitated the development of multiparameter assays that may identify breast cancer subtypes associated with distinct clinical outcomes that were not previously recognized, or provide more accurate information about prognosis or response to specific therapies than may be provided by classic clinicopathologic features alone. Several multiparameter gene expression assays are commercially available, and additional assays are being developed that will facilitate more accurate therapeutic individualization.
Genomics is defined as the study of all of the nucleotide sequences in an organism (see Table 1 for definition and glossary of other terms). Sequencing of the human genome in tumors is technically daunting, but is currently being performed as part of the Human Cancer Genome Atlas project. One report describing an analysis including 11 breast cancers concluded that the genomic landscape of breast cancer is characterized by a handful of commonly mutated gene mountains and a larger number of gene hills that are mutated at a low frequency. In addition to mutation of individual genes, it has recently become apparent that the genomes of breast tumors harbor many more somatic genomic rearrangements than had previously been identified, suggesting that novel fusion genes found at these translocations may also play a role in disease progression. These methods are being used to identify specific genetic changes that may contribute to the pathogenesis of breast cancer and that may be targeted with specific therapeutic interventions, similar to targeting mutated c-KIT with imatinib in gastrointestinal stromal tumor. These methods are not yet available for routine clinical application, however. For the most part, genomic profiling has focused on the evaluation of gene expression, or the translation of the information encoded in genomic DNA into an RNA transcript. RNA transcripts include messenger RNAs (mRNAs), which are translated into proteins, and various other RNAs (eg, transfer RNA, ribosomal RNA, micro RNA, and noncoding RNA) that have important biologic functions. For the most part, gene expression profiling in breast cancer has focused on the evaluating expression of mRNA. However, the same principles may be applied to the study of the epigenome, micro RNAs, proteins, or integrative approaches that evaluate combinations of profiling methods.
Term | Definition |
---|---|
Gene Expression Analysis | |
Genomics | Study of all of the nucleotide sequences, including structural genes, regulatory sequences, and noncoding DNA segments, in the chromosomes of an organism |
DNA microarray | A glass slide or silicon chip with DNA sequences complementary to thousands of genes arrayed at precise locations |
qRT-PCR | Quantitative reverse transcriptase polymerase chain reaction: method used for quantitative RNA expression in RNA extracted from specimens, including degraded RNA extracted from formalin-fixed paraffin-embedded tissues |
Analysis of Gene Expression Data | |
Hierarchical clustering | Commonly used method for performing unsupervised analysis of gene expression data |
PAM or SAM | Prediction analysis of microarray or significance analysis of microarray: commonly used methods to analyze gene expression data |
Centroid | Average gene expression profile defining a classifier |
Regulation of Gene Expression Assays | |
CLIA | Clinical Laboratory Improvement Amendments: regulations that cover approval of diagnostic tests, including multiparameter assays |
IVDMIA | In vitro diagnostic multivariate index assay: term used by the FDA to describe certain types of multiparameter assays that are regulated as medical devices |
510(k) clearance | Regularly approval by the FDA for medical devices characterized as an IVDMIA |
Standards | |
MAQC | Microarray quality control: effort initiated by the FDA to standardize methods for clinical application of microarray and other genomic assays |
REMARK Guidelines | Reporting recommendations for tumor marker prognostic studies: standard criteria for reporting publications about tumor markers, including multiparameter gene expression assays |
MIAME | Minimal information about a microarray experiment: set of standards for release of gene expression ion |
GEO | Genomic Expression Omnibus ( ): publicly available repository for gene expression data |
Interpretation of Published Literature | |
Hazard ratio | Relative risk of an event in a high- versus low-risk population |
Sensitivity | Proportion of actual positives which are correctly identified as such (TP/TP+FN) |
Specificity | Proportion of negatives which are correctly identified (TN/TN+FP) |
Positive predictive value (precision) | Proportion of patients with positive test results who are correctly diagnosed (TP/TP+FP) |
Negative predictive value | Proportion of patients with negative test results who are correctly diagnosed (TN/TN+FN) |
Accuracy | Proportion of true results (positive and negative) in a population (TP+TN/TP+PF+FN+TN) |
