Biostatistics and Epidemiology: The Basic Science of Clinical Research
Kerry L. Lee
In the basic sciences, there is an essential knowledge base and an array of tools and techniques that basic scientists must master in order to conduct state-of-the art laboratory and animal research in areas such as molecular and cell biology, biochemistry, and genetics, and to research the complex molecular or cellular mechanisms related to hemostasis and thrombosis. Similarly, there are vitally important concepts, scientific methods, and tools that must be understood for conducting clinical research, whether that research is in hemostasis and thrombosis, cancer, cardiovascular disease, or other areas of medicine. For example, to rigorously evaluate a promising new compound for reducing the incidence of thrombotic complications in a specific population of patients, a properly designed study with an adequate number of subjects where potential biases are minimized and the data are properly analyzed is required. The key question is how should you design, conduct, analyze, and interpret such a study. The answer to that important question can be found in the body of knowledge and the concepts, principles, and tools of biostatistics and clinical epidemiology. Indeed, the basic science of clinical research and the quantitative methodology required for conducting well-designed and properly analyzed clinical investigations largely exists within these disciplines. A basic understanding of biostatistical and epidemiologic methods is also essential to understand and critically evaluate the current medical literature in which the designs and results of clinical trials and observational studies are reported and discussed.
This chapter provides an overview of selected biostatistical and epidemiologic methods that are relevant for researchers who wish to design and conduct a clinical study. In addition, this overview is relevant for clinicians and medical practitioners who need to critically evaluate the published medical literature and use that information to make rational, evidence-based decisions in medical practice. An understanding of these methods is also important for properly synthesizing and summarizing information for the development of guidelines and policy decisions designed to improve health outcomes in the general public.
EPIDEMIOLOGY STUDY DESIGNS
The classical definition of epidemiology found in textbooks on the subject is that epidemiology is the study of the distribution and determinants of disease frequency in human populations.1,2,3,4 This definition embodies two principal areas, namely (a) the study of disease frequency and variation in the occurrence of disease and (b) the search for the determinants of the distribution and frequency of disease and reasons for the variation in its occurrence. Relevant to the methods discussed in this chapter is the concept of clinical epidemiology, defined by Weiss5 as “the study of variation in the outcome of illness and of the reasons for that variation.” Numerous textbooks have been written on the subject (see for instance references1,2,3,4,5), and graduate training programs in epidemiology have been developed at many wellknown colleges and universities, particularly in universities with a School of Public Health. Epidemiology, because it is based directly on observations of human subjects, has the advantage of relevance to populations of real people. However, classical epidemiology studies are commonly observational in nature and cannot take place under the controlled conditions possible in a laboratory experiment. Deriving reliable conclusions about cause-and-effect relationships from observational studies is challenging and must be done very cautiously and carefully. Observational epidemiologic studies have the disadvantage that possible confounding factors may bias conclusions about cause-and-effect relationships, and great care must be taken in designing such studies and analyzing the data in order to reduce the likelihood of spurious associations between a disease or disease outcome and a study factor of interest. In the epidemiology literature, the study factor of interest is often referred to as an exposure variable.6
There are two basic types of epidemiologic studies, which with some variations encompass most of the clinical research studies seen in practice today6 These include (a) case-control studies, and (b) cohort studies. In a case-control study, a group of cases or patients who have a disease of interest and a control group, or comparison series of subjects without the disease, are selected for investigation. Of primary interest is a comparison of the proportions in each group with a particular exposure (or factor) of interest. In contrast, in a cohort study, subjects are classified on the basis of the presence or absence of exposure to a particular factor (e.g., a particular treatment), and then followed for a specified period of time to assess the development of the outcome of interest (i.e., development of a particular disease or clinical event of importance) in each exposure group. Both case-control and many cohort studies are often criticized because of the potential for confounding and bias that is inherent in the fact that the design is observational. In a cohort study where the exposure factor of interest (e.g., use of a particular drug or treatment) is either self-selected or chosen by a health care provider, the subjects with that factor (e.g., people who use a particular treatment) may be systematically different from those without the factor (e.g., people who do not use the treatment) in ways that will affect the outcome of interest. In a case-control study, because the outcome of interest has already occurred at the time the exposure is assessed, there is potential for bias in the selection of subjects into the study and in their recall of prior events.
