Fig. 31.1
Hallmarks of response to immune checkpoint blockade. Identification of predictors of response to immune checkpoint hinges on an understanding of the influence of numerous factors on cancer development and antitumor immunity, with “concentric spheres of influence” from the tumor itself, the tumor microenvironment, local immunity (such as in the peri-tumoral environment and draining lymph nodes), systemic immunity, and the local and extended environment. Forces affecting each of these areas are listed and are highly interrelated and interdependent. Ultimate responses to immune checkpoint blockade are the result of a complex tally of these positive and negative interactions; thus, all must be taken into account when considering predictive biomarkers for use in these therapies
31.2.1 Tumor and Microenvironment
31.2.1.1 Tumor Genomics and Epigenomics
Significant inroads have been made in the understanding of carcinogenesis and cancer progression through the use of next-generation sequencing techniques, which are now integrated into the fabric of clinical care. Targeted sequencing panels are being used worldwide across multiple cancer types and are helping to inform therapeutic decision-making—particularly to guide treatment with molecularly targeted therapy. However, genomic profiling is also highly relevant when considering responses to immune checkpoint blockade, as there is a growing list of molecular alterations implicated in antitumor immune responses [2–6].
The genomic characterization of tumors through efforts such as The Cancer Genome Atlas (TCGA), has delivered tremendous insight into the types and burden of mutations across different cancer types [7–10]. With regard to genomic mutations, both quality and quantity “matter” and may influence antitumor immunity and subsequent responses to immune checkpoint blockade. Perhaps one of the best illustrations of this is the concept that total mutational load may influence response to immune checkpoint inhibitors; in general, cancers with a higher mutation rate or with defects in DNA mismatch repair genes are much more likely to respond to immune checkpoint blockade [2, 11, 12]. This is likely due to an associated higher burden of neoantigens, defined as tumor-associated antigens arising from somatic mutational events; as these are not expressed by other “normal” tissues, they may be much more immunogenic due to the presence of neoantigen-reactive T cells that have likely not been tolerized. However it is important to note that a relatively small percentage of neoantigens that are predicted using conventional algorithms are actually expressed, and even fewer are likely to be capable of eliciting a meaningful antitumor immune response [13].
Mutations can be a “double-edged sword,” as they may also contribute to therapeutic resistance. There is a growing list of examples of genomic mutations and other genomic or epigenomic alterations that weaken responses to immune checkpoint blockade. Aberrant antigen processing and presentation machinery resulting from mutations, copy number alterations, or altered expression patterns of such molecules as HLA and β-2 microglobulin have been described [3, 14, 15]. It is also important to consider that oncogenic genomic or epigenomic alterations may be associated with downregulation of tumor-specific antigens, and therapeutic targeting of these alterations may result in enhanced antigen expression. This is exemplified by the effects of kinase inhibitors targeting V600-mutated BRAF in the case of melanoma [16, 17] and is also seen in the setting of epigenetic regulation of cancer testis antigen expression across cancer types [18, 19].
Other mutations or alterations associated with oncogenic progression may be associated with immunoregulatory effects of relevance to immune checkpoint blockade, for example, increased expression of programmed death receptor ligand-1 (PD-L1) driven by AKT-mTOR [20] or EGFR [21] pathway activation in lung cancers or induction of an immunosuppressive cytokine milieu by functional insufficiency of the tumor suppressor gene PTEN [4], resulting in impaired responses to immune checkpoint blockade. Defects in interferon signaling within tumors may also confer resistance to immune checkpoint blockade [3, 22].
31.2.1.2 Tumor Microenvironment
In addition to considering molecular alterations in tumors, one must consider the tumor microenvironment in modulating responses to therapy. Indeed tumors are complex ecosystems, consisting not only of tumor cells but also blood vessels, tumor-infiltrating immune cells, cytokines, and numerous other stromal components including fibroblasts, myeloid-derived suppressor cells, extracellular matrix, and other cellular entities. Together, these components contribute to carcinogenesis and may ultimately contribute to therapeutic response and resistance to immune checkpoint blockade.
A strong foundation delineating the diverse roles played by distinct functional elements of the tumor microenvironment arises from the seminal work of Hanahan and Weinberg as well as that of Schreiber, who described the “hallmarks of cancer” [1] and principles of immunoediting [23], respectively. Together, these describe mechanisms by which tumors grow and metastasize, as well as the intimate and dynamic interactions between tumor and immune cells, leading to tumor elimination, equilibrium, or escape [23].
This foundation was subsequently built upon by other investigators, who described the “cancer-immunity cycle” [24], the “cancer-immune set point” [25], and the “cancer immunogram” [26]. Recently, these concepts have been explored in the setting of checkpoint inhibitor therapy, and “hallmarks of response to immune checkpoint blockade” have also been described [27]. Each of these acknowledges the complex relationship between tumor cells and cells within the microenvironment as well as external influences and strives to demonstrate the influence of these on therapeutic responses. These are outstanding models, though our incomplete understanding of the complex processes is certainly acknowledged—and these and other models will be revised using an iterative approach as more data emerges.