Receiver operator curve | Graphical plot of the sensitivity versus (1 − specificity) for binary classifier as its discrimination threshold is varied (fraction of TP versus fraction of FP) |
Substantial technical advances within the past decade have facilitated high-throughput analysis of clinical specimens for gene expression, a process that has been referred to as genomic profiling, although gene expression profiling is the more accurate term. There have likewise been important advances in bioinformatics that permit analysis and interpretation of the huge of amount of data generated by expression profiling. By combining high-throughput specimen evaluation and sophisticated bioinformatics analysis, one can identify distinctive patterns of expression that correlate with clinical behavior or response to specific therapies. Some have referred to these distinctive expression patterns as molecular portraits or signatures, and the assays used to detect these patterns as multiparameter assays; the latter term has been used because rather than relying on expression of a single gene or protein, these assays typically incorporate information from measuring expression of multiple genes by using mathematical algorithms to derive a qualitative (eg, high vs low risk) or quantitative (eg, score) test result. These assays may also be categorized as a tumor marker, a clinical assay that serves as a surrogate for defining clinical end points, such as disease response or progression, or predicting clinical end points, such as prognosis or response to therapy. Although the term tumor marker in the past has usually referred to a substance released from a tumor into the blood or other body fluids (eg, CA27-29, CEA, PSA), it more recently has been defined more broadly to include tissue-derived markers including multiparameter assays.
The promise and pitfalls in developing multiparameter assays have been reviewed elsewhere, and specific criteria have been proposed for the level of evidence required to define and support their clinical usefulness. Several multiparameter assays are currently approved for clinical use, including some which have been recommended by expert panels for clinical decision making. This article focuses on principles of gene expression profiling, and multiparameter assays that have been developed for breast cancer. The term multiparameter assay is used interchangeably with the terms assay, tumor marker, and marker.
Prognostic and predictive markers
A tumor marker is valuable only if it provides information above and beyond that provided by classic clinicopathologic features. A prognostic marker is one that is associated with clinical outcome, usually irrespective of the treatment given. Examples of prognostic markers include tumor size, number of positive lymph nodes, and tumor grade. A predictive marker is one that predicts clinical benefit from a specific therapy. Examples include estrogen receptor (ER) expression (predictive of benefit from endocrine therapy) and HER2/neu overexpression (predictive of benefit from anti-HER2 directed therapies). Some predictive markers are also prognostic, particularly when the therapy predicted to be beneficial is not used (eg, ER, HER2 expression). Predictive markers are more difficult to develop and validate, but are of intrinsically greater value because they are essential in selecting patients for beneficial therapies, more difficult to identify, and fewer.
Process for development of a multiparameter assay
There are several steps in the development of a marker, and a typical roadmap is summarized in Box 1 . Steps included in the process may be broadly classified as (1) conceptualization, (2) clinical development, (3) technical development, (4) validation, and (5) application. For the purpose of maker validation, prospective trials may be performed by retrospectively evaluating samples from completed clinical trials with mature clinical outcomes. Models have been proposed for appropriate strategies to validate markers prospectively in either newly initiated clinical trials, or in completed clinical trials. A critical issue is to ensure that there is sufficient sample size to conduct training and validation studies, and in particular a sufficient number of patients with the clinical event of interest (eg, recurrence). Development of an accurate marker is largely a function of the interplay between sample size and classification difficulty. It is not uncommon to find that several statistically equally good predictors can be developed for any given classification problem. In the postdevelopment process, there is potential for the assay to be less accurate and informative as a result of bias in clinical application of the assay. For example, clinicians may be more apt to use an assay in patients with intermediate clinical features, and not to use it in those with good or poor risk clinical features.
