In 2013, an estimated 1.7 million people in the United States will be newly diagnosed with cancer.1 With advances in multidisciplinary care, there has been a consistent decline in cancer death rates. Over the past two decades 1,177,300 cancer deaths were averted.2 However, cancer is still the second most common cause of death, exceeded only by heart disease, and accounts for nearly one of every four deaths in the United States.1 The cost of cancer care is rising faster than the other sectors of medicine, having increased from $72 billion in 2004 to $125 billion in 2010; costs are expected to increase further 39% to $173 billion by 2020.3 Even with improving survival rates, there remain gaps in cancer care, with large variations in access, quality, and outcomes. Coupled with the rising costs of health care over the past decades, as well as the discordance between spending and the overall quality of care, cancer care has become increasingly scrutinized in an era of ongoing health care reform.4
Thus, there has become an increasing need for an integrated multidisciplinary field of inquiry that guides practice and policy toward providing high-quality care, focusing on effectiveness, efficiency, and costs. Outcomes research has emerged as a robust field of study, with a focus on improving health by evaluating all aspects of health care delivery.
The field of outcomes research has evolved significantly over the past decade that no single definition fully encompasses its broadening spectrum. In essence, outcomes research is “the study of the end results of health services that takes patients’ experiences, preferences, and values into account and is intended to provide scientific evidence relating to decisions made by all who participate in health care.”5 In surgical oncology these end results include, but are not limited to “the 5 D’s”: Death, Disability, Disease, Discomfort, and Dissatisfaction.
This chapter provides an overview of the history and significance of outcomes research, reviews the key study designs and outcome measures relevant to surgical oncology, and examines the multiple aspects of quality in cancer surgery. The intent is to understand the scope of outcomes research and its implications for the field of surgical oncology.
The earliest reports of “outcomes” can be traced back to the early 1900s. Hospitals were reporting how many patients they treated, but not how many patients benefited from treatment. Ernest Codman, an acknowledged founder of the outcomes movement, was the first American surgeon to follow the progress of patients through their recovery in a systematic manner.6 He kept track of his patients, for at least a year, via “End Result Cards” which contained basic demographic data on every patient, along with the diagnosis, the treatment rendered, and their outcomes. It was his lifelong pursuit to establish an “End Results System” to track the outcomes of patient treatments as an opportunity to identify clinical gaps that could serve as the foundation for improving future care. Codman proposed that institutions should report their outcomes in a uniform and public way that allows comparisons between hospitals. He attempted to institute a “hospital report card” to measure and compare outcomes, and to determine how hospitals and surgeons may improve. Unfortunately, his attempts were met with much resistance.6,7
Half a century later, Avedis Donabedian immigrated to the United States from the Middle East to study public health at Harvard. Donabedian collated the growing literature of outcomes research through the 1960s and presented that in his landmark paper titled “Evaluating the Quality of Medical Care.” Donabedian promoted Codman’s concepts and defined the tripartite interaction between structure, process and outcomes, which is now the general framework of quality assessment.8
However, the biggest influences for the evolution of modern outcomes research were financial and political pressures in the 1960s. After the passage of Medicare and Medicaid legislations in 1966, nearly 85% of Americans were covered by some form of medical insurance and more than two-thirds received coverage through their employers.9 Medical schools increased in number and training became more specialized. At the same time, health care costs exploded as the number of physicians increased, advanced technologies were adopted, more patients were treated, and health insurance plans diversified.
Since then, health care expenditure had risen from 4% to more than 18% of the gross national product (GNP) today.9,10 The rising costs spurred concerns about whether increasing use of technology was justified since it was unclear if medical care was actually getting better. Echoing this, in his book Effectiveness and Efficiency: Random Reflections on the Health Services, Archie Cochrane warned that the pursuit of cure at all cost will inevitably result in bankruptcy and suggested that interventions should be supported by evidence of benefit.11
Along these lines, third-party payers and policymakers started to question the quality of care delivered and the outcomes achieved. These concerns materialized after the landmark works by Wennberg et al, which were the earliest reports of small area variation in health care utilization, examining use of surgical procedures, resources, hospitalization rates, and costs across contiguous counties in Vermont.12 These variations were not explainable by geographical differences in incidence rates or disease severity, and had no resultant effects on outcomes. Variations in utilization indicate that there is considerable uncertainty about the effectiveness of different health services and interventions. Importantly, wide variation in performance suggests substantial room for improvement.
