© Springer Science+Business Media New York 2015
Ashraf Khan, Ian O. Ellis, Andrew M. Hanby, Ediz F. Cosar, Emad A. Rakha and Dina Kandil (eds.)Precision Molecular Pathology of Breast CancerMolecular Pathology Library1010.1007/978-1-4939-2886-6_1010. Molecular Classification of Breast Cancer
(1)
Department of Histopathology, Division of Cancer and Stem Cells, School of Medicine, The University of Nottingham and Nottingham University Hospitals NHS Trust, Nottingham City Hospital, Nottingham, NG5 1PB, UK
Keywords
Breast cancerMolecular taxonomyGene expression profilingLuminalHER2Basal-likeTriple-negative breast cancerIntroduction
Breast cancer represents a heterogeneous group of tumours with different histological appearances, molecular features, varied behaviour and response to therapy. Scrutiny of these parameters at the individual level should improve patient management and therefore improves both the quality of life and the overall survival of the patient. The management of breast cancer patients currently relies on robust well-validated traditional clinicopathological prognostic factors and a limited number of predictive biomarkers (namely hormone receptors and HER2). Clinicopathological variables were the earliest used classification systems with strong overall association with patients’ outcome [1]. In addition to staging variables, histological grade, which represents morphological surrogate of tumour biological features has been validated by several independent studies as an independent prognostic stratifier in breast cancer [2]. Grade is incorporated into the well-validated Nottingham Prognostic Index (NPI) with equal weighting to that of lymph node stage to guide patients’ management [3]. Histological type refers to the growth pattern of the breast cancer as per microscopic examination [4] has also recognised to have prognostic value and can provide complementary prognostic information with grade [5]. Other clinicopathological prognostic variables include lymphovascular invasion, patients’ age, and menopausal status. Less validated variables include presence and extent of in situ component and tumour-associated inflammatory response.
There are several lines of evidence to suggest that the parameters currently available are insufficient to reflect fully the biological heterogeneity of tumours, and breast cancers of similar morphological appearances still vary in their behaviour and response to therapy. For instance, up to one-third of lymph node-negative breast cancer patients, classified as being within the good prognostic group, have been reported to develop recurrence later in their disease course [6]. Similarly, an equivalent proportion of node-positive patients, assigned into the poor prognosis group, remain free of distant metastases [7]. In addition, approximately 60 % of patients with breast cancer present as node negative, 80 % as oestrogen receptor positive and more than 40 % as grade 2 tumours. Further stratification of these cohorts is critically needed. Moreover, the widespread use of mammographic screening, improved understanding of the nature and biology of breast cancer and the increasing array of systemic therapy options (hormone, chemo and targeted therapy) [8] further emphasises our need to identify and stratify novel prognostic and predictive variables in breast cancer. This, in addition to the increasingly available systemic therapies and the move towards precision medicine have emphasised the need for identification of additional molecular prognostic classifiers that can reflect the degree of breast cancer biological and clinical heterogeneity.
Whenever possible, a molecular classifier test should be able to stratify patients into well-defined clinical outcome subgroups or into high positive and negative predictive response to a specific therapy. If assessment of a molecular marker does not lead to a decision in clinical practice, then its use in routine practice is discouraged [9]. Furthermore, recommendations for clinical introduction of molecular testing should be made only when the diagnostic methodologies are reliable, patients have access to the clinical services necessary to make informed decisions and interpret the results of testing, and it becomes clinically apparent how to utilise the results.