A key feature in governing responses to immune checkpoint blockade is the ability (or inability) to exclude T cells from the tumor microenvironment itself. The presence of a T cell infiltrate within tumors has been known to correlate positively with prognosis across multiple tumor types for several years [28], and more recent studies have evaluated the relationship between the density and distribution of CD8+ T cells, including location at the tumor center or invasive margin, and response to immune checkpoint blockade [29]. Additional assessment of the tumor microenvironment using both architecture and gene expression profiling has helped classify tumors into several different phenotypes including “inflamed vs non-inflamed” [30], with later refinement of these phenotypes to “immune-desert, immune-excluded, or inflamed” [25]. Mechanisms of immune exclusion have been described and may originate in tumor cells themselves such as defects in Wnt/β-catenin pathways [5] or MAPK hyperactivation [31, 32]. Alternatively, immune exclusion may arise from other cellular components in the tumor microenvironment, such as myeloid-derived suppressor cells (MDSCs) [33], or from secreted factors such as vascular endothelial growth factor (VEGF) [34–36], interleukin-1 (IL-1), and interleukin-6 (IL-6) [37–39]. An appreciation of the contributions from each of these factors is paramount to designing adequate immune monitoring strategies in the setting of treatment with immune checkpoint blockade and is also important in the rational design of strategies to overcome therapeutic resistance.
31.2.2 Immunity
In addition to the tumor and microenvironment, overall host immunity plays a key role in modulating tumor growth, spread, and response to therapy. Immune responses are dynamic and are shaped by exposure to antigenic stimuli but are also influenced by factors within and outside the host (Fig. 31.1). Debris from dying tumor cells are taken up by host antigen-presenting cells (such as dendritic cells), processed and presented to CD4+ (helper) and CD8+ (cytotoxic) T lymphocytes, but additional signals (co-stimulatory versus co-inhibitory) and secreted factors are critical to determining the fate of this T cell-antigen interaction, resulting in either T cell stimulation and generation of an antitumor immune response or T cell anergy. T cell recognition of presented antigen is, however, only the first of many steps in the process, and immunoregulatory influences at any stage (priming, trafficking, tumor infiltration, T cell-tumor cell engagement), arising locally or from distant sites, may impair an otherwise effective immune response (Fig. 31.2).
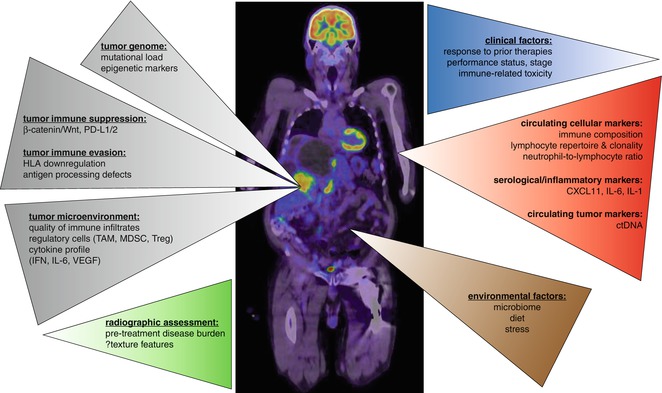
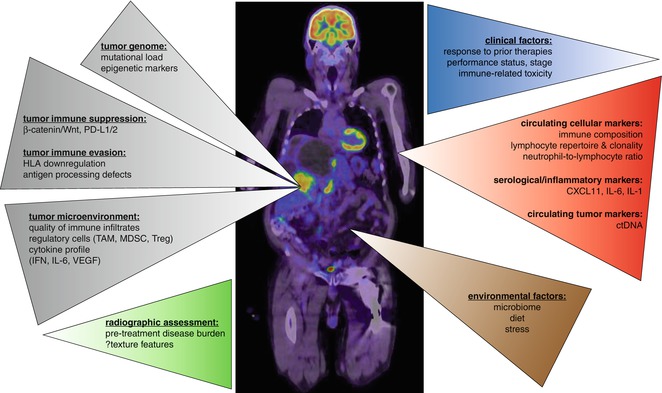
Fig. 31.2
Current and novel predictors of immune checkpoint blockade. Existing predictors of response to immune checkpoint blockade are predominantly focused on tumor-based assessments (gray) documenting tumor mutational and associated neoantigen burden, tumor cell-intrinsic immune suppression and evasion mechanisms, and microenvironmental factors, principally the presence or absence of an activated CD8+ T cell infiltrate. Emerging predictors extend upon these factors but are gradually broadening in scope to include historical factors as well as biomarkers sourced from other biocompartments; these include radiographic assessments (green), clinical factors (blue), blood biomarkers (red), and environmental influences, currently dominated by the effect of the gut microbiome (brown)
It has long been appreciated that overall host immunity may influence cancer development and progression, from reports of spontaneous regressions of cancer [40] to the concept of inducing immune responses against cancer by administering microbial products (Coley’s toxins) to patients beginning in the late 1800s [41, 42]. This is also substantiated by findings that patients with immunosuppression are at increased risk of developing several forms of cancer. These and other concepts are embodied in the seminal work of Schreiber in which the “three E’s” of immunoediting were described [23], highlighting the tight interaction between host immunity and tumor, albeit largely at the level of the tumor microenvironment.