However, there are two principal strengths of observational epidemiologic studies. First, the evaluation of exposure factors that require a long duration before the outcome of interest occurs may be able to be studied productively with one of these designs, particularly a case-control design. Second, if the effect to be detected is moderate to large (on the order of a 50% or greater difference in the disease outcome of interest), then one of these observational designs may suffice. The strong association between smoking and lung cancer observed in the case-control studies conducted by Doll and Hill7 in the 1950s is an example where although the amount of uncontrolled confounding may have affected the magnitude of the relative risk estimate, the relative risk of lung cancer due to smoking was sufficiently large that even with complete control of confounding factors, it is unlikely to have materially changed the conclusion that there is a strong positive association between smoking and lung cancer.6
RANDOMIZED CLINICAL TRIALS
In contrast to case-control and observational cohort studies where the “exposure” factor is observed rather than controlled as part of the experiment, a study design that can be viewed as an important special case of an epidemiologic cohort study is the randomized clinical trial.6 Since the conduct and publication of the earliest randomized trials in the 1940s, randomized clinical trials have increasingly become accepted as the principal and most reliable method (the “gold standard”) for acquiring information required for judging the efficacy and safety of therapeutic interventions. Variations of a “strength of evidence” hierarchy, such as that published in Green and Byar,8 (FIGURE 7.1) always place the information derived from a well-designed randomized clinical trial as having greater value than information from nonrandomized observational studies. Randomized trials are widely accepted today as a powerful tool and the key to providing information required for rational, evidence-based medical care and prevention of disease.6,9 Well-designed clinical trials are required by regulatory agencies prior to the registration of new drugs and devices. Clinical trials serve as the critical bridge between initial studies that have provided “proof of concept” of a new therapy and its ultimate widespread clinical application.
It is a legitimate question to ask why clinical trials are so essential. There are many reasons. First, most new interventions for use in clinical care have modest beneficial effects.10 Relative risk reductions in key clinical outcomes as high as 25% are rarely achieved or exceeded by new interventions, and frequently the benefit is less although still clinically important. Second, few interventions are free of undesirable effects, and both the benefits and risks must be evaluated in order to adequately assess the usefulness of an intervention.9 Given the uncertainties about the disease course and biologic variation in clinical outcomes, it is usually impossible to reliably judge the effects of a treatment or intervention on the basis of uncontrolled clinical observations. The randomized clinical trial has emerged as the principal research tool for developing evidence to inform and influence clinical practice. This is true in studies of hemostasis and thrombosis, as well as in numerous other areas of medicine.
In the past 20 years, many papers and a number of excellent textbooks have been published describing in considerable detail the methodology of randomized clinical trials. The popular text by Friedman et al.9 provides an excellent expository overview of the fundamental concepts of clinical trials and the major principles and components of a well-designed clinical trial. Other useful books include Piantadosi’s11 excellent methodologic perspective on clinical trials, Cook and DeMets’12 introduction to statistical methods in clinical trials, the older, but highly relevant text by Pocock,13 and the recently published four-volume Wiley Encyclopedia of Clinical Trials.14 In this chapter, selected topics that are pertinent to clinical researchers for planning and conducting a well-designed clinical trial and analyzing and interpreting the results of the study are discussed.
What is a Clinical Trial?
The text by Friedman et al.9 defines a clinical trial as “a prospective study comparing the effect and value of interventions(s) against a control in human beings.” The key elements of this definition are that a clinical trial (a) is prospective, rather than retrospective (i.e., subjects are followed forward in time), (b) involves one or more interventions or intervention strategies, (c) requires a control or comparison group against which the interventions are evaluated, and (d) involves human subjects.
The important prospective element of a clinical trial is in sharp contrast to the case-control epidemiologic study design mentioned earlier where participants are selected on the basis of the presence or absence of an event or condition of interest. Thus, by definition, a case-control study is not a clinical trial. Because a clinical trial involves human subjects, it introduces issues of ethics, safety, compliance, and the inherent biologic variation that exists among human subjects.
