Database
Curator
Publication
Gene Expression Omnibus (GEO)
National Center for Biotechnology Information (NCBI)
[111]
Riken Expression Array Database (READ)
Riken
[112]
ArrayTrack
U.S. Food and Drug Administration (FDA)
[113]
ArrayExpress
European Molecular Biology Laboratory – European Bioinformatics Institute (EMBL-EBI)
[114]
BioGPS
Genomics Institute of the Novartis Research Institute
[115]
Microarray Retriever (MaRe)
Leiden University Medical Center (LUMC)
[116]
The power of the various omics fields lies in the vast and detailed information that can now be extracted relatively quickly and easily from a biological sample. The integration of data from several areas of omics (e.g., genomics, proteomics, and metabolomics) can offer a more informative, holistic view of the system under investigation, as shown in Fig. 2.1. This should lead to advancements in disease prevention (genome sequencing) detection (biomarkers), better and more individualized treatments for patients (pharmacogenomic profiling), as well as a more thorough and accurate picture of disease prognosis (biomolecular profiling).
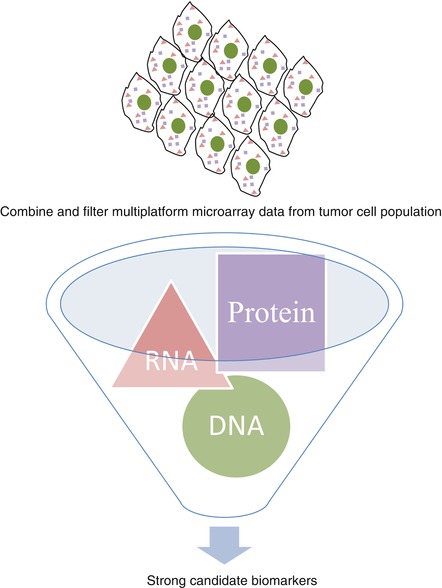
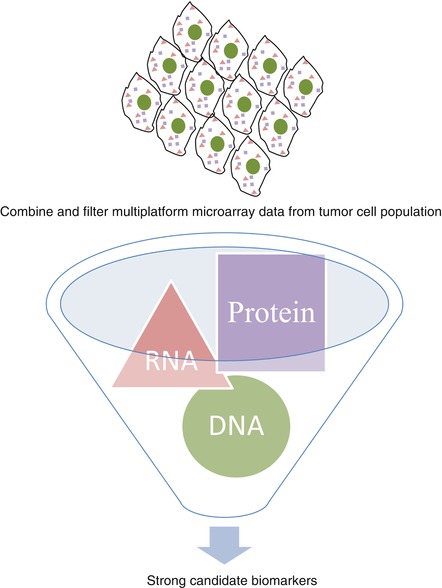
Fig. 2.1
Visual representation of the synthesis of high-throughput data for the identification of more robust predictive biomarkers
Although we saw in the first decade of the twenty-first century an explosion in new methods that allow for more detailed and comprehensive exploration of biological systems, improvements in both detection and analysis of omics data are needed [1, 3, 4]. Ultimately, in the context of medicine, the goal of the omics revolution is for a better understanding of pathophysiological processes and better prevention, detection, and treatment/management of disease. This chapter aims to describe some of the main omics methods currently utilized in cancer research and how they have contributed to our current understanding of hereditary breast cancer.
A Growing Problem
Cancer places a heavy economic burden on health-care systems, making the need for early detection and more effective treatments not only a medical imperative but also an economic one as well [5–7]. Current treatment regimens, while improved and often more targeted, are still harmful to healthy cells and tissues. This harm to healthy cells is responsible for unpleasant side effects and carries the possibility of causing secondary cancers [8–10].
Cancer is the leading cause of death in high-income nations and the second leading cause of death in nations of low to moderate income. For women, breast cancer is the leading cause of cancer-related death. In 2008, breast cancer accounted for 23 % of newly diagnosed cancers and 14 % of cancer-related deaths in women. Fifty percent of breast cancers are diagnosed in economically developing countries, and 60 % of breast cancer-related deaths worldwide occur in these nations, suggesting an even more urgent need for better early detection and targeted, cost-effective treatments [11, 12]. The impact of breast cancer on a patient and their family is both physically and emotionally devastating, and in some nations, like the United States, which lack a comprehensive social health care system, it can also be economically crippling for a family. While great strides forward have been made in early detection and identification of new treatments, there still remains much work to be done. A concentrated effort is required to improve our understanding of the genetic, biochemical, and environmental factors that contribute to the development of breast cancer.
Heterogeneity
One of the major challenges in understanding any form of cancer is the heterogeneity inherent in the disease [12]. In fact, the word “cancer,” while useful as a general descriptor, has led to a great deal of confusion and frustration among laypeople who may not be aware of the immense heterogeneity both between and within different cancer types. Owing to breakthroughs in understanding from the omics world, we have come to even better appreciate this aspect of cancer. Breast cancer is a particularly good example of why this term, while useful, is at the same time woefully inadequate [13]. Breast cancer is often broadly categorized as either hereditary or familial and sporadic. Tumors are also classified into subtypes based on various histological, genetic, and biomolecular characteristics. What has become increasingly clear in the past decade is that each tumor, while similar to others in many characteristics, is also unique. So while the search for new targets focuses on the similarities within subtypes, we must also remain aware of the unique nature of each tumor, which may make it resistant to any number of available therapies. The promise of the omics revolution is that routine, inexpensive molecular profiling of individual tumors will lead to truly personalized treatment modalities.
