10 Three-Dimensional Conformal Radiotherapy and Intensity-Modulated Radiotherapy
IMRT is becoming a mature technology, and is widely applied to many cancer sites. Many treatment-planning comparison studies have demonstrated the clear dosimetric advantages of IMRT.1–6 Clinical results from the past decade have shown the improvement of local tumor control and reduction of treatment toxicities for prostate cancer, head and neck cancer, and other types of cancer.7–14
Three-Dimensional Conformal Radiotherapy and Intensity-Modulated Radiotherapy Treatment Planning Process
Conventional radiotherapy entails irradiation of the patient from a few beam directions (as the accelerator rotates about the patient), using beam configurations such as that shown in Fig. 10-1, which depicts a five-field isocentric treatment of the prostate. Usually all beams are aimed at a single point denoted as the isocenter that geometrically represents the intersection of the axis of rotation of the linear accelerator gantry head, the collimator assembly, and the treatment couch. Although most often the geometric center of the tumor is purposely positioned at the isocenter, 3D-CRT and IMRT planning do not stringently require that the isocenter be located at the geometric center of the tumor. In many special clinical scenarios (such as in treatment plans for head and neck and breast cancer), the isocenter may be deliberately placed on a specific location, off the geometric center of the tumor. Even though the intervening superficial tissues receive higher radiation doses than does the tumor for each individual beam, the summation of all beams results in a higher dose to the tumor. With conventional radiotherapy, the radiation shape (or aperture) from each beam is manually drawn on the projected two-dimensional (2-D) images, taken from an x-ray machine (referred to as a simulator), which simulates the geometry of a treatment machine (a linear accelerator). With easy access to computed tomography (CT) in modern radiotherapy departments, planning CTs are acquired for most patients undergoing radiotherapy. Sometimes the CT unit, equipped with a flat couch top and alignment laser system, is referred to as a CT simulator. After acquiring a planning CT, the treatment tumor volumes are directly delineated on the CT images, and the radiation portals (or apertures) are designed to conform to the tumor volumes. The difference between conventional radiotherapy and 3D-CRT lies in whether the planning CT is used to define tumor volume and to design treatment portals accordingly. Therefore, 3D-CRT entails more sophisticated shaping of the dose distribution than does conventional radiotherapy because the collimation design (or shaping of fields) and the selection of beam directions are based on 3-D CT images of the patient. These images, projected in a so-called beam’s eye view (BEV) format (described more fully later), permit us to select beam directions with short pathways to the tumor and better avoidance of normal tissues. IMRT goes one step beyond 3D-CRT by enabling variations of the radiation intensity within each beam. This intensity modulation can be achieved via several different approaches, including fabrication of complex physical compensators (often fabricated with computer-controlled milling machines) to be placed in the radiation beam between the radiation source and the patient, but more commonly via the use of a multileaf collimator (MLC) capable of dynamic beam delivery or use of multiple static beams sequentially altered in shape by the MLC. The details of these delivery methods are discussed in the third section of this chapter, “Delivery of Intensity-Modulated Treatment.”
To precisely mimic the patient’s treatment position, simulation first determines a proper posture for the treatment position, including the use of a proper type of immobilization device to facilitate precise reproduction of patient position during simulation, other image acquisition, and multifraction treatment delivery. Often, during the simulation, a patient-specific body mold is fabricated to fix the treatment posture. Fig. 10-2 shows a patient fixed in a head, neck, and shoulder mask on the treatment table. Subsequently, 3-D images are acquired from which the radiation oncologists can delineate target and nearby sensitive structures. Sometimes medical physicists or dosimetrists assist radiation oncologists to contour common sensitive structures, such as the spinal cord, lungs, and liver. The delineation of anatomic volumes is usually done directly on a computer display of transverse CT images using standard computer graphics options such as a mouse, track ball, light pen, and so on. More often, MRI, PET, or PET/CT images are registered with the planning CT to provide better soft tissue contrast (such as in MRI images) and better physiologic information (such as in PET images).
