Fig. 17.1
Some characteristic issues of complex biological systems. We can notice that these are interdependent phenomena that cannot be treated separately, hence the need for integrative methodologies
HTOTs provide us with tools to measure each one of these types of data, in a multiplexed yet single-issued manner (i.e., we can have experiments done to measure the whole transcriptome or the proteome, even the [protein] interactome); for this reason, integrative frameworks [17, 18] are needed to organize and interpret experimental data [19, 20] in order to provide a fuller, deeper understanding of the way different pieces of evidence should be put in order to fit within a given biological mechanism or at least to an approximate model [21] of it [1, 8, 22, 23]. In reference [17], it is described as a multilevel data integration platform which integrates data about genes, transcripts, and proteins reported in literature as altered in breast cancer cells. Besides the data integration, the database provides an ontology-based query system and analysis tools related to intracellular pathways, protein–protein interactions, protein structure, and systems modeling in breast cancer. The rationale behind this effort is that cancer complexity needs to be studied from such an integrative standpoint as the one provided by systems biology. This is, in our opinion, the kind of studies that may provide a stronger improvement in cancer research in the future. In order to have a comprehensive view of the different omic technologies used in breast cancer research, some of them are shown in Fig. 17.2.
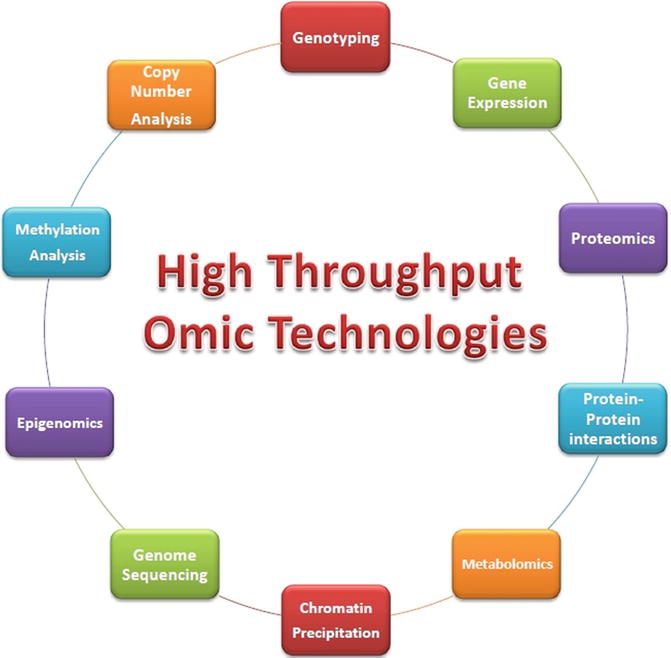
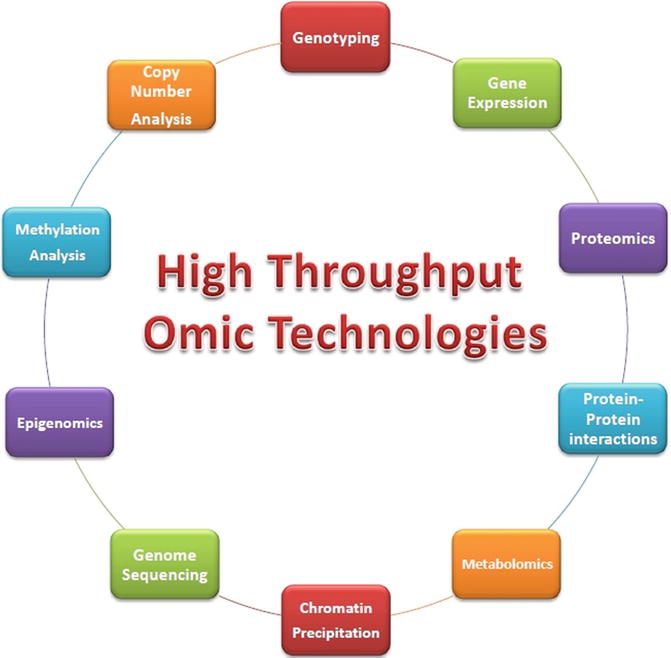
Fig. 17.2
Some high-throughput omic technologies (HTOTs) that are commonly used to probe the complexity of breast cancer biology. It is by integrating several of these data sources that systems biology builds a better understanding of the often complex interrelationships present at the molecular basis of breast cancer
These challenges are specially intriguing when it comes to cancer since the complexities associated with tumor phenotypes often call for deeply multidisciplinary approaches [23–25] in order to be properly disentangling the systems’ complexity to give birth to intelligible models useful in both the basic research and clinical settings. When approaching biological behavior integrating from these multidimensional studies, one is often led to reconsider well-established foundational principles such as the role of oncogenes [26, 27], the nature of transcriptional master regulators [28], the possibility of rewiring systemic metabolism to make anticancer therapies more effective [29–31], or even an entanglement of these three issues [32, 33].