There is now a growing appreciation of the influence of overall systemic immunity on tumor rejection and response to immunotherapy. Recent reports have helped to delineate the complex role of numerous cell types in multiple compartments of the body in generating an antitumor immune response, demonstrating that immune activation in the periphery is required and is heavily dependent on CD4+ T cells and that PD-L1 expression in the periphery is a major driver of immune escape [43]. Additionally, expression of 4-1BB [44] or PD-1 [45] is known to enrich for tumor-reactive T cells within both tumor and circulating lymphocytes, hinting at an as yet poorly understood immune circulation that may be relevant to the establishment and maintenance of the tumor-reactive T cell population. Given these findings, it is critically important to consider more general measures of host immunity when contemplating predictors of response to immune checkpoint blockade. However, ideal monitoring strategies for overall systemic immunity remain elusive and will require extensive development.
31.2.3 Environment
In addition to considering internal features such as the tumor, microenvironment, and general immunity, one must consider the influence of the wider environment in the setting of cancer development, progression, and treatment. These factors act in parallel with the local, or host immune parameters described above, and include physical influences from the extended environment such as ultraviolet radiation and carcinogen exposures (e.g., tobacco smoke), biological influences from the diet and microbiome, and neurophysiological influences from stress.
Environmental factors can have a significant impact on all domains affecting response to immune checkpoint blockade. One of the strongest examples of this is the influence of carcinogens on mutational load and response to immune checkpoint blockade, with patients suffering damage from UV exposure and smoking noted to have typically higher mutational load and enhanced responses to immune checkpoint blockade [46]. Environmental carcinogens are not the only example, as other factors of potential impact on antitumor immunity and response to immune checkpoint blockade are slowly being identified, including diet, stress [47], and the gastrointestinal microbiome [48, 49]. These have important implications not only for monitoring responses but also have significant potential therapeutic implications. This is highlighted by recent literature demonstrating that modulation of the gut microbiome can actually enhance therapeutic responses [48, 49] with clinical trials now being designed to capitalize on these insights.
31.3 Current and Emerging Predictors of Response, Resistance, and Toxicity
Based on an understanding of the complex influences on antitumor immunity, biomarkers have been and are being developed to predict response, resistance, and potentially even toxicity to therapy. It should be noted that there is currently a paucity of approved highly validated biomarkers at present, with the exception of PD-L1 and CD8—though each of these has limitations on its own. Nonetheless there is a large and growing list of putative predictors of response to immune checkpoint blockade that are currently being explored and tested, and these will be discussed herein.
31.3.1 Clinical Predictors of Response
Early clinical experience with checkpoint inhibitor agents raised concern that higher pretreatment burden of disease and more rapidly progressive disease were associated with a lower likelihood of response and poorer survival outcomes, due in part to the sometimes delayed onset of objective responses and low overall objective response rate observed in the setting of treatment with ipilimumab—the first checkpoint inhibitor to be approved in cancer therapy. This has not been recapitulated in the setting of treatment with immune checkpoint inhibitors targeting the PD-1 pathway or with combined CTLA-4 and PD-1 blockade—where response rates are higher and onset of response more brisk [50]. Nevertheless, disease burden clearly still plays a role, as baseline burden of disease (determined by radiologically assessable lesions), was independently prognostic of poorer overall survival in patients treated with the PD-1 inhibitor pembrolizumab [51], and was strongly inversely associated with survival in a small cohort of patients treated with ipilimumab in combination with bevacizumab [52]. These findings essentially confirm the adverse prognosis conferred by a larger burden of disease prior to checkpoint blockade without specifically identifying a predictive role. Furthermore, patients achieving a response by either RECIST or irRC criteria clearly have superior long-term outcomes to objective non-responders [53]; however, the prognostic and/or predictive value of additional imaging-based metrics such as density- and texture-based features remains to be determined and may ultimately require complex assessment criteria unique to distinct tumor types and sites of disease.
Prior durable response to ipilimumab appears to predict for benefit from subsequent treatment with pembrolizumab [54], but increasing evidence confirms that patients refractory to prior immune checkpoint blockade targeting CTLA-4 may still respond to subsequent challenge with anti-PD-1-based therapy and vice versa [55, 56]. Larger cohort data is required to determine the effects of confounding factors such as the interval between separate checkpoint blockade agents, the significance of sequential therapy without clear evidence of resistance to the first agent, post-progression fitness and disease burden, as well as subtle differences in toxicity profile arising from treatment sequence [56]. In general, it appears that prior treatment per se may not be predictive of immune checkpoint blockade response, but instead correlates with (and is confounded by) independently prognostic variables such as disease stage and performance status.
31.3.2 Tissue-Based Predictors of Response
The majority of research aiming to identify predictors of checkpoint blockade efficacy has focused on analysis of the tumor itself. Tumor-intrinsic factors that lead to immunogenicity are well described, arising ultimately from the genomic instability characteristic to all cancers and resultant somatic mutational events which may produce altered peptides, protein expression patterns, peptide processing/display machinery, and responses to immune attack.