IMRT usually incorporates computerized iteration of radiation beams as opposed to the manual optimization just described. In this process, the computer performs a large number of iterations in less time than a human could perform a few iterations. Further, the computer can modulate the dose intensity within each radiation portal, which is typically divided into multiple pencil beams, each on the order of several square millimeters. The combination of sub-beams of different intensities plus a large number of iterations often enables significantly improved dose distributions with IMRT as opposed to 3D-CRT.15
Patient Setup, Immobilization, and Image Acquisition
The minimization of patient set-up uncertainty is more important in 3D-CRT than in conventional radiotherapy because of the improved conformality of the dose distribution (i.e., quick dose gradient between the boundary of tumor volume and normal tissue). This becomes even more critical in IMRT because IMRT plans often produce even sharper dose gradients at the boundary between the tumor volume and normal tissue.16 Thus, immobilization devices and precise patient positioning procedures must be applied throughout the process of image acquisition, simulation, and treatment. Many different techniques and devices are in use to achieve this, including conventional devices such as vacuum cradles, plaster casts, face masks, and so on. More elaborate devices specific to IMRT are also being introduced such as immobilization devices that attach directly to the treatment couch to ensure that patients are positioned in the same location during each daily treatment. The same “index positioning method” is also used during the CT image acquisition. Stereotactic body frames have also been developed to achieve set-up accuracy approaching that of stereotactic radiosurgery.
PET/CT simulation is similar to the CT simulation except the procedure is carried out on a combined PET/CT scanner; again, a flat tabletop and alignment lasers are necessary for the purpose of radiotherapy treatment planning. Direct PET/CT simulation avoids the additional planning CT acquisition, improves the workflow, and reduces the potential errors during image registration if a PET scan is acquired separately or if a PET/CT scan is acquired in a nontreatment position.17
For some cancer sites (e.g., the prostate), MRI provides much better soft tissue contrast than CT images. MRI simulation can be desirable for reasons similar to the desirability of PET/CT. MRI scanners used in radiology are close scanners, making them inaccessible to the patient once the patient is in the scanner. To set up the patient in a desired treatment position and with a proper immobilization device, an open MRI simulator is preferred. Chen and colleagues18 reported their clinical implementation using a 0.23-tesla (T) open MRI scanner for prostate patients. The MRI scanner consists of two poles, which are each approximately 1 meter in diameter. The separation between the two poles is 47 cm. The MRI scanner table can move in and out along a set of rails mounted on the floor and laterally on an orthogonal set of rails built in the couch. Vertical movement of the table is made by using a composite flat top with hard foam spacers beneath the patient. A set of three triangulation lasers (center and laterals) identical to those used on linear accelerators has been used for patient positioning.
The challenge of directly using MRI for radiotherapy treatment planning is that MRI does not contain the electron density information needed for the dose calculation and image distortion in part because of the inhomogeneities in the main magnetic and gradient fields, and in part because of the differentiation in chemical shift and susceptibility of fat and water. With a low magnetic field of less than 0.5 T, Chen and colleagues18 report that the latter effect is reduced to 1 to 2 pixels. The distortion caused by the inhomogeneity of the magnetic fields increases as the patient size increases. Even with the distortion correction algorithm, residual distortion was reported greater than 2 cm for lateral patient size larger than 40 cm, whereas the distortion for patient lateral size smaller than 38 cm is insignificant dosimetrically.
Delineation of Treatment Volume and Critical Organs
Treatment volumes are defined on the CT images according to International Commission on Radiation Units and Measurements Report 62 nomenclature,19 with the gross target volume (GTV) defined as the visible tumor as seen on CT or other imaging studies; the clinical target volume (CTV) as the visualized tumor plus regions at risk such as microscopic extension of disease, nodal chains, and so on; and the planning target volume (PTV) as a CTV expanded to include setup errors, patient motion, linear accelerator alignment errors, and other uncertainties. For motion tumor volume, internal tumor volume includes magnitude of movement of the tumor. The manual delineation of CTV, PTV, plus adjacent critical organs is time consuming and subject to large variations among observers. The Radiation Therapy Oncology Group and other groups of experts attempted to establish a consensus to minimize these variations.20,21 Many treatment planning systems now incorporate various software tools for performing automatic delineation of tissue structures, but these are of limited utility as current algorithms are only accurate for outlining external body contours and internal contours of very high contrast such as bone, lung, and air cavities. Other commercial programs and research institutions22–26 developed deformable image registration algorithms, permitting the planner to map contours from a template case to a patient case with a similar tumor stage and site but different anatomic characteristics. Fig. 10-3 shows an example of computer-assisted contouring in pelvic lymph nodes. The deformable image registration tools are especially useful when adaptive planning is required for patients with anatomic changes during radiotherapy. Fig. 10-4 shows an example of using deformable image registration to generate contours from an early treatment planning CT (first CT) to the middle-course treatment planning CT (second CT) for a patient with nasopharyngeal cancer while comparing them with manually drawn contours on both CT images.