For instance, when discussing the role of master regulator genes (MRGs) in breast cancer, a team led by Califano [28] presented a probabilistic modeling, computational systems biology approach that is able to infer robust prognostic markers by the identification of MRGs, causally related to the presentation of the phenotype. This way, they were able to find groups of upstream transcription factors whose expression patterns correlate with the transition from the normal cell phenotype to the malignant one. They look up for MRGs since gene regulatory networks (GRNs) work commonly as amplification cascades; thus, genes that are most differentially expressed tend to be further downstream from the somatic events underlying phenotype differences. These downstream genes are also often less stable, as many cofactors and potential noise sources are involved in the transcriptional cascade that leads to their differential expression. One may recall that oncogenes and tumor suppressors are not generally the most differentially expressed genes.
In order to face such tremendous challenges, systems biology has developed a multidisciplinary approach based on several milestones. In essence, biological discovery in systems biology is based on tools aimed at a global study level of the different HTOTs; such methods are means for information classification, quantification, computation, visualization, archiving, and retrieval. A particularly useful paradigm is that of complex networks. Under the network view, biological systems consist in a (usually very big) set of elements or components in the form of biological molecules: DNA segments, RNA transcripts, enzymes and other proteins, biomolecular complexes, molecular machines, etc. These components interact through a variety of mechanisms: gene regulation, DNA–protein interactions, RNA–protein interactions, metabolism, protein–protein interaction, biochemical pathways, and so on. The components are the nodes in these bionetworks, while the interactions are the links. Systems network biology is built upon two disparate but complementary ways to construct such networks, often referred to as the bottom-up and top-down approaches [5].
In the bottom-up (sometimes called kinetic) view, models are developed based on the integration of information already available in databases, and then such models are tested under a variety of experimental conditions. In the top-down (or probabilistic) approach, a data-driven process is used to infer the correlation (or interaction) structure of the networks starting with massive data from HTOT experiments; then a model is built that can be tested. Both views are complementary to each other. The first one serves to assess model fine-tuning, whereas the latter is aimed at new discovery. The top-down approach may be exemplified with probabilistic inference of gene regulatory networks [34, 35] and their theoretical fundamentally thermodynamical characterization [36, 37], while the bottom-up approach may be devised in metabolic reconstruction [5] or mathematical models of well-known signaling pathways [1].
Classification methods for the discoveries made through either top-down or bottom-up systems biology are needed. Most of these methods are based on computational learning techniques trained with the data in public databases such as NCBI’s GEO [38] which is a repository for whole-genome gene expression (and some epigenomic) experiments or the catalog of biochemical pathways in the Reactome database [39]. An archetypic example of such methods is the Gene Set Enrichment Analysis or GSEA [40–42]. GSEA is a computational method to evaluate whether a given set of genes (a gene set) is differentially expressed between two different phenotypes (i.e., it is enriched in one of these). GSEA may be appropriately called a systems biology method since it is based on the recognition that genes do not act by themselves at an individual level, but rather there exist synergetic, cooperative effects in their biological function mechanisms. Such cooperative effects are at the basis of the whole systems biology view [40].
Integrative Omics in Breast Cancer
The brief introduction to systems biology given in the preceding section has likely made clear the point that a technological milestone for the development of such systems-based approaches was the rise of high-throughput omic technologies. HTOTs have paved the way to a global understanding of biological phenomena that extends from the relatively straightforward (but extremely important) task of clinical and phenotypical classification to a series of deeper, more complex issues related to the mechanistic approaches of systems-level behavior of biosystems and may extend even to the (relative) control of such systems by therapeutic interventions. This section will be thus aimed to describe some of the more important applications of omic technologies in the study of breast cancer; later we will analyze the impact of such single omic approaches in a global systems biology view of breast cancer phenomenology.
One of the first applications of omic technologies (in particular of the microarray-based whole-genome gene expression analysis) was the search for molecular signatures that may be used in the phenotypic profiling of breast cancer tumors aimed at predicting clinical outcomes [43–49] or response to chemotherapeutic agents [50]. Gene expression profiling has also been widely applied to improve prognosis [49, 51] and in the subclassification of tumors [15, 52]. Gene expression profiling has also been used to determine correlation of certain molecular phenotypes with breast cancer metastasis [53].