Oncogenic Transformation
Oncogenic transformation is a complex, multistep process that differs widely between and even within cancer types. However different each cancer case may be, common to all are the characteristics of oncogene activation and mutations in tumor suppressors and other genes involved in a multitude of different signaling pathways that cumulatively produce the phenotype of a cancer cell [14]. Monitoring biological samples (e.g., blood, serum, or urine) taken from high-risk candidates over time using global transcription, metabolomic, and proteomic methods may help us to understand the early changes that occur during this transformation. Some recent studies have utilized breast cancer cell lines and/or patient-derived samples in order to examine the global changes that occur during the transformation to metastasis with the aim of identifying more specific and sensitive biomarkers. The hope is that early identification and treatment can prevent a cancer’s advancement to metastasis [15]. Perhaps one day our understanding of the disease along with advancements in detection will even allow us to detect oncogenic transformation at a stage where its progression to cancer can even be blocked.
Four omics disciplines and their contributions to our understanding of hereditary breast cancer will be described in this chapter: genomics, transcriptomics, proteomics, and metabolomics. The order in which they are presented is meant to represent the flow of cellular information from genomics, the relatively fixed, molecular code of life; to transcriptomics, the first step in translating this code into “usable” parts; to proteomics, representing the workhorses of cellular activity; and finally ending with metabolomics, the downstream “end products” of the myriad cellular processes carried out by the aforementioned molecules.
Cancer Genomics
The discipline of genomics, as it is known today, started with the invention of DNA cloning in the 1970s and then the sequencing of the human genome [16]. “Classical” genomics is primarily concerned with the sequencing of genomes, the identification of all genes contained within a particular genome, and understanding gene structure and the complex interplay between genes and environment. There are now many subdisciplines within this field, such as structural and functional genomics, epigenomics, and pharmaco- and toxicogenomics. All aim to better understand the relationship between genetic sequences and biological processes or outcomes.
We are now living in the so-called “post-genomic” age. Gene mutations that increase a person’s risk of developing various types of cancer have been identified. In high-risk breast cancer families, genetic screening can be carried out so that preventive measures can be taken, such as lifestyle changes, beginning mammograms at an earlier age, or prophylactic mastectomy [17–20].
Genomics of Hereditary Breast Cancer
Many attempts have been made to classify breast cancers into meaningful subgroups to aid in diagnosis, optimal treatment determination, and prognosis. Breast cancer tumor classification systems have evolved over time as our understanding of the heterogeneity of this disease has increased. Breast cancer tumors may be separated into four main types based on clinical and therapeutic characteristics. The luminal group is the most numerous and diverse subtype and is often subclassified into luminal A and luminal B, and several genomic tests are available to predict outcomes to endocrine therapy. The second group is the human epidermal growth receptor 2 (HER2 or ERBB2) amplified or HER-2 enriched group, which has responded very well to targeting of HER2 with monoclonal antibodies. The third group is referred to as normal breastlike. The fourth group is referred to as triple negative (or basal like) and is so called because they lack estrogen receptor (ER), progesterone receptor (PR), and HER2 expression [21]. They have higher incidence in patients with germline BRCA1 mutations or who are of African ancestry and account for about 15 % of all breast cancer [22].
In 2009, Parker et al. reported subtype prediction by 50 genes using qRT-PCR and microarray technology, which came to be known as the Prediction Analysis of Microarray 50 (PAM50) and is commonly used to predict the best treatment modalities for individual cases [23]. In 2011, Ebbert et al. reported that the PAM50 system is generally accurate and that the assay is resistant to errors in the multivariate analyses (MVAs) used for classification. However, in the case of tumors that do not fit existing parameters very well, the system can lead to inaccurate conclusions [24]. In 2012, the IMPAKT task force compared the effectiveness of the PAM50 assay with a three-gene immunohistochemical (IHC) approach using antibodies against ER, HER2, and Ki67 and found that the former was “insufficiently robust” to make systemic treatment decisions. They recommend instead the combined use of ER and HER2 IHC.
In addition to the PAM50, there are germline genetic tests for BRCA1, BRCA2, and CYP2D6 and the Breast Cancer Index (BCI). OncotypeDX and MammaPrint assays are used in the United States and Europe for clinical decision-making [25]. Recently, more extensive and meaningful subgrouping has been made possible by genomic (as well as other omic) profiling of large sample groups [12, 26]. Such subtyping is essential for identifying and applying rational treatment combinations.
The first genes to be associated with hereditary breast cancer are also probably the best known. These breast cancer susceptibility genes, BRCA1 and BRCA2, are inherited in an autosomal dominant fashion and have high penetrance [27, 28]. Together, they account for about 30 % of familial cases of breast cancer [29]. Germline mutations in these genes result in what is called hereditary breast and ovarian cancer (HBOC) syndrome, which is associated with a lifetime risk of developing breast cancer of 50–80 % and of 30–50 % for ovarian cancer [30]. Interestingly, although primarily associated with breast cancer, the BRCA genes are more highly associated with ovarian cancer, with an overall mutation rate of about 12 % in women diagnosed with ovarian cancer [31]. After their identification, there was a great deal of excitement, with many hoping that more high-penetrance genes would be discovered. However, this has not been the case and this is one reason why many have great hope for advancements in understanding breast cancer via omics methodologies.
Although the two BRCA genes function in the same DNA repair pathway, homology-directed recombination repair (Fig. 2.2), the tumors that result from BRCA1 and BRCA2 mutation are remarkably different. BRCA2 tumors have characteristics similar to sporadic cases. BRCA1 tumors, on the other hand, are uniformly aggressive, difficult to treat, and are typically ER negative [30, 32]. In a recent review, Roy et al. propose several theories to explain why tumors arising from two genes involved in the same DNA repair pathway may vary so significantly, both genetically and clinically. It is possible that other gene mutations or polymorphisms are co-inherited with BRCA1; although, they note, there is no evidence currently available to support this. Another possibility they propose is that the role of BRCA1 in transcriptional co-activation or co-repression, which is not shared by BRCA2, may be able to modify expression of the ER biomarker. For this to be proven correct, the expression profiles of ER-negative BRCA1 and ER-negative sporadic tumors would need to be compared to identify a common mechanism. Finally, they suggest the possibility that BRCA1 and BRCA2 heterozygosity induce different mutational spectrums, perhaps resulting from their different roles in homologous recombination (HR) repair. Analyses using array-comparative genome hybridization (aCGH) show some similarities between BRCA1 and BRCA2 cancers, including large deletions and amplifications, but some differences are also seen [30].
