Methodology/platforms
Measurement
Advantages
Disadvantages
Value in drugdevelopment decisions
Single locus (<100) determination
PCR-based assays on different platforms
PCR products depending on allele by fluorescence, gel electrophoresis, or matrix-assisted laser desorption/ionization- time of flight (MALDI-TOF)
Provides data for single to few targets of interest; relatively inexpensive; customizable
Only applicable for sites of known value, i.e., requires a priori knowledge
Screening for known loci influencing drug efficiency, customizable for pharmacogenomic testing
Genomewide arrays
Microarray platforms from Illumina and Affymetrix
Hybridization and or extension products measured by fluorescence
Simple; relatively fast (high throughput) and inexpensive; applicable for many subjects
Predesigned sets different genome coverage, restricted to common variants; limited addition of customized sites
Hypothesis-free testing/potential of identification of unknown loci/pathways; screening for loci influencing drug efficiency/pharmacogenomic testing; customizable for large scales
Sequencing
Wide range of classical and NGS technologies for both whole genome or locus-specific analysis
Full sequence of bases of small fragments of DNA that are sequentially identified from fluorescence signals emitted as each fragment is resynthesized from a DNA template strand
Determination of DNA sequences without prior knowledge of genome or specific regions
Very cost intensive, high requirements for data handling
Determines full information on genome, epigenome, or transcriptome for decisions on treatment or disease prediction
Proteomics
Method | Measurement | Advantages | Disadvantages | Value in drugdevelopment decisions | |
---|---|---|---|---|---|
2DE and MALDI-MS | Two-dimensional protein separation coupled to peptide mass fingerprinting mass spectrometry | Stained separated protein spots; relative quantification based on differential intensity; after tryptic proteolysis a set of peptide masses indicative for the protein is measured | Protein isoforms and modifications can be detected | Restricted proteome coverage, very low throughput, technically challenging, low reproducibility | Identification of relevant targets |
Shotgun proteomics | High-resolution peptide liquid chromatography mass spectrometry (LC-MSMS) | Peptide masses; relative abundance of peptide based on intensity of extracted ion chromatogram; peptide fragment spectra translating into peptide sequence information | High coverage of proteomes, robust quantification methods available | Complex sample preparation for complete proteome coverage, low to medium throughput | Identification of relevant targets |
Interaction proteomics | Isolation of intact functional protein-protein complexes with specific affinity binders and identification of constituents by LC-MSMS | Peptide masses; relative abundance of peptide based on intensity of extracted ion chromatogram; peptide fragment spectra translating into peptide sequence information | Very sensitive discovery of novel functionalities of relevant proteins | Complex sample preparation, long development times including molecular engineering, low to medium throughput | Discovery of novel functional entities within disease relevant pathways |
Targeted proteomics | Selected reaction monitoring coupled with LC-MSMS | Transitions from peptide mass to peptide fragment mass; area under curve used for relative and absolute quantifications | Selected and specific coverage of proteomes, robust absolute quantification methods available | Long assay development times, high throughput once assay is set | Validation of targets detected in discovery approaches |
Metabolomics
Method | Measurement | Advantages | Disadvantages | Value in drugdevelopment decisions | |
---|---|---|---|---|---|
Nontargeted profiling | Mass spectrometry (MS) based: e.g., Metabolon applies tandem MS combining gas- (GC)and liquid-phase (LC) chromatography | MS based: ion peaks organized by mass, retention time, peak areas | The methods cover a wide nontargeted panel of known and unknown metabolites | Nontargeted methods often only provide semiquantitative traits, such as ion counts per sampling time, which may vary extensively between experiments | Allows the discovery of novel metabolites in disease relevant pathways and in reactions on drug treatment |
A combination of LC-MS and GC-MS assures a maximum coverage of a wide metabolite spectrum | |||||
NMR: Resonance frequency spectra, chemical shift compared to a reference is used to detect metabolites | |||||
NMR has the advantage of leaving the sample intact but requires much larger (10–100×) sample volumes | |||||
Nuclear magnetic resonance (NMR): e.g., Chenomx uses a compound database that matches NMR acquisition capabilities with support for field strengths of 400, 500, 600, 700, and 800 MHz | |||||
Targeted profiling | Mass spectrometry based (MS): e.g., Biocrates AbsoluteIDQ Kits are based on tandem MS and apply targeted metabolomics | Ion peaks organized by mass; detection is based on isotope-labeled internal standards | The metabolites are in advance known and thereby provide more precise measurements and are easy to replicate | The methods are limited to analyzing only a subset of preselected compounds | Validation of targets detected in discovery |
The targeted panel comes at the cost of missing potentially interesting metabolites | |||||
The methods provide absolute quantification by comparison to isotope-labeled external standards |
Conclusions
Technologies continue to propel the omics fields forward. However, translating research discovery into routine clinical applications use is a complex process not only from scientific prospective but also from ethical, political, and logistic points of view. Particularly the implementation of omics based tests requires changes in fundamental processes of regulation, reimbursement, and clinical practice. Altogether, developments in the field of omics technologies hold great promise to optimize patient care and improve outcomes and eventually lead to new tests and treatments that are well integrated in routine medical care.
Introduction
During the past few decades, there have been dramatic increases in type 2 diabetes and obesity worldwide with prevalence estimates expected to rise even further in the future [1]. Classic epidemiological studies have already successfully identified multiple key risk factors for many metabolic diseases including both, obesity and type 2 diabetes, by typically relating lifestyle and environmental exposures to disease end points. However, often molecular mechanisms that underlie the observed associations remain unclear.