Other HTOTs have been used to analyze breast cancer tumors. Carroll et al. [54] performed an exhaustive (genome-wide) analysis of all possible binding sites for the estrogen receptor (ER). This study’s results were of foremost importance since ER is a hormone-activated DNA-binding transcription factor which is differentially regulated in breast carcinomas. Under low-to-normal hormone levels, ER is usually located in the cytosolic region. However, when estrogen binds to the receptor, it enables the migration of the receptor from the cytosol into the nucleus and eases dimerization of the receptor and binding of the ER dimer to hormone response elements in the DNA, leading to (abnormal) transcription activation. Functional tests have proved that ER is extremely important for global gene expression deregulation, and metastasis in breast cancer and as such is determinant to evaluating the patient’s response to therapy and ultimately its final outcome.
In the case of proteomic approaches to breast cancer, these have also been used for the search of biomarkers, in particular due to the fact that oral-based proteomic tests may be relatively easy to perform since they are noninvasive. Some years ago, Sugimoto et al. [55] carried out a whole-proteome metabolomic study that revealed specific protein profiles not only in breast cancer but also in oral and pancreatic tumors. Their approach was based on capillary electrophoresis mass spectrometry of saliva. Other proteomic studies in breast cancer have been aimed at discovering risk factors, such as the case of the determination of adiponectin levels and their connection with an increase of breast cancer risk [56]. Similar studies have been carried out in relation with other tumors, such as Teiten et al.’s studies of differentially expressed proteins in cancer cell lines treated with curcumin [57].
Omic approaches to cancer have also been focused on genomic instabilities, chromosomic rearrangements, copy-number variations, and other DNA mutation abnormalities. For instance, Hicks et al. have found that certain patterns of genomic rearrangement can be associated with the survival of breast cancer patients [58], while the role of mutations in the gene PIK3CA is now associated with a higher prevalence of breast cancer [59]. Other high-throughput sequencing approaches point out to UTR reorganization in breast cancer [60], a fact that may be relevant when considering the patterns of genomic reorganization found precisely in epithelial breast cancer cells—for instance, as they have been revealed by comparative genomic hybridization arrays [61].
Systems Biology Approaches to Breast Cancer
As we already mentioned, one of the paramount issues within the systems biology approach is data integration [62]. At this point, it is clear that by developing strategies that allow a research team to integrate the individual pieces of evidence provided by different HTOTs, much can be gained with regard to actually disentangling the complexities associated with life. Due to the multifactorial nature of breast cancer, being a pathology that involves (at least) metabolic and hormonal deregulation, genomic instability, inflammation, abnormal immune response, chromosomal rearrangements, mutations, gene expression anomalies, signaling cross talk, and protein folding abnormalities, one may see that it is a natural candidate to be studied under a systems biology view.
We may start by recalling the work by Sun et al. [63] that perform an integrative analysis of gene expression, methylation profiles of CpG islands, and copy-number alterations in breast cancer cells, linking such functional genomic anomalies to mutation rates as called by deep sequencing experiments in those very cells or the efforts to categorize the effects that focal amplifications of genomic regions have in homozygous deletions and other sequence alterations not only in breast cancer but also in some types of colorectal tumors [64].
There is, of course, a need for computational strategies to integrate genomic data with proper mathematical models [34, 65, 66] and that considering such issues as microRNA targeting [67] and genomic network modularity [68], as well as data mining and integration techniques [69], leads to the identification of novel biochemical pathways [70] and other molecular mechanisms of oncogenesis [71] that, although not unique [72], may lead to the improvement of our current understanding of breast cancer biology [73]. Particular emphasis should be paid to those integrative omic approaches [74] that could result in better prediction of patient outcomes [75–77]. Such integrative studies possess a very broad spectrum ranging from the influence of major chromosomal breakpoints in epigenomic regulation differences in breast cancer [78] to the role played by plant homologs of breast cancer genes [79], in particular with regard to the interplay of these in cell differentiation and proliferation [8, 80]. In this respect, a number of biochemical pathways pertaining to cell signaling, gene regulation, and other biological functions are involved in breast cancer phenomenology; in Fig. 17.3, we can see the fundamental molecular pathways determining the evolution of breast cancer along with their relation either direct (pathway cascading) or indirect (pathway cross talk).
