Fig. 1
Karyotype aberrations. Doubling the karyotype results in polyploidy, whereas loss or gain of one entire chromosome results in whole chromosome aneuploidy. Unbalanced structural rearrangements such as gain or loss of regions as large as chromosome arms and unbalanced translocations can cause structural aneuploidy. Chromotripsis, an event of complex genome rearrangements, is often accompanied by amplifications and deletions and therefore results in structural aneuploidy as well.
Whereas copy number variations and possibly also segmental aneuploidy arise due to incorrect DNA replication and repair, whole chromosomal aneuploidy results from errors in chromosome segregation (reviewed in Gordon et al. 2012; Holland and Cleveland 2012). The propagation of aneuploid cells that have missegregated chromosomes is limited as they often arrest in the G1 phase directly following the erroneous mitosis (Thompson and Compton 2010; Li et al. 2010; Kuffer et al. 2013; Kumari et al. 2014). Even if aneuploid cells overcome this burden and continue to divide, their proliferation is often markedly impaired and they may suffer from additional detrimental changes (Torres et al. 2007; Williams et al. 2008; Stingele et al. 2012). The altered physiology of aneuploid cells is likely due to the large-scale changes in gene copy number and their expression. In humans, aneuploidy is associated with pathologies such as trisomy syndromes and cancer. Intriguingly, the effects of aneuploidy might become advantageous in adverse or rapidly changing environments (Pavelka et al. 2010; Lee et al. 2011). The molecular mechanisms underlying the cellular consequences of chromosome missegregation both acute, i.e., in daughter cells immediately after chromosome segregation errors, and chronic, i.e., during subsequent proliferation of aneuploid cells, remain incompletely understood.
The chronic consequences of aneuploidy per se can only rarely be analyzed, because in many cases aneuploidy is accompanied by chromosomal instability (CIN), an ongoing elevated frequency in mitotic errors that leads to additional chromosome gains or losses in a significant proportion of cell divisions. Cancer cells with CIN missegregate a chromosome about once every one to five divisions, compared to rates of one chromosome per a hundred cell divisions in stable, diploid cell lines (Cimini et al. 1999; Thompson and Compton 2008). Being accompanied by other types of instabilities, whole chromosome CIN manifests in the complexity of karyotypes in cancer and high inter- and intratumor genomic heterogeneity (Burrell et al. 2013). Additionally, cancer cells display multiple genomic alterations, such as point mutations, and small rearrangements, such as insertions, deletions, duplications, inversions, amplifications and translocations. The ongoing CIN in cancer cells means that both acute and chronic effects of aneuploidy act in tumor cell lines simultaneously. Thus, studies of aneuploidy in these cells resemble studies of a continually changing creature, a Proteus of sorts, who defies to be captured in its true nature.
Deciphering the consequences of aneuploidy has been recently advanced by analysis of cells from embryos and patients with trisomy syndromes as well as by novel aneuploid model systems (Fig. 2). To complete the picture, models carrying mutations that interfere with chromosome segregation and thereby induce the CIN phenotype were established (e.g. Sotillo et al. 2007; Weaver and Cleveland 2007; Baker et al. 2009 for a review see Ricke and van Deursen 2013). With the advent of microarray technology and mass spectrometry, transcriptome and proteome studies in both aneuploid model cell lines and cancers gave new insights into the response to aneuploidy. In our review we will focus on the consequences of aneuploidy in cancer and compare them with the recently characterized effects of aneuploidy in model systems. In the light of these findings we will discuss how these expression changes are linked to the physiological effects of aneuploidy.
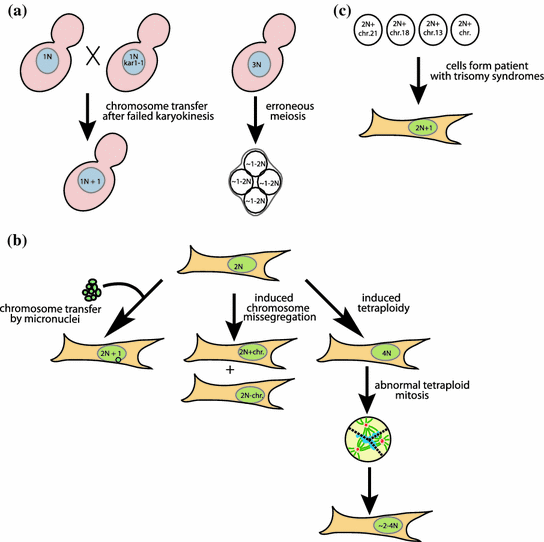
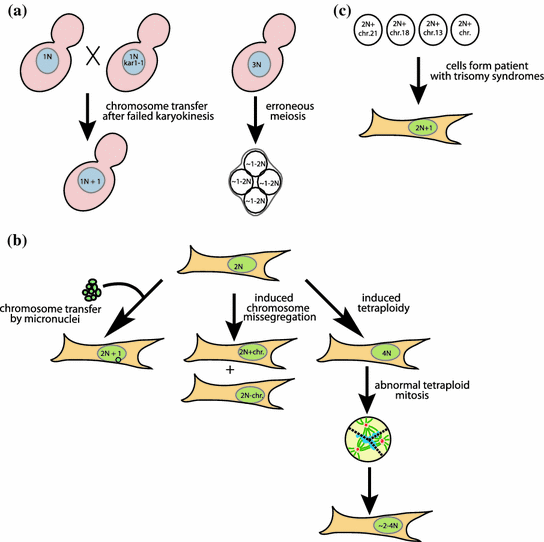
Fig. 2
Aneuploid model cell lines. a Yeast aneuploid model cell lines arise via chromosome transfer after failed nuclear fusion or from erroneous meiosis result in aneuploidy. b Mammalian aneuploid model cell lines of defined aneuploidy are generated by micronuclei-mediated chromosome transfer. Random aneuploidies arise from induced chromosome missegregation or via abnormal mitosis in tetraploid cells. c Cells derived from embryos or patients with trisomy syndromes present a source for cell lines with defined aneuploidy
2 Consequences of Aneuploidy on Transcriptome and Proteome
The fundamental characteristics of aneuploid phenotypes are determined by the impact of altered gene copy numbers on the transcriptome and proteome. In order to detect expression changes attributable directly to aneuploidy, multiple studies compared model cell lines with a defined aneuploidy to the parental diploid cell line. Yet, it should be noted that the quantitative analysis of transcriptome and proteome changes in aneuploid cells often suffers from severe technical difficulties. Part of the problem is that the quantitative change that needs to be determined is small, as the presence of one extra copy results in a 50 % expression increase. Thus, normalization strategies, used thresholding cut offs and statistical analysis may strongly affect the interpretation of the studies. The analysis of tumors is further complicated by the fact that they consist of cancer cells with heterogeneous karyotypes intermixed with a fraction of normal diploid cells (Mitelman 2013; Stevens et al. 2014). Thus, the transcriptome analysis determines the average gene expression changes affected by both the effects of gene copy number and the tumor heterogeneity. Despite these limitations, the global gene expression profiling markedly progressed our understanding of the cellular consequences of aneuploidy.