Recent developments in the field of high-throughput omics technologies have nourished the hope of incorporating novel biomarkers at multiple levels ranging from genetic predisposition (genome) and epigenetic changes (epigenome) to the expression of genes (transcriptome), proteins (proteome), and metabolites (metabolome) into epidemiological studies with the potential of obtaining a more holistic picture of disease pathophysiology [2, 3]. Thus, being at the intersection of classical epidemiology and systems biology, this “systems epidemiology” approach combines traditional research with modern high-throughput technologies to understand complex phenotypes [3]. The major advantage of systems epidemiology is its hypothesis-free approach which does not require a priori knowledge about possible mechanistic pathways or associations. By potentially improving our understanding of biological mechanisms that underlie disease pathophysiology in humans, this approach might also spur translational innovation and provide opportunities for personalized medicine through stratification according to an individual person’s risk and more precise classification of disease subtypes [4]. Examples for successful integration of omics data into epidemiology is provided by two recent studies in the field of metabolomics. In these studies several metabolites were identified to play a role in the pathogenesis of type 2 diabetes. The identified set of metabolite biomarkers may successfully be used to help to predict the future risk for type 2 diabetes long before any clinical manifestations [5, 6]. Another example derives from the field of genomics where a number of type 2 diabetes susceptibility loci such as adipokines, TCF7L2, IRS1, NOS1AP, and SLC30A8 have been shown to be associated with a distinct response to pharmacological and/or lifestyle intervention [7]. However, in general the results of most omics studies to date have shown that although an improved understanding of some pathogenic mechanisms is already emerging, the majority of identified omics-based biomarkers and signatures typically cannot be translated into clinical practice in a fast and straightforward process [3, 4]. To take genomics as an example, the recent development of high-throughput technology has led to large genome-wide association studies (GWAS) in which to date about 12,000 genetic susceptibility loci for common diseases, and in the case of type 2 diabetes, more than 70 genetic susceptibility loci have been identified [4, 8, 9]. Yet, these loci typically display rather modest effect sizes as exemplified by TCF7L2. Genetic variants of this gene display the strongest replicated effect in European populations on type 2 diabetes so far but confer odds ratios (OR) of only 1.3–1.6 [10]. Likewise, in spite of these large numbers of identified genetic susceptibility loci, the proportion of explained genetic heritability is still only marginal for the quantitative obesity traits body mass index (BMI) (2.7 % estimated [11]) and waist-to-hip ratio (WHR) (1.4 % estimated [12]) as well as for type 2 diabetes for which a proportion of only up to 10 % explained heritability is assumed [10]. Furthermore, the identified susceptibility loci so far do not add to the clinical prediction of type 2 diabetes beyond that of traditional risk factors, such as obesity, physical inactivity, family history of diabetes, and certain clinical parameters. These examples show that in spite of some early success, most of the omics fields are still in the discovery phase. This has been attributed to the time-consuming, expensive, and uncertain development process from disease biomarker discovery to clinical test, the underdeveloped and inconsistent standards to assess biomarker validity, the heterogeneity of patients with a given diagnosis, and the lack of appropriate study designs and analytical methods for these analyses [13]. Some critics have already questioned the excitement afforded omics-based discoveries, suggesting that advancements will have primarily modest effects in patient care [14]. It is generally agreed that methods used in systems epidemiology provide potential tools for future clinical application using omics-based tests. However, the discoveries of omics analyses as yet are insufficient to support clinical decisions. Altogether, it can be assumed that further developments in omics research together with the integration of omics data into risk interaction with relevant environmental exposures and lifestyle factors might yield the potential of a holistic understanding of molecular pathways underlying epidemiologic observations. Yet, the extent to which systems epidemiology can be translated into clinical practice will only become evident in a few years’ time.
Background
Several omics technologies can be used to identify genetic susceptibility loci or biomarkers which are associated with a certain disease of interest and could potentially be used to better understand the disease mechanisms or to develop an omics-based test for clinical application. In this chapter we aim to give an overview of the most important omics research fields along with their potential clinical value to date.
Genomics
The genome is the complete sequence of DNA in a cell or organism. This genetic material may be found in the cell nucleus or in other organelles, such as mitochondria. A major advantage of genomic research is that except for mutations and chromosomal rearrangements, the genome of an organism remains essentially constant over time and is consistent in distinct cell types and tissues. Thus, the genome can easily be assessed in appropriate blood samples at any time. With the introduction of the first sequencing technologies allowing the readout of short deoxyribonucleic acid (DNA) fragments, the year 1977 heralded a new era of genomics research [15, 16]. However, due to the enormous costs of sequencing, researchers focused on candidate approaches to assess genetic variation, involving a number of polymerase chain reaction (PCR)-based methods to determine few single nucleotide polymorphisms (SNPs). In 2005, the first successful GWAS identified a major susceptibility gene for a complex trait, the factor H gene as a genetic cause of age-related macular degeneration [17]. Since then, rapid technological development has been an enabling force, providing transformative tools for genomic research including GWAS and next-generation sequencing (NGS) to assess complete genetic variation of the genome or specific genetic regions [18]. GWAS are performed using arrays of thousands of oligonucleotide probes that hybridize to specific DNA sequences in which SNPs are known to occur. Despite of its restriction to common variants, incomplete genome coverage, and inherent challenge of discerning the actual causal genetic variant, GWAS have been a transformative technology, representing a major advance over the candidate gene approach previously used for decades. By assessing variation across the entire human genome in an unbiased hypothesis-free fashion, this approach offers an unprecedented opportunity to uncover new genetic susceptibility loci for certain diseases with a potential clinical utility [18]. Apart from SNPs, genomic analyses can detect insertions, deletions, and copy number variations, referring to loss or amplification of the expected two copies of each gene. Personal genome sequencing is a more recent and powerful technology, which allows for direct and complete sequencing of genomes. Initially not affordable for large-scale studies, the costs of sequencing a human genome has now dropped to about $1,000 US to date; these costs continue to decline. Current sequencing machines can read about 250 billion bases in a week compared to only about 5 million in 2000 [19]. This massively parallel sequencing applied in NGS technology now allows for direct measurement of all variation in a genome [20], which is estimated to be about three million variants by the data from the 1,000 Genomes Project [21]. Effects of genetic variants in the ~1 % coding regions are more or less predictable. However, the biological implications of variants that occur in the vast remaining noncoding regions of the genome remain largely unknown. This challenge is addressed by the National Human Genome Research Institute initiated project ENCODE, the Encyclopedia of DNA Elements, which aims to functionally annotate noncoding regions of the genome (7). Waiting for those results, researchers have focused on exome sequencing, examining rare and potentially deleterious coding region variants. Today, GWAS and NGS are integral tools in basic genomic research but are increasingly being explored for clinical applications. Because of its high complexity, the elucidation of the genetic basis of common diseases has been challenging. However, research in this area has already broadened our understanding of underlying disease mechanisms and has revealed new therapeutic approaches through repurposing of existing drugs for treating diseases they were not originally intended to treat [22–24].
Epigenomics
Epigenetic regulation of gene expression is mediated through diverse mechanisms such as several kinds of reversible chemical modifications of the DNA or histones that bind DNA as well as nonhistone proteins and noncoding ribonucleic acid (RNA) forming the structural matrix of the chromosomes [25]. Epigenomic modifications can occur in a tissue-specific manner, in response to environmental factors or in the development of disease states, and can persist across generations. The epigenome can vary substantially over time and across different cell types within the same organism. Since epigenetic mechanisms may function as an interface between genome and environment, epigenetic deregulation is likely to be involved in the etiology of human diseases associated with environmental exposures [26, 27]. As of today, biochemically epigenetic changes that are measured at high throughput belong to two categories: methylation of DNA cytosine residues (at CpG sites) and multiple kinds of modifications of specific histone proteins in the chromosomes. Human studies to date concentrate particularly on DNA methylation which is why this chapter focuses mainly on methods for DNA methylation profiling. Analogous to early genomics studies, DNA methylation studies were initially confined to a candidate gene approach to identify alterations occurring in disease states. New powerful technologies, such as comprehensive DNA methylation microarrays [28, 29] and genome-wide bisulphite sequencing [30] reinforce the notion of epigenetic disruption at least as a signature of human diseases [31]. As DNA methylation patterns are affected by genetic variation and due to their variability over time, it is one of the major challenges today to distinguish whether the methylation profile is a result of the disease or whether it is a contributing cause of it. First results from candidate regions as well as epigenome-wide association studies (EWASs) indicate that epigenetic profiles will be useful in practical clinical situations, providing a promising tool for the diagnosis and prognosis of disease and for the prediction of drug response. The pioneering efforts at generating a whole-genome single-base-pair resolution methylome map of eukaryotic organisms were made by two independent groups in 2008. Using two different shotgun bisulphite high-throughput sequencing protocols named BS-seq [32] and MethylC-seq [33], both groups generated comprehensive cytosine methylation maps of the Arabidopsis thaliana genome. MethylC-seq was also later applied to generate the first ever single-base resolution map of human methylome in embryonic stem cells and fetal fibroblasts [34]. Remarkably, this study identified that nearly one quarter of cytosine methylation in embryonic stem cells is in non-CG context, which emphasizes the need of an unbiased, genome-wide approach.