Conceptually, predicting the antigenicity of a tumor is difficult to assess in the context of checkpoint blockade, given that the underlying T cell repertoire being targeted by therapy, and the antigens to which these T cells may be reactive, is unknown. Furthermore, the T cell repertoire is highly diverse and partially redundant, comprised of innumerable unrelated TCR sequences, many of which may be reactive to the same peptide epitope. Computational methods to predict protein targets from TCR sequence data alone are lacking and additionally hampered by the largely private T cell repertoires between patients. However, given the relationship between neoantigen burden and inherent antigenicity of a tumor, the underlying somatic mutational burden of a tumor has been found to correlate with response to both CTLA-4 and PD-1 blockade. Despite this, profiling of the mutational landscape in large cohorts of tumors reveals that consistently, a large range of mutational burden exists across tumor types [10], and somatic mutational load lacks sufficient negative predictive value as a biomarker of immune checkpoint response.
Unsurprisingly, the most heavily investigated biomarker for PD-1 and PD-L1 inhibitors is PD-L1 expression on the tumor itself. In advanced melanoma, positive PD-L1 expression is clearly predictive of improved response to PD-(L)1 targeted therapy; however, low or absent PD-L1 expression does not entirely preclude therapeutic response, leading to significant reticence toward its widespread strict application as a treatment selection tool [57, 58]. Elements of specific tumor biology likely also impact on the utility of direct target biomarkers like PD-L1. For example, in non-small cell lung cancer, tumoral expression of PD-L1 demonstrated a continuous predictive association with response and survival following nivolumab treatment in non-squamous patients [59] but appeared to lack either prognostic or predictive value in a large cohort of squamous NSCLC patients treated with the same agent [60]. Precise mechanisms for this stark contrast remain to be defined but may reflect etiological differences (e.g., smoking history) and both burden and type of cumulative mutational load.
Direct assessment of the intratumoral immune landscape has also been employed as a biomarker discovery strategy. In anti-CTLA-4-treated patients, higher baseline expression of FOXP3 and IDO was associated with clinical response, as was an increase in TIL from baseline to 3 weeks after treatment initiation. Exploratory gene expression profiling found increases in expression of genes associated with immune response, and decreased cancer-associated genes, consistent with expectation [61]. Multiparameter flow cytometric analysis of TIL from fresh melanoma samples demonstrated that the frequency of PD-1+ CTLA-4+ CD8+ T cells prior to initiation of anti-PD-1 therapy was highly predictive of response and progression-free survival; this was associated with accumulation of an activated, and relief of an exhausted, CD8+ T cell phenotype in TIL populations during treatment [62].
The use of baseline predictors of response is increasingly being supplemented with the use of early on-treatment markers in light of data demonstrating the superior utility of longitudinal tumor assessment in tumor [14]. In a study of baseline and longitudinal tumor biopsy samples from melanoma patients, pretreatment T cell enrichment in TIL was associated with response to PD-1 inhibitor treatment regardless of prior CTLA-4 inhibitor therapy; however, early on-treatment biopsies displaying enrichment of T cell infiltrate, immunomodulatory marker expression (PD-1, PD-L1, LAG3), and, particularly, FOXP3 and granzyme B expression were highly associated with likelihood of response [14].
Importantly, the tumor-immune interaction likely evolves in parallel with the process of cellular transformation, and as such, a substantial degree of adaptation and evolution has already occurred long before the clinical diagnosis, or scientific interrogation, of a tumor. Implicit in this is the notion that the mechanisms which may lead to failure of any immunotherapy strategy, including checkpoint inhibition, may be selectively driven by that therapy but may also have spontaneously arisen prior to therapy, in response to preexisting immune pressure. Thus, much can be considered directly transferrable from our knowledge of immune checkpoint blockade resistance mechanisms into the treatment-naïve setting and thus used as predictive factors.
The central role of interferon signaling in shaping the tumor-immune interface is well described, with IFN-γ critically important for the establishment of a suitably inflamed tumor microenvironment but equally important for the emergence of immune checkpoint-driven adaptive resistance. Due to the presence of this high-level negative feedback mechanism, it is not surprising that the role of IFN in antitumor immunity can be confusing, promoting aspects of both tumor cell destruction and survival. Mutations in IFN signaling pathway components have been identified as molecular mechanisms of checkpoint blockade resistance in melanoma; JAK1 and JAK2 mutations not identified in pretreatment samples of patients who initially responded, but subsequently progressed while receiving anti-PD-1 therapy, have been shown to directly facilitate tumor cell resistance to IFN-mediated growth suppression and apoptosis [3]. Similar mutations were subsequently demonstrated to result in lack of response in patients previously untreated with anti-PD-1 therapy [63].
General immune exclusion may also predict for a lack of immune checkpoint inhibitor response, in keeping with the necessity of immune effector cells (principally tumor-specific T cells) to physically engage with or enter the tumor in order to facilitate tumor killing. Several mechanisms of tumoral immune exclusion have been identified including tumor cell expression of β-catenin [5]; however, firm evidence of specific molecular mechanisms predicting success or failure of immune checkpoint inhibition in patient samples is lacking.