2.1 Transcriptome and Proteome Response to Model Aneuploidy
The majority of genes encoded on the supernumerary chromosomes are differentially expressed according to the gene copy numbers in most model aneuploid cells, such as in yeast (Torres et al. 2007; Chikashige et al. 2007), murine (Williams et al. 2008) and human cell lines (Stingele et al. 2012; Upender et al. 2004; Nawata et al. 2011), Arabidopsis thaliana (Huettel et al. 2008; Sheltzer et al. 2012) and maize (Birchler 2013). The gene expression largely scales with the copy number changes also in trisomies from patients’ samples and mouse models: trisomy of chromosome 21 resulted in correlative expression change of the genes in both human and mouse models (Ait Yahya-Graison et al. 2007; Chou et al. 2008; Wang et al. 2011; Vilardell et al. 2011). Markedly, gene dosage compensation was found for 25 % to 50 % of the genes on the trisomic chromosome (Ait Yahya-Graison et al. 2007; Chou et al. 2008; Wang et al. 2011; Vilardell et al. 2011). In Drosophila the transcripts originating from aneuploid chromosomes are buffered towards the diploid levels (Stenberg et al. 2009; Zhang et al. 2010; Lundberg et al. 2012). This reflects the specificity of gene dosage compensation mechanisms in Drosophila rather than a general feature of aneuploidy (reviewed in Birchler 2013; Stenberg and Larsson 2011; Donnelly and Storchova 2014).
Global protein expression scales with the gene copy number changes as well, as the expression from the extra chromosome is increased by 1.6–1.9-fold in yeast and mammalian cells (Stingele et al. 2012; Pavelka et al. 2010; Torres et al. 2010). However, initial small-scale analysis in disomic yeast strains determined that 13 out of 16 proteins were not differentially expressed as expected from the gene copy numbers (Torres et al. 2007). Genome-wide comparison revealed that around 20 % of the proteins encoded on supernumerary chromosomes did not show an enhanced expression in yeast as well as in human cell lines (Stingele et al. 2012; Torres et al. 2010). Intriguingly, the proteins whose abundance was compensated to euploid level were enriched for subunits of macromolecular protein complexes (Torres et al. 2007, 2010; Stingele et al. 2012). In concordance, global quantitative analysis demonstrated a significant reduction in the expression of protein complex core subunits encoded on the aneuploid chromosomes in 3 out of 5 yeast strains with a complex aneuploid karyotype (Pavelka et al. 2010). Moreover, other protein classes such as protein kinases and transcription factors are subjected to dosage compensation to restore the stoichiometric balance at least in human cell lines (Stingele et al. 2012 and our unpublished results). The mechanisms enabling the dosage compensation are not known, but it has been suggested that they may contribute to restoring the protein stoichiometry (Torres et al. 2007; Donnelly and Storchova 2014). In summary, gene and protein expression in model aneuploid cell lines generally scales according to the gene copy number, but specific protein classes are compensated towards normal diploid expression levels by an unknown mechanism.
2.1.1 Global Transcriptional Response to Aneuploidy
Aneuploidy affects the expression not only of the genes located on the supernumerary chromosomes, but also the expression of multiple other genes across the entire genome (Torres et al. 2007; Stingele et al. 2012; Upender et al. 2004; Sheltzer et al. 2012; Gemoll et al. 2014). This global gene deregulation can originate from at least two sources. First, transcriptional regulators that are located on the aneuploid chromosome regions and therefore present in altered copy numbers can affect the expression of genes on the other chromosomes. In this case, the response to aneuploidy should be largely dependent on the specific extra chromosome. Intriguingly, a uniform transcriptional response was identified in all aneuploidy model cell lines (Sheltzer et al. 2012; Dürrbaum et al. 2014). This rather suggests a second model, where the expression changes throughout the whole genome are driven by aneuploidy per se and the resulting protein imbalance triggers a specific cellular response that feeds back to the transcriptional regulation. The gene expression pattern in aneuploid yeast strains of different origins resembles the transcriptional pattern of a previously described yeast environmental stress response—ESR (Torres et al. 2007; Sheltzer et al. 2012). In particular, the enriched gene ontology terms for differentially expressed genes suggested aneuploidy-driven alterations in RNA processing and energy metabolism. Similarly, aneuploid mammalian cells of different origins show a uniform aneuploid pathway response pattern (Williams et al. 2008; Stingele et al. 2012; Sheltzer et al. 2012; Dürrbaum et al. 2014; Foijer et al. 2013). The aneuploidy response pattern is similar also in cultured amniocytes from trisomic pregnancies (Sheltzer et al. 2012). In detail, gene ontology terms such as endoplasmic reticulum (ER), Golgi apparatus, lysosomes and vacuoles and membrane metabolism were consistently upregulated, whereas DNA and RNA metabolic pathways—e.g., DNA replication, repair, transcription and RNA splicing—were downregulated on the transcriptional level (Dürrbaum et al. 2014). These deregulated pathways were confirmed by proteome analysis of six different human aneuploid cell lines (Stingele et al. 2012).