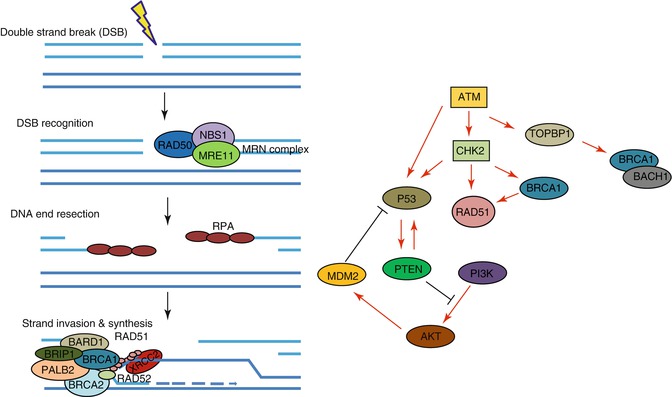
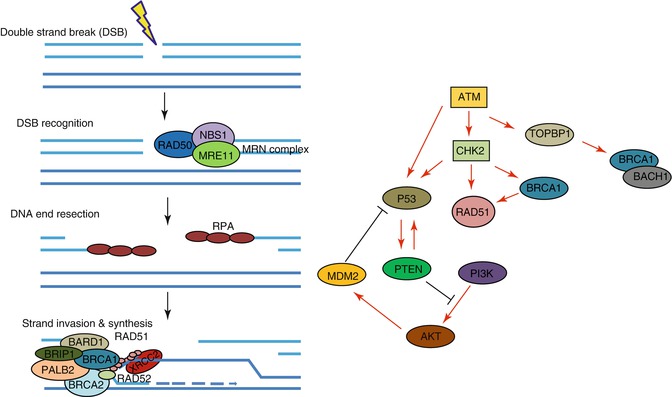
Fig. 2.2
Relationship between various genes involved in/implicated in the development and/or progression of breast cancer. Role of several proteins implicated in breast cancer susceptibility in homologous recombination directed repair of double strand breaks (DSBs) (left); Signaling between various proteins implicated in breast cancer susceptibility (right). Gene/protein abbreviations: RAD50 RAD50 homolog (S. cerevisiae), NBS1 Nijmegen breakage syndrome protein 1, MRE11 MRE11 meiotic recombination 11 homolog (S. cerevisiae), RPA replication protein A, BARD1 BRCA1 associated RING domain 1, BRIP1 BRCA1 interacting protein C-terminal helicase 1, PALB2 partner and localizer of BRCA2, BRCA1 breast cancer 1, early onset, BRCA2 breast cancer 2, early onset, XRCC2 X-ray repair complementing defective in Chinese hamster cells 2, RAD51 RAD51 homolog (S. cerevisiae), RAD52 RAD52 homolog (S. cerevisiae), ATM ataxia telangiectasia mutated, CHK2 checkpoint kinase 2, Topoisomerase (DNA) II binding protein 1, P53 tumor protein p53, PTEN phosphatase and tensin homolog, AKT v-akt murine thymoma viral oncogene homolog 1, MDM2 MDM2 oncogene, E3 ubiquitin protein kinase, PI3K phosphatidylinositol-4,5 bisphosphate 3-kinase, catalytic subunit alpha, BACH1 BTB and CNC homology 1, basic leucine zipper transcription factor 1
In a response to this review, Simon A. Joosse puts forward an intriguing alternative to these explanations. He starts by noting that all mammary stem cells, from which all epithelial breast cells originate, begin as ER negative and that BRCA1, but not BRCA2, is required for their maturation to ER-positive cells. Thus, BRCA1 deficiency may result in an accumulation of undifferentiated ER-negative stem cells with oncogenic potential. Following this line of thought, BRCA1 tumors would originate from a common cell lineage, explaining why they are so homogeneous as compared to tumors in BRCA2 mutation carriers. It could also explain why BRCA1 mutation carriers have a higher overall risk for developing breast cancer and why they are typically diagnosed at an earlier age than BRCA2 mutation carriers [32]. Further genomic and proteomic analyses will hopefully provide answers to these questions in the near future.
Apart from the BRCA genes, several others have been associated with familial cases of breast cancer. Among the high-penetrance genes are the tumor suppressors P53, PTEN, and STK11 [33–35]. Also implicated are genes of moderate penetrance, which include ATM, CHK2, RAD51D, and RAD51B [36–39]. Other moderate-penetrance genes that are part of the Fanconi anemia (FA) pathway are PALB2, BRIP1, RAD51C, and XRCC2 [38, 40–43]. A further 21 low-risk alleles have been identified [44–47]. Together these genes, including BRCA1 and BRCA2, account for approximately 35 % of all familial breast cancer worldwide. This leaves a gaping hole in our knowledge of what causes the majority of familial breast cancer; a hole that is slowly being filled with valuable omics data. (See Table 2.2 for a list of genes mentioned in this chapter, including brief descriptions.)
