Luminal A
Luminal B
Basal-like or TNCB
HER2/ErbB2
Normal-like
Claudin-low
Genetic signature
Luminal A (estrogen receptor positive and/or progesterone receptor positive, HER2 negative)
Luminal B (estrogen receptor positive and/or progesterone receptor positive, HER2 positive)
Basal-like (estrogen receptor negative, progesterone receptor negative, HER2 negative, cytokeratin 5/6/17 positive, and/or epidermal growth factor receptor positive)
HER2-like (estrogen receptor negative, progesterone receptor negative, HER2 positive)
Gene expression of adipose and other nonepithelial genes, high basal-like and low luminal gene
Unclassified (negative for all five markers)
HER2 –, ER+, Ck5/6, and EGFR +/−
HER2 +, ER+, Ck5/6, and EGFR +/−
HER2 –, ER –, Ck5/6, and/or EGFR +
HER2+, ER+/−, Ck5/6, and EGFR +/−
NA
ER –, HER2 –, Ck5/6 –, and/or EGFR –
Clinical outcome
Most favorable prognosis
Low response
Worst prognosis and high incidence of metastasis
Shortest relapse-free and overall survival
Intermediated prognosis
NA
Treatment response
Low response to chemotherapy previous to surgery
Low response to chemotherapy previous to surgery
High complete response to chemotherapy previous to surgery
High complete response to chemotherapy previous to surgery
Low response to chemotherapy previous to surgery
NA
Low response to tamoxifen and aromatase
Targeted therapies
One of the most aggressive phenotypes that present chemoresistance and metastasis is the triple-negative breast carcinomas (TNBC), characterized by the absence of ER, PR, and HER2 expression and with a poor prognosis. It is epidemiology relevant because of its prevalence in younger patients (<50 years) [6].
Omics technologies have contributed toward finding and establishing important molecular aspects of the pathogenesis involved in another key area: the BRCA1 connection, apoptosis resistance, proliferation, epithelial-mesenchymal transition, angiogenesis, and microRNA expression [5, 7].
It is important to highlight that host germline genetics may determine the tumor subtype, and this has been demonstrated because of the presence of BRCA1-associated breast cancers cluster within the basal-like subtype [2]. Moreover, the assessment of those women with inactivating germline mutations in BRCA1 or BRCA2 shows that they have up to an 85 % chance of developing breast cancer during their lifespan [8].
The translation from the bench to bedside of the clinical molecular findings gives power to gene expression signatures (GES) that can become specific and validated biomarkers used by the FDA and other international expert panels. These markers will shed light on prognostic responses to breast cancer treatments, for example, mutated HER1/HER2, BRCA1/BRCA2 genes, etc. Moreover, new, targeted therapies will have had their fundamental basis in the specific molecular profiles and pathways discovered [9].
However, the complex nonlinear relationship between the driver mutations and the phenotype of individual patients introduces difficulties in developing a next generation of biomarkers and therapeutic biological agents [10].
In spite of the great advances in overcoming these difficulties, in reality there is a lack of robust and validated biomarkers for predicting the responses to specific therapies. Moreover, the discovery of key targets for the development of effective drugs has not yet been achieved. For this reason, systematic studies of more than 25,000 cancer genomes at omics (genomic, epigenomic, transcriptomic, etc.) levels will reveal the catalogue of oncogenic mutations and enable the development of new cancer therapies. The aim is to link the global omics data with clinical data and establish algorithms for the classification of risk, prognosis, and therapy, as performed already by Glinsky [1], a stemness cancer therapy outcome predictor (CTOP). The approach in this new era of knowledge requires a multidisciplinary management of breast cancer patient healthcare.
Genetics in Breast Cancer
A short panoramic view of known genetics in breast cancer is necessary to understand the complexity of the theme developed in this chapter. Thus, familial breast cancer comprises approximately 20–30 % of all breast cancers. Currently, mendelian approaches or empirical data models can be used in clinical practice for risk prediction of breast cancer in order to facilitate genetic counseling.