What triggers these conserved changes? Recently, it was shown that aneuploidy affects molecular pathways linked to the maintenance of protein homeostasis (Torres et al. 2007; Stingele et al. 2012; Tang et al. 2011; Oromendia and Amon 2014). That is, gain of an extra chromosome may disturb cellular proteostasis by flooding the cellular system with proteins and exhausting the resources for their synthesis, thus causing proteotoxic stress (Donnelly and Storchova 2014; Oromendia and Amon 2014). As a consequence, protein degradation pathways might be activated to eliminate overexpressed proteins and accumulated misfolded proteins. Indeed, aneuploidy activates autophagy and upregulates annotations associated with lysosome, vacuoles and membrane metabolism (Dürrbaum et al. 2014; Tang et al. 2011; Stingele et al. 2013). In addition, the upregulation of ER and Golgi might be also attributed to autophagy, as these organelles serve as a membrane source for autophagosomes (Lamb et al. 2013). In concordance with the hypothesis that the transcriptional response reflects the cellular changes upon proteotoxic stress, autophagy inhibition in near- diploid HCT116 cells resulted in transcriptional changes similar to the aneuploid transcriptional response pattern (Dürrbaum et al. 2014) and aneuploid cells are sensitive to drugs triggering proteotoxic stress (Torres et al. 2007; Oromendia and Amon 2014). Taken together, the current data suggest that the aneuploid transcriptional response pattern arises as a direct consequence of proteotoxic stress due to karyotypic imbalance.
Another possibility is that the transcriptional pattern of aneuploid cells reflects their slower proliferation. Impaired proliferation and delayed progress through the G1 and S phases was observed in disomic yeasts as well as in trisomic and tetrasomic mammalian cell lines (Torres et al. 2007; Williams et al. 2008; Stingele et al. 2012). Two budding yeast strains carrying mutations in cell cycle regulatory factors that delay cell cycle progression show similar transcriptional changes as observed in response to aneuploidy (Sheltzer et al. 2012). Further, the differential transcription was changed when disomic and euploid yeast were grown at the same growth rate in the chemostat (Torres et al. 2007). However, slow proliferation cannot fully explain the aneuploidy response pattern, as complex human aneuploid cell lines exhibit the same transcriptional changes, yet their growth rate is similar to diploid cell lines (Dürrbaum et al. 2014). Moreover, similar expression changes were also identified in chromosomally unstable cancer cell lines of the NCI-60 panel that generally proliferate well (Roschke et al. 2008; Sheltzer 2013). This finding suggests that either both, CIN and aneuploidy, trigger a similar transcriptional response or that the aneuploidy response pattern is in fact a cellular response to CIN, as aneuploidy can promote CIN (Sheltzer et al. 2011, our unpublished data). Interestingly, despite the striking similarities among the aneuploid pathway response, there is no significant overlap of the individual deregulated genes between different aneuploidies (Williams et al. 2008; Gemoll et al. 2014; Dürrbaum et al. 2014). This indicates that there are common physiological changes in response to aneuploidy, but the specific molecular determinants are diverse, likely as a consequence of heterogeneous karyotypes (Dürrbaum et al. 2014). Taken together, aneuploidy triggers activation and inhibition of certain pathways and these global changes likely influence all other physiological consequences of aneuploidy.
2.2 Cancer Signatures and Transcriptome Dynamics in Aneuploid Cancers
A longstanding interest of cancer research is to characterize the transcriptome and proteome changes of cancer tissues. This is a necessary step for classifying tumor subtypes and for identifying molecular contributors to tumor progression and biomarkers of tumor aggressiveness or treatment strategies (Sara et al. 2010; Eddy et al. 2010; Chibon 2013). The gene expression changes are often seen as a result of large-scale epigenetic remodeling or gene mutations. Recent studies of aneuploid model cell lines emphasized that global gene expression is also altered by aneuploidy. The relative contribution of aneuploidy to the cancer transcriptome remains a subject of ongoing investigations.