Table 2.2
Brief description of genes reviewed in this chapter: their basic function and role in various cancer types
Gene name | Function | Role in various cancer types |
---|---|---|
PPP2R2A (Protein phosphatase 2, regulatory subunit B, alpha) | Negative control of cell growth and cell division | |
MTAP (methylthioadenosine phosphorylase) | Polyamine metabolism, adenine and methionine scavenger | Loss is common in human cancer, addition of MTA to MTAP negative tumor cells increased sensitivity to 6TG and 5FU without affecting MTAP positive cells [145] |
MAP2K4 (MKK4) (mitogen-activated protein kinase kinase 4) | Serine threonine protein kinase, phosphorylates and activates JNK | Metastasis repressor in prostate and ovarian cancers [146] |
STK11 (LKB1) (serine/threonine kinase 11) | Serine threonine protein kinase, regulates cell polarity, tumor suppressor | |
DUSP4 (MKP2) (dual-specificity phosphatase 4) | Negatively regulates ERK, p38 and JNK | |
RUNX1 (AML1 or CBFA2) (runt-related transcription factor 1) | Transcription factor regulating differentiation of HSCs, ERα antagonist | |
AMBP (alpha-1-microglobulin/bikunin precursor) | Found in complexes with prothrombin, albumin, and immunoglobulin A (CD79a) in plasma | Differentially expressed in bladder cancer [153] |
ABAT (4-aminobutyrate aminotransferase) | Catabolizes neurotransmitter GABA into succinic semialdehyde | Differentially expressed in ARMS |
CDH1 (cadherin 1, type 1, E-cadherin (epithelial)) | Suppresses re-accumulation of mitotic cyclins by recruiting them to APC for ubiquitination and subsequent proteolysis | |
RB1 (retinoblastoma 1) | Tumor suppressor, regulates cell growth, interacts with proteins involved in apoptosis and differentiation | |
HSP90 (Hsp90 chaperone) | Role in folding and activating proteins in various signal transduction pathways | |
PIK3CA (phosphatidylinositol-4,5-bisphosphate 3-kinase, catalytic subunit alpha) | Gene with highest frequency of gain-of-function mutations in breast cancer [160] | |
MLL3 (myeloid/lymphoid or mixed-lineage leukemia 3) | Histone methyltransferase, involved in circadian transcription | |
GATA3 (GATA binding protein 3) | Transcription factor regulating luminal epithelial cell differentiation in the mammary gland, involved in regulation of T-cell development | |
MAP3K1 (mitogen-activated protein kinase kinase kinase 1, E3 ubiquitin protein ligase) | Serine threonine kinase | Correlated with breast cancer susceptibility in BRCA2 carriers [167] |
CDKN1B (P27KIP1) (cyclin-dependent kinase inhibitor 1B) | Controls cell cycle progression at G1 by binding cyclin E-CDK2 or cyclin D-CDK4 complexes | |
TBX3 (T-box 3) | Transcription repressor | |
CBFB (core-binding factor, beta subunit) | Beta subunit of a core-binding transcription factor complex involved in development and stem-cell homeostasis | |
NF1 (neurofibromin 1) | Negative regulator of several signal transduction pathway involved in proliferation, including Ras pathway | |
SF3B1 (splicing factor 3b, subunit 1, 155 kDa) | Subunit of U2 snRNP complex and minor U12-type spliceosome | |
CCND3 (cyclin D3) | Forms regulatory subunit of CDK4 and CDK6 which are required for G1/S cell cycle transition | |
PDZK1 (PDZ domain containing 1) | Scaffolding protein mediating localization of cell surface proteins and involved in cholesterol metabolism | |
PTX3 (pentraxin 3, long) | Involved in innate immunity and extracellular matrix formation | May be used as FGF2 antagonist in tumor cells resistant to anti-VEFG therapy [188] |
Gracia-Aznarez et al. analyzed seven BRCA1/BRCA2-negative families, each with six to ten affected family members across generations who were diagnosed under the age of 60. A known moderate susceptibility indel variant, CHEK2 1100delC, was identified. CHEK2 (or CHK2) is a gene integral to cell cycle checkpoint regulation and is found within the same signaling pathway as ATM and p53 (see Fig. 2.2). Additionally, 11 rare variants were identified, although their association with breast cancer was not clear due to insufficient statistical power. Targeted re-sequencing of these gene candidates would need to be carried out in a larger cohort to determine whether or not an association actually exists [48].
In 2011, Rebbeck et al. published the results of a study in which they analyze a set of genes known to code for BRCA1 interacting proteins in 2,825 BRCA1 mutation carriers to try to identify breast cancer risk-modifying genes. The following genes were identified as potential modifiers: ATM, BRCC45, BRIP1, CTIP, MERIT40, NBS1, RAD50, and TOPBP1 [49]. ATM, BRIP1, NBS1, and RAD50 had previously been associated with hereditary breast cancer cases [50]. Mutation screenings of the MERIT40 and TOPBP1 gene had been previously carried out by the Winqvist group. In their work, MERIT40 was not found to be associated with disease in familial breast cancer cases. However, the sample size of the study was relatively small (125 families) and geographically limited (families originating in northern Finland); thus, their results may not be relevant to other populations [51]. The same group had performed a similar study in 2006 in which they examined TOPBP1 and identified several variants in familial breast cancer cases [52]. Two other studies examined the possible role of TOPBP1 in modifying breast cancer risk. The first found aberrant subcellular localization of the protein in breast carcinoma from an unselected consecutive cohort of 61 patients [53] and the other, specifically examining familial breast cancer cases in Poland, found that decreased mRNA levels and increased protein levels of TOPBP1 were associated with disease progression [54].
The power of genomic analysis is well illustrated in a study published by Banerji et al. in 2012 in which whole-exome sequences of 103 breast cancers from patients in Mexico and Vietnam were compared to matched normal DNA. They also performed whole-genome sequencing for 22 breast cancer/normal pairs. Results confirmed a number of previously identified somatic mutations as well as discovering some new mutations, including a recurrent MAGI3-AKT3 fusion enriched in triple-negative breast cancers. The fusion causes constitutive activation of AKT kinase. They found that treatment with an AKT small-molecule inhibitor was able to abolish AKT activation [55]. Although this work does not specifically assess cases of hereditary breast cancer, it illustrates the powerfully informative nature of high-throughput sequencing technologies that are now at many researchers’ disposal.