In oncology, the first large-scale effort to study practice variation was the radiation therapy-focused Patterns of Care Study, funded by the National Cancer Institute (NCI). This study was the first systematic effort in the United States to evaluate the patterns of care and patient outcomes of an entire specialty by surveying radiation therapy practices for six commonly radiated malignancies.11 The study reported the national averages for disease-free survival and major complication rates, which served as initial benchmarks for treatment and demonstrated variation in radiation processes.
In response to several other similar reports, patients, providers, payers, and policymakers demanded better assessment, accountability, and objective evidence of value on a larger national level. Through the Health Care Financing Administration and the Agency for Healthcare Research and Quality (AHRQ), the federal government launched multiple programs to gauge the effectiveness of medical interventions and develop guidelines based on the assessment of patient outcomes.
The results of national level efforts have pushed the field of outcomes research into the forefront of clinical research, especially in oncology. By the late 1990s, concerns about the quality of cancer care had been underscored by the National Cancer Policy Board of the Institute of Medicine (IOM), by the President’s Cancer Panel, and by more than a decade of NCI-supported research showing substantial variations in the use of proven interventions.13 NCI established an Outcomes Research Branch, whose mission is to focus on “multi-dimensional measures of patient function, quality of life and health status, preference-based utility measures and measures of economic costs of cancer-specific interventions.” Today, many health care organizations have or are establishing a dedicated outcomes research team or division.
Collectively, outcomes research represents a wide collection of research methodologies, each with distinct designs and scientific foundations compared to clinical research. Traditionally in oncology, the randomized clinical trial (RCT) has been the standard for determining treatment efficacy. Efficacy is defined as the ability of an intervention to work under controlled circumstances. However, results of RCTs are frequently not generalizable as they were performed in idealized settings. Moreover, the development of an RCT is costly and there are situations where an RCT design might not be feasible (e.g., rare conditions) or not practical (e.g., questions of equipoise, perceived difficulty in patient accrual).
Outcomes research can determine effectiveness. Effectiveness refers to how well an intervention works and how appropriately it is used in everyday practice, rather than an idealized RCT setting. The different types of outcomes studies, while largely observational, are heralded for their more pragmatic nature, possibly making research findings more easily generalizable to the entire patient population. Furthermore, quality assessment and economic analyses studies may better assess care delivery.
There are several limitations in outcomes research that must be taken into account. Although large databases and advanced statistical packages are readily available, granular clinical data may be limited and there may be gaps in data. Furthermore, the results may be sometimes harder to study, as with low-frequency procedures (e.g., sarcoma resection) or rare events (e.g., mortality after cholecystectomy). Nevertheless, significant advances in outcomes research have shed light on important health care topics and were instrumental to policy changes.
In the next section we will address some of the major fields of interest and study design concepts that are key to the field of outcomes research.
Area variation is the phenomenon of observing differences in the rates of medical and surgical services across different geographical regions, such as countries, states, provinces, or health service areas. This phenomena prompts concern as it suggests that similar patients are receiving dissimilar care. For example, in their study using the Ontario Cancer Registry, Iscoe et al showed that the rates of breast conserving surgery in women with newly diagnosed breast cancer in the province of Ontario, ranged by county, from 11% to 84%.14 Ultimately, this variation was not found to be related to patient factors, but was more associated with hospital factors and underlying differences in physician opinion.
The results of area variation studies and utilization patterns are meant to spark further research into the causes and consequences of the uncovered variation, in order to develop and implement strategies to minimize them. Studying utilization patterns and area variation does not by itself provide answers to which rate is right for a specific procedure, and some procedures may not have a “right rate” if patient preferences are considered.15 However, it does identify instances most in need for research geared toward reaching physician consensus about surgical indications and effectiveness.
From small area variation research emerged a different study design termed volume-outcome analysis. A growing number of studies in surgical oncology have shown a direct impact of surgical volumes on patient outcomes. As a good example, Birkmeyer et al studied postoperative mortality following pancreaticoduodenectomy from 1992 to 1995, using Medicare data. Patients were divided into even quartiles according to hospital average annual volume of pancreaticoduodenectomies. Remarkably, 53% of patients underwent such surgery at a hospital that performs less than two of this procedure per year. Furthermore, only patients who underwent surgery at a high-volume hospital (≥5/year) had mortality rates of less than 5%, while mortality rates at low-volume centers were three- to fourfold higher. The strong associations between volume and outcome could not be attributed to other case-mix differences or referral bias.