2.2.1 Correlation of mRNA and DNA Copy Number Changes in Aneuploid Cancers
In general, gene expression in regions with copy number variations as a result of aneuploidy is correspondingly altered in all types of cancer tissues. However, the estimations of the correlation between gene copy numbers and gene expression is strongly influenced by the methods used to integrate the DNA copy numbers with the gene expression data. In brief, there are three main approaches: (1) simple comparison of the fold changes and calculation of the percentages of genes with high/low copy number that is co-directional with mRNA expression changes; (2) gene-by-gene correlation of gene copy number with gene expression; and (3) correlation of the average gene expression with the averaged copy number across a chromosome arm or segment (Fig. 3; Table 1). Using the first approach the mRNA expression derived from trisomic chromosomes or more than twofold amplified regions is increased within a range from 1.14 to 3-fold compared to the normal diploid case (Schoch et al. 2005; Xu et al. 2010). This broad range already indicates that the results of a fold change comparison will vary according to the cutoffs applied. When no cutoffs were applied, 62.5–87 % of the genes on trisomic chromosomes in acute myeloid carcinoma showed increased expression (Schoch et al. 2005). Similar percentages were reported in breast cancer, when twofold amplified regions were considered (Pollack et al. 2002) and in colon cancer, when genes with the highest 20 % of expression were analyzed (Tsafrir et al. 2006). If considering only more than 2.5-fold amplified regions, 44 % of genes are correspondingly expressed in colon cancer (Hyman et al. 2002). In contrast, several studies report exceptions of amplified regions that do not show correlative changes in gene expression (Tsafrir et al. 2006; Habermann et al. 2007; Taylor et al. 2010). For example, the loss of 8p is common in cancer, but a study in prostate cancer did not detect a correlative decrease in gene expression for all genes in the corresponding region (Taylor et al. 2010). A silencing of chromosomal amplifications in colon cancer has been also reported, but the results are based on a very stringent analysis; here, only 3.8 % of genes located in twofold amplified regions showed a twofold change in mRNA expression (Platzer et al. 2002).
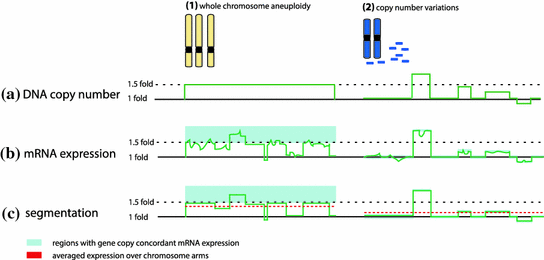
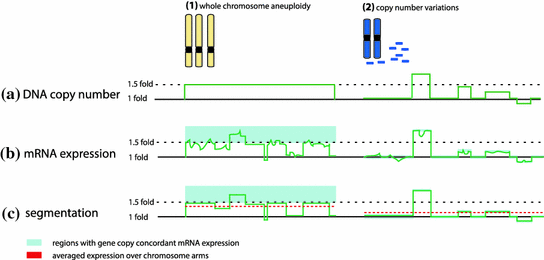
Fig. 3
Consequences of aneuploidy. Aneuploidy can arise from incidental chromosome missegregation or ongoing chromosomal instability. The acute response to chromosome missegregation, such as proteotoxic stress, growth defects, energetic stress and DNA damage, can activate cell cycle arrest or cell death. In a chronic response the aneuploid cells can adapt to the cellular stresses. Ongoing genomic instability generates genetic diversity and increases the adaptive potential. Cells adapt to aneuploidy by overcoming the adverse effects on i.e. proliferation due to growth-promoting mutations or by mutating the p53 signaling pathway
Table 1
Selection of recent results on correlation of gene copy number and gene expression in aneuploid cancers
Cancer tissue | Method | Results | Remarks | Publication |
---|---|---|---|---|
Comparison of fold changes | ||||
Oral squamous cell carcinoma | Genes were divided into subgroups according to their level of copy number change and compared to the average gene expression fold change of the respective groups | Dosage-response relation between copy number changes and mRNA expression | 20 samples | Xu et al. (2010) |
Colorectal carcinomas | Upper/Lower 20 % of global mRNA and CGH levels were used as cut offs for comparison of expression levels and corresponding copy number changes | 63 % of genes overexpressed showed also amplification; 62 % of downregulated genes showed also lower CGH (in lowest 20 %) | 114 samples | Tsafrir et al. (2006) |
Ovarian carcinomas | Analysis of 93 genes on chromosome 22 | Correlation of gene copy number and expression changes | 18 samples | Benetkiewicz et al. (2005) |
Acute myeloid leukemia | Average expression on aneuploid/trisomic chromosome was calculated | 1.14–1.27-fold increase in gene expression and 62.5–87 % of genes expressed higher than in diploid | 7–12 samples per trisomy, 104 controls | Schoch et al. (2005) |
Colon cancer liver/hepatic metastases | Twofold cut off for amplified regions on 7p, 8q, 13q, and 20q | 3.8 % genes in the amplified regions with ≥2 fold change in mRNA expression; 90 % of genes showed a 0.5–2-fold change | 23 samples, only 7p, 8q, 13q, and 20q | Platzer et al. (2002) |
Breast cancer cell lines | 2.5-fold cut off for amplified regions; overexpression defined as top 7 % of global expression levels | 44 % genes in amplified regions showed overexpression; 10.5 % of overexpressed genes showed >2.5-fold copy number amplification | 14 cell lines | Hyman et al. (2002) |
Primary breast cancer | ≥4-fold change cut off for highly amplified genes | 62, 42 % of high amplified genes showed >2-fold, >4-fold increase in mRNA expression, respectively | 44 samples | Pollack et al. (2002) |
Gene-by-gene correlation of copy number and mRNA expression | ||||
Oral squamous cell carcinoma | Gene-by-gene correlation of mRNA expression and copy number | Normal distribution of correlations with a peak shifted towards positive correlations. Significant correlation of 30 % of transcripts | 20 samples | Xu et al. (2010) |
Primary breast cancer, breast, pancreatic, prostate, lung cancer cell lines | Median of gene-wise correlation of mRNA expression and gene copy number | Copy number variations explain 12–40 % of expression changes | Gu et al. (2008) | |
Colorectal carcinoma | Gene-by-gene correlation of mRNA expression and gene copy number | Correlation value: 0.52 colon carcinoma; 0.56 rectal adenocarcinoma | 32 colon carcinomas, 17 rectal adenocarcinoma | |
Primary breast cancer | Gene-by-gene correlation of mRNA expression and gene copy number | 10 % of genome showed significant correlations | 145 samples | Chin et al. (2006) |