Family linkage studies have identified the high-penetrance genes, BRCA1, BRCA2, PTEN, and TP53, that are responsible for inherited BC syndromes (Table 14.2). Moreover, a combination of family-based and population-based approaches has indicated that the genes involved in DNA repair, such as CHEK2, ATM, BRIP, and PALB2, are associated with moderate risk [11]. However, not all familial breast cancer can be linked to a single gene. Susceptibility alleles in other genes, such as PTEN, ATM, STK11/LKB1, and MSH2/MLH1, are also rare causes of inherited breast cancer. The majority of women with sporadic or nonhereditary breast cancer have common low-penetrance genetic risk variant genes such as FGFR2, TNRC9, MAP3K1, LSP1, 2q35, 5p12, and 8q24 of the eight variants discovered thus far and, together, account for 60 % of breast cancer in the general population of women of European ancestry [2].
Table 14.2
Familial genes in hereditary breast cancer
Gene | Percentage |
---|---|
BRCA1 | 20 |
BRCA2 | 20 |
TP53 | <1 |
CHEK2 | 5 |
Emerging genes: CASP8, MAP3K1, LSP1, TNRS9 | 5 |
PTEN, ATM, STK11/LKB1, BRIP1, PALB2, NBS1 | <1 |
Unknown candidate genes | 50 |
Molecular biology and gene expression signatures of breast cancer are critical for developing novel approaches to the prevention and healthcare management of this disease. Despite intensive work already developed, new molecular pathways should have to be identified to further contribute to the understanding of breast cancer genetics.
Omics Applications Toward Chemoresistance in Breast Cancer: “Chemoresistome”
Omics discoveries are transforming the traditional approaches to breast cancer management. However, to date, there are no validated sensitivity and/or resistance predictive factors available in clinical settings, and the mechanisms involved in cancer cell chemoresistance are still largely unknown. All the biomarkers included in this category will be referred to as “chemoresistome” for identifying present and future key molecular marker determinants of resistance to diverse therapies.
Up to now, multiple molecular pathways may contribute to the sensitivity/resistance of cancer cells to chemotherapy. Specifically, the mechanisms that may contribute to chemotherapeutic resistance in breast cancers could include decreased intracellular drug concentrations, mediated by drug transporter’s overexpression and metabolic enzymes; antiapoptotic factors and DNA repair; cell cycle deregulation or arrest by impaired cellular responses; the promotion of progression of cancer cell populations; perturbations in DNA methylation and histone modifications; and alterations in the availability of drug targets [12, 13]. Moreover, tumor heterogeneity may also contribute to resistance, where small subpopulations of cells may acquire or stochastically already possess some of the features enabling them to emerge under selective drug pressure.
The present chapter has identified the main omics approaches pursued by several authors that can explain key factors involved in the chemoresistance of breast cancer (Table 14.3). In this context, the most important aim will be to validate those biomarkers for use at a clinical level.
Table 14.3
“Chemoresistome and metastasisome in breast cancer”: omics methodologies for chemoresistance and metastasis
Omics methodology applications | Key short definitions | Techniques | Biomarkers type | Databases | References | References |
---|---|---|---|---|---|---|
Chemoresistome | Metastasisome | |||||
Genomics Genetic variability | Interindividual variability gene profiles | Affymetrix/Luminex PCR-RFLP | SNP, CNV | ICGC HAPMAP SNP | Chen et al. [4] Frati et al. [14] Kim and Paik [15] | Thomassen et al. [44] Dawson et al. [45] |
Genomics Epigenenetics | Methylation pattern independent of primary DNA sequence | Immunoblot for histone hyperacetylation ChIP-Seq (specific epigenetic modifications | SCFA, HATs, HDACi | Databases and Tools EPIGENIE_Epigenetics | Kutanzi et al. [12] Fang et al. [26] | Rodenhiser et al. [49] Samantarrai et al. [50] Bentires-Alj et al. [81] |
Genomics Pharmacogenomics | Interindividual variability gene profiles in drug therapy | Affymetrix/Luminex TaqMan real PCR, KASPAR PCR-RFLP | Drug transporters Drug targets Pathways | Cypalleles Pharmagkb | Cascorbi and Haenisch [19] Cascorbi [20] | Hill et al. [48] |
Transcriptomics | Gene expression profile | RNA microarrays; RT-PCR; Qpcr | Transcripts of RNA cDNA | H-DBAS – H-invitational database | Gröger et al. [29] MacCartan et al. [30] Flamant et al. [31] | Saunus et al. [42] Lorusso and Ruegg [56] |