Selection of Treatment Beams
To facilitate selection of beam angles and field shapes based on the relative positions of various anatomic structures, various display schema have been developed for 3D-CRT to represent the PTV and the adjacent critical organs in 3-D perspective on a 2-D computer display monitor. The treatment geometry is usually best visualized in BEV when the anatomy is viewed from the perspective of the radiation source,27,28 as shown in Fig. 10-5. Beam orientations are chosen by observing the patient in BEV from various beam directions and selecting those directions for which the PTV appears to be best separated from the normal tissues. The BEV, however, considers only the geometric property of the patient. The concept has been extended to BEV dosimetrics (BEVDs), in which both anatomy and dosimetric tolerances of the target and involved organs are taken into account for guiding the beam-placement process. BEVD objectively evaluates the goodness of angular space, and it has shown that the incorporation of the BEVD score in IMRT planning can greatly facilitate the selection of beam configuration.29–33 In Fig. 10-6, we show the score functions for an IMRT treatment of a paraspinal tumor obtained with and without BEVD guidance.29
Clinically, selection of beams is based on a combination of experience, standard protocols, and patient-specific anatomy. For example, the relatively small variations from patient to patient in the anatomic relationship between the prostate and its nearby critical structures (most importantly the rectum, bladder, and urethra) enables IMRT prostate treatment plans to follow a more or less standard protocol with regard to beam selection. A treatment planning template can be developed for each specific cancer site.33,34 For brain tumors, on the other hand, beam selection is depends on the patient-specific anatomy and the experience of the individual planner. In general, IMRT fields with large fluctuations in intensity require higher velocities and accelerations of MLC leaves during beam delivery. Selection of optimum beam directions is often more critical when treating PTVs with a complex shape or where there is minimal separation between the PTV and a critical normal structure. Carefully selecting optimum beam directions in IMRT may also reduce the complexity of intensity profiles (i.e., the magnitude of intensity gradients within the field), thus improving delivery accuracy and efficiency. Automation of beam configuration selection is still an active area of research. Unfortunately, at present, none of the available IMRT treatment planning systems can effectively determine optimum beam directions, and thus still rely on the treatment planner’s experience and intuition.
Treatment Planning
Forward Planning
In contrast to IP (discussed in the following section), forward treatment planning involves the planner manually selecting the number of beams, their directions and shapes, inclusion or exclusion of hard or dynamic wedges (wedges modulate beam intensity monotonically along a selected direction), and the relative weightings of each beam shape (the static beam intensities). The computer simply calculates the resultant dose distributions from these beam parameters. In the sense of FP, the dose distribution is predictable by the beam parameters set by the planners. This is fundamentally different than IP in which the human treatment planner specifies the desired dose constraints (ideally, the desired dose shapes or distributions) and the computer calculates the required beam intensities and shapes to best meet the specified dose constraints. The specified dose constraints are a simplified description of dose shapes or distributions because it is not intuitive to depict a desired dose distribution. Table 10-1 provides an example of the desired dose constraints of a concurrent treatment of the pelvic lymph nodes and prostate cancer plus a cone down to the prostate alone.