Colorectal carcinomas | Correlation of average mRNA and gene copy number expression over all samples for each probe | mRNA and CGH correlation r = 0.69 | 114 samples | Tsafrir et al. (2006) |
Primary breast cancer | Gene-by-gene correlation of mRNA expression and gene copy number | Distribution of correlations showed normal- shaped curve with a peak shifted towards positive correlations | 44 samples | Pollack et al. (2002) |
Correlation of averaged copy number and mRNA expression over chromosome segments | ||||
Glioblastoma multiforme | Gene expression and copy number for a chromosome segment defined in a segmentation algorithm | Positive correlations of ≥0.6 was found for 55 % of the human gene loci | 64 samples | Fontanillo et al. (2012) |
Gastric cancer | Correlation of average mRNA and gene copy number over samples of 20 Mb chromosome arm bins | Positive correlation of mRNA expression with gene copy number of the respective genomic region | 64 samples | Fan et al. (2012) |
Glioblastoma multiforme and acute lymphoblastic leukemia | Gene expression and copy number for a chromosome segment defined in a segmentation algorithm | Segmented gene expression and copy number significantly correlated with r = 0.6 and 0.19, respectively | Ortiz-Estevez et al. (2011) | |
Liver carcinoma | Transcriptome correlation map method (Stransky et al. 2006) was used to identify regions with strong concordance in gene expression and copy number changes | General strong association of gene expression and copy number changes | 139 samples | Woo et al. (2009) |
Colon carcinoma | Chromosome arm average gene expression compared to chromosomal copy number | Positive correlated shift in expression profiles | 32 samples | Grade et al. (2007) |
Neck squamous cell carcinoma | For each chromosome arm average Z-score gene expression was compared to average CGH; for arms 3p and 22q, averaged expression of smaller chromosomal regions was analyzed | 9/39 arms showed concordance in the direction of average gene expression versus copy number, 4/39 was opposite; loss of 3p and gain of 22q were reflected in consistent expression changes | 13 samples | Masayesva et al. (2004) |
In addition to the statistical method applied, sample size might influence the results; thus, studies calculating the gene-by-gene correlation as in (Holland and Cleveland 2012) over a large tumor sample set give more robust results. The correlation of median gene expression and copy number was consistently reported to be between 0.52 and 0.63 in colorectal cancer (Tsafrir et al. 2006; Grade et al. 2007). Analyses suggest that 10–40 % of the mRNA expression of five different types of tumors can be explained by copy number changes (Xu et al. 2010; Chin et al. 2006; Gu et al. 2008). Moreover, plotting the distribution of gene-by-gene expression and copy number correlation results in a normal-shaped curve with the mean shifted towards positive correlations (Xu et al. 2010; Pollack et al. 2002). The weak positive correlation becomes stronger by applying the approach described by Thompson and Compton (2010), i.e. correlating the average gene expression and copy number changes of a chromosome arm or applying a segmentation algorithm. This approach identified high correlation in three meta-studies on preexisting data (Gu et al. 2008; Ortiz-Estevez et al. 2011; Fontanillo et al. 2012). Moreover, average gene expression along a chromosome has been shown to predict chromosomal aneuploidy (Hertzberg et al. 2007).
Taken together, the effect of aneuploidy on the transcriptome is less pronounced in cancer tissues compared to model aneuploid cell lines owing to the population heterogeneity as well as genetic and epigenetic changes that were selected throughout the tumor evolution. Thus, aneuploidy is only one of many factors influencing the gene expression. Despite the heterogeneity of the approaches and interpretations, there is a trend towards a weak but consistent correlation of chromosome copy number changes and abundance of transcripts in cancer, which is prone to noise at the gene level, but becomes robust when only distinct regions of copy number changes are considered. In future, single cell analysis of cancer cells may provide more insights into the transcriptome changes and their correlation with copy number changes within cancer cell populations.
2.2.2 Aneuploidy and the Cancer Proteome
There are only a few studies that have systematically investigated the influence of aneuploidy on the cancer proteome. Myhre et al. (2013) compared DNA copy number, mRNA and protein levels of 52 breast cancer-related proteins in order to determine at which level the protein expression in cancer is regulated. A large fraction of the analyzed proteins (48.07 %) exhibited no correlation to DNA or mRNA expression values, 28 and 26 % showed a correlation of DNA to mRNA and mRNA to protein, respectively, and 15 % showed a correlation on all three levels. However, the sample size is too small to assume a general genome-wide effect of gene copy number on protein expression. In-depth proteomic analysis of two breast cancer cell lines revealed only weak correlations of 0.22 and 0.28 between gene copy number and protein expression changes (Geiger et al. 2010). Comparison of average expression to average copy number of adjacent chromosome regions increased the correlation. These results indicate that the effect of copy number alterations on the proteome level is even smaller than the effect on the transcriptome level. However, it should be noted that protein levels are influenced not only by gene copy numbers, but also by regulation of transcription, translation and by protein stability. In fact, only 40 % of individual protein concentrations can be explained by mRNA abundance in normal eukaryotic cells (Vogel and Marcotte 2012), which may partially explain the rather minor effects of gene copy number on mRNA and protein expression observed in cancer samples. This also suggests that many gene copy number changes are not reflected on protein levels and extensive proteome analysis will be required to fully estimate the effects of copy number changes on cancer proteome.
