Artificial neural networks, prediction tables, and clinical nomograms allow physicians to transmit an immense amount of prognostic information in a format that exhibits comprehensibility and brevity. Current models demonstrate the feasibility to accurately predict many oncologic outcomes, including pathologic stage, recurrence-free survival, and response to adjuvant therapy. Although emphasis should be placed on the independent validation of existing prediction tools, there is a paucity of models in the literature that focus on quality of life outcomes. The unification of tools that predict oncologic and quality of life outcomes into a comparative effectiveness table will furnish patients with cancer with the information they need to make a highly informed and individualized treatment decision.
- •
The contemporary patient is a savvy consumer of medical goods and services with information requirements that typically extend beyond that which is provided by physicians limited by the demands of modern-day clinic schedules.
- •
Prediction tools function to replicate the critical synthesization of data regularly conducted by physicians in a succinct, reproducible, unbiased, and evidence-based format that can be seamlessly incorporated into the patient visit.
- •
Current models have been shown to accurately predict a wide variety of oncologic outcomes, but there is an emerging need for the development of prediction tools that address quality of life end points.
- •
The incorporation of multiple validated prediction models into comparative effectiveness tables, which provide a side-by-side comparison of medical options with respect to the expected benefits and harms of therapy, can provide patients with the high-quality informed consent that they require to make a fully informed treatment decision.
Introduction
Now more than ever, patients who receive a diagnosis of cancer have the potential to be overwhelmed with the abundance of decisions presented to them for oncologic therapy. Long gone are the times when the therapeutic options were limited simply to such alternatives as surgery or chemotherapy. Rather, with the advent of novel chemotherapeutic regimens, intensity-modulated radiation therapy protocols, and advances in surgical technique, particularly with the evolution of minimally invasive approaches, the oncologic patient might be presented with choices A through G as opposed to merely A and B. As the number of available therapeutic options expands, the amount of diagnostic and prognostic information that is pertinent to the patient grows concurrently.
The contemporary patient is a savvy consumer of medical goods and services, and patients are no longer approaching medical visits ready to take notes with a blank sheet of paper. Quite the opposite, the modern visit to the physician’s office is often accompanied by knowledge gathered from the anecdotal information of friends and family, in addition to the overabundance of information available by way of a basic Internet connection. On-line forums allow a patient to connect with others with similar diagnoses who, previously worlds apart, now are united by a few simple keystrokes. Although these facts and figures (sometimes valid, oftentimes not) are considered invaluable to the patient with cancer teeming with anxiety and angst over a new diagnosis, the burden falls on the physician to sift through this newfound information and provide an accurate and unbiased representation of prognosis, diagnosis, and therapy. It is this critical synthesization of data that patients rely on most when making treatment decisions.
Unfortunately, despite the wide range of information provided to patients, the evidence demonstrates that physicians often fall short of meeting the information needs and expectations of patients. There is widespread recognition that patients who are better-informed before therapy experience improved psychosocial outcomes after therapy. Several studies have indicated that patients would prefer to receive even more information and physician guidance than is generally presented to them, and accurate estimates of the likelihood of treatment success, complications, and morbidity are critical to patients seeking to make fully informed treatment decisions. However, in the current state of medicine, the limitations placed on physicians with crowded clinic schedules and constrained counseling time are not minor, and appropriately navigating the landscape of limited time with increased expectations requires that physicians maximize efficiency and at the same time adequately address the concerns of the patient.
Artificial neural networks, prediction tables, and nomograms
Conventionally, risk estimations and outcomes prognosis have been based primarily on physician judgment. However, physician estimations are inherently fraught with unintentional and intentional bias, and interest has grown in the development of prediction tools that can provide patients with a concise and balanced representation of their diagnostic and prognostic outcomes. Many of these instruments have developed into “bedside” tools that can be used expeditiously and provide patients with information in an easily digestible format. Examples of prediction tools that have been popularized include artificial neural networks (ANN), probability tables, and nomograms.
ANN are computer-generated algorithms that function to assimilate information in the same way that a brain does in response to various inputs. Although the algorithmic generation of the ANN is beyond the scope of this article, generally the ANN is comprised of several layers of information, and these layers, which consist of discrete neurons or knots, are bound together by various links along with signals travel. The output generated depends on the various inputs received from the input layers and has the advantage of incorporating multiple complex variable relationships. The ANN model has been used in areas of oncology including breast, gastric, and prostate cancer for diagnosis and prognosis but has been largely supplanted by nomograms and prediction tables because of the latter’s improved accuracy and ease of incorporation into modern medical practice.
