Fig. 14.1
Effect of allele frequency and relative risk on the required sample size to generate 90 % power to show significant associations (P = 0.01) for co-dominant susceptibility alleles, assuming one control per case.
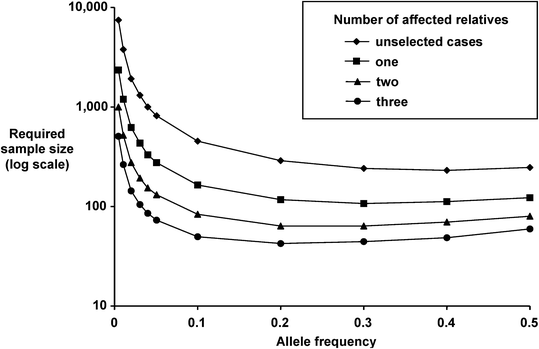
Fig. 14.2
Effect of affected family members on sa mple sizes required to generate 90 % power to show significant associations (P = 0.01) for co-dominant susceptibility alleles conferring a relative risk of 2, assuming one control per case.
The identification of the rare protein truncating variant 1100delC in the cell-cycle-checkpoint kinase 2 (CHEK2) gene in breast cancer patients illustrates the potential of association studies using familial cases to detect rare susceptibility alleles conferring modest relative risks. This variant has a population frequency of less than 1 % and confers a 1.7-fold increase in breast cancer risk [29]. Among unselected breast cancer cases the frequency of CHEK2 1100delC was not significantly increased, but in familial cases not carrying BRCA1 or BRCA2 mutations the frequency was markedly increased. Subsequently the relative risk in pooled unselected cases has been demonstrated to be 2.3 [30]. Enrichment for genetic factors may also be achieved by selecting cases with an early age of onset, although this has limited effects on power to detect associations in comparison to family history [28].
14.5 Applications of SNP Genotyping in Cancer Susceptibility Gene Discovery
14.5.1 Direct Association Studies
Most of the known disease alleles in Mendelian cancer syndromes are SNPs within coding regions that result in protein trun cation and hence total or very severe loss of function [6]. Ninety-five per cent of germ line mutations in the APC gene giving rise to the colorectal cancer susceptibility syndrome familial adenomatous polyposis (FAP) , for example, are protein truncating [31]. Low penetrance cancer susceptibility alleles are similarly most likely to be coding variants, and the majority of analyses performed to date have been direct association studies which focus on SNPs that are thought to alter protein function or gene expression [18]. The analysis of functional SNPs has the potential to be a powerful method of cancer gene discovery since the number of common coding SNPs is only a fraction of the total number. It is estimated that there are 50,000–250,000 SNPs which confer a biological effect, most of which are distributed in and around the 30,000 genes [5]. Most cancer association studies have focused on functional SNPs in candidate genes that encode proteins thought to be involved in carcinogenesis, such as those involved in apoptosis, cell-cycle control, carcinogen metabolism, or DNA repair, or those known to be somatically altered in cancer [24]. Candidate genes may also be identified from linkage analysis, expression array analysis, and comparative genomics. The disadvantages of the direct association approach are that it relies on existing knowledge to select candidate genes, identify potentially functional SNPs within these genes through database searching, and accurately predict their functional effects.