2.2.3 Gene Expression Signatures and Aneuploidy in Cancer
Finding commonly deregulated genes associated with cancer subtypes, prognosis or response to treatment remains central to cancer research (reviewed in Chibon 2013). Since aneuploidy is mostly associated with poor prognosis (McGranahan et al. 2012), a predictive gene expression signature for aneuploidy might be a promising approach for tumor classification. A gene signature is a group of genes, for which the expression is associated with a characteristic feature of cancer such as prognosis, subtype, instability or aneuploidy (Chibon 2013). In most cases, gene signatures are derived from transcriptome data, where the gene expression is correlated with cancer features and genes with the highest correlation build the signature. A well-known signature is the CIN70 signature derived from the NCI60 cancer cell line panel of cells to characterize cells with high CIN (Carter et al. 2006). The signature consists of 70 genes strongly correlated with the “total functional aneuploidy” as a proxy of overall aneuploidy across the NCI60 cancer cell lines. Therefore, CIN70 correlates with both CIN and aneuploidy without clear distinction. CIN70 successfully predicts the clinical outcome in multiple cancers, as a high net expression of the 70 signature genes predicts poor prognosis in the majority of cancer samples tested (Carter et al. 2006; Birkbak et al. 2011; Muthuswami et al. 2013). Recently, it was suggested that the CIN70 signature does not correlate with numerical heterogeneity in the NCI60 cancer cell line panel, but with proliferation (Sheltzer 2013). Nevertheless, the high predictive potential of CIN70 was confirmed. Moreover, a novel “HET70” gene signature, whose expression correlates with karyotype heterogeneity in the NCI60 panel was also shown to predict poor prognosis (Sheltzer 2013). In addition, more signatures, mostly for very specific cancer subtype classifications were published in recent years, all either based on or enriched for CIN genes (Chung et al. 2013; Ying et al. 2013; Szasz et al. 2013; Al-Ejeh et al. 2014). In uveal melanoma, a direct transcriptional comparison of high aneuploid and low aneuploid tumors, defined as tumors with >20 and <5 % of nondiploid chromosome arms, respectively, identified a 54-gene signature (Ehlers et al. 2008). Significant overlap of this 54-gene signature genes with genes upregulated in breast cancer, multiple myeloma, keratinocytes treated with UVB irradiation, and with cell cycle genes was found, but the prognostic relevance remains to be proven.
All above described gene signatures are linked to proliferative cellular processes such as replication, chromosome segregation (CIN70) and centrosome function, cell cycle regulation, DNA damage repair (54-gene signature), and the high net expression of the signatures are predictive. In contrast, these gene ontology terms are largely downregulated in model aneuploid cell lines. Accordingly, a gene signature (TRI70) associated with aneuploidy in model aneuploid cell lines predicted good prognosis (Sheltzer 2013), whereas aneuploidy in cancer is generally associated with poor prognosis (McGranahan et al. 2012). These results reflect a general reverse transcriptional pathway deregulation observed when comparing the transcriptome of aneuploid model cell lines and aneuploid cancers (Sheltzer 2013 and our unpublished results). We hypothesize that aneuploidy per se acts as a cellular stress factor and impairs essential cellular pathways. This results in a strong selection pressure for enhanced expression of the impaired factors. Thus, cells that gained mutations, gene amplification, additional aneuploidies and other changes that reverse the aneuploidy response may overcome the adverse effects. Along this line, comparison of different colorectal cancer stages revealed a selection for specific chromosomal imbalances throughout tumor progression that was accompanied by correlating transcriptional changes affecting an increasing number of cellular pathways (Habermann et al. 2007). In future, this hypothesis should be tested by determining the effects of the evolutionary adaptation to aneuploidy on transcriptome and proteome in aneuploid model cell lines and aneuploid tumors.
3 Consequences of Aneuploidy on Proliferation
In describing the cellular response to aneuploidy it is useful to distinguish the acute response to sporadic chromosome missegregation from the chronic consequences of aneuploidy (Fig. 4). In the acute response cells that missegregate their chromosomes often arrest their cell cycle or die (e.g., Thompson and Compton 2010; Li et al. 2010; Kuffer et al. 2013; Basu et al. 1998; Lanni and Jacks 1998; Dobles et al. 2000). The high frequency of cell cycle arrest after chromosome missegregation in higher eukaryotes suggests that not only chromosome loss but also chromosome gain is detrimental for cells. Missegregating cells accumulate the tumor suppressor p53 and the cyclin kinase inhibitor p21 in their nuclei in subsequent G1 (Thompson and Compton 2010; Li et al. 2010; Kuffer et al. 2013). Deletion of p53 or inhibition of the p38 mitogen-activated protein kinase (MAPK), which directly phosphorylates p53, abolishes the cell cycle arrest following chromosome missegregation (Thompson and Compton 2010). Thus, chromosome missegregation generates a p38 kinase–dependent stress response that activates p53 and triggers the cell cycle arrest. Other reports have described a role for the ataxia-telangiectasia mutated (ATM) gene product and its activation by increased levels of reactive oxygen species (ROS) and possibly also by oxidative DNA damage (Li et al. 2010; Kuffer et al. 2013). The high levels of oxidative DNA damage and ROS generated in cells immediately upon chromosome segregation errors might arise due to increased energy metabolism in aneuploid cells or due to the response to protein folding defects. The transcriptional pattern of chronic aneuploidy is suggestive of increased oxidative metabolism, although there is no overlap with transcriptional changes observed in cells subjected to oxidative stress (Stingele et al. 2012; Dürrbaum et al. 2014). In Drosophila epithelial cells, the response to chromosome missegregation is characterized by the activation of the c-Jun N-terminal kinase (JNK) signaling cascade, which triggers apoptosis independently of p53 (Dekanty et al. 2012; Clemente-Ruiz et al. 2014). Both JNK pathway and p38 MAP kinase belong to the class of MAP kinases that are responsive to stress stimuli such as osmotic shock, inflammatory cytokines, translation inhibitors, heat shock and ER stress, growth factors and elevated ROS. However, it remains to be clarified whether the presence of a single extra chromosome can indeed cause oxidative or ER stress and how quickly this response commences.