DNA methylation is an epigenetic mechanism that is thought to contribute to the control of gene expression [56]. In a recent review the possibility of targeting epigenetic enzymes to specific DNA sequences to attain a more thorough understanding of epigenetic effects on gene expression was discussed [57]. With recent major advances in genome editing, it seems that epigenomic editing may be a reality in the near future [58, 59]. Recent research linking DNA methylation of particular genes with breast and other types of cancers suggests that the ability to edit epigenetic marks could be immensely useful both to basic and translational cancer research [60–63].
Swift-Scanlan et al. reported the use of quantitative multiplex-methylation specific PCR (QM-MSP) to examine the precise levels of methylation of genes known to be hypermethylated in breast cancer [64]. In a set of 99 formalin-fixed archival breast cancer tissue samples from patients with germline mutations in BRCA1 or BRCA2 and/or a family history of breast cancer, the authors were able to identify associations between levels of DNA methylation in several genes (APC, RASSF1A, TWIST, ERα, CDH1, and cyclin D2) and tumor stage, hormone receptor status, growth receptor status, and history of recurrent or metastatic disease. While not as high throughput as other methods, QM-MSP is very sensitive, allowing analysis of samples that are very limited in size (50–1,000 cells) [64]. Other studies investigating DNA methylation in breast cancer have found that GSTP1 and FOXC1 promoter methylation status could be used as a prognostic marker [65].
Another interesting and promising method that is increasingly being utilized is single-nucleus sequencing (SNS) from flow-sorted nuclei. This has clearly illustrated that tumors are composed of a number of distinct subpopulations, each with unique genetic characteristics but also shared genomic mutations. These various subpopulations may then travel to different parts of the body, forming genetically distinct metastases [15].
According to a number of different studies, the majority of mutations present in metastases are also present in the primary tumor [66]. This is potentially good news in that the transformation to metastasis may be more easily inhibited than previously thought. Then again, cancer is a very “smart” disease with quickly evolving genetic characteristics allowing tumor cells, even if only a small subpopulation, to escape our attempts at its eradication.
Cancer Transcriptomics
With the development of microarray technologies and advanced bioinformatics analysis software, the focus of many researchers turned to such efforts as identifying patterns of differential gene expression in cells under various conditions. In 1999, Golub et al. showed that identification of tumor subtypes could be carried out using global gene expression data rather than histological and clinical observations [67, 68]. However, some recent reports suggest that a combination of various methods is currently the most effective way to make accurate diagnoses and prognoses [69].
Transcriptomics is the study of all the transcripts of a particular organism, including mRNAs, small RNAs (microRNA and siRNA), and noncoding RNAs. It also includes the characterization of transcriptional structure, splicing patterns, and other posttranscriptional modifications of genes. The characterization of differential gene expression between different types of cells or in the same cell type under variable conditions is an invaluable tool in cancer research. The gene expression profile of a cancer cell is strikingly different from surrounding noncancerous cells. It can also be used to define tumor subtypes for a variety of different cancers, including breast cancer [21, 70–73]. Examination of the changes in gene expression between cells of primary and metastatic tumors adds to our understanding of the mechanisms underlying metastatic transformation.
While traditional sequencing techniques have been modified for transcriptional profiling (serial analysis of gene expression (SAGE), cap analysis of gene expression (CAGE), massively parallel signature sequencing (MPSS)), these methods are low throughput, expensive, and imprecise. Microarray and gene chip technologies are currently the main tools of choice in this omics field. RNA-Seq (RNA sequencing), a relatively recent development, is a method that uses deep sequencing technology and has vastly improved precision in transcript measurement, does not rely on known genomic sequences, and can identify single nucleotide polymorphisms (SNPs) present in transcripts [74].
Transcriptomics of Hereditary Breast Cancer
The heterogeneity inherent in triple-negative breast cancer (TNBC), frequently associated with BRCA1 germline mutations, makes it an especially intractable disease. A recent study published by Cascione et al. examined miRNA and mRNA expression profiles of samples (formalin fixed and paraffin embedded) that had been obtained from women with TNBC between 1995 and 2005 (see Table 2.3 for a complete list of the miRNAs identified). Samples were obtained from tumor, adjacent non-tumor, and lymph node metastatic lesions from 173 patients. Due to low RNA yield, a somewhat limited array analysis was necessary. A human cancer-specific mRNA array and the human miRNA expression profiling v1 panel were used. Two miRNA signatures were linked to patient survival (miR-16, 155, 125b, 374a and miR-16, 125b, 374a, 374b, 421, 655 497) and miRNA/mRNA anticorrelations were used to identify four distinct molecular subclasses. One (subclass) group included seven mRNAs overexpressed in tumors compared to normal tissue (SPP1, MMP9, MYBL2, BIRC5, TOP2A, CDC2, and CDKN2A). The second group had 43 mRNAs downregulated in tumors with the top gene ontologies being enriched in NF-κB, PPAR, and PTEN signaling pathways. The third group had ten deregulated mRNAs and was enriched with gene ontologies associated with growth factors. Finally, the fourth group is composed of 64 mRNAs with NF-κB signaling pathway as the most enriched gene ontology [22].