Transcriptomics
The transcriptome is the complete set of transcripts in a cell, and their quantity, at a specific developmental stage or physiological condition. Understanding the transcriptome is essential not only for the interpretation of functional elements of the genome but also for understanding of the pathogenic mechanisms and disease manifestation. The key aims of transcriptomics comprise indexing of all species of transcript, including mRNAs, noncoding RNAs, and small RNAs; determination of transcriptional structure of genes, in terms of their start sites, 5′ and 3′ ends, splicing patterns, and other posttranscriptional modifications; as well as quantification of changing expression levels of each transcript during development and under different conditions. Various technologies such as hybridization or sequence-based approaches have been developed to deduce and quantify the transcriptome. Hybridization-based approaches typically involve incubating fluorescently labeled cDNA with custom-made microarrays or commercial high-density oligo microarrays. Furthermore, specialized microarrays have been designed, for example, with probes spanning exon junctions that can be used to detect and quantify distinct spliced isoforms [35]. Genomic tiling microarrays that represent the genome at high density have been constructed and allow the mapping of transcribed regions to a very high resolution, from several base pairs to ~100 bp [36–39]. Hybridization-based approaches are high throughput and relatively inexpensive, except for high-resolution tiling arrays that interrogate large genomes. However, these methods have several limitations, which include: reliance upon existing knowledge about genome sequence; high background levels owing to cross-hybridization [40, 41]; and a limited dynamic range of detection owing to both background and saturation of signals. Moreover, comparing expression levels across different experiments is often difficult and can require complicated normalization methods.
Proteomics
The proteome is the complete set of proteins expressed by a cell, tissue, or organism. The proteome is inherently quite complex because proteins can undergo posttranslational modifications (glycosylation, phosphorylation, acetylation, ubiquitylation, and many other modifications to the amino acids comprising proteins), have different spatial configurations and intracellular localizations, and interact with other proteins as well as other molecules. This complexity may be substantially challenging for the development of a proteomics-based test that might be used in clinical practice. The proteome can be assayed using mass spectrometry and protein microarrays [42, 43]. Unlike RNA transcripts, proteins do not have obvious complementary binding partners, so the identification and characterization of capture agents is critical to the success of protein arrays. The field of proteomics has benefited from a number of recent advances. One example is the development of selected reaction monitoring (SRM) proteomics based on automated techniques [44]. During the past 2 years, multiple peptides which are specific for distinct proteins derived from each of the 20,300 human protein-coding genes known today have been synthesized and their mass spectra determined. The resulting SRMAtlas is publicly available for the entire scientific community to use in choosing targets and purchasing peptides for quantitative analyses [45]. In addition, data from untargeted “shotgun” mass spectrometry-based proteomics have been collected and uniformly analyzed to generate peptide atlases which are specific for plasma, liver, and other organs and biofluids [46]. Furthermore, antibody-based protein identification and tissue expression studies have progressed considerably [47, 48]. The Human Protein Atlas has antibody findings for more than 12,000 of the 20,300 gene-encoded proteins. Already now the Protein Atlas is a useful resource for planning experiments and will be further enhanced by linkage with mass spectrometry findings through the emerging Human Proteome Project [49]. In addition, recently developed protein capture-agent aptamer chips also can be used to make quantitative measurements of approximately 1,000 proteins from the blood or other sources [50]. A major bottleneck in the successful deployment of large-scale proteomic approaches is the lack of high-affinity capture agents with high sensitivity and specificity for particular proteins. This challenge is exacerbated in highly complex mixtures such as whole blood, where concentrations of different proteins vary by more than ten orders of magnitude. One technology that holds great promise in this regard is “click chemistry” [51], which uses a highly specific chemical linkage to “click” together low-affinity capture agents to create a single capture agent with much higher affinity.
Metabolomics
The metabolome is the complete set of small molecule metabolites found within a biological sample (including metabolic intermediates in carbohydrate, lipid, amino acid, nucleic acid, and other biochemical pathways, along with hormones and other signaling molecules, as well as exogenous substances such as drugs and their metabolites). The metabolome is dynamic and can vary within a single organism and among organisms of the same species because of many factors such as changes in diet, stress, physical activity, pharmacological effects, and disease. The components of the metabolome can be measured with mass spectrometry [52] as well as by nuclear magnetic resonance spectroscopy [53]. This method also can be used to study the lipidome [54], which is the complete set of lipids in a biological sample and a rich source of additional potential biomarkers [55]. Improved technologies for measurements of small molecules [56] also enable the use of metabolomics for the development of candidate omics-based tests with potential clinical utility [57]. Promising early examples include a metabolomic and proteomic approach to diagnosis, prediction, and therapy selection for heart failure [58].
Key Methods
Various emerging omics technologies are likely to influence the development of omics-based tests in the future, as both the types and numbers of molecular measurements continue to increase. Furthermore, advancing bioinformatics and computational approaches and a larger integration of different data types steadily improve evaluation and assessment of omics data. Given the rapid pace of development in these fields, it is not possible to give detailed description of all current and emerging technologies or data analytic techniques. Instead the following section focuses on key methods and techniques which are most relevant in today’s practical research.
Genomics
When designing a study to assess genetic variation, the selection of an appropriate genotyping or sequencing platform for particular types of experiments is an important consideration. The decision depends on various factors, including speed, cost, error rate, and coverage of different genotyping arrays.
In spite of immense technological advance since the introduction of the first sequencing technologies, a number of early genotyping technologies which are capable of genotyping small numbers of previously selected SNPs and which were thus originally used in candidate gene studies are still in use to replicate susceptibility loci identified in discovery GWAS. For example, the iPLEX Assay developed by Sequenom provides the possibility to custom design a set of assays for up to 36 SNPs using single nucleotide extensions with mass modified nucleotides that are distinguished in MALDI-TOF MS [59]. Also the TaqMan System allows a high flexibility through customizability. This technology uses hybridization of allele-specific oligonucleotides with discriminative fluorescent labeling and a quencher suppressing fluorescence while the oligonucleotide is intact. Applying PCR the hybridized oligonucleotides are destroyed and the label is released to distinguish alleles by different fluorescence. These technologies have the potential to design specific low-scale arrays for clinical use. One example for a small custom array used successfully in pharmacogenomics is a genotyping array that was designed based on TaqMan chemistry providing a broad panel of SNPs chosen from PharmGKB to cover 120 genes of pharmacogenetic relevance including 25 drug metabolism genes and 12 drug transporter genes [60]. Together with the sequencing methods mentioned below, up to now, customized SNP chips are the most cost-efficient methods available to assess genetic variation in specific regions with a moderate number of variants.