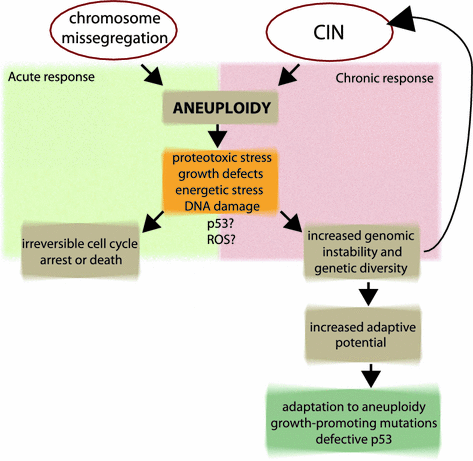
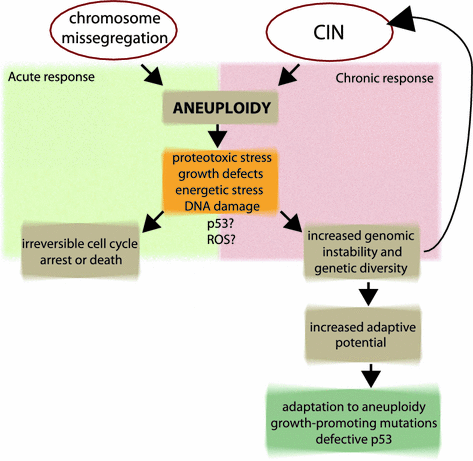
Fig. 4
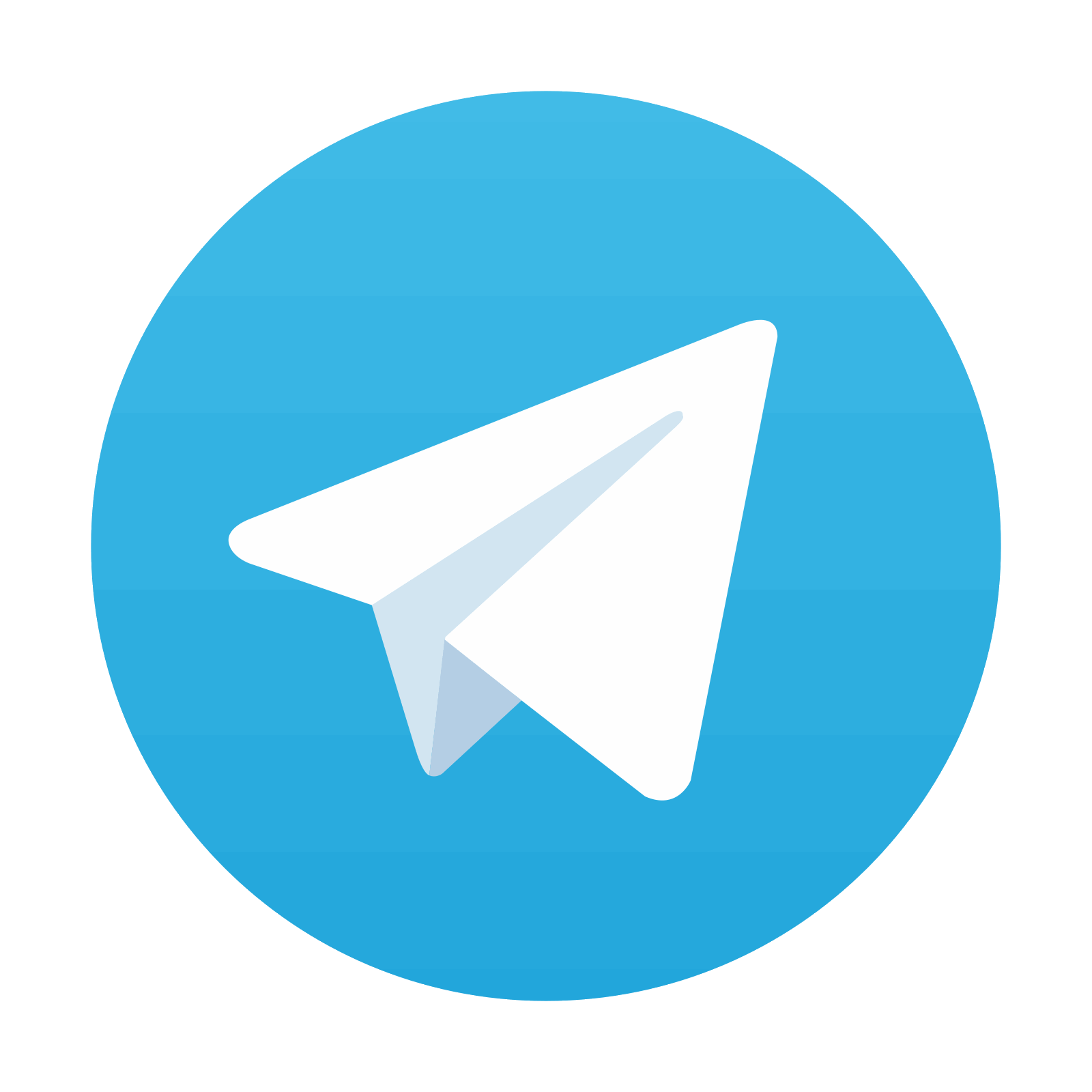
Correlation of gene copy and mRNA expression. a Gain of a chromosome (1) or unbalanced structural changes (2) result in DNA copy number changes, such as 1.5 fold increase in case of trisomy. b As a result, mRNA expression levels change as well, but the percentage of genes with mRNA abundance in concordance with DNA copy numbers might vary largely