Prediction tables have the benefit of providing outcome measures in an easily interpretable format that can be presented and applied to any patient in clinic. Typically, the prediction tables incorporate relevant pretreatment variables and provide individualized risk estimates based on the variables that each patient brings to the table. This theory of individualized medicine (outcomes based on a patient’s personal clinicopathologic parameters) is immensely more attractive than receiving an overarching percentage chance of cure based on a clinical trial comprised of patients with widely varied disease states. One of the primary drawbacks of prediction tables lies in their inherent inability to keep variables that are continuous in clinical practice (eg, serum prostate-specific antigen [PSA]) continuous in the model. By categorizing those continuous variables and placing them into discrete boxes, one assumes that risk is constant within groups and increases sharply at categorical boundaries, and in the process, part of the intrinsic predictive value of the variable is wasted. This is a shortcoming that is not shared with nomograms, and several studies have identified an advantage in predictive accuracy in nomograms compared with prediction tables.
Nomograms are a graphical representation of a mathematical formula. Regardless of the statistical methods used (most often multivariate logistic regression or Cox proportional hazards analysis) the development of a nomogram includes multiple clinical parameters, which may be either continuous or categorical, each of which is represented by a scale based on its statistical impact on the specified outcome. The scales for each statistically significant predictive variable are then plotted in a visual format such that each corresponds to a numerical value; the tally of these values among all variables can then be converted into the probability of reaching the outcome. The use of nomograms in clinical practice offers the advantage of condensing a complicated mathematical formula into a simple and easily interpretable format and one that can be applied efficiently at the time of a patient visit, thereby providing a wealth of information in a short period of time. The nomogram essentially functions to replicate the synthesization of data the physicians conduct on a daily basis in a reproducible, unbiased, and evidence-based format that can be seamlessly incorporated into each patient visit. Furthermore, although nomograms function to reproduce clinical judgment, their predictive value has been shown to outperform the predictions of clinicians in some circumstances.
Artificial neural networks, prediction tables, and nomograms
Conventionally, risk estimations and outcomes prognosis have been based primarily on physician judgment. However, physician estimations are inherently fraught with unintentional and intentional bias, and interest has grown in the development of prediction tools that can provide patients with a concise and balanced representation of their diagnostic and prognostic outcomes. Many of these instruments have developed into “bedside” tools that can be used expeditiously and provide patients with information in an easily digestible format. Examples of prediction tools that have been popularized include artificial neural networks (ANN), probability tables, and nomograms.
ANN are computer-generated algorithms that function to assimilate information in the same way that a brain does in response to various inputs. Although the algorithmic generation of the ANN is beyond the scope of this article, generally the ANN is comprised of several layers of information, and these layers, which consist of discrete neurons or knots, are bound together by various links along with signals travel. The output generated depends on the various inputs received from the input layers and has the advantage of incorporating multiple complex variable relationships. The ANN model has been used in areas of oncology including breast, gastric, and prostate cancer for diagnosis and prognosis but has been largely supplanted by nomograms and prediction tables because of the latter’s improved accuracy and ease of incorporation into modern medical practice.
Prediction tables have the benefit of providing outcome measures in an easily interpretable format that can be presented and applied to any patient in clinic. Typically, the prediction tables incorporate relevant pretreatment variables and provide individualized risk estimates based on the variables that each patient brings to the table. This theory of individualized medicine (outcomes based on a patient’s personal clinicopathologic parameters) is immensely more attractive than receiving an overarching percentage chance of cure based on a clinical trial comprised of patients with widely varied disease states. One of the primary drawbacks of prediction tables lies in their inherent inability to keep variables that are continuous in clinical practice (eg, serum prostate-specific antigen [PSA]) continuous in the model. By categorizing those continuous variables and placing them into discrete boxes, one assumes that risk is constant within groups and increases sharply at categorical boundaries, and in the process, part of the intrinsic predictive value of the variable is wasted. This is a shortcoming that is not shared with nomograms, and several studies have identified an advantage in predictive accuracy in nomograms compared with prediction tables.