“Phase” Designation for Clinical Trials
In the medical literature, clinical trials are often characterized as being of a certain “phase.” This terminology generally places clinical trials into four phases (phase I through phase IV).9 Although preclinical data may be obtained from in vitro studies or animal models, phase I studies are generally small studies that represent the initial step or phase of testing a drug or biologic in humans to understand how well it can be tolerated and assess its toxicity. A typical goal of a phase I trial is to estimate how large a dose can be given before unacceptable toxicity is experienced by subjects. Although it does not meet the definition of a clinical trial (there is no control arm), this phase of development for a
new intervention is important and is commonly referred to as a phase I trial, although a “phase I study” is a more appropriate designation.
new intervention is important and is commonly referred to as a phase I trial, although a “phase I study” is a more appropriate designation.
A phase II trial is one in which the objective is to assess the biologic activity or effect of a new compound, acquire further information regarding the appropriate level of dosing, determine whether there are encouraging signals (typically on surrogate endpoints [discussed later in the chapter]), and obtain data for designing a larger, more definitive (phase III) study that would be required to test the effectiveness of a new therapy in order to obtain regulatory approval. Finally, phase IV studies, generally referred to as postmarketing surveillance studies, are longer-term surveillance studies, designed to obtain additional information on long-term safety issues that may be associated with adoption of a new intervention.
Efficacy versus Effectiveness Trials
The terminology “efficacy” and “effectiveness” is often used in the context of clinical trials.9 An efficacy trial is meant to distinguish clinical trials designed to assess the effects of an intervention in an ideal setting (where subjects and health care providers are highly compliant with the protocol, the best trained and most experienced center(s) are involved, and perhaps the study population and spectrum of risk of the population are narrowly defined). In contrast, an effectiveness trial is one in which the effects of an intervention are assessed in a study population enrolled across different types of clinical centers, taking into account the realities of less-than-perfect compliance with the study protocol, and typically involving a broader spectrum of patient risk. Most moderate to large multicenter trials would be considered “effectiveness” trials. The terms “comparative effectiveness trials” and “pragmatic trials” have recently become quite popular, but both terms relate to the general class of studies referred to as “effectiveness” trials.
Study Questions and Endpoints
The planning of a clinical trial depends on the specific objectives and the questions that the investigators wish to address. It is essential that those objectives and questions be well focused, clearly stated, and specified in advance. Closely related to the specific aims of the study and the questions of interest is the choice of endpoints to be examined in a trial. As advocated by Friedman et al.,9 each clinical trial should have a primary endpoint. This choice is a key decision in designing a trial and is usually the major determinant of the study size. The primary endpoint should be (a) consistent with the primary study question and the hypothesized effect of the intervention, (b) clearly defined and specified in advance, (c) capable of being ascertained as completely as possible (ideally in every subject), (d) measured in the same way for all subjects, and (e) capable of unbiased assessment. For nonfatal endpoints, these last two points may require that the study have a blinded core laboratory to make the endpoint measurements or a blinded clinical events committee to review and adjudicate the clinical endpoints.
Beyond the important choice of the primary endpoint, it is reasonable in a clinical trial to consider one or more secondary endpoints in order to address important secondary or subsidiary questions. Again, prespecification and prioritization of these endpoints is important.
There are a variety of types of endpoint variables, including (a) binary (e.g., developing the disease of interest; whether the patient lived or died), (b) ordinal (e.g., worsening, no change, or improvement; number of significantly diseased arteries), (c) continuous (e.g., cholesterol level; lymphocyte count; distance walked in a 6-minute walk test), and (d) time to event (with censoring) (e.g., time to death or to significant nonfatal events [e.g., stroke] in long-term follow-up studies). Time-to-event endpoints typically arise in studies where patients are followed over a relatively long period of time.