Current genotyping arrays used in GWAS can detect up to five Mio SNPs with different designs in terms of genome coverage. These genotyping chips are widely used for the discovery of new loci associated with a certain disease as they are relatively cheap for the amount of information generated. Based on information from the 1,000 genomes project [21], DNA array data can be enhanced by estimating SNPs that are not covered by the chip. This so-called imputing of SNPs gives reasonably good information on the genotypes for all known common SNPs throughout the whole genome with some limitations regarding some problems to estimate rarer variants. The two most prominent genotyping platforms are provided by the companies Illumina and Affymetrix. While the genome-wide bead arrays (Illumina) were mainly designed to cover coding regions, chip arrays (Affymetrix) focused on the more or less evenly coverage of the whole genome. Both technologies are based on hybridization, but while chip arrays bind labeled fragments of the targets genomic DNA to multiple probes for the specific alleles, bead arrays bind genomic DNA using single-base elongation with labeled nucleotide to detect the specific alleles. Furthermore, the different length of probes between chip arrays (25 nucleotides) and bead arrays (50 nucleotides) influences selectivity [61]. The latest generation of SNP chips provides the possibility to include custom SNPs, mutations, and CNVs to increase flexibility. One example for a complete custom genome-wide bead array is the Illumina Cardio-Metabochip, which was designed by a number of GWAS consortia (viz., DIAGRAM, MAGIC, CARDIoGRAM, GIANT, ICBP GWAS + QT-IGC, Global Lipid Consortium) investigating different but related disease and quantitative phenotypes. All participating consortia selected SNPs of interest either to replicate previous findings or for finemapping of previously identified candidate loci. Because costs for the Illumina Cardio-Metabochip were below those of the genotyping chips used in discovery, this customized chip could be applied for a much larger number of cohorts. The approach turned out to lead to the successful identification of new loci for all traits and clinical endpoints being in the focus of the participating consortia.
Not quite a quarter of a century after the implementation of classical DNA sequencing technologies, the advance of sequencing technologies named “next-generation sequencing” (NGS) succeeded in decoding of (almost) the whole human genome (2.7 billion bp). The advance was to some extent an extension of readout lengths but was mainly based on a massive parallelization of the sequencing process. In the currently used advanced Sanger capillary sequencing systems, for example, millions of copies of the sequence are determined in parallel using an enzymatic primer extension and a mixture of deoxynucleotides (the “natural” building blocks of DNA) and base-specific labeled dideoxynucleotides which cause a nonreversible termination of the extension reaction and thereby DNA products of different lengths and molecular weight. Eventually, this can be used to sort the distinct DNA products and readout sequences with a high validity (error rates between 10−4 and 10−5) [62, 63]. After the introduction of genotyping array platforms, GWA studies conducted in large samples sizes boosted the knowledge of the influence of common genetic variants on type 2 diabetes as well as on measures of adiposity. Meanwhile, sequencing technologies were mainly used for finemapping or replication of previously identified susceptibility regions. Because of its high validity, besides the MALDITOF-Sequenom technology, particularly Sanger sequencing has been as yet the most frequently used technology for a valid sequencing of a limited number of previously identified InDels or SNPs and the finemapping of low-complex regions of interest in small sample sizes [63]. The lately developed NGS technologies all allowed an even higher parallelization and throughput of data mainly by usage of beads or well plates and the development of new methodologies like bridge amplification. Some of these technologies are like the Sanger sequencing principally based on an allele-specific primer elongation of DNA polymerases such as the 454/Roche pyrosequencing in which the enzymatic extension of the DNA strand together with a sulphurylase and luciferase is translated into a light reaction, the Illumina GA sequencing which uses reversible terminator chemistry with blocked and labeled nucleotides [64] and the allele-specific primer extension Sequenom iPLEX techonolgy while others incorporate distinct methodologies such as Applied Biosystem’s SOLiD which uses oligonucleotide ligation or HeliScope which sequences single molecules using asynchronous virtual terminator chemistry [63].
As a cost-saving and efficient alternative to whole genome sequencing, innovative study designs refine to whole exome sequencing. Exomes are the part of the genome which is eventually translated into a protein and may therefore have direct clinical importance. For patients with extreme phenotypes, this approach has already yielded some successes, as was shown exemplarily by the identification of DCTN4 in the case of clinical sequelae in cystic fibrosis patients infected with Pseudomonas aeruginosa [65]. In the case of type 2 diabetes and obesity, large-scale associations by huge consortia are currently under way. However, the success of whole-exome sequencing is not quite guaranteed as GWA studies have revealed that a lot of genetic susceptibility loci are not located within an exome or can even not be clearly assigned to a protein coding region in the genome. Traditional Sanger sequencing delivers highly valid DNA sequences but has a slow throughput and is rather cost intensive compared to next-generation methods. Recent advances in sequence-based technology permit massive parallel sequencing. Real-time sequencing has replaced natural nucleotides or reversible terminators by detection of continuously added fluorescence-labeled nucleotides to the growing DNA strand thereby enhancing the speed and output length of nucleotides (18). Established sequencing libraries and post-sequencing bioinformatics algorithms have further facilitated the generation, reconstruction, and analysis of sequence reads, while the optimization of the sequencing accuracy together with redundant sequencing has constantly reduced sequencing errors. Bioinformatics tools are continuously being refined to store and process the massive amount of sequence data. In spite of all these advances, there are several challenges which remain with next-generation sequencing. Firstly, platforms differ by template preparation, sequencing chemistry, imaging, read length, and quantity per run. And although quality measures are provided by the respective manufacturers, a uniform quality assessment protocol has not been implemented so far. Secondly, statistical analyses need to account for a possible type I error (i.e., false-positive findings) in the resulting huge data sets and thus need to develop methods to dissect phenotypically relevant variants from commonly shared alleles. Finally, the identification of disease-specific genetic variants from bystanders remains a challenge. Despite these limitations, next-generation sequencing has already been applied, for example, to follow up GWAS loci for CVD phenotypes, to identify rare forms of CVD traits by exome sequencing, and to identify structural variation in the genome [66]. Altogether, next-generation sequencing has already shown great success and the methods for sequencing have evolved to the point that sequencing of an entire genome has become considerably less expensive and straightforward.
Epigenomics
A wide range of methods and approaches for the identification, quantification, and mapping of DNA methylation within the genome are available [67]. While the earliest approaches were nonspecific and were at best useful for the quantification of total methylated cytosines in the chunk of DNA, there has been a considerable progress and development over the past decades. Methods for DNA methylation analysis differ in their coverage and sensitivity, and the method of choice depends on the intended application and desired level of information. Potential results include global methyl cytosine content, degree of methylation at specific loci, or genome-wide methylation maps.
Numerous methods have been reported for locus-specific analysis. While the earlier ones relied exclusively on restriction enzymes (RE), application of bisulphite conversion has revolutionized the field. Methylation at one or more CpG sites within a particular locus can be determined either qualitatively (presence or absence) or quantitatively. DNA samples are usually derived from a heterogeneous population of cells, in which individual cells may vary vastly in their DNA methylation patterns. Hence, most of the methods aimed at quantitative measurement of DNA methylation determine the average methylation level across many DNA molecules.