Proteomics | Determinations of protein differentially expressed | 2-DE analysis, multidimensional, chromatography, LC-MS/MS, MALDI-TOF, SELDI-TOF | Proteins, peptides, oligopeptides | UniProt Proteomics databases | EBI | Chuthapisith et al. [33] Hortobagyi et al. [32] | Terp et al. [58] Niu et al. [59] Qin and Ling [82] |
Metabolomics | Quantitative measurement of multiparametric metabolic responses | NRM analysis, MS, GC, HPLC CE/MS, FTIR analysis | Metabotype: lipids, sugars, vitamins, antioxidants | The Human Metabolome Database (HMDB) | Martinez-Outschoorn et al. [35] | Martinez-Outschoorn et al. [35] Tenori et al. [64] Oakman et al. [62] |
Nutrigenomics | Correlated data between genomics and diets collections | Programmed diets/sequencing | Metabolic enzymes; key diet parameters | European Nutrigenomics Organization | Saukko et al. [36] Riscuta and Dumitrescu [37] Corella and Ordovás [38] | Ozdemir et al. [65] Nieman et al. [67] Taniya et al. [83] |
Lipidomics | Lipid global levels in clinical measurements | GC, HPLC | Lipid profile | Lipid database | Xue and Wong [39] Antoon et al. [40] Aluise et al. [41] | Nieva et al. [69] |
Genomics in Breast Cancer Chemoresistance
While the genomic predictors are of current interest, the evolution of patients’ characteristics and treatments requires a permanent optimization. Several studies have attempted to report these genomic predictors. Frati et al. [14] established a genetic profile involved in the chemoresistance and extrapolated the drug sensitivity to breast cancer. ER+/HER−/low gene expression proliferation tumors are categorized as chemoresistant, whereas tumors with an ER−, HER2+, or ER+/HER2-/high proliferation gene expression profile can be categorized as chemosensitive tumors. Moreover, there is scientific evidence of chemoresistance in patients with ER+/HER2−/low proliferation tumors when treated with endocrine therapy, and evidence exists among oncologists to allow clinical use of gene expression tests to identify patients who do not require chemotherapy among node-negative ER+ patients [15]. A genomic predictor combining ER status, predicted chemoresistance, predicted chemosensitivity, and predicted endocrine sensitivity, identified patients with a high probability of survival following taxane and anthracycline chemotherapy.
Epigenetics in Breast Cancer Chemoresistance
Epigenetics and RNA-related markers are promising for the explanation of dysregulation cell pathways involved in cancer. Indeed, miRNA profiling has been convincingly demonstrated to classify tumor and non-tumor samples, sensitivity/resistance to drugs therapies, etc. Thus, evidence of miRNA-mediated reversal of multidrug resistance in human cancer [16] warrants further studies of miRNA-based approaches for treating drug-resistant tumors. Some specific results indicated that miRNA-125b played an important role in chemotherapeutic resistance in breast cancer cells, specifically in primary breast cancer cells. Experiments were carried out on breast cellular models showing that the expression of miR-125b decreased 5-FU-induced cytotoxicity and increased 5-FU resistance under various concentrations of 5-FU treatment in MCF-7 cells [17].
Pharmacogenomics in Breast Cancer Chemoresistance
It is well known that treatment efficacy is optimal for one certain genotype group, while a similar drug is most efficacious for another genotype group, so that therapy can be personalized to achieve maximum success in patient care. If a polymorphism is identified to be associated with drug dosing, physicians can change clinical care using genotype in the dosing algorithm. Likewise, if a polymorphism is associated with a serious adverse event, an alternative treatment could be selected for such patients [18].
The aim of pharmacogenetics and the main targeted drug therapy of breast cancer are to determine whether there is a correlation between genetic polymorphism, such as in targeted molecular structures, and response to treatment or the development of drug-associated toxicity [19, 20].
Table 14.4 compiles the genes that have shown significant variability according to each drug that can be prescribed in breast cancer. These data have been obtained through www.pharmagkb.com database, which stratify the validated SNP according to clinical impact and outcome, FDA mandatory label, and other parameters. The majority of the genes are related to transporters, CYP450 alleles, and specific targeted therapies.