- 1.
Conceptualization: identify clinical need and how marker addresses the clinical need
- •
Identify clinical problem
- •
Identify treatment options and costs of misclassification using standard clinicopathologic criteria, and potential costs of misclassification with standard criteria (eg, over treatment, under treatment)
- •
Identify potential clinical relevance of information provided by marker (prognostic, predictive, or both)
- •
- 2.
Clinical development: marker developed (trained) in an appropriate population, typically referred to as a training set
- •
Population sufficiently homogeneous and receiving uniform treatment
- •
Perform internal validation of classifier to assess whether it seems sufficiently accurate relative to standard prognostic factors that it is worth further development
- •
- 3.
Technical development: establish technical specifications of marker to ensure reproducible performance in clinical samples
- •
Establish reproducibility and reliability of assay
- •
Identify and minimize sources of preanalytic variability that may occur in sample collection and processing in the clinic
- •
Identify and minimize sources of analytical variability in the laboratory that is conducting the assay
- •
- 4.
Validation: validation of marker in other independent data sets in prospectively planned studies
- •
Identify appropriate subject population for marker validation
Population appropriate for prognostic (no treatment or uniform treatment) or predictive assay (2 or more treatment regimens administered with differing therapeutic outcomes)
Sufficient sample size and sufficient number of events of interest
- •
Identify relevant clinical end point
Distant recurrence, local recurrence, organ-specific recurrence, all recurrences
Other clinically relevant end points (eg, specific toxicities)
Death (eg, from primary cancer, other cancers, toxicity, or other causes)
- •
Establish reliability of marker in correlating with clinical end points of interest in different populations
- •
- 5.
Application: establishment or confirmation of clinical usefulness of the assay
- •
Prospective testing of marker in data sets that are independent of data sets used for initial validation
- •
Postmarking experience
Evaluate potential biased used of assay in clinical practice (eg, use preferentially in patients with intermediate-grade tumors)
Evaluate how test information influences clinical decision making
- •
Adapted from Simon R. Roadmap for developing and validating therapeutically relevant genomic classifiers. J Clin Oncol 2005;23:7332.
Process for development of a multiparameter assay
There are several steps in the development of a marker, and a typical roadmap is summarized in Box 1 . Steps included in the process may be broadly classified as (1) conceptualization, (2) clinical development, (3) technical development, (4) validation, and (5) application. For the purpose of maker validation, prospective trials may be performed by retrospectively evaluating samples from completed clinical trials with mature clinical outcomes. Models have been proposed for appropriate strategies to validate markers prospectively in either newly initiated clinical trials, or in completed clinical trials. A critical issue is to ensure that there is sufficient sample size to conduct training and validation studies, and in particular a sufficient number of patients with the clinical event of interest (eg, recurrence). Development of an accurate marker is largely a function of the interplay between sample size and classification difficulty. It is not uncommon to find that several statistically equally good predictors can be developed for any given classification problem. In the postdevelopment process, there is potential for the assay to be less accurate and informative as a result of bias in clinical application of the assay. For example, clinicians may be more apt to use an assay in patients with intermediate clinical features, and not to use it in those with good or poor risk clinical features.
- 1.
Conceptualization: identify clinical need and how marker addresses the clinical need
- •
Identify clinical problem
- •
Identify treatment options and costs of misclassification using standard clinicopathologic criteria, and potential costs of misclassification with standard criteria (eg, over treatment, under treatment)
- •
Identify potential clinical relevance of information provided by marker (prognostic, predictive, or both)
- •
- 2.
Clinical development: marker developed (trained) in an appropriate population, typically referred to as a training set
- •
Population sufficiently homogeneous and receiving uniform treatment
- •
Perform internal validation of classifier to assess whether it seems sufficiently accurate relative to standard prognostic factors that it is worth further development
- •
- 3.