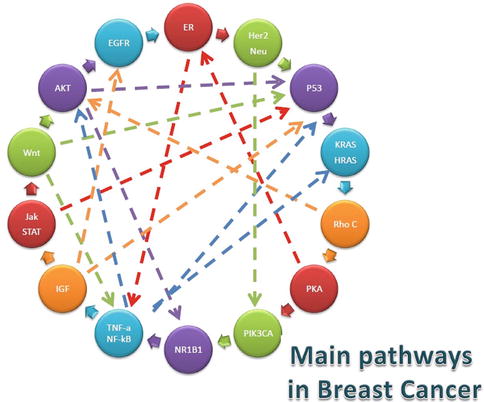
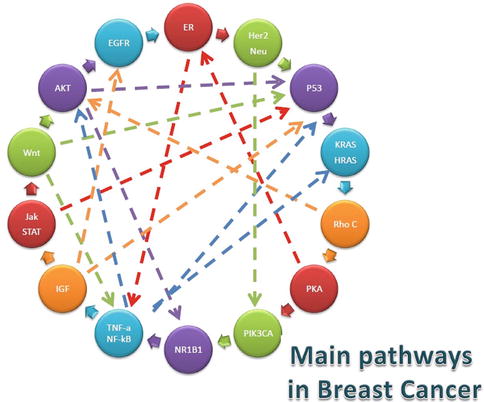
Fig. 17.3
Main molecular pathways involved in breast cancer tumorigenesis and metastasis. Direct relationships among such pathways (or pathway cascading) are shown in small, bold arrows, whereas indirect entanglement of biochemical routes or pathway cross talk is shown in long broken arrows
In relation to metastasis prognosis, reference [77] discusses a common conundrum in breast cancer genomic studies: i.e., that most mutated genes are not the ones with statistical significance in their differential expression patterns. We can see that genes such as P53, KRAS, HRAS, HER-2/neu, and PIK3CA, though not always significant in expression differences, play a fundamental role through the protein network by interconnecting many expression-responsive genes. Thus, in order to find out the concerting mechanisms, they overlaid the expression values of each gene with its associated protein in the protein interaction network and then look for subnetworks whose expression profiles were more fit to discriminate for metastasis. The discriminative potential of a candidate subnetwork was computed based on the mutual information (MI) metric between its activity score and the metastatic/non-metastatic disease status over all patients: i.e., high MI subnetworks were better at discriminate between conditions. These considerations shed new light in the genotype/phenotype paradigm since the phenotypic changes that are most indicative of breast cancer metastasis are not necessarily regulated at gene expression level.
In reference [66], the researchers have studied anomalous complex patterns of transcriptional regulation and its relation with other genomic alterations as central to carcinogenesis. They claim that “…the interplay of alterations in DNA copy number and epigenetic states is complex, and to understand the full picture data from multiple sources needs to be integrated. Since both copy number and epigenetic alterations result in changes in gene expression patterns, analysis of microarray gene expression data in the context of specific genomic regions is an efficient means of integrating the effects of genomic changes in cancer….”
Dexter et al. [66] even developed a new soft-constraints data clustering profiling method called genomic distance entrained clustering, which is based on a measure they propose. Such a clustering algorithm is aimed at identifying genomic regions where gene expression is coordinately altered. Aided by such a method, they were able to discover that—contrary to expected belief—high copy number correlates with high gene expression levels, and not all differentially expressed regions showed corresponding differences in DNA copy number, something that suggested them to consider alternative mechanisms of gene expression regulation like epigenetic repression are important in defining altered genomic regions. Such a class of integrative studies lies at the core of systemic approaches to cancer research.
Some systems-level studies are, however, guided by strong hypotheses deeply founded in the results of a variety of omic technology experiments in the past. Paradigmatic examples are the role of hormone receptors such as ER or the epidermal growth factor receptor (EGFR), which belong to a family of the so-called ErbB of receptor tyrosine kinases since they are known to play a central role in breast cancer phenomenology. Along these lines, Uhlmann and collaborators [81] have analyzed the patterns of global microRNA (miRNA) regulation in protein networks characterizing changes in the cell cycle due to EGFR overexpression in breast cancer cells, since it is well known [82] that EGFR inhibitors may play quite an important role in breast cancer therapeutics. For instance, trastuzumab resistance has been linked to cell cycle dynamics modulated by ErbB proteins (in particular EGFR and Her2/Neu) [83]. Her2 transitions have been long known to have radical consequences for breast cancer patients [84].
The role that specific mechanisms of transcriptional regulation will play in breast cancer signaling was decoded by Uhlman et al. [81]. They make use of a combined strategy to analyze the multiple miRNA–protein interactions that regulate cell proliferation in response to EGFR. Such kinds of systems biology studies lead to an unprecedented view of the combinatorial effort of miRNAs to control a signaling pathway. This may be of central pharmacogenomic importance, since oncogenic pathways commonly resist inhibition signals by single regulators. Combinatorial analysis of this kind could enable us to discern the molecular basis for selecting either individual miRNAs or small sets of these with combined activity that may be used to treat breast tumors by transcriptional regulation targeting. In particular, EGFR pathway is quite relevant for breast cancer therapy, but a number of additional downstream signal transducers, such as RAS, AKT, or CDKs, are also of some importance.