Methylation-specific PCR (MSP) is one of the most widely used methods in DNA methylation studies. Using primers that can discriminate between methylated (M primer pair) or unmethylated (U primer pair) target region after bisulphite treatment, DNA is amplified using PCR. One primer in both M and U primer pairs necessarily contains a CpG site near its 3′ end. This CpG site is the one under investigation, and both M and U primers contain the same site. A forward primer in M pair having the C nucleotide in its sequence for the CpG position under investigation will fail to amplify the region if that particular cytosine is unmethylated (hence, converted to uracil during bisulphite reaction) and vice versa. Success or failure in amplification can qualitatively determine the methylation status of the target site [68]. Although rapid and easy to use, MSP suffers from various disadvantages such as reliance on gel electrophoresis and the fact that only a very few CpG sites can be analyzed using a given primer pair. Using TaqMan technology in a method, named as MethyLight, bisulphite-converted DNA is amplified and detected by methylation-state-specific primers and TaqMan probes in a real-time PCR [69]. As the two strands of DNA no longer remain complementary after bisulphite conversion, primers and probes are targeted for either of the resulting strands. Initial template quantity can be measured by traditional real-time PCR calculations. Incorporation of various quality controls for bisulphite conversion and recovery of DNA after bisulphite treatment have improved the quantitative reliability of this method. MethyLight has many advantages over MSP and other locus-specific DNA methylation analysis methods. It avoids gel electrophoresis, restriction enzyme digestion, radiolabeled dNTPs, and hybridization probes, yet there are a few shortcomings above all PCR bias a phenomenon owing to the investigation of cell mixtures. Caused by a potentially distinct cytosine content of heterogeneous cell populations and a resulting distinct amplification with varying efficiencies, PCR bias can potentially affect the accurate quantitative estimation of DNA methylation [70].
In addition, pyrosequencing, a sequencing by synthesis approach, has been widely used for locus-specific DNA methylation analyses. Pyrosequencing offers a highly reliable, quantitative, and high-throughput method for analysis of DNA methylation at multiple CpG sites with built-in internal control for completeness of bisulphite treatment. As bisulphite treatment converts unmethylated cytosines into uracils (which will be converted to thymine upon subsequent PCR amplification) leaving methylated ones unchanged, the methylation difference between cytosines is converted into a C/T genetic polymorphism [71]. Although bisulphite pyrosequencing is one of the most widely used methods for quantitative determination of methylation, it is limited by a few drawbacks. Thermal instability of enzymes used in pyrosequencing reactions, particularly luciferase, requires the reaction to be carried out at 28 °C. Therefore, optimal amplicon size to be subsequently used for pyrosequencing reaction is around 300 bp or less to avoid secondary structures [72]. As bisulphite conversion results in low-complexity DNA molecules (A, T, and G nucleotides, except very few methylated cytosines), designing optimal primer sets for every region of interest is a difficult task [73].
In yet another high-throughput quantitative approach, Sequenom’s EpiTYPER assay, bisulphite-treated DNA is first amplified with specific primers. The reverse primer is tagged at the 5′ end with a T7 promoter sequence to facilitate in vitro transcription by phage RNA polymerase in the next step. Endonuclease RNase A, which cuts after every C and U in an RNA molecule, is used to generate short fragments. However, for only C-specific or U-specific cleavage, two separate in vitro transcription reactions are run. In a U-specific cleavage reaction, dCTP is used instead of CTP. This blocks cleavage after C and RNaseA only cut after U. Similarly, a separate C-specific cleavage reaction is set up. That way, a complex mixture of short oligonucleotides of varying lengths is generated. Methylation-dependent C/T polymorphism in bisulphite-converted DNA is reflected as G/A in transcribed RNA molecules and results in a 16-Da mass difference for each CpGsite in cleavage products, which is then analyzed by MALDI-TOF spectrometry [74].
The introduction of microarray technologies opened unprecedented horizons in methylome research. In contrast to methods discussed above, thousands of regions of interest can be analyzed simultaneously. Various platforms differing in their resolution and targeted regions have been used to study the DNA methylome. Ranging from CGI or promoter region-specific platforms to oligonucleotide-tiling arrays virtually covering the whole genome with high resolution, various arrays have been custom designed or are commercially available [67]. As only a very small portion of the methylome can be studied using restriction enzyme-based approaches, affinity enrichment of DNA has emerged as an effective alternative. As a general approach, DNA is first sheared randomly, and a portion of DNA is set aside to be used later as reference. The resulting enriched fraction and input control are differentially labeled and hybridized to a custom-made or commercially available array platform [75, 76]. Bisulphite treatment of DNA results in reduced sequence complexity and increased redundancy, thus reducing hybridization specificity [77]. Therefore, coupling bisulphite treatment with array hybridization has not been much successful. However, the Illumina GoldenGate BeadArray and the Infinium platform are well suited for this purpose and have been widely used.
On the Illumina GoldenGate platform, two pairs of oligos, allele-specific oligo (ASO) and locus-specific oligo (LSO), each ASO-LSO pair specific for methylated or unmethylated target CpG, are used. Each ASO has two parts: a sequence complementary to target and a priming site (P1 or P2). LSO consists of three parts: a stretch of sequence complementary to target, a priming site (P3), and an address sequence which identifies its genomic location and is complementary to capture probes on the BeadArray. The hybridization of pooled oligos to bisulphite converted fragmented immobilized DNA results in specific hybridization. ASO is then extended and ligated to corresponding LSO. During the following PCR amplification, methylated and unmethylated fractions are differentially labeled with fluorescent dyes using differentially labeled P1 and P2 primers. The hybridization to the bead array results in annealing of address sequence in LSO with a unique capture probe on the array. Resulting fluorescence intensities can be compared to quantitatively measure the methylation status at a particular locus [78]. The Infinium platform which is a modification of the genotyping array is based on a similar principle but uses slightly distinct design. On an Illumina methylation assay chip, two types of beads are present, one for methylated (M) and one for unmethylated (U) template. Each bead is covered with hundreds of thousands of copies of a specific 50-mer oligonucleotide. Bisulphite-converted DNA is subjected to whole-genome amplification, fragmented, and hybridized to the chip. Only in case the specific bases match, this is followed by a single-base extension using hapten-labeled ddNTPs. Complex immunohistochemical assays differentiate the two bead types and produce type-specific signals, of which relative intensities are used for quantitative estimation of methylation status of the corresponding CpG site [79]. While the GoldenGate assay can be used to assess up to 1,536 CpG sites, which are completely customizable [78], the actual version of the Infinium platform, the HumanMethylation450 BeadChip, covers >485,000 CpG sites per sample including more than 99 % of RefSeq genes with multiple CpG sites per gene spread across the promoter, 5′ untranslated region (UTR), first exon, gene body, and 3′ UTR. In addition to 96 % CGIs, the Infinium chip also includes various CpG sites corresponding to CGI shores and flanking regions [29].