Composite Endpoints
For many therapies under investigation, the goal is not necessarily to reduce mortality, but rather to prevent nonfatal events or improve symptoms. In such cases, a variety of negative outcomes are important (e.g., nonfatal myocardial infarction, stroke, or unstable angina). Furthermore, it is important with a new therapy to be relative certain that excess mortality is not being caused in the pursuit of reduced symptoms or nonfatal events. An approach frequently used is to specify a composite endpoint that includes more than one relevant outcome (e.g., death or stroke; death, myocardial infarction, or hospitalization for unstable angina).9,15 This type of composite endpoint has been valuable in a number of studies and has led to acceptance of several new therapies in patients with acute coronary syndromes and heart failure. An advantage of a composite endpoint is that by including multiple possible outcomes where the occurrence of any one of them is counted, the event rate will be increased (compared for example to an endpoint consisting of mortality alone), and therefore the number of patients required for the study will be less. However, the interpretation can be problematic, particularly if some components of the composite are affected differently by the treatment being studied compared to its effect on other components. Furthermore, the common approach to analyzing such composite endpoints counts each component with equal weight, which may be somewhat counterintuitive. Some attempts at developing weighting schemes have been used, but clinicians have a difficult time agreeing on appropriate weights.15,16 If considering a composite endpoint, a useful guideline is to (a) include only components that are expected to be affected by the intervention being studied, (b) include “harder” or more objective measures that are clinically important, (c) include mortality as one component, and (d) limit the number of components to a relatively small number.
Surrogate Endpoints
With clinical events as the primary endpoint, a trial can be quite long and expensive, and therefore consideration is often given to designing trials with a surrogate endpoint, namely a laboratory measurement or physical sign that is used as an alternative to a clinically meaningful endpoint.9,15,17 Surrogate endpoints are of interest for their potential to reduce the size, cost, and duration of major trials. Furthermore, they may be helpful in explaining the mechanism of action of a therapy on clinical endpoints. Examples of surrogate endpoints might be such things as blood pressure, cholesterol reduction, CD-4 lymphocyte level, or suppression of ventricular arrhythmias. Lowering blood pressure in a population of hypertensive patients is an example of a surrogate for the clinical outcome of death, myocardial infarction,
or stroke. Surrogate endpoints are essential for early-phase studies to elucidate pathophysiological effects, but the pitfalls of assuming that a positive effect of an intervention on a surrogate endpoint will translate into a positive effect on a relevant clinical outcome have been well described. The Cardiac Arrhythmia Suppression Trial18 and heart failure trials of inotropic drugs19,20,21,22 are classic examples of the failure of surrogate endpoints.
or stroke. Surrogate endpoints are essential for early-phase studies to elucidate pathophysiological effects, but the pitfalls of assuming that a positive effect of an intervention on a surrogate endpoint will translate into a positive effect on a relevant clinical outcome have been well described. The Cardiac Arrhythmia Suppression Trial18 and heart failure trials of inotropic drugs19,20,21,22 are classic examples of the failure of surrogate endpoints.
There is a common misconception that if an outcome is correlated with the true clinical event, it can be used as a valid surrogate endpoint and serve as a replacement for the true clinical endpoint of interest. A valid surrogate endpoint must fulfill two criteria.17,23 First, changes in the surrogate must be predictive of the relevant clinical outcome. The second important requirement, which can be difficult in practice to ascertain, is that a potential surrogate must fully (or nearly so) capture the effect of the intervention on the true clinical outcome. The considerations that underlie this determination are well described in the excellent article by Fleming and DeMets.17
Choice of Study Population
An important consideration in planning a clinical trial is the choice of the inclusion and exclusion criteria that define the study population.9 The impact of these enrollment criteria on the ability to recruit patients and the ability to generalize the results must be considered. As general principles, eligibility criteria should include patients for whom (a) the intervention is likely to have an effect, (b) there is a high likelihood of detecting the hypothesized effect, (c) the possibility of adverse effects or safety concerns do not outweigh the possible benefits, (d) competing risks are minimal, and (e) adherence is not likely to be a major issue. There are tradeoffs between using broad, less restrictive enrollment criteria versus narrow, more restrictive criteria. With broad criteria, the results will be generalizable to a broader population, there will be a larger pool of potential patients, enrollment will be easier, and protocol violations may be reduced. On the other hand, broader inclusion criteria will generally lead to enrollment of some lower risk patients, which will lead to a lower event rate, and therefore require a larger trial. With a more narrowly defined patient population, the study can be restricted to higher risk patients, leading to a higher event rate and a smaller size trial. The negative aspects of narrow criteria are that the study is less generalizable, there will be a smaller pool of eligible patients, enrollment is typically more complicated, and thus protocol violations may be increased.