While the volume–mortality relationship holds true across surgical specialties, its strength varies by procedure. In his landmark study, Birkmeyer et al performed a volume-outcomes study using national Medicare data on 14 different high-risk surgical procedures, finding that for procedures such as esophagectomy or pancreatectomy there was more than 10% difference in mortality between high- and low-volume hospitals. In contrast, for other procedures such as coronary artery bypass surgery and carotid endarterectomy, the difference was only about 1%.16
Multiple studies have examined the volume–outcome relationship in surgical oncology.17–22 For example, a large national study using Surveillance, Epidemiology, and End Results (SEER)–Medicare linked data examined the volume–outcome relationship between hospital volume and 5-year survival after surgical resection of six complex cancers. Volume-related differences in 5-year survival were most pronounced after esophageal, gastric, pancreatic, and lung cancer surgery, but less significant for colon and bladder cancer. The study suggested that patients with specific types of cancer can improve their chances of long-term survival if they undergo surgery at a higher volume hospital.22 Volume-outcomes relationships are not limited to the hospital level. Multiple reports show a relationship between surgeon characteristics and patient outcomes. In patients undergoing surgery for lung cancer, multiple studies have shown that surgeon volume and/or specialty impact the adequacy of oncologic staging, short-term outcomes, and late survival, favoring specialty training and higher volume.23–27
The implications of volume-outcome studies are substantial. From a policy and payer perspective, regionalization of services has been considered, moving complex operations to high volume centers with experienced specialty surgeons. Alternatively, major efforts at quality improvement could make results better in all settings.
With the significant advances in multidisciplinary care for cancer, patients and physicians are increasingly being faced with an overwhelming number of decisions. Whether it’s a novel chemotherapeutic agent, radiation therapy protocol, or advanced surgical technique, the cancer patient faces myriad choices for therapy. Moreover, patients in the modern era are savvy consumers with access to a wealth of information. Similarly, physicians are increasingly challenged to guide informed decision making and focus on patient-centered care.
Unfortunately, physicians can fall short of meeting the information needs and expectations of patients, especially that they are oftentimes asked to predict risk and/or benefit. Bidirectionality of information exchange is important and there is growing evidence that patients who are better informed before therapy experience improved psychosocial outcomes.28–30
To this end, multiple tools have evolved under the umbrella of decision sciences, such as prediction models and nomograms. Instead of assigning patients into categories of risk, such as cancer stage, these tools can provide a quantitative estimate of the probability of a specific event for an individual patient, have greater accuracy than reliance on stage or risk groupings, can incorporate novel predictors such as molecular markers, and can be easily presented to any patient in clinic.
Prediction models are constructed with relevant pretreatment and treatment factors, which provide risk estimates based on a patient’s individual set of clinical, demographic, and pathologic variables. For example, researchers from the Mayo Clinic developed prediction models for overall and disease-free survival for patients with colon cancer, to answer the question of who is most likely to benefit from Fluorouracil-based adjuvant therapy.31 Other examples of widely used models include the Gail model for predicting a woman’s risk of invasive breast cancer within 5 years, and the “Adjuvant! Online” model, which estimates the absolute reduction in the risk of breast cancer recurrence with chemotherapy, hormonal therapy, or combination therapy.32
Nomograms are a graphical representation of a mathematical formula, where variables are represented using scales of their respective statistical impact on outcome. For example, researchers at the Memorial Sloan Kettering Cancer Center in New York City developed a series of nomograms to aid physicians in the management of selected cancers.33,34 These nomograms accurately predict the prognosis and can be easily applied to patients in the clinic, showing them firsthand which features have the most impact on their risk on an individualized basis.
Although the field of decision sciences is exponentially growing for oncologic outcomes, very few tools incorporate quality of life. This lack of information may bias patients into foregoing definitive therapy for fear of treatment-related side effects. Thus, there is a need for prediction tools that combine treatment-related morbidity with oncologic outcomes.