The association between the MTHFR C677T polymorphism and colorectal cancer (CRC) risk can be seen as an illustration of the successful application of the direct association paradigm. Folate metabolism impacts on both DNA methylation and DNA synthesis and repair, and aberrations of both these processes are known to be important in colorectal carcinogenesis [32]. Epidemiological studies lend further support for a role of folate metabolism in CRC development with high folate intake individuals generally showing a reduced CRC incidence [33]. The methylenetetrahydrofolate reductase (MTHFR) enzyme occupies a pivotal position in the folate metabolism pathway, directing the flow of one-carbon moieties towards DNA methylation and away from DNA synthesis (Fig. 14.3). Thus, there is strong a priori evidence that genetic variants in folate metabolism genes, and in the MTHFR gene in particular, might confer susceptibility to CRC. In vitro studies have demonstrated that the C677T polymorphism in the MTHFR gene gives rise to an enzyme with 35 % of normal activity, making this SNP an attractive candidate for direct association studies [34]. A number of such studies in a variety of different populations have been performed, and the pooled estimate from a recent updated meta-analysis indicated that compared to the homozygous wild-type genotype the MTHFR 677TT genotype was associated with a 17 % reduction in CRC risk [OR 0.83; 95 % confidence interval (CI): 0.75–0.93] [35]. Although an OR of 0.83 is modest, because of the high frequency of the wild-type allele conferring an increased risk (0.68 in Caucasian populations) this translates into a relatively high population attributable risk, and it was estimated that MTHFR C677T genotype contributed to approximately 15 % (95 % CI: 9–22 %) of the total incidence of CRC. It is noteworthy that only a few of the studies included in this meta-analysis individually showed a significant association with CRC risk, and pooling of data from over 12,000 individuals with CRC was required to demonstrate a significant association. This illustrates the order of magnitude of the sample sizes required to generate adequate statistical power to reliably identify low penetrance susceptibility alleles.
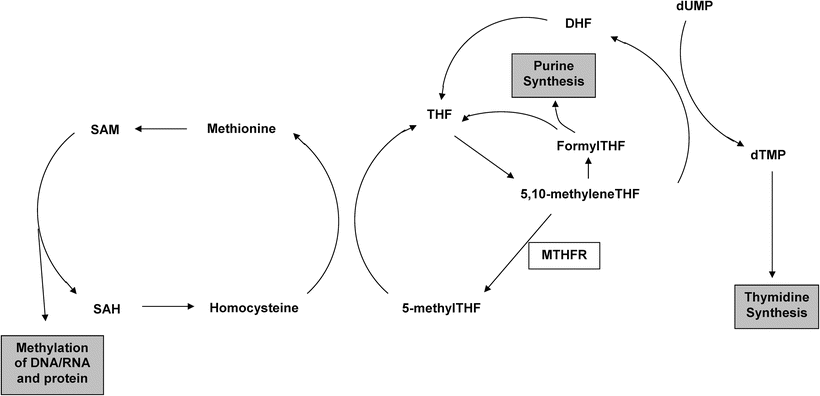
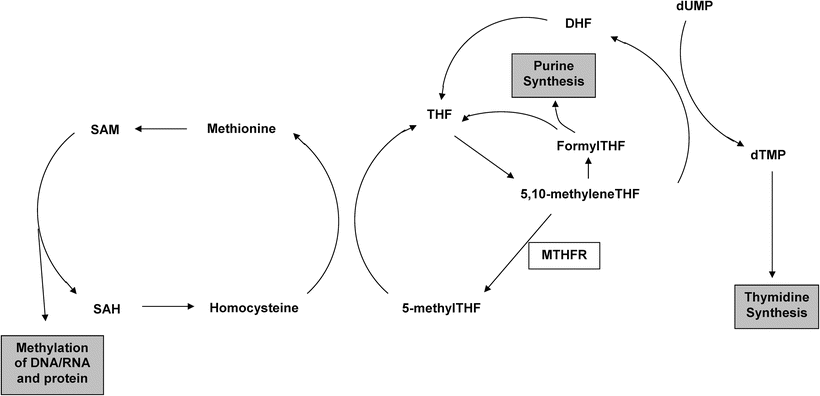
Fig. 14.3
Schematic representation of fola te metabolism. MTHFR methylenetetrahydrofolate reductase, THF tetrahydrofolate, DHF dihydrofolate, dUMP deoxyuridine monophosphate, dTMP deoxythymidine monophosphate, SAM S-adenosylmethionine, SAH S-adenosylhomocysteine.