31.3.3 Blood-Based Predictors of Response
Significant interest is emerging in the use of cell-free circulating DNA as a noninvasive marker that can be used to monitor the response to treatment, typically by quantitating mutated DNA originating uniquely from tumor cells (circulating tumor DNA, ctDNA). In melanoma and colorectal cancer, ctDNA has been shown to vary in accordance with disease burden measured by more conventional means (e.g., radiologically) with the distinct advantage of potentially identifying changes in response status (either response or progression) significantly earlier than clinico-radiological assessment [64–67]. Whether this can be exploited as an early clinical decision-making trigger to improve long-term treatment outcomes is not yet known. Additionally, although preliminary evidence suggests at least a correlation between dynamic ctDNA alterations early during therapy and eventual treatment outcome [64], the potential clinical use of ctDNA as a predictive marker for immunotherapy has not been defined. As highly recurrent tumor mutations are found in a minority of cancer patients overall, future interest lies in the identification of personalized, sequence-independent tumor markers such as aberrantly methylated ctDNA as a means of noninvasive monitoring. Given the increasingly apparent role of epigenetic changes in modulating the response to immune checkpoint blockade, it will be of significant interest to see whether methylation-specific ctDNA assessment of molecular targets known to influence immunotherapeutic outcomes can be devised as predictive assays in this setting. Other serological markers specifically predictive of checkpoint blockade response have not yet been identified. In one study, independent and clearly clinically useful markers were not identified other than LDH, which is itself more prognostic than predictive [68].
Similarly to tumor biopsy-based analyses, circulating immune markers have been studied prior to and early after initiation of immune checkpoint blockade. In several retrospective studies, pretreatment neutrophil-to-lymphocyte ratio (NLR) has been shown to be an independent predictive factor of ipilimumab benefit (lower NLR predictive of improved PFS and OS) although this would be a difficult metric to apply to patients requiring steroids, and no consistent optimal NLR cutoff to stratify patients into “good” or “bad” responders has yet been agreed upon [69–71]. PD-1 expression on CD4+ T cells in peripheral blood is negatively prognostic in NSCLC and correlated inversely with response to anti-PD-L1 therapy in a small cohort of patients [72]. Conversely, elevated circulating regulatory T cell numbers during treatment are associated with non-response to PD-1 inhibitor treatment [55], while baseline levels of the chemokine CXCL11 and—less robustly—soluble MHC class I-related chain A (sMICA) may be predictive of poor survival in anti-CTLA-4-treated patients [73]. Also of predictive value early after starting therapy, an increased frequency of inducible T cell co-stimulatory (ICOS)-expressing CD4+ T cells in peripheral blood has been shown to be a reproducible biomarker of anti-CTLA-4 therapy efficacy after as few as one or two doses [74]. Early on-treatment elevation in absolute lymphocyte count and eosinophil count may also be predictive of improved overall survival in patients treated with ipilimumab [75].
31.3.4 Noninvasive Predictors of Response
Efforts are underway to establish novel imaging techniques to help predict responses to immunotherapy; however, the use of noninvasive imaging has thus far been limited in routine clinical practice to staging investigations performed at baseline and throughout treatment. Notwithstanding the prognostic and potentially predictive value of imaging-based assessments of burden of disease prior to treatment initiation, standard radiologic assessments based on the response evaluation criteria in solid tumors (RECIST), while extensively validated across tumor types and treatment modalities, were found to be misleading in a small proportion (3–15%) of patients treated with immunotherapy due to the unique mechanism of action and response kinetics inherent to this treatment type [53, 76]. The phenomenon of pseudo-progression, in which therapy-related tumoral inflammation may temporarily lead to an increase in size of evident lesions and appearance of apparently new lesions at sites of previously occult micrometastatic disease, belies the potential for subsequent response in and/or disappearance of these lesions once the antitumor immune response progresses and ultimately subsides. To account for these “atypical” patterns of response, a new set of imaging-based assessment criteria were proposed, dubbed the “immune-related response criteria” (irRC), being also applicable to other forms of immunotherapy based on a common tumoral inflammation mechanism of action [76].
The necessity to derive new criteria in the form of irRC suggests that radiologic assessment is inherently a flawed monitor and worse predictor of response in checkpoint blockade-treated cancers. It must be noted, however, that atypical radiological patterns of response are uncommon. In standard clinical settings, radiologic response is commonly obtained only 10–12 weeks after the initiation of immune checkpoint blockade therapy, and any response at that time may be unreliable until confirmed upon repeat scanning at least 4 weeks later. Indeed, cases of profoundly delayed responses occurring with an onset of 6–9 months after the initiation of therapy have been noted.
However, novel imaging-based metrics are under development which may afford clinically useful insights for diagnosis and for therapy, either prior to treatment commencement or at early on-treatment time points, potentially facilitating prompt changes in management in patients who appear destined not to respond to their current treatment regimen [77]. In a cohort of patients treated with ipilimumab and the anti-angiogenic agent bevacizumab, tumoral density features were incorporated into radiologic assessment using the Choi criteria; unfortunately changes in tumor density during treatment were not predictive of response [52]. In a modest cohort of patients treated with pembrolizumab on the KEYNOTE-001 protocol, both tumor size and density, or combined metrics (e.g., Choi or modified Choi criteria) were associated with overall survival [78]. Notably, density features suggest yet more complex patterns of response than can be assessed by either RECIST or irRC, thus opening the door wide to future development of radiomic-/texture-based features as putative prognostic or predictive features in the setting of immune checkpoint blockade.