Sample Size and Statistical Power
One of the most important aspects of designing a clinical trial is to determine the number of patients that must be enrolled to accomplish the objectives of the study. The all-too-frequently used nonscientific approach for making this determination is to (a) assess the amount of money available for performing the study, (b) determine the amount that each patient will cost, and then divide (a) by (b). The adequacy of the sample size has been a deficiency of clinical trials in the past. A review of the statistical power of 71 published randomized trials that failed to find significant differences between groups revealed that 67 of the trials had a >10% risk of missing a true 25% therapeutic improvement, and with the same risk, 50 of the trials could have missed a 50% improvement.24
In the context of sample size for clinical trials, the basic concepts of statistical power are briefly reviewed. The power of a study is the probability of a positive result (a statistically significant result) if the intervention under study has a real effect on the primary outcome of the trial. Thus power is the probability of a “true positive” study; of correctly detecting true differences. The level of power depends on the magnitude of the effect of the intervention, and on the number of patients enrolled (sample size). The power of a study equals 1 minus the type II error probability, where the type II error probability is the probability of failing to detect a real difference.
With that basic information as background, the preferred (scientific) approach for determining sample size in a clinical study is as follows:
Develop an estimate of the outcome expected in the control arm. This could be an event rate if the endpoint is a clinical outcome or a mean ± standard deviation if the outcome is a continuous measure. Typically, such estimates will be based on pilot data, previous studies, or other sources of relevant information.
Determine the treatment effect to be detected (the effect should be clinically important and realistic).
Select the desired level of power (90% is preferable; 80% may be acceptable for some studies).
Specify the desired level of significance (commonly the level used in clinical studies is a = 0.05).
Calculate the number of patients required.
Formulas exist in numerous textbooks for calculating the required number of patients for a binary endpoint, for a continuous endpoint measure, and for comparing groups with respect to time-to-event endpoints.9,25,26 Commonly available statistical software packages (SAS and others) contain procedures for performing sample size calculations.
For trials with a continuous response, a key parameter that must be specified for sample size and power calculations is the variability of the response (outcome) variable, which is typically characterized in terms of the standard deviation. Greater variability in the outcome variable requires more patients to achieve the same level of power. A continuous outcome also requires specifying a clinically meaningful difference between the mean outcome response in the intervention and control arms that is desired to be able to statistically detect.
For trials with a binary endpoint, the sample size depends on the event rate estimated for the control arm, and the magnitude of the hypothesized benefit from the intervention. Low event rates require a larger sample size for detecting meaningful differences.
For trials with a time-to-event outcome variable, the power is driven by the number of events rather than the total number of enrolled patients. Therefore, the sample size must be selected so that the number of events will be adequate. The sample size calculations involve first determining the number of events needed to achieve the desired level of power, and then based on the projected event rates, the number of patients required can be calculated.27
A type of trial design that has been growing in popularity is what is termed as an “event-driven” trial, meaning that the study will continue until the required number of events has been achieved. If the event rate proves to be less than projected, the study must either enroll more patients or extend the duration
of follow-up. The advantage of such a design is that the study is assured of achieving the required number of events. The main disadvantage is that it is more difficult to plan the exact number of patients and the length of follow-up and hence to develop the overall study budget.
of follow-up. The advantage of such a design is that the study is assured of achieving the required number of events. The main disadvantage is that it is more difficult to plan the exact number of patients and the length of follow-up and hence to develop the overall study budget.
For studies with more complex designs or features such that the standard sample size formulas may not apply directly, computer simulation of the proposed design features can be used to perform sample size and power analysis. This would normally require the help of an experienced statistician.
Useful points to remember in determining an appropriate sample size:
Appropriately account for a realistic amount of patient withdrawals from the study, premature discontinuation of the randomly assigned study treatment, crossover from one arm to another, or other noncompliance with the protocol.
Avoid overly optimistic projections of event rates and treatment benefit.