The assembly of adequately sized sample sets has been a frequent obstacle to identifying low penetrance susceptibility alleles. Pooling of data from a number of different studies that have analysed the same SNP in independent sample sets has therefore been a popular method of generating adequate statistical power [21, 23, 36]. Examples of genetic variants with evidence from pooled analyses for association with colorectal and breast cancer risk are listed in Table 14.1. When considering such pooled analyses it is important to identify methodological issues which may affect their reliability. Firstly, an extensive search of all studies potentially suitable for inclusion in the pooled analysis should be performed, and few, if any, exclusion criteria applied in order to avoid ascertainment bias. Authors should be contacted where necessary, if the required data has not been presented in publications. Secondly, evidence of significant heterogeneity between the individual studies included in the analysis makes interpretation of the pooled estimate problematic [40]. Where there is evidence of such between-study heterogeneity, attempts should be made to identify potential sources, such as differences in study design, in particular the use of hospital-based rather than population-based control subjects, differences in ethnicity or geographic location of study subjects, and the methods of genotyping employed [41]. Thirdly, an assessment of publication bias should made, since significant publication bias means the pooled estimate is unlikely to reflect the true influence of the genetic variant under study [42]. Despite these potential methodological problems, carefully designed pooled analyses remain a useful tool, and most of the low penetrance cancer susceptibility alleles identified to date have been confirmed by data-pooling.
Table 14.1
Summary of significant associations in pooled analyses between specific polymorphisms and risk of colorectal and breast cancer
Cancer | Polymorphism | Number of studies (total number of cases) | Risk group | Pooled OR (95 % CI) | Reference |
---|---|---|---|---|---|
Colorectal | APC I1307K | 3 (670) | K carriers | 1.58 (1.21–2.07) | [23] |
GSTT1 deletion | 11 (1490) | Homozygous deleted | 1.37 (1.17–1.60) | [36] | |
HRAS-1 VNTR | 5 (394) | Rare alleles | 2.50 (1.54–4.05) | [23] | |
MTHFR C677T | 25 (12,243) | TT genotype | 0.83 (0.75–0.93) | [35] | |
NAT2 | 4 (201) | Fast acetylators | 1.67 (1.11–2.46) | [36] | |
Breast | CASP8 D302H | 14 (16,423) | Per alleleb | 0.88 (0.84–0.92) | [37] |
CYP1b1 V432L | 9 (3391) | L carriers | 1.50 (1.10–2.1) | [38] | |
CYP19 (TTTA) n | 3 (1404) | (TTTA)12 carrier | 2.33 (1.36–4.17) | [21] | |
IGFBP3 –202C>A | 3 (5673) | AA genotype | 0.88 (0.80–0.98) | [65]a | |
PGR V660L | 5 (7593) | Per alleleb | 1.08 (1.01–1.14) | [65]a | |
TGFB1 L10P | 11 (12,946) | Per alleleb | 1.08 (1.04–1.11) | [37] | |
TGFBR1*6A | 3 (555) | *6A allele carriers | 1.48 (1.11–1.96) | [39] | |
TP53 A72P | 3 (412) | P carriers | 1.27 (1.02–1.59) | [21] |
14.5.2 Indirect Association Studies
The identification of large numbers of SNPs across the human genome has allowed association studies to progress from the analysis of a small number of specific candidate SNPs, to assessing a much greater proportion of the genetic variation within a particular gene or gene region to detect any allelic association. Such indirect association studies rely on LD between multiple SNPs across a small region, allowing analysis of all SNPs within the LD block through the genotyping of one or a few tagging SNPs. With indirect association studies it is assumed that any cancer causing SNP within the region is unlikely to be analysed directly, rather SNPs in the same LD block will be genotyped, and hence show association with disease. The recent rapid advances in our knowledge of polymorphic variation, and the availability of this information in public databases, has allowed the development of methods and software to select SNPs spanning gene regions such that at least one SNP per LD block is chosen for analysis [43, 44]. This set of tagging SNPs can then be genotyped in a series of cases and controls to test for association. If an association is found, the component SNPs within the LD block should be examined to determine the causal variant, a process that may involve genotyping of additional SNPs to better define the haplotype structure.