31.3.5 The Gut Microbiome as a Predictive Biomarker
An emerging field of major significance to cancer immunotherapy is the study of commensal microbiota and their influence on checkpoint inhibitor responses, principally driven by the gut microbiome. In a seminal translational study of the gut microbiota using animal models and fecal microbial transplants from CTLA-4 inhibitor-treated patients, three key observations were made: firstly, response to therapy was clearly linked to the abundance of specific Bacteroides species in the gut; secondly, CTLA-4 inhibitor treatment in patients altered the gut microbial composition; and thirdly, CTLA-4 inhibitor efficacy was dependent on the presence of microbe-specific T cell responses [49]. In another study, intestinal Bifidobacterium was associated with stronger spontaneous antitumor responses in mice, and specific microbial “supplementation” independently phenocopied—or synergized with—PD-L1 checkpoint blockade [48]. Similarly, differences in gut microbial composition and diversity were identified between responders and non-responders to PD-1 inhibitor therapy [79]. Although the gut microbiome presents a clear interface between environment, diet, host immunity, and antitumor immunity, the precise mechanisms by which this interaction acts to modulate immune checkpoint inhibitor response are yet to be fully elucidated and will likely prove to be complex.
31.3.6 Predictors of Toxicity
Based on the imperfect tumor specificity of immune checkpoint blockade therapies, off-target immune stimulation leading to immune-related toxicities (immune-related adverse events, irAEs) is common. Anecdotally, the occurrence of irAEs has frequently been seen as a surrogate for the likelihood of antitumor immune stimulation; however, reported data regarding a putative association between irAE occurrence and antitumor clinical benefit in the setting of CTLA-4- and PD-1-directed therapy are conflicting across tumor types [80–83].
Given the potential severity of many irAEs, identification of reliable predictors of toxicity is equally as important as predictors of response; however, none have been identified thus far. Mechanistically, it is thought that preexisting autoreactive T cell clones, present at likely low frequencies, are subject to checkpoint blockade-induced reactivation leading to autoimmune toxicities. Low pretreatment prevalence of such clones, combined with the current lack of any technology able to reliably predict the antigen target of a given T cell receptor based on sequence information alone (other than a very limited number of previously described sequence-target relationships), makes the prospective identification of autoreactive T cell populations effectively impossible. Furthermore, it is not yet known why some of these preexisting but suppressed/tolerized clones are induced to reactivate, while presumably more numerous other clones do not. In a study of whole blood gene expression profiling in CTLA-4 inhibitor-treated patients, potential predictive signatures of GI toxicity were identified, primarily in on-treatment samples; however, independently predictive genes were not [84].
31.4 Static Versus Dynamic Assessment of Systemic and Antitumor Immunity
Conventional analysis of biomarkers of response to cancer therapy has traditionally hinged on analysis of static time points in tissue or blood samples—typically taken just before the start of therapy. However, limitations with this approach exist, as analyzed samples may be archived for a significant period of time—thus biomarkers may not necessarily be accurate if measured in temporally distant samples due to evolution of genomic and immune profiles over time. This, together with spatial heterogeneity and sampling error, may account for some of the modest predictive value of pretreatment biomarkers such as PD-L1. Additionally, static assessment of a pretreatment sample does not provide a complete picture given the dynamic nature of the antitumor immune response.
As evidence regarding predictive biomarkers of response to immune checkpoint blockade grows, it is becoming evident that analysis of adaptive immune signatures in early on-treatment samples (or changes from pre- to on-therapy) may be far superior in their predictive power, reflective of the dynamic state of the tumor microenvironment (Fig. 31.3). This is exemplified in several recent studies, including one demonstrating that immune profiling via immunohistochemistry and gene expression profiling in early on-treatment samples revealed robust biomarkers of response, whereas few biomarkers were evident on analysis of pretreatment samples alone [14]. Similar dynamic findings have been noted in several other studies investigating both tissue-based and blood-based profiling [29, 62, 85]. Together, these studies suggest that consideration should be made for a new paradigm in immune monitoring, with routine assessment of early on-treatment responses to help guide therapeutic strategies—at least until better pretreatment biomarkers are identified (Fig. 31.3). Importantly, such analyses should be incorporated into contemporary clinical trial design—and ultimately may even be incorporated into standard of care therapy (Fig. 31.4).