Technical development: establish technical specifications of marker to ensure reproducible performance in clinical samples
- •
Establish reproducibility and reliability of assay
- •
Identify and minimize sources of preanalytic variability that may occur in sample collection and processing in the clinic
- •
Identify and minimize sources of analytical variability in the laboratory that is conducting the assay
- •
- 4.
Validation: validation of marker in other independent data sets in prospectively planned studies
- •
Identify appropriate subject population for marker validation
Population appropriate for prognostic (no treatment or uniform treatment) or predictive assay (2 or more treatment regimens administered with differing therapeutic outcomes)
Sufficient sample size and sufficient number of events of interest
- •
Identify relevant clinical end point
Distant recurrence, local recurrence, organ-specific recurrence, all recurrences
Other clinically relevant end points (eg, specific toxicities)
Death (eg, from primary cancer, other cancers, toxicity, or other causes)
- •
Establish reliability of marker in correlating with clinical end points of interest in different populations
- •
- 5.
Application: establishment or confirmation of clinical usefulness of the assay
- •
Prospective testing of marker in data sets that are independent of data sets used for initial validation
- •
Postmarking experience
Evaluate potential biased used of assay in clinical practice (eg, use preferentially in patients with intermediate-grade tumors)
Evaluate how test information influences clinical decision making
- •
Adapted from Simon R. Roadmap for developing and validating therapeutically relevant genomic classifiers. J Clin Oncol 2005;23:7332.
Methods for analyzing gene expression
There are several methods for analyzing gene expression, which have been reviewed extensively elsewhere and which are illustrated in Fig. 1 and summarized in Table 2 . Irrespective of the analysis platform used, messenger RNA (mRNA) is first extracted from the tissues of interest (see Fig. 1 A–C). Because mRNA is highly vulnerable to degradation, sample handling at this step is critical; surgical specimens should be frozen as soon as possible when used for analysis, or placed in appropriate preservative (eg, RNA Later, Qiagen, Valencia, CA, USA). Following mRNA purification, several platforms exist for gene expression profiling. The first gene expression microarray technology to enter widespread use was the 2-color microarray (see Fig. 1 D). mRNA from 2 samples (an experimental sample and a reference sample) is converted to fluorescently labeled cDNA. Each sample is labeled with a different color (red or green) and the samples are pooled at a 1:1 ratio and hybridized to the same microarray. For all microarrays, each spot on the microarray represents 1 gene and the fluorescence intensity at each spot is proportional to the expression level of that gene in the sample. In a single-color array (see Fig. 1 E), each tumor sample is labeled with the same fluorescent dye and hybridized to its own microarray. For both array types, after removal of nonhybridized material by washing, images are obtained using laser scanning, which detects the relative fluorescent intensity of the hybridized probe at each spot. Before statistical analysis, the data must be normalized (see Fig. 1 H) to compensate for variation in labeling, hybridization, and fluorescent detection, and filtered using specific criteria to reduce the likelihood of detecting noise. In the example shown (see Fig. 1 H), array 3 had a higher average signal intensity (red) than array 1, which, in turn, was higher than array 2. Mathematical correction by normalization results in each array having the same average signal intensity, thereby largely eliminating variation caused by technical issues and allowing detection of biologically relevant differences in gene expression between samples. When many samples are analyzed, it is often convenient to summarize the data in a heatmap (see Fig. 1 I). In this format, the patient samples are typically represented in columns, and genes in rows. Genes that are expressed at levels above the median are colored in red, close to the median in black, and below the median in green. Other color schemes are also commonly used (eg, blue-yellow), which may be especially helpful for individuals with red-green color blindness. By comparing the expression level of a large number of genes in each of the samples, the technique of hierarchical clustering can be used to determine which samples are most similar in gene expression. For example, in Fig. 1 I, the samples from 2 distinct clusters are indicated by the blue and purple branching in the dendrogram at the top of the heatmap. These groups may correspond to samples with different biologic properties (eg, ER-positive vs ER-negative tumors, or high-grade vs low-grade tumors) or groups with different clinical outcomes (see Fig. 1 J).