Table 14.4
Drugs therapy for breast cancer and target genetic variability
Drugs | Chemical structure | Gene (PHARMAGKB) |
---|---|---|
Anthracyclines | Variable general structure | ABCB1 |
ABCB4 | ||
SLC28A1 | ||
SLC22A2 | ||
ABCC1 | ||
ABCB4 | ||
SLC28A3 | ||
SLC10A2 | ||
Doxorubicin | – | ABCB1 |
FDA drug label at DailyMed: | ABCC2 | |
102358 dc-2109-4829-b333-b99417334e39 | ABCC4 | |
CBR3 | ||
Antiestrogens | – | CYP2D6 |
Tamoxifen | CYP3A | |
FDA drug label at DailyMed: | ABCC2 | |
7ee3d3d2-85d1-4018-8e70-5ed8a64ae1f0 | SULT1A1 | |
Antiestrogens | – | ESR1 |
Fulvestrant | ESR2 | |
FDA drug label at DailyMed: | ||
83d7a440-e904-4e36-afb5-cb02b1c919f7 | ||
Aromatase inhibitors | – | CYP19A1 |
Letrozole | GLDN | |
Anastrozole | CYP2A6 | |
FDA drug label at DailyMed: | ||
11628cdc-4e3c-4063-ee9f-c51e2386a820 | ||
Docetaxel | – | ABCB1 |
FDA Drug Label at DailyMed: | SLCO1B3 | |
82731db6-92 fc-483b-9d73-9b2aed79b104 | ATP7A | |
ABCC6 | ||
SLC10A2 | ||
CYP1A1 | ||
GSTP1 | ||
Paclitaxel | – | ABCB1 |
FDA drug label at DailyMed: | ||
88d7cdd2-e650-4a16-adec-873927e03e93 | ||
Platin derivatives | – | ABCB1 |
Cisplatin | ABCC2 | |
Therapeutic targets database: | ABCC4 | |
DAP000215 | SLC31A1 | |
SLC19A1 | ||
SLC22A2 | ||
Monoclonal antibodies | Herceptin | ERBB2 |
FDA drug label at DailyMed: | Anti-HER2 | FCGR2A |
492dbdb2-077e-4064-bff3-372d6af0a7a2 | Ig gamma-1 chain C region | FCGR3A |
Clinical trials | Lexatumumab | |
Monoclonal antibodies | Anti TRAIL-R1 | TRAIL-R1 |
Mapatumumab | ||
Anti TRAIL-R2 | TRAIL-R2 | |
Clinical trials | Inhibitors pathway | |
New combined therapies | Hedgehog | SNAIL, GLI1 |
Notch | Notch receptors | |
WTN | NA | |
IPARP | BRCA1/BRCA2 | |
Olaparib |
In the context of chemoresistance, among others, special attention should be paid to drug transporters, including ATP-binding cassette (ABC) transporters and solute carrier (SLC) transporters, which are also expressed at cancer cells.
The ABC transporters are a family of large proteins in membranes and are able to transport a variety of compounds including metabolites and drugs through membranes at the cost of ATP hydrolysis. Physiological functions of ABC transporters include the transport of lipids, bile salts, toxic compounds, and peptides for antigen presentation or other purposes, such as ion channel regulation. The human genome contains 48 ABC transporter genes; at least 14 of these are reportedly associated with heritable human diseases [21].
Commonly, polymorphisms in P-glycoprotein or ABCB1, ABCG2 have been widely described as affecting treatments of different diseases [22]. Moreover, the potential impact of ABCC11 genetic polymorphisms on the physiological function, breast cancer risk, and patients’ response to nucleoside-based chemotherapy has been described [13].
An important discovery has been the breast cancer resistance protein (BCRP), whose expression in the liver is needed for in vitro to in vivo extrapolation of the biliary clearance of a BCRP substrate drug. A polymorphic variant C421A (rs2231142) allele was significantly lower in breast cancer patients than that in the wild-type livers [22]. Currently, there are no arguments to determine the pharmacogenetic status of HER2 to individualize trastuzumab treatment [9]. The searching of biomarkers of trastuzumab resistance are focused on several mechanisms—i.e., deficiency of phosphatase and tensin homologue and activation of phosphoinositide 3-kinase result in greater activity of the Akt-mammalian target of rapamycin signal transduction pathway [23]. Also, the overexpression of other surface receptors, such as insulinlike growth factor, provides alternative growth factor signaling and is related to lower trastuzumab sensitivity [24].