Whole-genome bisulphite sequencing (WGBS) allows for an unbiased assessment of the profile of complete DNA methylomes. Using bisulphite-treated DNA converting unmethylated cytosines into thymidines [80], next-generation sequencing (NGS) technology is used to obtain a complete overview of CpG methylation level at base-pair resolution. Massively parallel revolution in sequencing has shifted the paradigm of genome-wide DNA methylation analysis. A whole methylome map of a particular cell type can now be generated in a matter of 3–5 days [81]. As for genomics compared to array hybridization, sequencing-based analysis provides more detailed information with less DNA input. Restriction enzyme treatment and affinity enrichment methods have been adapted to downstream massive sequencing, with additional advantage of reduction in target DNA complexity and amount of sequencing. Improving the original HpaII tiny-fragment enrichment by ligation-mediated PCR (HELP) assay by using two sets of adaptors to amplify <200-bp fragments during the LM-PCR step and coupling HELP output with NGS, Oda et al. analyzed 98.5 % of CGIs in the human genome. In this study, methylated spots were identified by their absence in the HpaII-cut fraction’s sequence reads using MspI digestion as control [82]. Using a similar approach named Methyl-seq [83] revealed important differences in methylation patterns between hESCs, their in vitro differentiated derivatives, and human tissues. Another widely used method, so-called reduced representation bisulphite sequencing (RRBS), couples restriction enzyme representation followed by bisulphite sequencing on a massively parallel platform [84]. MspI digestion before bisulphite conversion allows reducing redundancy by selecting a CpG-rich genomic subset [84]. The affinity-enrichment-based methods MIRA-seq [85], MeDIP-seq [86], MethylCap-seq [87], and MBD-isolated genome sequencing [88], which have been adapted to downstream analysis by massive parallel sequencing, follow more or less similar protocols. However, enrichment methods target different compartments of the genome. For example, while MeDIP captures methylated regions with low CpG density, MBD favors high-CpG-density regions.
Raw sequencing reads from each type of high-throughput platform need to undergo dedicated and complex bioinformatics analysis pipelines, which differ from each other according to the platform used and the particular type of experiment and protocol. As a general approach, enzyme- and affinity-based sequencing methods determine the relative abundance of different genomic regions in enriched fraction by counting the number of reads that uniquely map to the reference genome as compared to input control. On the other hand, bisulphite sequencing extracts information directly from the sequence [77]. All sequencing approaches discussed above have their own advantages and shortcomings. Shotgun bisulphite sequencing, though still a gold standard because of its genome coverage, is cost and effort intensive which makes this approach unfeasible for studies involving large numbers of samples. Sequence selection strategies though useful are invariably prone to particular biases. While RE-based strategies are limited by the number and distribution of enzyme recognition sites, affinity enrichment methods cannot yield information on individual CpG dinucleotides [77]. Therefore, while the whole-genome approach is useful to generate reference methylome maps, sequence selection strategies can yield useful information about most relevant regions. Relative merits and demerits of each method have been excellently reviewed elsewhere [77].
DNA methylation is a dynamic modification that is put in place and removed by a range of enzymes that may be targeted for disease treatment [89]. Given their dynamic nature, epigenetic disease biomarker genes have to be determined by considering interindividual and intraindividual variations. In this respect, the definition of reference DNA methylation data sets will be of a high value, facilitating biomarker selection by initially defining sites that show consistent levels of DNA methylation in healthy individuals. The comprehensive study of profiles in different healthy individuals and across different types of tissue allows a reasonable estimate of the variance of specific CpG sites or regions such as promoters. Reference data sets are now being created in consortia such as Blueprint, the International Human Epigenome Consortium (IHEC), and Roadmap using high-resolution technologies [90, 91]. Focusing on healthy tissue types, these joint efforts aim to release reference data sets of integrated epigenomic profiles of stem cells, as well as developmental and somatic tissue types allowing for free access by the research community. As a paradigm, the estimation of genetic variance in the human population and the identification of SNPs improved mutational analyses by excluding false-positive hits for disease-linked mutations before screening. Concordantly, filtering out loci that are unstable in DNA methylation between individuals excludes unsuitable CpG sites before selection of appropriate biomarker candidates. Systematic screening of reference data sets will allow us to identify and to exclude variable CpG sites and regions, highly facilitating future biomarker selections.
Transcriptomics
Like the field of genomics and epigenomics, also the field of transcriptomics profits from the rapid development in microarray as well as sequencing technologies. Various microarray systems have been developed. While some platforms of high quality and low cost are commercially available, others are produced primarily in research laboratories. Microarrays used for transcriptomics differ according to type of solid support used, the surface modifications containing various substrates, the type of DNA fragments used on the array, as well as whether the transcripts are synthesized in situ or presynthesized and spotted onto the array, and how DNA fragments are placed on the array [92]. Widely used probes on array platforms are complementary DNA (cDNA) or oligonucleotides. Ideal probes should be sequence validated, unique, and representative of a significant portion of the genome, and they should have minimal cross-hybridization to related sequences. Probes for cDNA arrays are composed of cDNAs from cDNA libraries or clone collections that are “spotted” onto glass slides or nylon membranes at precise locations. Spotted arrays composed of a collection of cDNAs allow for a larger choice of sequences incorporated in the array and may thus enhance the discovery of genes in case unselected clones from cDNA libraries are used [93]. Oligonucleotide arrays consist of probes composed of short nucleotides (15–25 nt) or long oligonucleotides (50–120 nt) that are directly synthesized onto glass slides or silicon wafers, using either photolithography or ink-jet technology. The usage of longer oligonucleotides (50–100 mers) may increase the specificity of hybridization and increase sensitivity of detection [94]. Arrays fabricated by direct synthesis offer the advantage of using reproducible, high-density probe arrays containing more than 300,000 individual elements, with probes specifically designed to contain the most unique part of a transcript. This method allows for increased detection of closely related genes or splice variants.
In contrast to microarray methods, sequence-based approaches determine the cDNA sequence directly. Apart from being expensive and generally not quantitative, Sanger sequencing of cDNA or EST libraries is relatively low throughput [95, 96]. To overcome these limitations tag-based methods were developed, including serial analysis of gene expression (SAGE) [97, 98], cap analysis of gene expression (CAGE) [99, 100], and massively parallel signature sequencing (MPSS) [101–103]. These tag-based sequencing approaches have a high throughput and can provide precise, “digital” gene expression levels. However, most of these approaches are based on the rather cost-intensive Sanger sequencing technology. Furthermore, a significant proportion of the short tags cannot be uniquely mapped to the reference genome, only few transcripts can be analyzed, and isoforms are generally indistinguishable from each other. These disadvantages restrict the application of traditional sequencing technology in transcriptomics research. Like in all omics fields also for transcriptomics, development of novel high-throughput DNA sequencing methods has opened new possibilities for both mapping and quantifying of whole transcriptomes. This method, termed RNA-seq (RNA sequencing), has clear advantages over existing approaches and is expected to revolutionize the manner in which transcriptomes are analyzed. It has already been applied in several species like Saccharomyces cerevisiae, Schizosaccharomyces pombe, Arabidopsis thaliana, and mouse but also human cells [104–109].