Table 10-1 An Example of the Desired Dose Constraints of a Concurrent Treatment of the Pelvic Lymph Nodes and Prostate Cancer Plus a Cone Down to the Prostate Alone
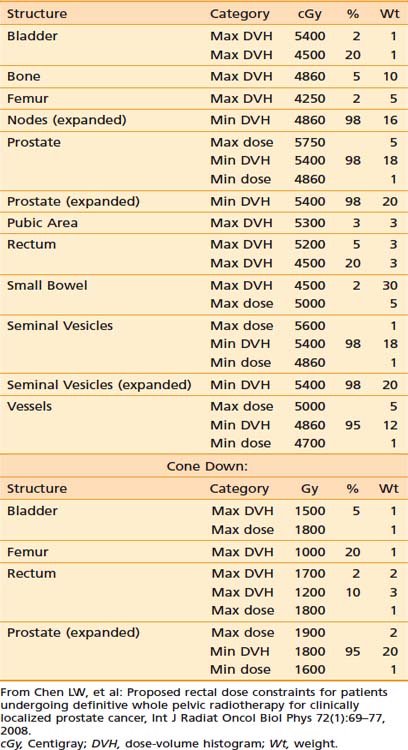
The advantage of FP is in its ability to produce a simple plan with a small dose variation inside the tumor volume.35,36 For example, in a treatment plan of a breast, Mayo and colleauges37 compared 3D-CRT plans with wedges to FP-IMRT, IP-IMRT, and a hybrid of open fields and some IP fields. This study reported that the tangent-only IMRT plans, created with an IP method, resulted in the worst dose homogeneity inside the breast, the worst maximum dose outside the target, and 2.3 times more monitor units (MUs) than the FP-IMRT plans. In combining two open tangents with tangent IMRT beams,37 it was found that a plan quality comparable to FP-IMRT could be achieved in significantly less planning time.
Inverse Planning
Principle of Inverse Planning
Inverse treatment planning was first proposed by Brahme in 1988.38 With this process, the user does not directly attempt to optimize or readjust beam shapes and their associated intensities. Instead, after defining the orientation and energies of all beams (but not their intensities or shapes) the planner specifies the desired dose limits (or constraints) for the PTVs and all regions of interest. The computer optimization algorithm first divides each beam into many small “beamlets” (or “rays” or “pencil beams”) and then iteratively alters the intensities of beamlets until the composite 3-D dose distribution best conforms to the specified dose objectives. After the optimal beam intensities and resulting dose distribution have been determined, the computer must then calculate the sequence of MLC leaf motions that will achieve this in the most efficient way. The details of the various steps in this process are described in the following sections.
Cost Function of Inverse Planning
Central to the success of any optimization schema is the specification of an objective or cost function. The cost function is a mathematical definition of the “goodness” of a treatment plan, and the computer optimization algorithm attempts to minimize the cost function as it adjusts the beam weights from one iteration to the next. Objective functions (OFs) can be based on biologic criteria,39,40 but more often are based on dose criteria.41–43 Biologic-based OFs use a calculated radiobiologic response as a measure of the merit of a plan (with calculations based on some model that relates radiation dose plus volume of irradiated tissue to predicted biologic response). The use of biologically weighted OFs is in principle more relevant because treatment outcome is determined by the biologic response. However, a universally accepted biologic model that predicts treatment outcome is yet to be developed. In the meantime, it seems that a clinically sensible and feasible approach is to construct the OF empirically based on the clinical outcome data.44,45
In reality, most popular IP algorithms are still relying on dose-based cost functions for historical reasons. Some commercial planning systems have recently started including the option of biologic model–based IP. The numerical value of a dose-based OF is calculated from some weighted average of the differences between delivered and prescribed doses for every voxel in every tissue defined in the treatment plan (i.e., the PTV plus all normal tissues for which a dose constraint has been specified).41,43,46 The prescription dose to the target, specified tolerance doses to normal tissues, and weighting factors that reflect the importance of each tissue are designated as the constraints.
For the PTV, one of many possible OFs is:
in which Σi represents a summation over all voxels in the PTV and wi equals the weighting (or penalty) factor for the ith voxel.
Usually within a PTV all voxels would have equal weighting factors, but this does not have to be true—a voxel-specific penalty scheme can, in general, significantly improve the final dose distribution because of greatly enlarged solution space.42,47 The calculated dose equals dcal (using the current beam parameters) for the ith voxel. The prescribed dose equals dpres for the ith voxel.
The exact formulation for the objective, however, could take many other algebraic forms. For example, the absolute value of (dcal − dpres)i could be used rather than its square. Or one could include (dcal − dpres)i in the summation only if (dcal − dpres)i is negative; that is, one must assign a penalty only if the calculated dose is less than the prescribed dose. Conversely, when calculating the contribution to the OF for normal tissue, one would usually assign a penalty only if dcal is larger than dpres. The total OF is then the sum of the OFs for each tissue, weighted by the wi values, which will likely differ for each tissue. For example, one would often assign a higher penalty or weighting factor to the spinal cord than to the rectum or bladder, as the former is a more radiosensitive structure with more disastrous consequences if overdosed. Automation of the weighting factors in IP has been reported by Xing and colleagues.48
Several OFs are illustrated graphically in Fig. 10-7. For the PTV or target, the graph illustrates the concept of the “allowable inhomogeneity.” That is, if the dose is between a lower limit Pl and an upper limit Pu, no penalty is assessed. Also, a larger weight can be assigned to penalize underdose as opposed to overdose (or vice versa). For normal tissue, a penalty can be applied if the dose exceeds a certain critical value (Dc) or is based on dose-volume considerations, as represented schematically in the rightmost graph of Fig. 10-7.