Genome-Wide Association Studies in Breast Cancer
Genome-wide associations (GWAS) based on large prospective randomized trials have the potential to greatly elucidate the genomic basis for optimizing drug efficacy and reducing toxicity. The studies on breast cancer have been mainly focused on how both disease susceptibility loci and risk prediction may lead to a better understanding of the biological mechanism for BC in order to improve prevention, early detection, and treatment. They are of clinical importance and may explain an appreciable proportion of the genetic variance in BC risk. There are few studies that specifically describe results affecting chemoresistance [25] and metastasis [26]. The power of GWAS may be increased by enlarging the number of samples in both the cases and the controls and by identifying clinical and molecular subtypes [27]. There is a long way to go toward improving the controversial results of different GWAs, and thus a novel multi-SNP GWAS analysis method called pathways of distinction analysis was developed. This method includes GWAS data and pathway-gene and gene-SNP associations to identify pathways that could permit the distinction of cases from controls [28]. Thus, new GWAS in breast cancer generation of large-scale association studies, in combination with replication analyses and multiple scans, could have the potential to identify many more loci.
In order to further exploit the transcriptome and genome data, it is usual to carry out an integrated analysis of transcriptome, proteomic, and metabolomic data, observing how this corresponds to the varying physiological and phenotype status.
Transcriptomics in Breast Cancer Chemoresistance
One of the main aspects studied by transcriptomic studies is the epithelial to mesenchymal transition (EMT) that represents a crucial event during cancer progression and dissemination. EMT is the conversion of carcinoma cells from an epithelial to a mesenchymal phenotype that is associated with a higher cell motility as well as enhanced chemoresistance and cancer stemness [29]. This issue will be developed further in the discussion below on metastasis of breast cancer. Moreover, some specific markers have been determined to impact the chemoresistance on breast cancer patients treated with endocrine therapy. The steroid coactivator protein SRC-1 drives tumor adaptability through ADAM22, a non-protease member of the ADAM family of disintegrins [30]. Taxol and etoposide resistance have been demonstrated to be mediated by TMEM45A [31].
Proteomics in Breast Cancer Chemoresistance
Based on gene expression profiles, or proteomics of three or four validated biomarkers, it is apparent that there are multiple subtypes with different clinical characteristics, clinical courses, and sensitivities to existing therapies (ER, PR, HER2, and Ki-67 represent critical molecular markers that identify the largest molecular subsets and therapeutic decisions [32]). Current sensitivity levels may allow whole-cell proteomics approaches and subcellular fractions to now be sequenced at the protein level with success [33, 34].
Metabolomics in Breast Cancer Chemoresistance
Important molecules to be determined for classification are the metabolic enzymes and metabolism mediators that affect individually the impact of drug therapy [12, 18]. In particular, some authors have found important metabolism enzymes required for ketone body production that are highly upregulated within cancer-associated fibroblasts. L-Lactate and ketone body metabolisms are critical for tumor progression and metastasis [35].
Nutrigenomics in Breast Cancer Chemoresistance
There are nutrigenetic testing companies, which provide DNA-based nutritional advice analyzing the individual genome of patients or healthy people. The validity of biomarkers is not accurate in all cases and requires expert counseling [36]. Some authors have studied the impact of single nucleotide polymorphism, copy number, epigenetic events, and transcriptomic homeostasis as factors influencing the response to food components and breast cancer risk, among them the dietary n-3 fatty acids [37, 38]. There are also foods that can induce or inhibit the action of CYP450 enzymes involved in metabolism of drugs.
Lipidomics in Breast Cancer Chemoresistance
Lipid pathway analysis is acquiring importance in many clinical areas, as diet-controlled components, as metabolic mediators, and even as therapeutic molecules for breast cancer chemoresistance [39, 40]. Moreover, it is known that oxidation of membrane phospholipids is associated with cancer. Oxyradical damage to phospholipids results in the production of reactive aldehydes that adduct proteins and modulate their carcinogenic function [41].
Omics Application Toward Breast Cancer Metastasis: “Metastasisome”
“Metastasisome” is the term used to denote all of the important molecular omics developments investigated by several authors with respect to breast cancer metastasis (Table 14.3). Metastasis is defined as the spread of cancer cells from a primary site resulting in the establishment of secondary tumors in distant locations [42, 43]. These steps for metastasis include escape from the primary tumor (intravasation), dissemination via the blood or lymphatic system, survival within the circulation, arrest and extravasation into a secondary site, initiation of growth into micrometastases, and maintenance of growth as vascularized, clinically detectable macrometastases.