RNA-seq uses deep-sequencing technologies. In general, a population of RNA is converted to a library of cDNA fragments with adaptors attached to one or both ends. Each molecule, with or without amplification, is then sequenced in a high-throughput manner to obtain short sequences derived from one end (single-end sequencing) or both ends (paired-end sequencing). The reads are typically 30–400 bp, depending on the DNA sequencing technology used. In principle, any high-throughput sequencing technology can be used for RNA-seq [110]. For example, Illumina IG [104–108], Applied Biosystems SOLiD [109], and Roche 454 Life Science [111, 112] systems have already been applied for this purpose. Following sequencing, the resulting reads are either aligned to a reference genome or reference transcripts or assembled de novo without the genomic sequence to produce a genome-scale transcription map that consists of both the transcriptional structure and/or level of expression for each gene. Although RNA-seq is still a technology under active development, it offers several key advantages over existing technologies. First, unlike hybridization-based approaches, RNA-seq is not limited to detecting transcripts that correspond to existing genomic sequence. RNA-seq can reveal the precise location of transcription boundaries, to a single-base resolution. Furthermore, 30-bp short reads from RNA-seq give information about how two exons are connected, whereas longer reads or paired-end short reads should reveal connectivity between multiple exons. These factors make RNA-seq useful for studying complex transcriptomes. In addition, RNA-seq can also reveal sequence variations (e.g., SNPs) in the transcribed regions [107, 109]. A second advantage of RNA-seq relative to DNA microarrays is that RNA-seq has very low background noise and DNA sequences can be unambiguously mapped to unique regions of the genome. There is no upper limit for quantification, which correlates with the number of sequences obtained. Consequently, RNA-seq has a large dynamic range of expression levels over which transcripts can be detected. For example, one study analyzed 16 million mapped reads corresponding to more than 9,000-fold, the estimated number in Saccharomyces cerevisiae analyzed [105], another study analyzing assessed 40 million mouse sequence reads making up a range spanning five orders of magnitude of what was estimated [106]. By contrast, DNA microarrays lack sensitivity for genes expressed either at low or very high levels. Determined by usage of quantitative PCR (qPCR), RNA-seq has been shown to be highly accurate for quantifying expression levels [105] and spike-in RNA controls of known concentrations [106]. The reproducibility is rather high, for both technical and biological replicates [105, 109]. Finally, because there are no cloning steps, and with the Helicos technology, there is also no amplification step, RNA-seq requires only small amounts of biomaterial. Taking all of these advantages into account, RNA-seq is the first sequencing-based method that allows for assessment of the entire transcriptome in a high-throughput and quantitative manner. This method offers both single-base resolution for annotation and “digital” gene expression levels at the genome scale, often at a much more cost efficient than either tiling arrays or large-scale Sanger EST sequencing.
The ideal method for transcriptomics should be able to directly identify and quantify all RNAs, small or large. Although there are only a few steps in RNA-seq, it does involve several manipulation stages during the production of cDNA libraries, which can complicate its use in profiling all types of transcript. Unlike miRNAs, piRNAs, and siRNAs, which can be directly sequenced after adaptor ligation, larger RNA molecules must be fragmented into smaller pieces (200–500 bp) to be compatible with most deep-sequencing technologies. Common fragmentation methods include RNA fragmentation by RNA hydrolysis or nebulization and cDNA fragmentation by DNase I treatment or sonication. Each of these methods creates a different bias in the outcome. For example, RNA fragmentation has little bias over the transcript body [106] but is depleted for transcript ends compared to other methods. Conversely, cDNA fragmentation is usually strongly biased towards the identification of sequences from the 3′ ends of transcripts and thereby provides valuable information about the precise identity of these ends [105]. Some manipulations during library construction also complicate the analysis of RNA-seq results. For example, many shorts reads that are identical to each other can be obtained from cDNA libraries that have been amplified. These could be a genuine reflection of abundant RNA species, or they could be PCR artifacts. One way to discriminate between these possibilities is to determine whether the same sequences are observed in different biological replicates. Another key consideration concerning library construction is whether or not to prepare strand-specific libraries [33, 109]. These libraries have the advantage of yielding information about the orientation of transcripts, which is valuable for transcriptome annotation, especially for regions with overlapping transcription from opposite directions [39, 104, 113]; however, strand-specific libraries are currently laborious to produce because they require many steps [109] or direct RNA–RNA ligation [33], which is inefficient. Moreover, it is essential to ensure that the antisense transcripts are not artifacts of reverse transcription [114]. Because of these complications, most studies thus far have analyzed cDNAs without strand information.
Proteomics
In addition to genetic predispositions and markers, changes in expression and function of proteins in an individual are influenced by their complex interplay within cells and organs and by environmental factors throughout the total life span. Analyzing the proteomic changes in the context of diabetes and obesity thus promises to gain a direct access to perturbances in relevant protein networks on the level of single cells or subcellular compartments of tissues or on a systemic level.
The main challenge for proteomic studies, however, comes from the extreme dynamics of proteomic changes and of the very high complexity of proteomes. Consequently, depth and coverage of proteomic studies have been mainly driven by development of technologies during the past two decades. The key enabling technology for studying proteomes is high-resolution peptide mass spectrometry coupled to a variety of pre-fractionation methods in order to cope with the very high complexity of proteomes.
In early times, a popular pre-fractionation method, the two-dimensional gel electrophoresis (2DE), was used to separate intact proteins extracted from a given cell, tissue, or organ by means of isoelectric point and molecular mass, resulting in complex maps of protein expression snapshots. This was combined with methods for relative quantification, the most advanced being 2D fluorescence difference gel electrophoresis (2DE-DIGE), where proteins from different conditions are labeled with different fluorophores and combined before separation by 2DE. Inclusion of a standard labeled with a third fluorophore enabled to further control for technical variations across samples. As a result, differential expression of proteins or posttranslationally modified protein isoforms could be detected, and identification of the respective proteins was performed by excision of a protein spot from the gel, digestion with endoproteinase trypsin, which proteolyses amino acid chains with strict sequence specificity after glutamine and arginine residues, and thus produced a protein-specific pattern of peptide fragments. These masses are then detected by matrix-assisted laser desorption ionization mass spectrometry (MALDI-MS) and compared to “in silico digests” of known proteins deposited in public databases. While this method offered the advantage of monitoring potential isoforms of a given protein by detecting shifts in mass or charge on the 2DE maps, it is severely restricted with respect to sensitivity and throughput and thus has been outperformed by other mass spectrometry (MS)-based methods (see below) [115].
Large-scale MS approaches, specifically liquid chromatography-tandem mass spectrometry (LC-MSMS), are nowadays used for both discovery of novel biomedical knowledge (“shotgun proteomics”) and for validation of potential biomarkers in high throughput. The typical discovery workflow using LC-MSMS comprises reproducible biological samples preparation potentially including pre-fractionation methods to reduce complexity of the proteome to be studied, protein digestion with trypsin, or other specific proteases, and then the resulting peptides are separated using liquid chromatography (so-called bottom-up approach). Separated peptides are typically directly injected into the mass spectrometer where the respective mass-over-charge ratios are detected. From these so-called survey scans, a subset of masses are selected for fragmentation and masses of fragments together with the “parent mass” are used to identify the respective peptide by searches against public databases. A key prerequisite to study proteomes, however, is the inclusion of relative or absolute quantification strategies, since only changes in proteomes in response to, e.g., environment or disease will enable advancement of systemic understanding. Several quantification methods for peptide-based proteomics are currently in place: stable isotope labeling by/with amino acids in cell culture (SILAC), which introduces metabolic labeling in appropriate biological samples, isotope-coded affinity tag (ICAT) and isotope-coded protein label (ICPL), which label intact proteins at specific sites, isobaric tags for relative and absolute quantitation (iTraq), which is used to label peptides and finally several label-free approaches [116]. A special and very successful variation of the shotgun proteomics approach is the combination of targeted purification of intact functional protein complexes with LC-MSMS with the aim to discover functions of novel proteins relevant for diseases (“interaction proteomics”) [117]. Shotgun proteomics experiments have the capacity to simultaneously detect thousands of proteins and when combined with additional pre-fractionation strategies, e.g., strong cation exchange (SCX) chromatography or off-gel fractionation (OGE) of peptides based on isoelectric points, the complexity of cellular proteomes can be nearly fully covered [118, 119]. However, the time and cost efforts for such full coverage experiments are profound and thus throughput and applicability of shotgun proteomics is limited to discovery projects aiming to reveal proteomic changes in response to very specific systems perturbations. Another disadvantage of shotgun proteomics is the missing data points during data-dependent acquisition. While high sensitivity of mass spectrometry theoretically enables to fully cover complex proteomes, the speed of the MS instruments still limits the capacity of recorded sequence spectra resulting in a limited coverage of simultaneously analyzed peptide masses. Both limitations of shotgun proteomics are overcome by advancements in targeted MS approaches, which aim to increase speed and sensitivity of MS by preselection of monitored proteins/peptides relevant for a biological/clinical question and, in addition, enable to introduce methods for absolute quantification. Targeted MS is based on selected reaction monitoring (SRM) assays, a process which records predefined peptides and predefined fragment ions for each peptide simultaneously in triple quadrupole mass spectrometers [120]. The transitions between the peptide and its fragments are strictly co-eluting, and area under curves for the fragments can be used as measure for relative intensities. Those SRM assays are predefined, optimized, and multiplexed and thus can be tailored to monitor changes of few to hundreds of proteins of interest for a specific context. Since relevant peptides are predefined, stable isotope-labeled peptides at known concentrations can be spiked into the experimental samples and thus enable absolute quantification for all monitored peptides. Advantages of SRM-based targeted proteomics are very high sensitivity, specificity, and throughput, while development of tailored multiplexed SRM assays is still quite time-consuming. Any mass spectrometry-based method is currently still limited in sensitivity as compared to methods involving application of specific high-affinity binders [121].