Remember that large studies are required to reliably detect moderate treatment effects.
Randomization
Although not specifically mentioned as part of the definition of a clinical trial, a critical component of a well-designed trial is the randomization of study participants to the intervention and control arms of the study9
The unique feature and strength of randomization is that it controls, on average, for the effects of potentially confounding factors that are unknown or unmeasured as well as those that are known. It is this feature that makes the randomized trial such a powerful strategy, especially for studying small-to-moderate effects.
Three important contributions of randomization include (a) it eliminates bias in allocating subjects to the different arms of a study, (b) it ensures that there are no systematic differences between treatment groups with respect to known and unknown risk factors, and (c) it provides a valid basis for statistical inference.28 This third advantage is somewhat more subtle, but means that randomization guarantees the validity of the statistical tests of significance that are used to compare the treatments. Simply stated, this means that the process of randomization makes it possible to ascribe a probability distribution to the difference in outcome between treatment groups receiving equally effective treatments and thus to assign “significance levels” to observed differences.28 This is the basis of the P-values reported in randomized clinical trials or any other randomized experiment.
Stratified randomization is often used in clinical trials and simply means that a separate randomization is performed within prespecified strata, defined by selected patient characteristics such as a prognostic factor known to be strongly related to the outcome of interest.9,13 Stratification by clinical center is often used in multicenter clinical trials to ensure that treatments are allocated in similar proportions at each center. Stratification may improve balance among treatments with respect to prognostic factors and emphasize subgroups (or strata) of special interest. Stratification by clinical site is usually desirable, but stratifying by more than one or two key other factors is unnecessary and undesirable.29,30,31
Blocked randomization, also called “permuted block randomization,” is commonly used in clinical trials to avoid any major imbalance or deviation from the desired proportion of patients allocated to each arm. Blocked randomization guarantees that any deviation will never be large, and at certain points the numbers assigned to each group will reflect exactly the correct proportions.9,13 Blocked randomization is especially desirable if the mix or level of risk of patients enrolled might change over time.
Consideration may be given in a clinical trial to equal versus unequal allocation of patients to the different arms of the trial.13 There are several points to consider in this matter. Equal allocation will maximize the statistical power of the treatment comparisons for a given number of patients. Equal allocation is more consistent with equipoise (indifference) toward which group a patient is assigned. Unequal allocation (allocating more patients to the experimental intervention) (a) allows obtaining more information and experience with a new therapy with respect to side effects or toxicities, (b) could reduce cost if the comparator (control) therapy is expensive, (c) does not substantially decrease efficiency (i.e., decrease statistical power) as long as the proportions allocated to each treatment arm are not too different (e.g., not more extreme than three to one),13 and (d) may have the effect of causing participants to think that one arm of the study is preferred or more desirable than other arms.
The term adaptive randomization may be encountered in the clinical trials literature. There are various schemes that have been proposed for adaptive randomization, for example, adapting the allocation ratio or making other adaptations during the course of the trial. These schemes are discussed extensively in the textbook by Pocock.13
For the practical implementation of a randomization scheme, a commonly used approach, particularly in phase III trials, is to employ a centralized interactive voice response system where a telephone call center can be used to verify patient eligibility and provide the treatment assignment. Internet-based systems for randomization are also growing in use. The older, simple approach of an envelope system is still used in some smaller studies, but is subject to misuse (either intentional or unintentional) such as prematurely opening the envelope containing the randomization assignment before eligibility is fully ascertained or informed consent has been obtained. Selecting envelopes out of sequence is also a potential limitation. Although it is inexpensive, this approach to randomization is generally discouraged.
One important point in planning and conducting a clinical trial is that it is critical that the treatment intervention begin as soon as possible after randomization in order to avoid bias that can arise from events occurring between randomization and initiation of the assigned therapy. Thus whenever possible, it is desirable to delay randomization until the last practicable time before initiation of therapy so that there is not a major delay between randomization and the start of therapy.