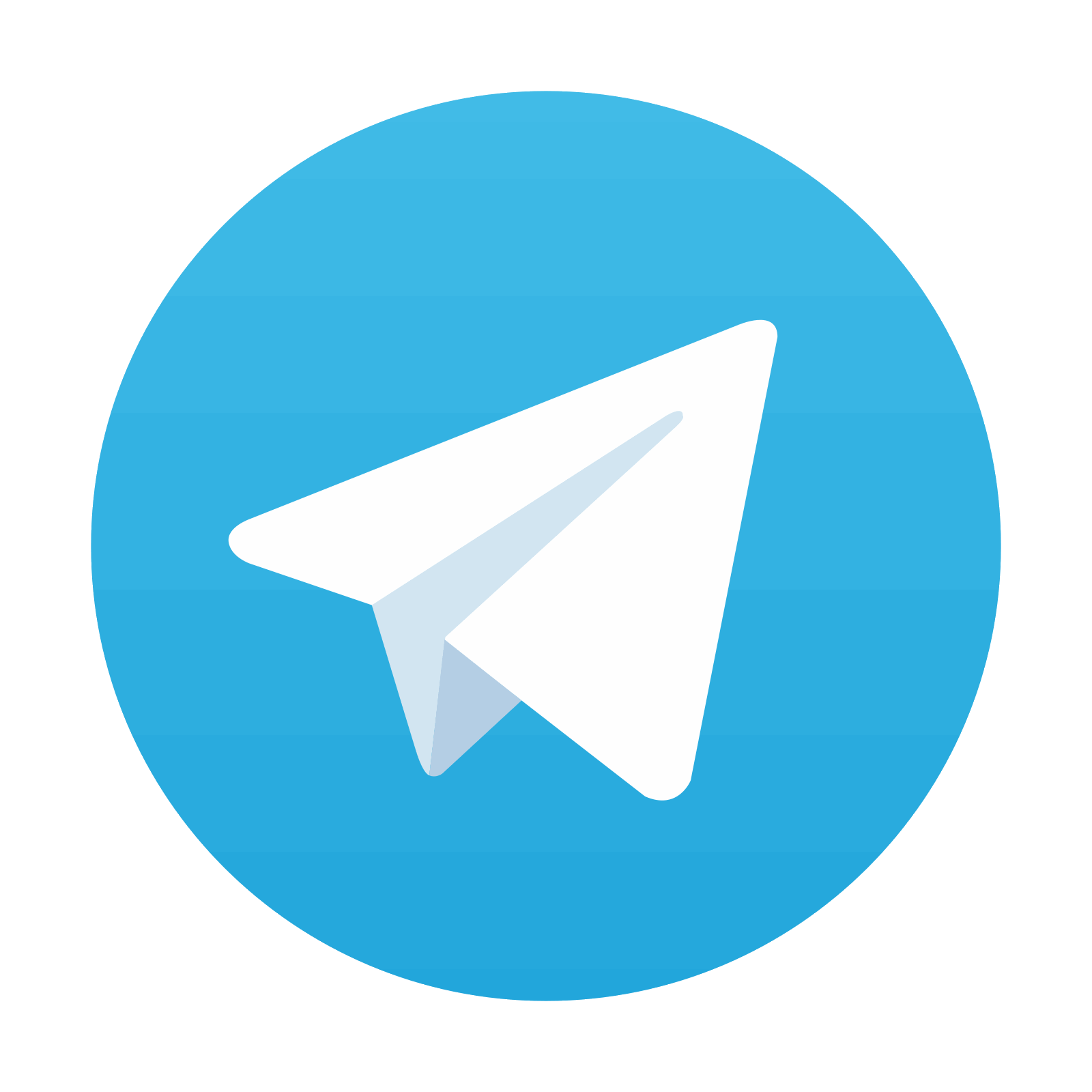
Stay updated, free articles. Join our Telegram channel

Full access? Get Clinical Tree
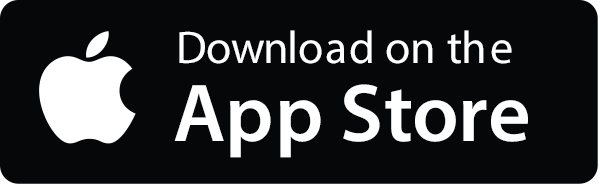