To specify realistic dose constraints, users need to learn the relationship between the dose constraints and the resulting dose distributions for the specific IP system they are using, plus have some familiarity with basic radiation dosimetric concepts. This learning process is one of trial and error, and is less intuitive than the trial and error planning process involved in 3D-CRT planning. Once the treatment planner has gained this experience, it becomes possible to develop disease-specific templates that can be used as starting-point dose constraints for commonly treated tumor sites such as the prostate,49,50 the nasopharynx,45 and the oropharynx.51 Patient-to-patient anatomic variations obviously limit the use of standard dose constraints, but they can be good starting points.
Generation of Leaf Motion Files
There are many possible sequences of dynamic leaf motions (or equivalently, combinations of multiple static field segments) capable of producing a desired intensity pattern because the problem of leaf sequencing is underdetermined mathematically.15 Depending on the delivery method, algorithms have been developed to minimize the number of segments, the number of total MUs, the leaf travel distance, and the total delivery time.52–57 Here, we describe one basic leaf sequencing method using DMLC (sliding window).
This leaf sequencing method54 divides the 2-D intensity distributions into a number of one-dimensional intensity profiles, with each profile delivered by one pair of leaves. The leaf paths are illustrated in Fig. 10-8, which shows a schematic representation of radiation delivery using the sliding window method. The dotted lines represent the positions of a leaf pair (x-axis) as a function of beam-on time (y-axis). Both leaves start at the extreme left edge of the intended treatment field. As the beam is turned on (a), both leaves move at different speeds from left to right (initially the right leaf moves more rapidly than does the left leaf). The point P begins to receive radiation when the right leaf edge moves past it (b), and continues to receive radiation until the left leaf blocks the beam (c). By controlling the movement of the leaves and therefore the “beam-on-time” duration between b and c, one can deliver any desired intensity to point P, or any other point under this leaf pair. The solid line depicts the total integrated beam intensity to all points underneath the strip of tissue being treated by this leaf pair.
Direct Aperture–Based Optimization
The typical pixel-based or fluence-based (described previously) IP optimization often results in complex IMRT plans, which prolongs the treatment time and dramatically increases the number of MUs by two to five times.58 The latter could lead to increased leakage and scatter radiation through the MLC, and this indirect radiation contribution has been shown to adversely affect the accuracy of treatment delivery.59 More importantly, the increased exposure from complex IMRT plans may also increase the frequency of radiation-induced secondary malignancies. It has been recently reported that the transition from 3D-CRT to IMRT resulted in a larger volume of normal tissue exposed to a low dose of radiation, which was estimated to increase the incidence of secondary cancers at 10 years from 1% to 1.75%.60
To simplify IMRT plans (or avoid overly complex IMRT plans) while improving delivery and radiation efficiency, many researchers have been working on increasing the efficiency of leaf sequencers.55,57,60–63 Some leaf sequencers minimize the total number of segments, whereas others minimize the total required MUs. Other researchers have proposed the use of smoothing filters to eliminate unnecessary noise inside the intensity profiles, either during optimization or prior to leaf sequencing.64,65 With most commercial planning systems, options for controlling the complexity of an IMRT plan are often limited to choosing coarse intensity levels during conversion, selecting a leaf sequencer that can provide an optimal delivery efficiency for the specific delivery method (e.g., sliding window or step-and-shoot methods, discussed further in “Static Versus Dynamic Multileaf Collimator Delivery,” later in this chapter), or using smoothing filters.66–68 However, the effectiveness of these methods in controlling the complexity of an IMRT plan is limited, often resulting in significant deteriorations in plan quality.