Nomograms are a graphical representation of a mathematical formula. Regardless of the statistical methods used (most often multivariate logistic regression or Cox proportional hazards analysis) the development of a nomogram includes multiple clinical parameters, which may be either continuous or categorical, each of which is represented by a scale based on its statistical impact on the specified outcome. The scales for each statistically significant predictive variable are then plotted in a visual format such that each corresponds to a numerical value; the tally of these values among all variables can then be converted into the probability of reaching the outcome. The use of nomograms in clinical practice offers the advantage of condensing a complicated mathematical formula into a simple and easily interpretable format and one that can be applied efficiently at the time of a patient visit, thereby providing a wealth of information in a short period of time. The nomogram essentially functions to replicate the synthesization of data the physicians conduct on a daily basis in a reproducible, unbiased, and evidence-based format that can be seamlessly incorporated into each patient visit. Furthermore, although nomograms function to reproduce clinical judgment, their predictive value has been shown to outperform the predictions of clinicians in some circumstances.
Examples of clinical prediction tools
Predicting Pathologic Stage in Prostate Cancer
Although most men diagnosed with prostate cancer have clinically organ-confined disease (clinical stage T2 or less), some evidence suggests that more than 50% of patients who go on to radical prostatectomy have pathologic evidence of extraprostatic spread of the disease and thus may require multimodal therapy for any chance of cure. Partin and colleagues devised a series of tables based on clinical T stage, serum PSA, and grade of prostatic adenocarcinoma by transrectal ultrasound-guided biopsy that provides percentage estimates of pathologic stage, including organ-confined disease, extracapsular extension, and invasion of the seminal vesicles to help guide treatment decisions; the tables have since been updated to reflect more contemporary clinicopathologic data. These tables have come to be known as the “Partin tables,” and were one of the first ubiquitous prediction models in prostate cancer, which has now experienced a surge in the number of available prediction tools.
Nomogram Predicting Malignancy in Thyroid Nodules
With the continual progress of radiologic techniques, an increasing number of subclinical thyroid nodules are being detected. The appropriate management of these nodules is controversial, because only a small percentage are truly malignant and the limitations of an often nondiagnostic percutaneous fine-needle aspiration are well-recognized. Thus, preemptive surgical excision likely overtreats most nodules, whereas a protocol of observation until the appearance of more definitive signs of malignancy delays curative treatment for an unpredictable period of time. To tackle this clinical dilemma, Nixon and colleagues developed a nomogram based on clinical, biochemical, radiologic, and pathologic features to predict the risk of malignancy in thyroid nodules. Although the counseling physician certainly could take the time to explain the relevance of thyroid-stimulating hormone levels, nodule echo-texture, and nodule vascularity and how those variables affect risk of malignancy, the clinical nomogram provides a visual representation from which patients can see with their own eyes what factors have the most bearing on the outcome of interest. Additionally, patients can be reassured that the nomogram produces a result that is individualized based on his or her clinicopathologic parameters and does not contain anecdotal bias.
Preoperative and Postoperative Nomograms Predicting Disease Recurrence after Radical Prostatectomy
In terms of cancer screening and posttherapeutic surveillance, the advent of the PSA era has permanently transformed the field of urologic oncology. By increasing the lead time diagnosis of prostate cancer, there has been an expected decline in the average age at diagnosis for the disease worldwide, and an increasing number of men are surviving multiple decades as opposed to multiple years after radical prostatectomy in what has historically been a disease of older men. With PSA now serving as the primary mode of postprostatectomy surveillance, a rising PSA after definitive therapy has emerged as a new end point for oncologic recurrence before symptomatic disease and has been termed “biochemical recurrence.” Seeking to characterize postoperative outcomes before radical prostatectomy, our group first developed a nomogram predicting a patient’s preoperative risk of biochemical recurrence based on tumor grade, PSA level, and clinical stage. The nomogram has since been updated to include other preoperative variables, such as biopsy cores positive for cancer, and a postoperative version has also been developed to further aid in patient counseling ( Fig. 1 ). Thus, this predictive model provides patients with critical information regarding prognostic outcomes after definitive therapy for adenocarcinoma of the prostate with performance that has been validated externally in multiple patient cohorts.
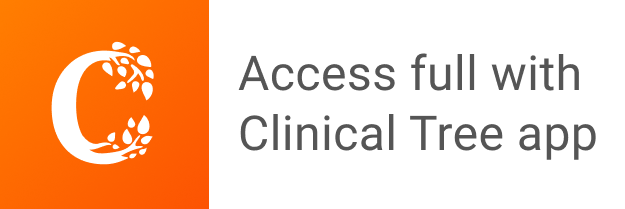