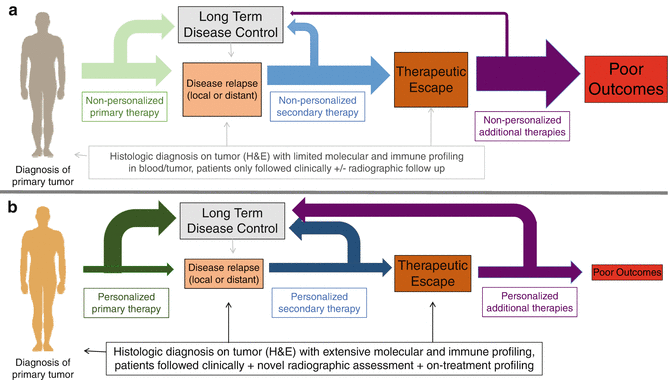
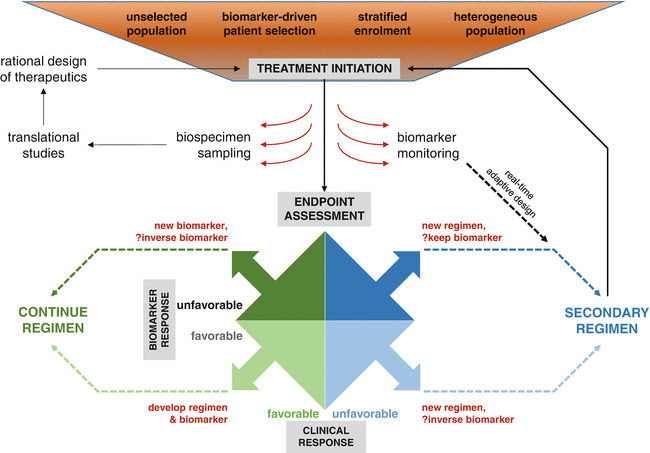
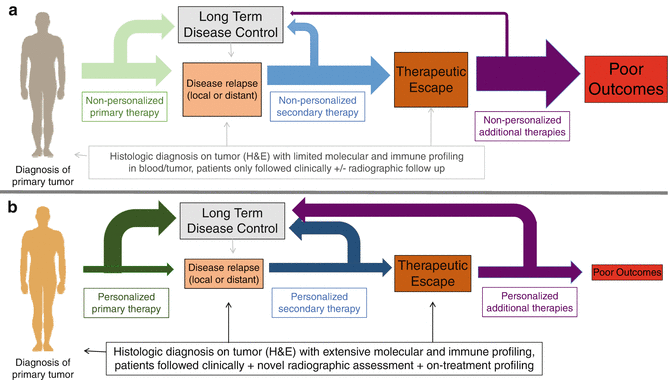
Fig. 31.3
(a) Importance of dynamic and static markers for checkpoint inhibitor blockade. In conventional cancer therapy, diagnosis of a primary tumor is followed by an evaluation of the tumor via histologic diagnosis with limited molecular and immune profiling in tumor +/−blood. Non-personalized primary therapy is then chosen empirically, with patients followed clinically and potentially with radiographic follow-up. In this scenario, a significant proportion of patients experience disease relapse, and a secondary form of non-personalized therapy is initiated—again with minimal to no biomarker assessment. Again, few patients respond and the majority experience therapeutic escape with poor outcomes overall. (b) In innovative approaches to cancer therapy, histologic diagnosis of a primary tumor is augmented via molecular and immune profiling at baseline. Personalized primary therapy is chosen based on insights gained, and a higher proportion of patients achieve long-term disease control. Disease relapse occurs in a smaller proportion of patients, and iterative approaches to personalized secondary therapy are utilized incorporating molecular and immune profiling of the tumor at relapse as well as on-treatment profiling of tumor and blood. Fewer patients experience disease escape, and when this does occur, responses to additional personalized approaches are higher based on a more customized treatment strategy. With this approach, far fewer patients experience poor outcomes to therapy
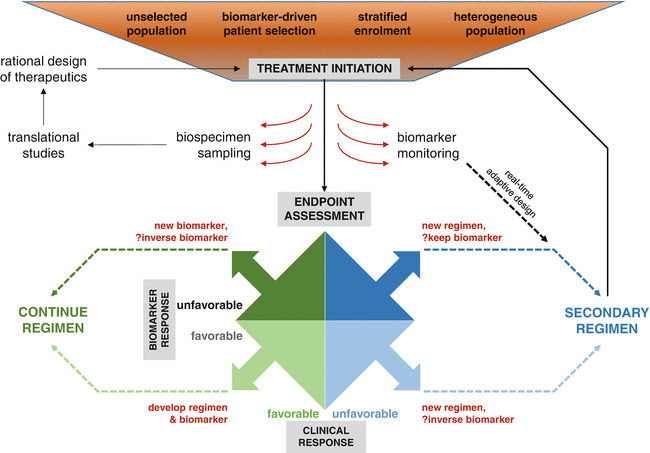
Fig. 31.4
Incorporating biomarkers into clinical trial designs for immuno-oncology. Existing biomarkers may be used to guide patient selection and/or enrolment into clinical trials, with validated biomarkers providing predictive information to inform treatment assignment. Alternatively, prognostic and predictive markers may be used to stratify subject enrolment in order to optimize translational and clinical outcomes. Intentional enrolment of heterogeneous populations may be desirable in order to remain essentially therapeutically “agnostic” and identify biomarkers of response that are independent of tumor histology. Patients being treated on clinical trials may undergo biospecimen collection procedures prior to and during therapy, with significant emphasis on longitudinal sampling to provide vital information about dynamic changes while on therapy, including the development of resistance. Adaptive designs incorporating “on-the-fly” biomarker monitoring may facilitate early, biomarker-directed, therapy changes in patients who appear unlikely to respond. Alternatively, achievement of a relevant clinical endpoint may trigger correlative analysis of clinical and biomarker outcomes, leading to critical decisions regarding acceptance or rejection of the biomarker, the treatment regimen, or both. In discordant cases, the biomarker may be found to be predictive of treatment failure and could be subsequently validated as an inverse treatment assignment biomarker. In patients failing to achieve clinical benefit, secondary or subsequent regimens may be chosen ad hoc or adaptively in light of biomarker information
31.5 Building Predictors into Clinical Trials and Standard of Care Therapy
The continued success of immune checkpoint blockade strategies will be realized through the maximization of efficacy and minimization of immune-related toxicity, both of which will require careful focus on biomarker discovery. Due to the current lack of robust or universal predictors of non-response, “real-world” clinical practice is as yet unable to function in the same way that clinical trials do, with their typically “responder-enriched” populations. Substantial efforts are required to ensure that the reach of immunotherapy is extended by ongoing clinical trial research, rather than increasingly focused on patient populations already known to have greater likelihood of benefit.