Table 2.3
Deregulation of miRNAs identified by Cascione et al. (in TNBC expression signatures)
miRNA | Dereguation of identified miRNAs in other types of cancer cells |
---|---|
miR-16 | Prostate [117] |
Myeloma [118] | |
Breast [119] | |
Colon [120] | |
Oral [121] | |
Lung [122] | |
Liver [123] | |
Brain [124] | |
miR-155 | Colon, cervix, pancreas, lung, thyroid, lymphoma, leukemia [125] |
Pancreas [126] | |
miR-374a | Lung [127] |
Breast [128] | |
Colon [129] | |
miR-421 | Head and neck [130] |
Stomach [131] | |
Liver [132] | |
Pancreas [133] | |
Prostate [134] | |
Breast [135] | |
miR-497 | Cervix [136] |
Breast [137] | |
Skin [138] | |
Colon [139] | |
Brain [140] | |
Head and neck [141] | |
Stomach, lung [142] |
Adjuvant and neoadjuvant treatments are often coupled with primary therapeutic modalities with the aim of improving the effectiveness of the primary therapy. For tumors that do not respond well to treatment, there is a good chance of disease recurrence and/or progression. Some theorize that this is due to the difficulty of eradicating tumor cells especially resistant to cancer therapy, what are commonly referred to as cancer stem cells [75]. To identify genes associated with drug resistance in TNBC (also referred to in the paper as basal-like breast cancer, BLBC), transcriptional profiling was performed on 49 archival samples that had been surgically resected following neoadjuvant treatment. To estimate long-term clinical outcome, IHC staining with Ki67 (a commonly used marker of proliferation) was performed on all samples. Ki67 staining highly correlated with tumor subtype, both clinically and molecularly, with the highest positive staining observed in BLBC cases. Expression profiling data from BLBC samples with high Ki67 staining, when compared with the Molecular Signatures Database, indicated activation of the Ras-ERK pathway.
To rule out KRAS mutation, which is infrequent in breast cancer, DNA sequencing was performed and no mutations were found. However, expression of DUSP4, a negative regulator of the Ras-ERK pathway, was significantly downregulated in these samples. Low DUSP4 expression had previously been correlated with shorter DFS in a cohort of 286 patients who had not received adjuvant therapy. To further verify the significance of DUSP4, the authors measured its expression in another cohort composed of samples obtained from 89 TNBC patients after neoadjuvant treatment and found a similar pattern of high Ki67 staining together with low DUSP4 expression. Experiments conducted in BLBC cell lines with siRNA knockdown of DUSP4 resulted in decreased apoptosis, increased mitogen-activated protein kinase (MEK)-dependent proliferation, and an increased half-maximal inhibitory concentration (IC50) of docetaxel, an antimitotic drug used in the clinic.
After restoring DUSP4 expression in three BLBC cell lines, phosphorylation of ERK was inhibited and viability in two of the three cell lines was reduced. The addition of MEK inhibitors was found to increase sensitivity to docetaxel in 17 BLBC cell lines. In cell lines with loss of PTEN expression, the PI3K pathway is activated resulting in what is likely MEK-independent proliferation and evasion of apoptosis. Thus, the authors conclude DUSP4 expression coupled with PTEN status may effectively predict efficacy of MEK inhibitors in patients with BLBC tumors [76].
Taking advantage of the vast number of tumor samples available in tissue banks, Curtis et al. carried out an analysis of copy number variation and its effects on the transcriptome using a discovery set of 997 fresh-frozen primary breast tumor samples with accompanying clinical information. Another set of 995 tumors was then used as a test set to verify the predictive ability of data gleaned from the discovery set. The aim was to identify the underlying genetic mechanisms that translate into observed variance between and among breast cancer subgroups. Patients were clinically homogenous with most ER-positive/lymph node (LN)-negative patients having not received treatment while ER-negative/LN-positive patients had received treatment. A number of putative cancer genes were identified including PPP2R2A, MTAP, and MAP2K4. The patients also stratified into a high-risk, ER-positive 11q13/14 cis-acting subgroup and a subgroup without any copy number aberrations, which corresponded to favorable prognosis, providing a new method for identifying breast cancer subgroups [77].
Cancer Proteomics
Proteomics is the study of the entire complement of proteins expressed by a particular biological system. As with genomics, subspecialties of proteomics have developed. The four major subfields are expression proteomics, functional proteomics, structural proteomics, and the proteomics of posttranslational modifications [78]. The aim of proteomics is not only to identify all proteins in a particular system, but also to understand the regulation of their expression, the interactions that occur between them, and their effects on cellular function. An example of one of the many programs available for visualizing protein-protein interactions is presented in Fig. 2.3.
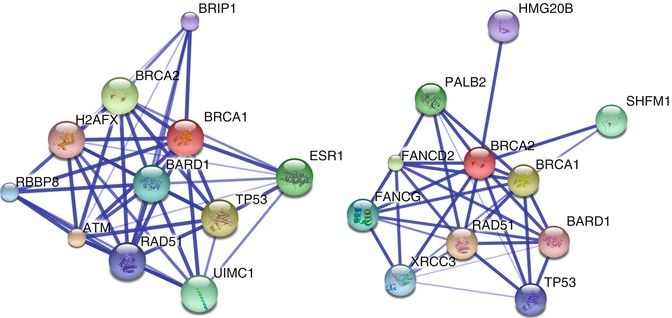
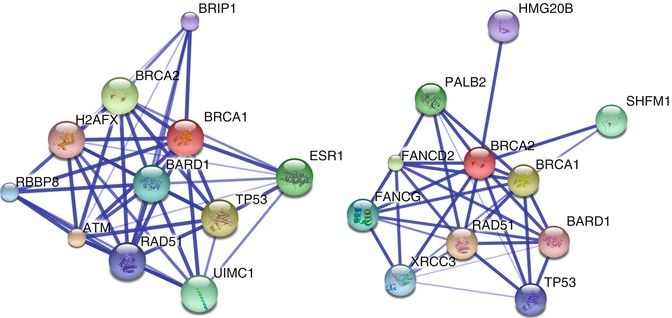
Fig. 2.3
Protein interaction networks for BRCA1 (left) and BRCA2 (right) generated using Search Tool for the Retrieval of Interacting Proteins/Genes (STRING) 9.05. The thickness of lines represents strength of association, with thicker lines indicating stronger associations
The primary method in the proteomics toolbox is mass spectrometry (MS), a technology that measures the mass-to-charge ratio of ions in the gas phase. Throughout the twentieth century, MS technologies developed, but it was not until the late 1980s that its widespread use in biological research became feasible. This was made possible by the development of electron spray ionization (ESI) and matrix-assisted laser desorption/ionization (MALDI) [78, 79].