Protein interaction approaches go far beyond while suggesting a definite oncogenic role to ErbB heterodimers. In particular, Holbro and collaborators have shown that ErbB2-positive breast tumor cells require ErbB3 to enhance their proliferation rates [85]. The oncogenic role of ErbB proteins in epithelial breast cells gets further support, since blocking heregulin expression (thus difficulting ErbB heterodimerization) inhibits breast cancer tumorigenicity and metastasis [86]. Further functional genomic experiments show that cell signaling is anomalous in the presence of specific kinase–kinase inhibitors [87]. An important connection can be noticed between ErbB protein modifications and signaling in breast cancer, since it has been proved that low (or null) levels of estrogen receptor signaling may give rise to differential methylation profiles (epigenetic silencing) of downstream targets [88].
This kind of signaling cascade results is extremely important to understanding breast cancer phenomenology. In fact, chemical immunoprecipitation studies have recently shown that breast cancer-associated gene regulatory networks possess a hierarchical structure; indeed, they go beyond and hypothesize a central role in the hierarchy that is played by processes centered in estrogen receptor activity [89]. Other important hubs in transcriptional networks have been already identified; among these, we can mention E2F transcription factors [90], ATF3 [91], and a broad family of MAPK-structured transcription elements [92].
Estrogen receptor alpha (ERα) is an intermediary in the process of genomic transcription regulation in breast cancer cells. The actual mechanism involves nuclear-initiated steroid signaling and non-genomic activation of various protein kinase cascades. This ER regulation actually carries importance because it is known that tamoxifen-resistant breast cancer cells have enriched expression in gene sets targeted by estrogen treatment as compared with wild-type cells [89].
In connection with the interplay of estrogen receptor signaling in these regulatory networks, a full interactome network of estrogen receptor alpha-bounded elements has been identified by means of whole-genome chromatin precipitation experiments [93]. Estrogen regulatory response is, as we already said, of particular importance in connection with currently available chemotherapeutics, since the response to agents like tamoxifen and fulvestrant depends on complex regulatory interactions involving both transcription factor hierarchic networks and epigenomic mechanisms, in particular differential methylation profiles [94]. A probabilistic network biology study combining the use of HTOT data to probabilistically infer the Bayesian regulatory networks with a topology-driven analysis identified regulatory subnetworks driven by estrogen receptor α dynamics [95]. We cannot stress enough the importance of having such mathematical–computational models relating phenotypic traits (systemic estrogen response) to molecular features, since these may allow the performance of simulations to assess the effects of differential therapeutics, thus paving the way to individualized medicine. In this regard, it is worth mentioning that such studies have even pointed out the cyclic effects that hormone clocks may have at the onset of breast cancer tumorigenesis [96].
These systems-based studies, however, may go well beyond the descriptive stage to actually have a real impact in the clinic. We can mention as an example how these whole-genome functional genomic studies have identified mechanisms leading to the estrogen activation of the retinoid acid receptor (NR1B1) pathway (a well-known therapeutic target) [97]. Estrogen-mediated ErbB signaling is connected with abnormal proliferative processes in breast cancer [98], and for this sole reason it has been extensively studied at both the transcriptional and translational levels [99]. However, its impact goes much further than genomic regulation, since it is also well known the effect that it can have (by means of the so-called glucose-deprivation network) [100] in the counteraction of the cytotoxic effects of lapatinib in ErbB2-positive breast cancers. ErbB signaling thus has been subjected to extensive computational and mathematical modeling [101] to explore what are the main ligand-response mechanisms behind its complex signaling in breast cancer.
At the multiple-cell (tissue) level, we know that HER2 overregulation is able to inhibit E-cadherin transcription in breast tumors, thus destabilizing the catenin–cadherin complex, causing decreased adhesion. Indeed, high phosphorylation of catenin-d and catenin-g in HER2-positive cells under EGF stimulation contributes to lower levels of cell adhesion and increased cell motility. These facts, together with IGF signaling, may lead to discoveries of considerable pharmacological impact: IGF-1R induces cell proliferation and survival via MAPK and PI3K pathways, a phenomenon related to EGFR signaling in tamoxifen-resistant breast tumor cells by increasing their mitogenic strength indexes [98]. HER2 overexpression thus is able to increase tumor proliferation, angiogenesis, and invasiveness, and its presence correlates with bad outcomes. Pharmacological targeting of Her2 and its receptor kinase complex by using ectodomain binding monoclonal antibodies like Herceptin is often enough to reverse the malignant phenotype.