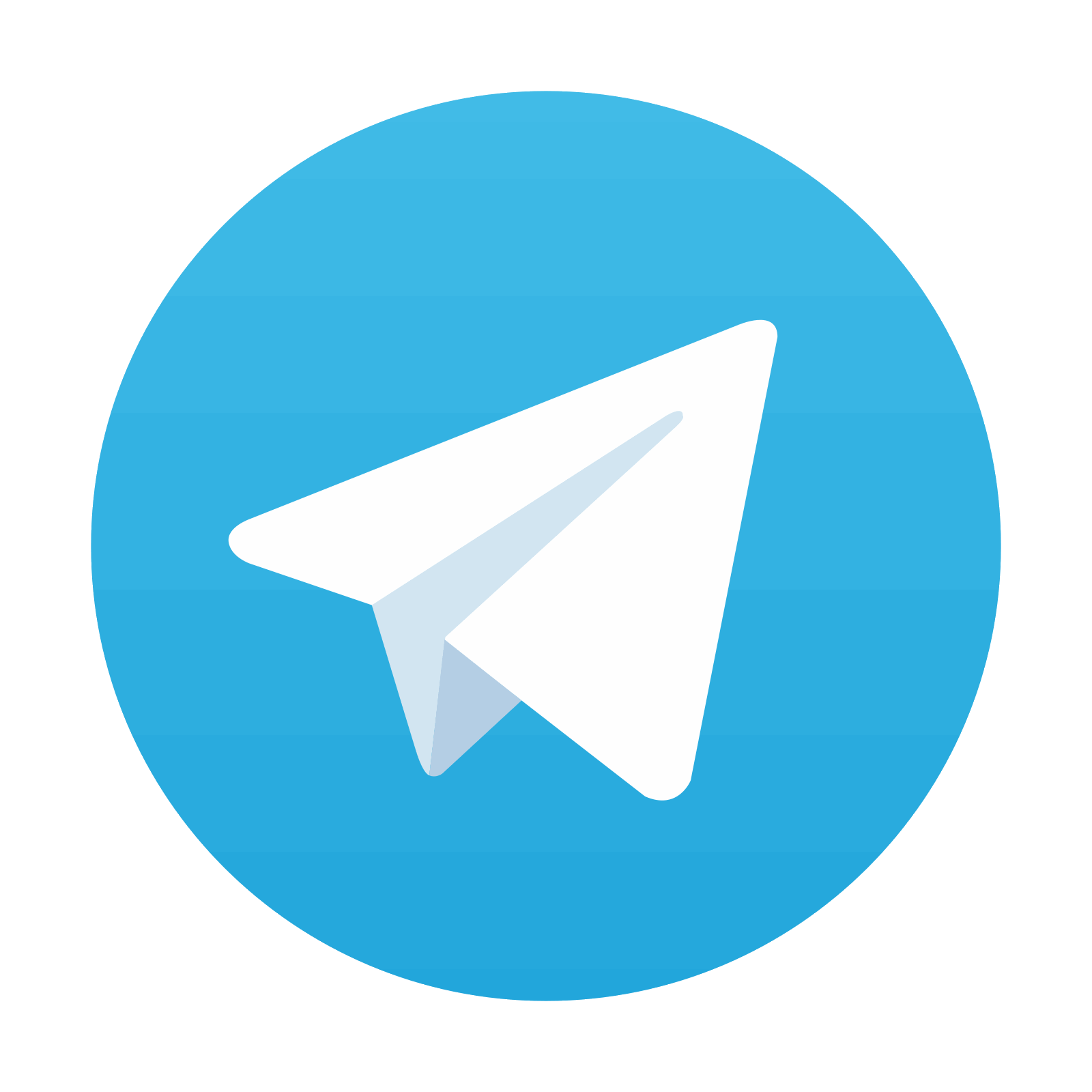
Stay updated, free articles. Join our Telegram channel

Full access? Get Clinical Tree
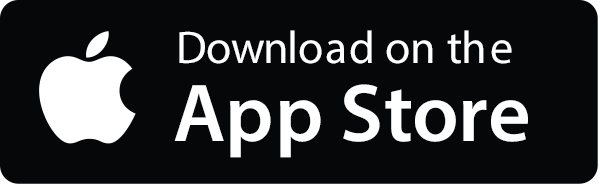