A recent review outlines the main challenges facing the field of proteomics. According to the authors, the primary bottleneck in proteomics development is in data analysis [3]. For a review of available methods for MS data analysis and protein identification via database search, we refer the reader to Brusniak et al. and Eng et al., respectively [80, 81]. For a basic look at how to analyze protein-protein interaction networks and regulatory networks, we recommend Koh et al. and Poultney et al. [82, 83]. Finally, for integrated analysis of omics data from multiple platforms, the reader is referred to Chavan et al. [84].
Proteomics of Hereditary Breast Cancer
Cohen et al. examined plasma from 76 breast cancer patients and were able to identify a signature consisting of four proteins previously found to be associated with breast cancer tissue: fibronectin, clusterin, gelsolin, and α-1-microglobulin/inter-α-trypsin inhibitor light chain precursor (AMBP). The plasma levels of these proteins differed between the two tumor types such that they were able to distinguish between infiltrating ductal and invasive mammary breast carcinomas [85].
A powerful methodology for identifying putative breast cancer biomarkers was used in a recent study by Pavlou et al. First, proteomic data was collected from the secretome (the full complement of proteins secreted by a cell) of eight different breast cancer cell lines, representing the three major breast cancer tumor subtypes. Out of 5,200 nonredundant proteins identified by MS, 23 were unique to basal breast cancer cells, 4 were unique to HER2-neu-amplified, and another 4 were unique to luminal breast cancer cells. These results were then compared with four publicly available breast cancer mRNA microarray data sets queried from the National Center for Biotechnology Information Gene Expression Omnibus (NCBI GEO). In total, 24 out of the 30 candidate proteins had microarray expression patterns similar to those identified in the proteomic approach.
They next tested the clinical applicability of this data by performing MS on cytosol collected from eight ER-positive and eight ER-negative breast cancer tissue samples. Eighteen out of the 30 subtype-specific proteins were identified and three proteins in particular (ABAT, PDZK1, and PTX3) had significantly different expression in the different subtypes. Finally, they examined the 2-year and 5-year disease-free survival (DFS) data that accompanied the four gene expression array data sets. ABAT was the most robust candidate of the three potential biomarkers. Expression levels of ABAT were, on average, 2.3 times higher for patients with DFS of more than 2 years. ABAT expression remained significantly different in all four datasets at the 5-year DFS mark.
They also queried the Gene Expression-Based Outcome for Breast Cancer Online Database and found that patients with higher ABAT expression had slightly longer disease-free survival than those with low expression. Patients with ER-positive disease and high ABAT expression as well as tamoxifen-treated patients with high ABAT expression had better prognosis than those with low expression [86]. This work demonstrates that in vitro proteomic analysis of breast cancer cell lines combined with publicly available transcriptomic data from patients can be used to successfully identify new candidate biomarkers that are breast cancer subtype specific.
Lee et al. carried out protein expression profiling of 38 sample pairs from lymph node metastases of varying grades (classified according to the TNM staging system, which includes physical examination, biopsy, and imaging) alongside adjacent normal tissue collected from patients with infiltrating ductal carcinoma (IDC). Using two-dimensional polyacrylamide gel electrophoresis (2D-PAGE) and high-performance liquid chromatography and tandem mass spectrometry (LC-MS/MS), they found a number of proteins upregulated specifically in metastatic tissue and also identified possible markers to distinguish between the various metastatic stages. Calreticulin was significantly upregulated in metastases of all three stages with a rate of 77 % in stage N0, 92 % in stage N1, and 83 % in stage N2. Tropomyosin alpha-3 chain was also upregulated in all three stages, albeit at lower overall incidence (N0 and N1 69 %, N2 75 %). They suggest that HSP70 is a possible marker for stage N0 metastases, 80 k protein H precursor and PDI may serve as biomarkers for N1 stage metastases, and immunoglobulin heavy chain binding protein (BIP) is a potential identifier of stage N2 metastases [87].
Another study aimed at stratifying tumors into subgroups based on protein expression profiles found increased expression of STAT1 and CD74 to be associated with metastatic potential in TNBC, both in patient samples and in MDA-MB-231 cells. The authors suggest that the mechanism by which this increased capability occurs is likely the CD74/CD44/ERK, MIF receptor pathway with a positive feedback loop between CD74 and STAT1 [88].
Cancer Metabolomics
Metabolomics is another piece of the omics puzzle that will improve our understanding of cancer cells and their transformation to the metastatic state. Metabolomics may be defined as “the comprehensive analysis of the low-molecular-weight molecules, or metabolites, that are the intermediates and products of metabolism” [89]. The Human Metabolome Database (www.hmdb.ca) is a publicly available collection of detailed information about the 40,250 small-molecule metabolites that have been thus far identified in human cells. “The large number of different metabolites, differences in their relative concentrations and variability in their physicochemical properties (polarity, hydrophobicity, molecular mass or chemical stability) require the application of different technologies and a huge range of experimental conditions” [90]. The most common techniques used in metabolomic profiling are nuclear magnetic resonance (NMR) and mass spectroscopy (MS) [89].
While metabolomics, like the other omics disciplines, offers great hope, it also comes with many challenges. The complete human metabolome is very large, almost twice that of the human proteome, and they exist in a constant dynamic flux. While collection of samples for metabolomic analysis is relatively easy and noninvasive (typically serum, plasma, or urine), because the molecules of interest are so easily modified during the process of sample transport and preparation, this presents potential variability that may be very difficult to control for. For translation to use in medicine, standard protocols and conditions for collection, storage, and processing must be designed and strictly adhered to.