Apart from the hormone effect of ErbB-estrogen signaling networks, there are other fundamental genes/pathways to be considered when studying breast cancer. One that has recently gained importance is the RhoC GTPase [102] that has been mentioned as a transforming oncogene with the capacity to transform human breast cells into an inflammatory breast cancer phenotype. RhoC activation has even been linked to metastatic processes [103]. RhoC is a RAS homolog and as such is a small GTPase that after activation may regulate response proteins driving the expression of genes involved in cell growth, differentiation, and survival. With RAS being the most common human oncogene, it is no wonder that RhoC has been so widely studied in connection to cancer, in particular since it has been shown that RhoC overexpression is able to control the metastatic potential as well as the abundance of the so-called breast cancer stem cells [104].
Following a very detailed mathematical and computational modeling, Visvanathan and collaborators [1] proposed an integrative systems biology approach to analyze experimental data from the TNFα–NFκB signaling pathway model, integrating in a knowledge-based model database, both mathematical modeling data and literature information as well as biological data. The importance of this study relies on the fact that deregulation and overproduction of TNFα are very important components of many breast tumors; thus, by knocking off its expression by a specific monoclonal antibody, like infliximab, one may induce important drawbacks in the diseased phenotype. The mechanism of action of drugs such as infliximab involves pathway disruption, in particular the deregulation of the TNF pathway (infliximab belongs to a class of monoclonal antibodies anti-TNF): its action involves binding TNF, preventing it from being recognized by its receptor, and then nullifying their cytokine activity as a second messenger. But infliximab is also able to trigger programmed cell death of TNF-activated T lymphocytes. This is relevant for its action against autoimmune diseases, but it is also important for breast cancer due to the role played by pro-inflammatory cytokines in tumorigenesis and even more in metastasis [102].
Their main interest was in shifting from intercellular signals, such as the ones caused by TNFα, to intracellular signals, such as the signal transduction pathway from the membrane receptors of TNFα to the transcription factors AP1 and NFκB and to apoptosis, that is, by following the apoptosis route of TNF extracellular signaling with a view of having more impact in cell populations (i.e., tumors) instead of single cells. They did so by considering protein–protein interaction networks in their pathway mathematical modeling that includes kinetic equations for TNFα–NFκB signaling supplemented with their constants and initial concentrations.
Since glucose metabolism is involved in both tumorigenesis and proliferation in cancer cells, another well-studied set of signaling molecules is the family of insulin growth factors. The double role of IGFBP3 [105] in malignant and nonmalignant epithelial cells and in the transformation of the latter to the former in the presence of integrin receptor complexes led to a hypothesis of metabolic switching oncogenicity in breast epithelial cells. Not surprisingly, IGFBP3 molecular activation mechanisms involve cross talk with other oncogenic pathways, such as those involving PKA, RhoC, and ceramide [106]. We already mentioned the systemic role of RhoC; it is noticeable that cross talk with PKA (a protein kinase involved in regulation of glycogen, sugar, and lipid metabolism), and with ceramide (involved in the sphingomyelin signaling but more importantly involved in the protein-recruiting core of processes such as differentiation, proliferation, programmed cell death, and apoptosis).
Estrogen signaling and insulin response are not the only hormone-related processes involved in breast cancer. Recently, the role of deiodinase deregulation in cell proliferation and tumor growth has been mentioned [107], and other thyroid hormones (such as tyrosine kinases) are also involved [108] via heregulin-induced activation [109]. Such genomic processes may be also related to the response to thermal stress in epithelial cells [110] and ultimately to mechanisms of DNA repair [111] such as ATR signaling [112, 113]. Interestingly enough, ATR signaling is negatively regulated by estrogen dynamics [113], so that high estrogen levels inhibit cell cycle checkpoint and DNA repair processes while at the same time is cross-regulated by BRCA1/BRCA2 [112]. Apart from this known functional feature of BRCA genes, it is worth mentioning that they are also involved in stem cell transformation [114] as well as in epigenomic-regulated genomic instability [115].
Also in connection with epigenomics and chromatin modifications, FOXA1 has gained importance in recent times as a candidate target molecule in breast cancer [116] as well as in other neoplasms [117], and it is believed that it is an important molecule, since it may be involved in cross talk of biochemical and signaling pathways in hormone response [117]. Other hormone-related endocrine and paracrine pathways deregulated in cancer have been found through the application of HTOTs studied under a systems biology paradigm. Such is the case of serotonin/prolactin cell reuptake in breast cancer tissues [118], the hormone resistance to PARP inhibitors in triple negative breast tumors [119], and the effects of cancer phenotypes over mevalonate metabolism [120].