and depends on the applied cut off (light blue; see method 1). c Segmentation algorithms or averaging of the expression values improves the correlation of gene copy number and gene expression by diminishing the signal noise at the single gene level (see method 3)
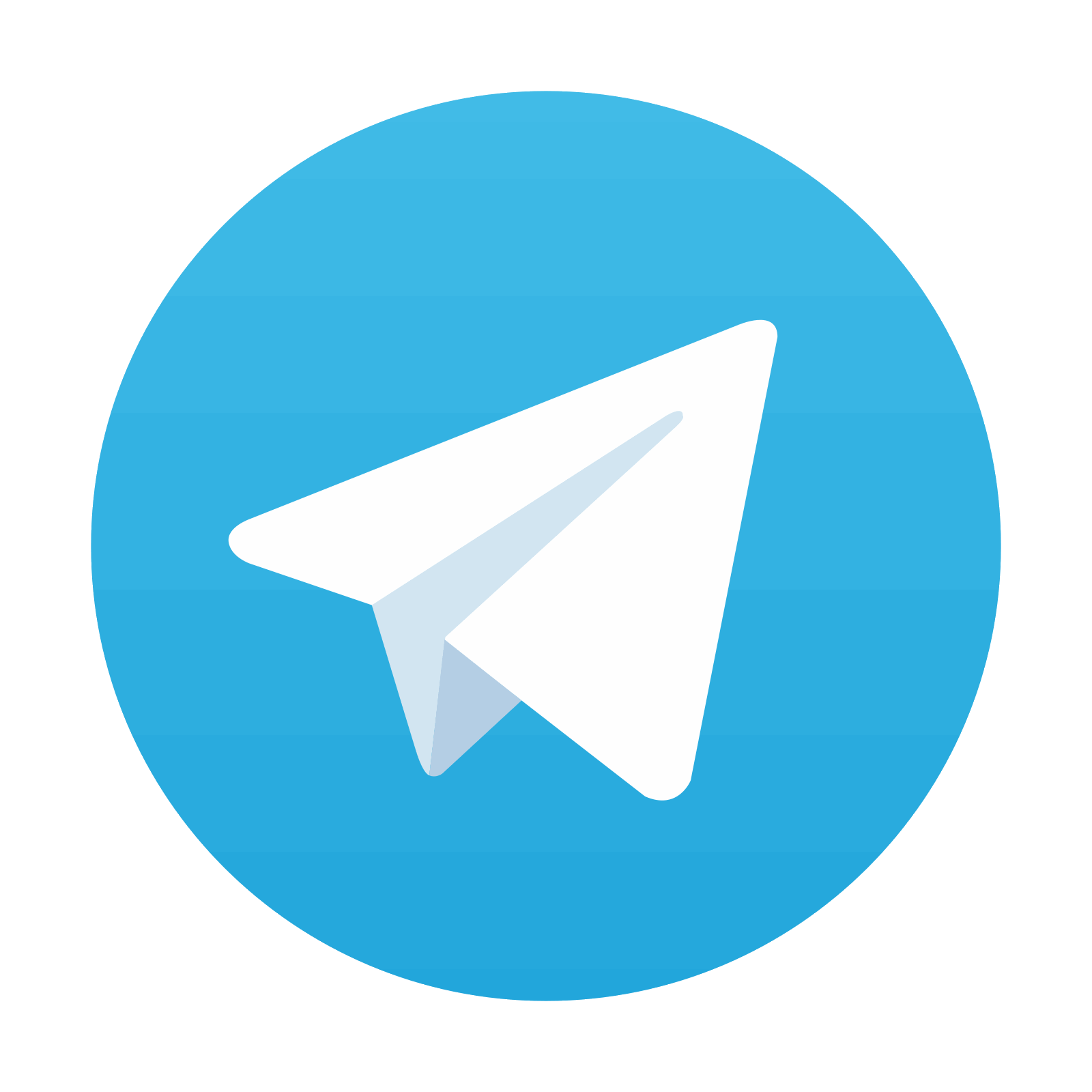
Stay updated, free articles. Join our Telegram channel

Full access? Get Clinical Tree
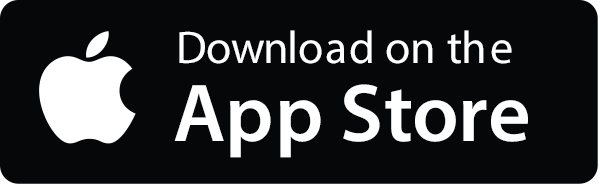

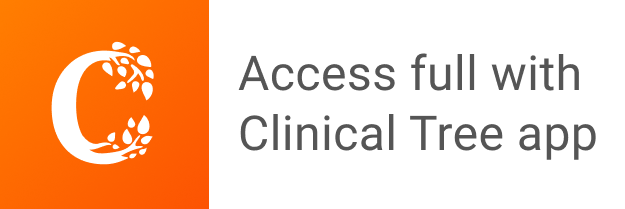