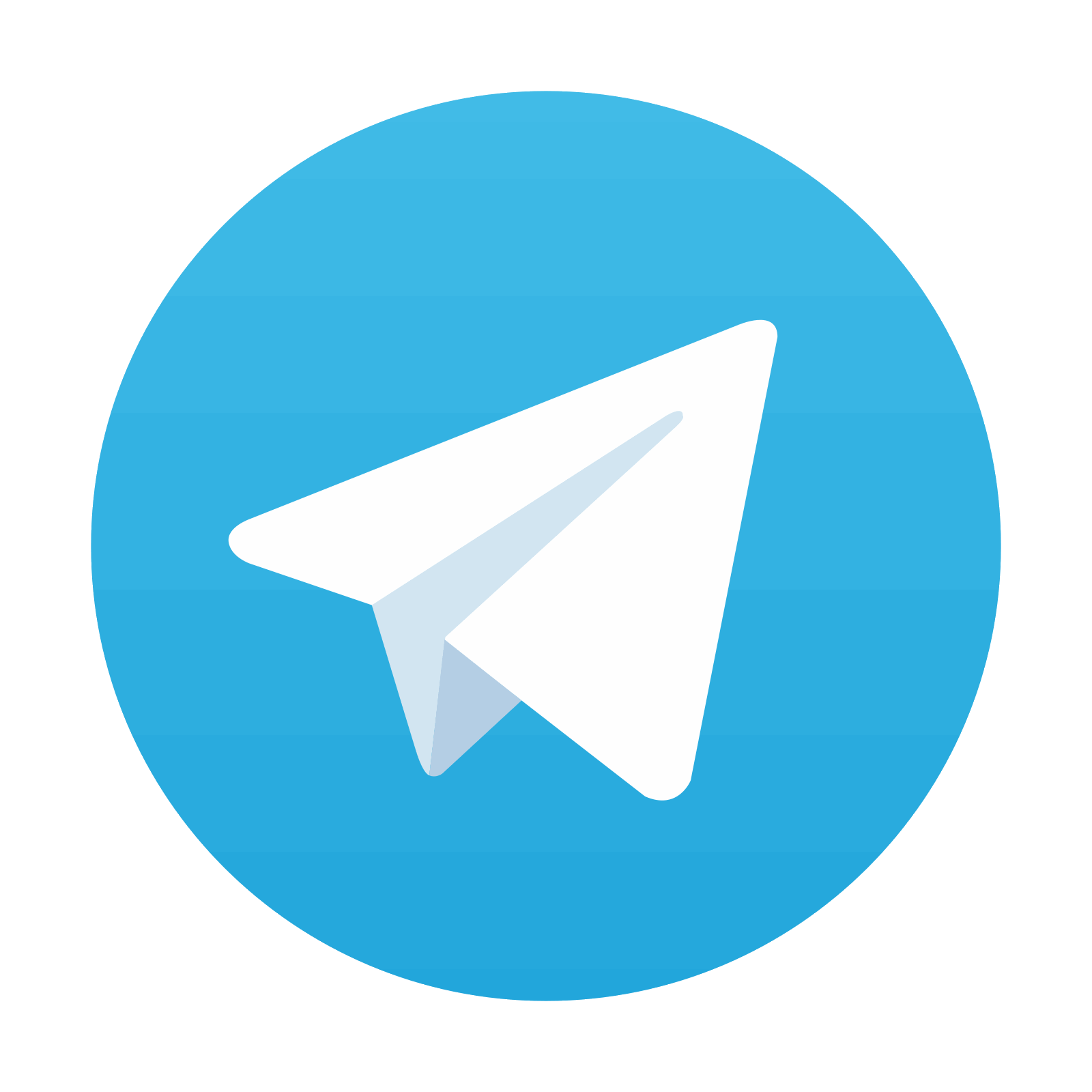
Stay updated, free articles. Join our Telegram channel

Full access? Get Clinical Tree
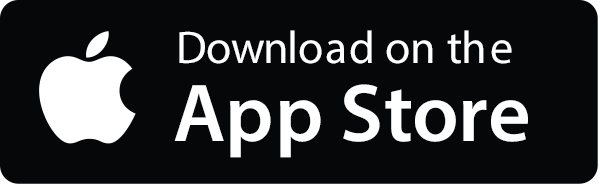