Systems biology studies have also enable us to develop prognostic tools that may allow better clinical decisions and more appropriate therapeutic strategies, strongly dependent on patient-specific traits (in particular molecular phenotypes). We can mention the case of a 76-gene signature developed by Desmetd et al. [121] and extensively validated in node-negative breast cancer patients in a multicentric cohort study. Other omic studies have revealed more specific traits, such as a link between inflammatory processes and survival [122], pointing out to scalability of gene expression signatures (a must for its wide clinical application), specific genes that determine breast cancer metastasis to the lungs [123], and even an integrative panel that reveals hidden relationships between p53 status to whole-genome mutation patterns and ultimately to patient survival [124]. Following similar studies, progression biomarkers have been developed, such as the protein BNIP3 [125], whose tissue levels (and even more important, serum levels) anticorrelate with tumor progression.
It is well known that most fatalities related to breast cancer are not due to the primary tumor but to metastatic processes. For this reason, whole-genome studies of tumor metastasis are extremely important from a clinical standpoint. The role of transcription factor SMAD3 in breast cancer metastasis has been discussed recently [8, 126, 127]. It has been mentioned that the mechanisms of functional interaction behind SMAD3-driven metastasis involve the activation of metastatin (S100A4) mediated by TGF-β [126] as well as antagonistic relationships between SMAD3 and SMAD2 in angiogenic processes [127]. Due to homology between SMAD2 and SMAD3, it is likely that antagonistic interactions arise due to protein moonlighting [127].
Multilevel genomic approaches to breast cancer metastasis have led us to the development of molecular signatures at both the gene expression and protein interaction levels. In the latter case, highly reproducible sets have been designed [128] by taking into account that different signature hubs may be altered in different patient sets that may affect the dynamics of the same pathways associated with cancer metastasis through their interaction neighbors, in other words, that cancer metastasis (some claim that cancer all in all) is not a gene-centered condition but rather a pathway (or network)-centered condition [8]. They find that pathways such as cell cycle, apoptosis, Jak-STAT, MAPK, ErbB, Wnt, and P53 signaling were among the ones more prone to be multi-gene/multi-target deregulated; that is, disease-associated pathways may depend on the co-expression changes of different signature hubs with the same set of neighbors enriched in this pathway.
For instance, changes of co-expression of interaction neighbors of either IL2 or IL6 might disrupt the Jak-STAT signaling pathway and contribute to breast tumor progression. Gene-centered approaches may overlook this disruption. Such designs are based on the hierarchical hub-centered structure of the associated protein interaction networks. This study implies that changes in the global modularity of the human interactome might be able to improve our understanding of the complex biochemical mechanisms behind breast cancer metastasis. To some degree, this is similar to what one can see in cancer pathways that may be deregulated by either abnormalities in cancer genes or modification of microRNAs regulating these genes. Similar network-based studies have also shed light on the rising challenge of therapy-resistant breast cancer lineages, i.e., subclonal cell populations in tumor tissues that acquire resistance to chemotherapeutics such as trastuzumab [129]. These systems-level models may enable (and are actually enabling) clinicians’ and basic scientists’ cooperation in the development of improved clinical strategies and therapeutic interventions. Some examples that have been to a certain degree successful will be discussed in the following section.
Clinical Impact of Breast Cancer Systems Biology
While it is true that systems biology-based research in the past has been mostly devoted to building and analyzing molecular models of disease (in particular of cancer) under a basic science perspective, in recent times a number of studies have started to move their efforts to more applied, clinical settings [130]. We are, of course, still quite far from the long-promised individualized therapies and personalized medicine; however, the systems approach to cancer biology is starting to narrow the gap.
To date, most of the clinical applications of systems biology approach belong to either improving the prognosis (and the prognostic!) or pointing out to leads on novel therapeutic strategies. The field of cancer molecular biomarkers is a tough one. It is known that improving the accuracy of prognostic biomarkers may lead to an optimal, target-specific use of coadjuvant therapies, thus improving the patient’s quality of life and lowering the associated costs of unnecessary chemotherapeutics. In practice, however, at most 30 % of patients are appropriately diagnosed with available clinical biomarkers [130]. This situation, as we may see, may be drastically changed by incorporating systems biology strategies to prognosis.
A first class of improvement of molecular diagnostics and prognostics of breast cancer relies on the systems analysis of metabolic pathways and systemic alterations such as the ones prescribed by obesity and metabolic syndrome (two common comorbidities of breast cancer). The study of Krebs et al. [131], for instance, presents a clear statistical association between measurements of adiposity and increased risk of breast cancer in aged women, while reference [132] presents a systems-level cohort study that also associates the functional role of adipokines, with the development of insulin resistance and metabolic syndrome, and later with breast cancer onset and recurrence.