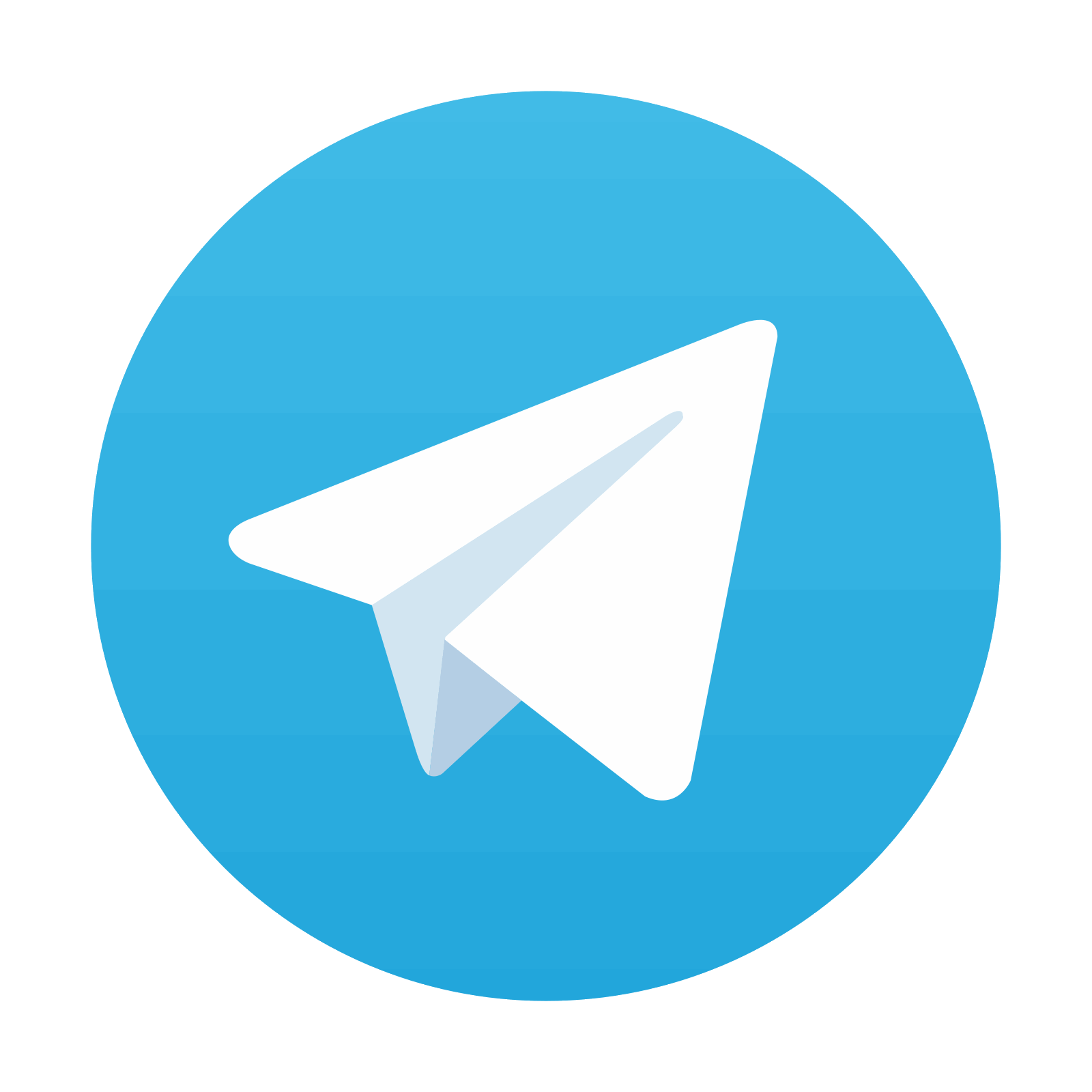
Stay updated, free articles. Join our Telegram channel

Full access? Get Clinical Tree
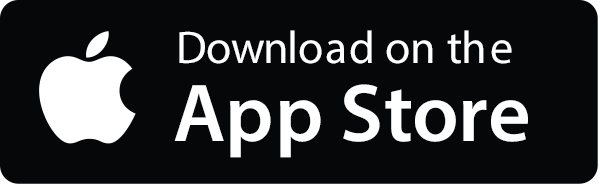