One of the distinguishing characteristics of cancer cells is their unique “reprogramming” of metabolic pathways, in which they acquire changes that affect the metabolism of the four major types of macromolecules (carbohydrates, lipids, proteins, and nucleic acids) [14, 91, 92]. This phenomenon was first formally described by Otto Warburg in the 1920s and refers specifically to a cancer cell’s “preference” for performing glycolysis even in the presence of oxygen (aerobic glycolysis) [14, 93, 94]. Known as the Warburg effect, this characteristic of “glucose addiction” is exploited in the clinic for the identification of cancerous lesions. Positron emission tomography (PET) is used to detect radioactively labeled glucose (2-deoxy-2-[18F]fluoro-D-glucose, FDG), which accumulates more in tumor cells relative to other cells due to their heavy reliance on glycolysis [93]. This has helped thrust cancer metabolism back into the spotlight in recent years. Evidence that activated oncogenes and mutant tumor suppressors can impact metabolism has also helped feed this interest. Nature and Nature Reviews Cancer published a “Web focus” on cancer metabolism where they highlight some recent developments in the field [27]. One review notes that the oxygen and nutrient-rich environment in which cancer cell lines are typically maintained and studied is markedly different from the in vivo tumor microenvironment [91]. Cocultures of breast cancer cells with fibroblasts may more accurately reflect the conditions in which tumors grow and can add to our understanding of how cancer cells evade death during treatment.
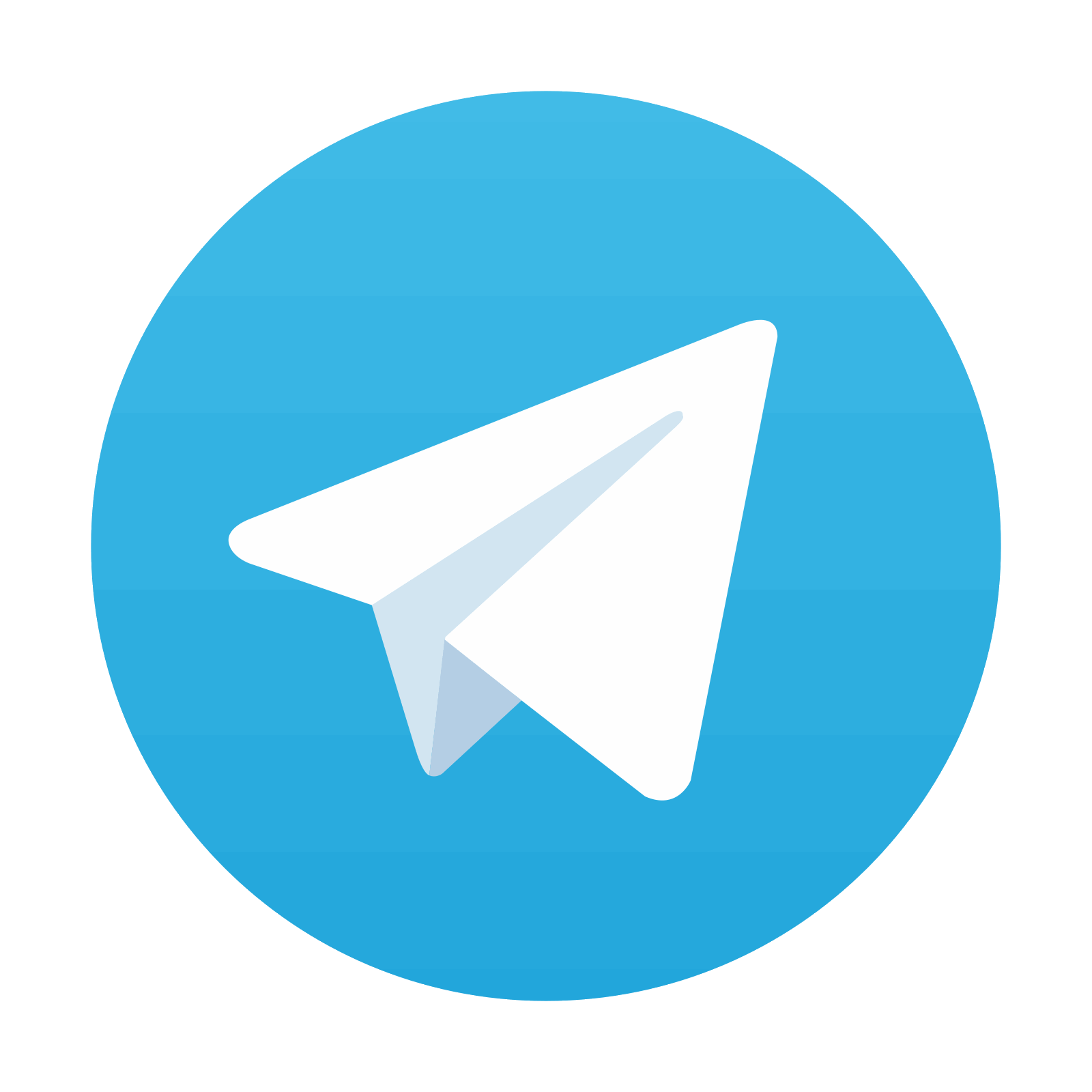
Stay updated, free articles. Join our Telegram channel

Full access? Get Clinical Tree
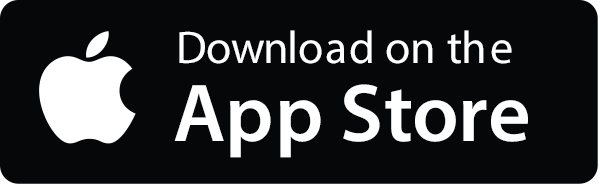