In a more detailed-specific molecular model, Gunter and collaborators [133] analyzed the role that insulin and the insulin-like growth factor I (IGF-1) pathway processes have in the clinical risk of breast cancer in postmenopausal women. We may recall that systems biology studies have previously pointed out to IGF signaling as having a fundamental role in breast cancer tumorigenesis. Interestingly, recent studies in papillary thyroid cancer [35] have also highlighted the role that cross talk of apoptotic pathways and IGF signaling may have in oncogenesis [134]. Systemic metabolic deregulation analyses may also allow the development of population-specific biomarkers of breast cancer prognosis; for instance, we may mention the study of Alokail et al. [135] of Saudi women in which biomarkers of metabolic syndrome and stress response correlated quite well with early onset of breast cancer in this population. This study discusses how alterations in the levels of leptin and adiponectin were followed by an increase in breast cancer risk incidence, and they also mention adiponectin as having a prognostic significance in breast cancer recurrence. Omic technologies played an important role in their findings; in this case, serum insulin, adipocytokines, and plasminogen activator inhibitor-1 (PAI-1) concentrations were measured using a customized multiplex Luminex assay. Other low-throughput technologies were used: hypersensitive C-reactive protein (CRP), tumor necrosis factor-alpha (TNF-α), and angiotensin II (ANG II) were measured using ELISA.
Often prognosis is related to the degree and velocity of metastatic processes. Systems biology approaches have also identified molecular features or patterns that may result in prognostic tools for metastasis. Kim et al. [136] have recently discovered such molecular links between the presence of certain gene expression patterns and lymph node invasion in breast cancer tumors, and their findings even correlate with prognostics and survival estimates as well as with other studies [28, 70].
Important advances in breast (and in general in all) cancer prognosis deal with the discovery of molecular signatures, i.e., with a set of molecules or molecular states that may be measured by means of HTOTs or other laboratory techniques and after analysis may allow the determination of prognostic outcomes. Sophisticated mathematical and computational techniques have been developed to this aim [40, 51, 137, 138]. For instance, Kim et al. [136] discussed how a sudden increase in S1P, a growth-promoting lipid, triggered by the lack of p53, may be a regulator of proliferation, apoptosis, migration, and angiogenesis in breast tumor cells and is thus a potential therapeutic target.
We have already discussed the Gene Set Enrichment Analysis [40]. In reference [137], Carrivick and coworkers developed a statistical analysis tool based on the Bayesian correlation calculations in gene expression datasets from whole-genome microarray experiments. Since expression microarrays are established as powerful clinical aids (next to bed in some cases), such discoveries have enormous importance. Molecular signatures of breast cancer have allowed for prospective identification in some cases [51, 138], allowing large improvements in the clinical decision-making process, and have hence established themselves as fundamental tools, in particular for extremely aggressive metastatic phenotypes that often result in mortality because of misdiagnosis and poor prognosis and therapeutics.
In the particular case of breast tumors, one of the factors causing such poor prognostics and diagnostics is the strong heterogeneity of the tumor cells, a challenge for molecular pattern-recognition strategies. However, likely the strongest achievement of systems biology approaches to breast cancer has been the discovery of different tumor subtypes. The presence of one of these subtypes or the other depends on a number of molecular features such as patterns of DNA copy-number variations [139] that have an impact on both gene expression signatures and clinical/pathological aspects of the malignancy, histologic grades [140], and again gene expression signatures [15, 76]. There is a large, yet not comprehensive, set of subclassifications of breast tumor subtypes; however, the one by Perou and collaborators is perhaps the more consistent [15]. In Fig. 17.4, we present a quick view of such classification.
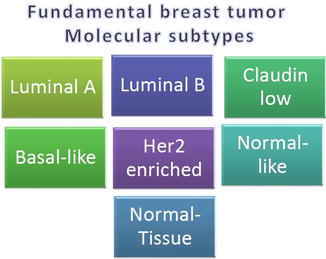
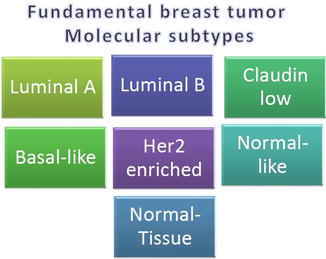
Fig. 17.4
Fundamental breast tumor molecular subtypes according to the classification of Perou et al. [15]. Tumor subtypes are color coded according with their approximate molecular likeness (i.e., closer color and brightness level indicate closer molecular profile). Color coding is, however, not quantitatively reliable, but is used just for illustrative purposes
Prognostics aside, the real concern of the clinicians is therapeutics. Also in this aspect, systems biology approaches may have resulted in significant advances. In Fig. 17.5, we can see an illustrative table of drugs designed as therapeutic agents to treat breast cancer and that instead of trying to induce generalistic responses (e.g., apoptosis) are designed to trigger (or to block) the action of specific pathways involved in breast cancer and its metastasis.