Recently proposed direct-aperture optimization (DAO) directly optimizes weights and leaf positions with a predetermined number of MLC shapes permitted for each beam.69–72
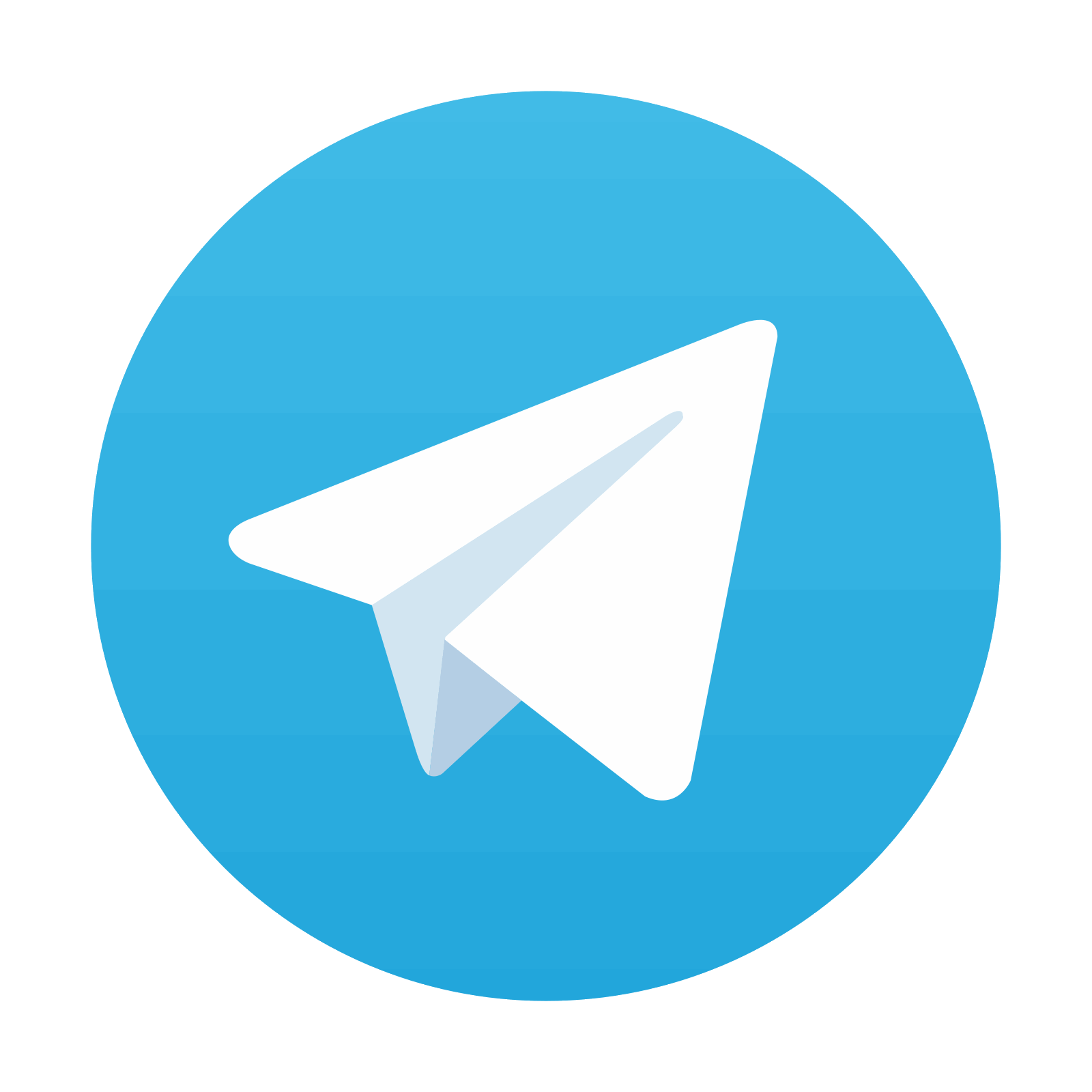
Stay updated, free articles. Join our Telegram channel

Full access? Get Clinical Tree
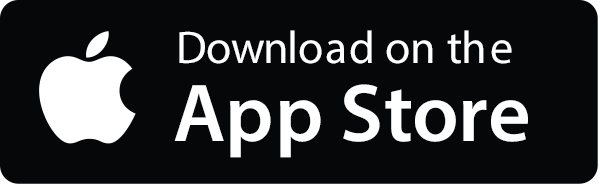