In diabetes research, proteomics methods have mainly been applied for identification of disease relevant proteins/pathways in tissues from animal models, e.g., lep/lep [122, 123] and db/db mice [124] or high-fat-diet-induced obesity in BL6 mice [125–130] or type 2 diabetes patients [131–134]. These studies (and many additional studies not cited here) have resulted in a comprehensive list of proteins which have been rated as being central to the condition of diabetes and are currently collected and published in a publicly available database (http://www.hdpp.info/hdpp-1000/) through the Human Diabetes Proteome Project (HDPP). The HDPP initiative was founded to integrate international research expertise enabling to generate systems-level insights into cellular changes by gathering multivariate data sets over time from cells and organs of healthy subjects and patients with diabetes [135]. Since application of proteomics methods in diabetes research covers very different areas with inherent huge complexity of approaches reflecting the complexity of the proteome as such, the HDPP initiative has identified some focus areas where proteomics methods are especially well suited to advance target discovery and functional understanding of disease pathogenesis. These areas comprise the Islet Human Diabetes Proteome Project (i-HDPP) aiming at a comprehensive islet expression data set, the human blood glycated proteome database, collecting qualitative and quantitative data on glycated proteins in plasma, and functional analyses of mitochondria, beta cells, insulin-producing cell lines, as well as bioinformatics and network biology approaches for data integration and systemic analyses (http://www.hdpp.info).
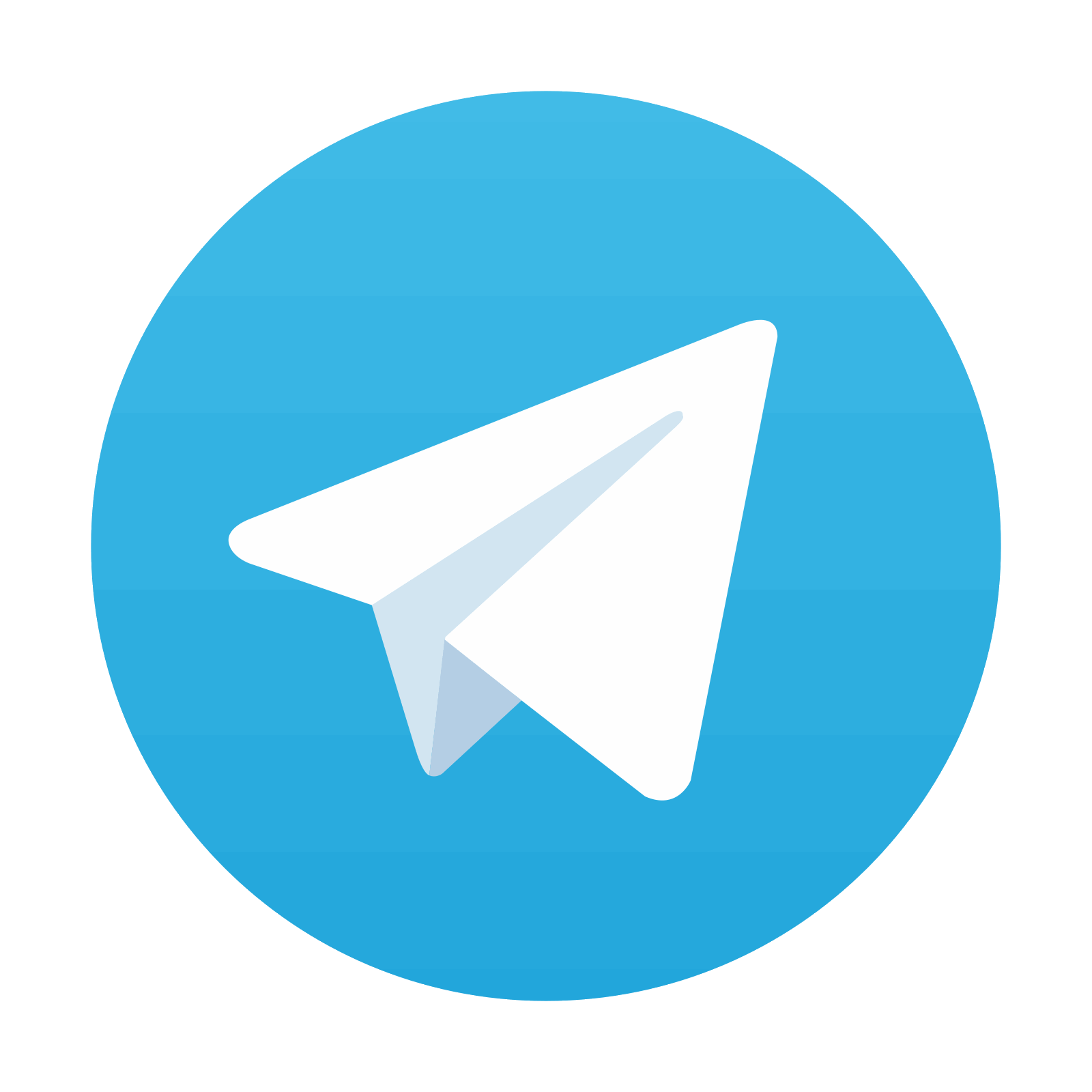
Stay updated, free articles. Join our Telegram channel

Full access? Get Clinical Tree
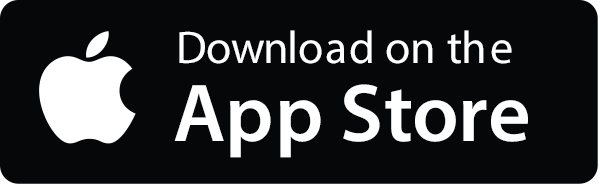