Method | Description | Advantages | Disadvantages |
---|---|---|---|
Spotted cDNA microarray (eg, Agilent) | Glass slides robotically spotted with purified cDNA clones, PCR products from clones, or oligonucleotides | Ability to design custom arrays | Operator dependent, labor intensive, not always reproducible, requires fresh or frozen tissue |
Photolithography (eg, Affymetrix Gene Chips) | DNA probes directly synthesized on silicon chips | Ability to design custom arrays | Requires frozen tissue or placement in RNA preservative media |
Real-time reverse transcriptase polymerase chain reaction (RT-PCR) | Generate DNA copies of RNA by reverse transcription, amplify DNA by PCR, quantify DNA product using specific fluorescent reagents | May be performed using RNA extracted from paraffin tissue | Requires development and validation of probes, technical limitations in number of genes that may be assayed |
RNA sequencing | Massively parallel sequencing of all mRNAs in a sample | Can detect mutations, splice variants, and fusion genes in addition to changes in gene expression level | Expensive to run the experiments and time consuming to perform the analysis |
Two nonmicroarray-based technologies are also finding applications in this area. In quantitative reverse-transcriptase polymerase chain reaction (qRT-PCR), RNA from the tumor is converted to cDNA and arrayed in different wells of a multiwell plate, each well containing specific PCR primers for a particular gene (see Fig. 1 F). qRT-PCR analysis can then be used to rapidly and accurately quantify the expression level of each gene of interest within the sample. Unlike microarray analysis, which interrogates tens of thousands of genes, the qRT-PCR technology is more appropriate when a limited group of genes is being investigated, although this method may still allow analysis of several hundred genes simultaneously.
The recent advent of high-throughput massively parallel sequencing machines has opened up an entirely new possibility for gene expression profiling, termed RNA sequencing. It is now feasible to profile samples by direct sequencing of cDNAs derived from many millions of RNA transcripts in a given sample. In addition to providing absolute expression levels (as the precise number of transcripts can be counted), this technology also allows the identification of alternatively spliced isoforms, mutations, and novel transcripts arising from fusion genes. In the example shown (see Fig. 1 G), the blue gene is more highly expressed than the red gene, resulting in many more sequencing reads. Furthermore, mutations and fusion genes may be detected using this technology, which is not possible using the microarray platforms or qRT-PCR. In this example, the yellow gene has a mutation that is detected in the sequencing reactions, whereas the other transcript results from the fusion of 2 independent genes (green and purple) and is recognized from sequencing reads spanning the boundary between the 2 genes. Recent studies in breast, prostate, and leukemic cell lines show the potential of this approach.
Quality control of gene expression analysis methods
There are multiple sources of error in clinical application of multiparameter assays, including preanalytical, analytical, and postanalytical. Approval requires meeting specific technical requirements regarding performance, reliability, and reproducibility. The Microarray Quality Control (MAQC) project was organized by the US Food and Drug Administration (FDA) to improve current and next-generation molecular profiling technologies and foster their proper applications in discovery, development, and review of FDA-regulated products ( http://www.fda.gov/ScienceResearch/BioinformaticsTools/MicroarrayQualityControlProject/default.htm ). The effort includes multiple stakeholders, including multiple centers within the FDA and other federal agencies, major providers of microarray platforms and RNA samples, academic laboratories, and others. In MAQC-I, 2 human reference RNA samples were evaluated, and differential gene expression levels between the 2 samples were calibrated with microarrays and other technologies (eg, qRT-PCR). The resulting microarray data sets were used for assessing the precision and cross-platform/laboratory comparability of microarrays, and allowing individual laboratories to more easily identify and correct procedural failures. In MAQC-II, teams developed classifiers for 13 end points from 6 relatively large training data sets, and produced more than 18,000 models that were tested by independent and blinded validation sets generated for MAQC-II. In MAQC-III, also called sequencing quality control, the technical performance of next-generation sequencing platforms is being evaluated by generating benchmark data sets with reference samples and evaluating advantages and limitations of various bioinformatics strategies in RNA and DNA analyses.