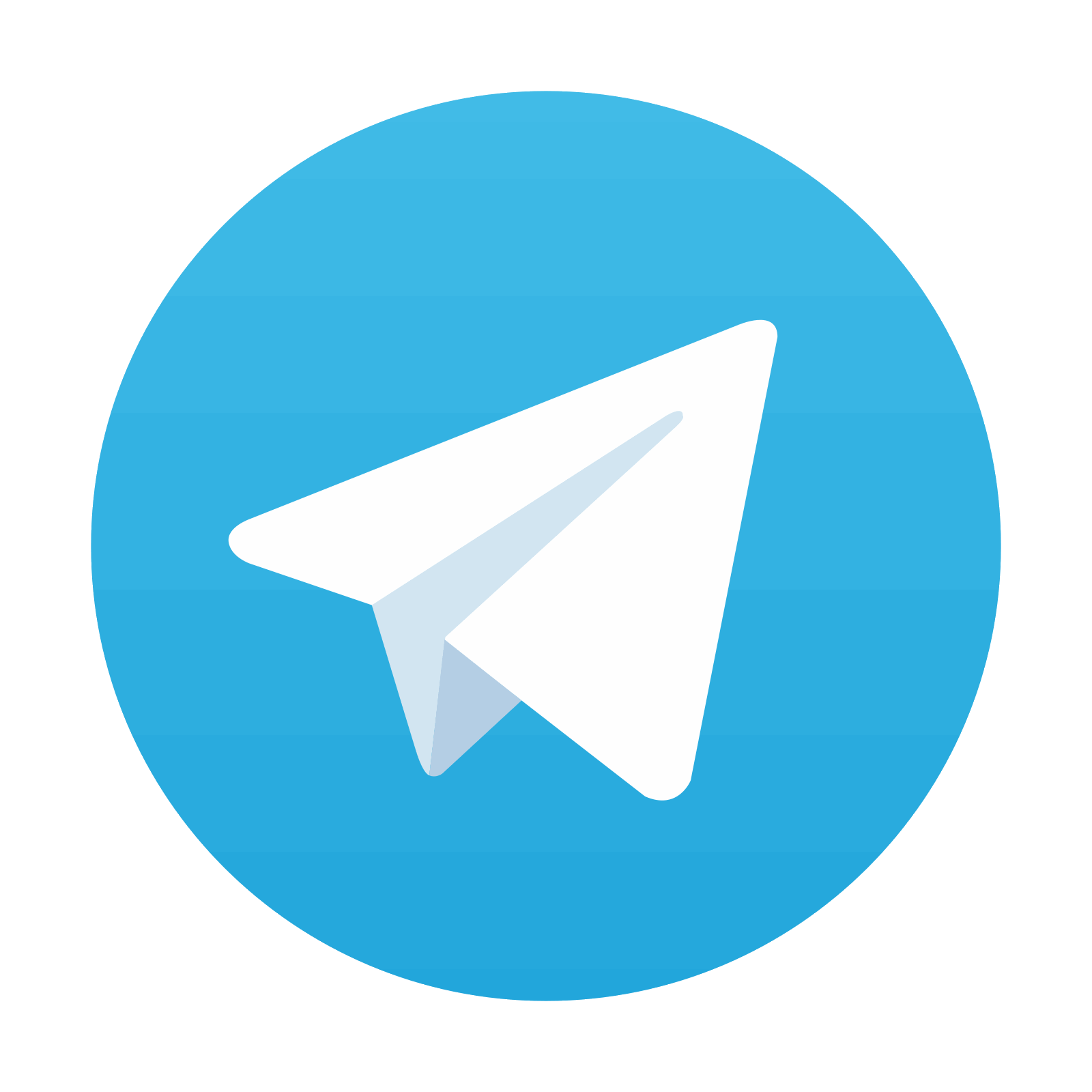
Stay updated, free articles. Join our Telegram channel

Full access? Get Clinical Tree
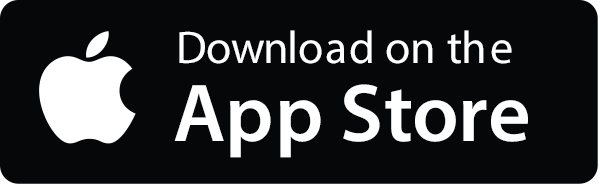