Methods for bioinformatics analysis of gene expression
The method by which gene expression data are analyzed depends on the objectives of the analysis, which may be broadly classified as class comparison, class prediction, or class discovery, as described by Simon and colleagues. A description of the statistical analytical methods is beyond the scope of this article, but has been reviewed by others. Class comparison involves determining differences in expression profiles associated with a specific known clinical characteristic (eg, BRCA mutation-associated cancer) or outcome (eg, recurrence or organ-specific recurrence). The primary goal of this type of analysis is to find an informative set of genes and to estimate corresponding population parameters, such as the individual effect of increased expression in each gene on the probability of recurrence. Given the multiplicity of testing at the gene level, gene importance is inferred by ranking all of the genes measured on the array by statistical significance, summarized by the magnitude of the test statistic, corresponding P -value or adjusted P -value. Extending the univariate approach, modeling approaches such as linear or logistic regression may be used to adjust for other genes of interest or known clinical factors such as tumor stage, age, or treatment modality. Model building must take relationships between predictors (included in and omitted from the model) into account to produce precise estimates of effect as well as valid inferences. Statistical association does not confer predictive ability; in classification, for example, a marker exhibiting an odds ratio as high as 3.0 is a poor classification tool. Similar to, but distinct from class comparison, class prediction involves developing a gene expression–based algorithm that accurately predicts group membership of a particular sample. It is well understood that high correlation between predictors in the model does not preclude a good fit; therefore less emphasis is placed on the interpretability of the final model in favor of highly accurate predictions. To this end, the error rate or mean squared error is of primary importance in assessing model performance. Predictive ability is first assessed through internal cross-validation approaches. However, testing the model in independent and more heterogeneous data sets is vital to properly evaluate its true predictive value.
Class comparison and prediction fall into the category of top-down approaches, in which gene expression data from cohorts with known clinical outcomes are compared with genes that are associated with prognosis without any a priori biologic assumption. After this unbiased evaluation, it has become standard to test the subset of important genes from the ranked list or model for enrichment of specific molecular pathways using tools such as Ingenuity IPA (Ingenuity Systems, Redwood City, CA, USA). Alternatively, a bottom-up approach may be used, in which gene expression patterns that are associated with a specific biologic phenotype or deregulated molecular pathway are first identified and then subsequently correlated with the clinical outcome. Class discovery may also be based on unsupervised statistical clustering algorithms such as hierarchical or k-means clustering. An analysis of 4 validated gene expression signatures developed either by the class discovery or bottom-up approach (intrinsic gene set, wound-response signature) or class comparison or top-down approach (70-gene assay, 21-gene assay) showed significant agreement in their outcome predictions for individual patients, suggesting that they are tracking similar biologic phenomena despite being developed by differing methodologies. Although proliferation is the strongest parameter predicting clinical outcome in the ER-positive/HER2-negative subtype and the common denominator of most currently available prognostic gene signatures, immune response and tumor invasion are predominant molecular processes associated with prognosis in the triple negative and HER2-positive subgroups, respectively.
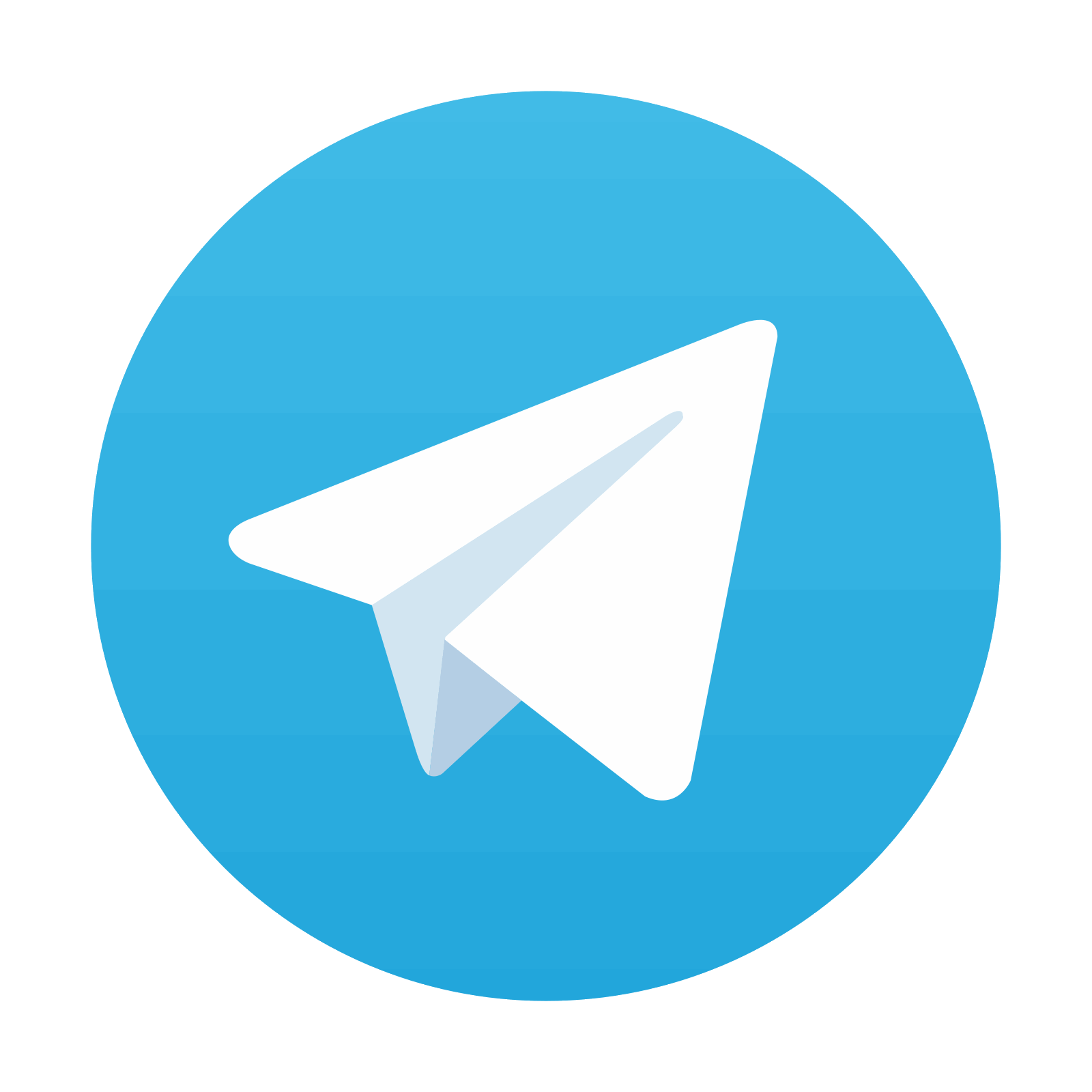
Stay updated, free articles. Join our Telegram channel

Full access? Get Clinical Tree
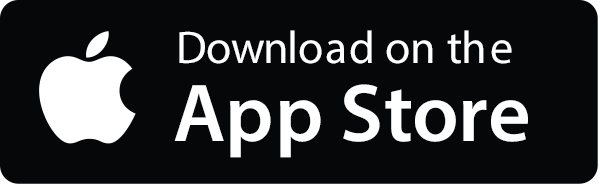