Biological variables in breast cancer can be assessed in routine practice using morphological surrogates such as tumour differentiation, proliferation status, and lymphovascular invasion or more accurately using molecular parameters such as assessment of gene/protein status individually or in consort (e.g. molecular profiling at the level of DNA, RNA or protein). Assessment of individual gene status in routine practice can be performed using different techniques such as immunohistochemistry (IHC; proteins), in situ hybridization (HER2) and enzyme-linked immunosorbent assay (tumour markers). Recent advances in human genome research, high-throughput molecular technologies and advances in bioinformatics have enabled the analysis of several hundreds and thousands of genes and gene products in one experiment and allowed the analysis of genes in the context of pathways and networks tackling the molecular complexity of breast cancer. Genome-wide profiling of chromosomal changes and rapid screening of genes for mutations/single nucleotide polymorphisms and methylation can be performed using techniques such as high-resolution single nucleotide polymorphism (SNP) arrays (SNP chips) [10], array comparative genomic hybridization (CGH) [11], multiplex polymerase chain reaction (PCR) and massively parallel sequencing (next-generation sequencing) [12]. Transcriptome and proteome profiling techniques include differential display [13], serial analysis of gene expression (SAGE) [14], gene expression microarrays (global gene expression profiling; GEP) [15] and massively parallel sequencing [16]. Massively parallel sequencing combines the high throughput of SAGE with the accuracy of EST sequencing. These molecular techniques hold promise for improving diagnosis, for the prediction of recurrence, and in aiding selection of therapies for individual patients.
Single Biomarker Classifiers
Of the individual molecular markers, the oestrogen receptor (ER), the progesterone receptor (PR) and the human epidermal growth factor receptor 2 genes (HER2) have proven predictive and prognostic value. ER, PR and HER2 status, which are essential part of the diagnostic workup of all breast cancer patients and they are routinely determined using a standardised technique and well-defined published guidelines [17–19]. It is currently recognised that the main consideration for treatment decision is endocrine responsiveness. Adjuvant endocrine therapy accounts for almost two-thirds of the overall benefit of adjuvant therapy in patients with ER-positive breast cancer. Use of anti-HER2 therapy is based on risk stratification and tumour HER2 status [20]. The current gold standard to asses ER status is IHC performed on formalin-fixed, paraffin-embedded cancer tissue. This diagnostic test is routinely used in the clinic, and major therapeutic decision-making dependent on the results; however, its reliability is not perfect. It has been reported that the existing IHC assays have only modest positive predictive value (30–60 %) for response to single-agent hormonal therapies. However, the negative predictive value of ER expression is high (i.e. ER-negativity which accounts for 20–30 % of breast cancer can identify the population of patients who will not benefit from endocrine therapy) [21–24]. Therefore, it is important to identify variables that allow identification of patients who can be safely spared adjuvant therapy or benefit from hormone therapy alone or combined with chemotherapy and/or targeted therapy. Approximately 40 % of ER-positive tumours are PR negative. Lack of PR expression in ER-positive tumours may be a surrogate marker of aberrant growth factor signalling that could contribute to tamoxifen resistance and that ER+/PR− tumours are generally less responsive than ER+/PR+ tumours [25, 26]. PR status can help to predict respond to hormone treatment, both in patients with metastatic disease [27] and in the adjuvant setting. Multiple studies have provided evidence for the prognostic and predictive importance of PR assessment in BC [26, 28–30].
Amplification of HER2 gene occurs in 13–20 % of BC and more than half (approximately 55 %) of these cases are ER-negative [31, 32]. Numerous studies found that HER2 gene amplification/protein overexpression is a predictor of poor prognosis and response for systemic chemotherapy [33–35]. Following the development of a humanised monoclonal antibody against HER2 and clinical trials demonstrating benefit of the use of anti-HER2 agents in patients with HER2 positive breast cancer [36], the reasons for establishing the HER2 status in routine clinical practice has changed, since it is a prerequisite for clinical use of anti-HER2 in patients with HER2-positive advanced disease as well as in the adjuvant setting for HER2-positive early stage disease.
Hormone receptor and HER2 are assessed in routine practice to provide information on response to endocrine therapy and anti-HER2 targeted therapy respectively. However, the expression of these biomarkers overlap and their prognostic and predictive value can be improved by using them in combination [37] or combined with the proliferation marker Ki67 [38]. Most IHC studies have also used a combination of ER, PR and HER2 as IHC surrogates to define the molecular classes initially identified by GEP. For instance, ER/PR positivity was used as a surrogate for luminal class and HER2 expression for HER2-positive tumours, while triple-negative (ER−, PR−, HER2−) phenotype is used to define the basal-like molecular class (BLBC) [39, 40]. In addition, some authors have classified HR-positive tumours that are also HER2-positive as the Luminal B subclass [39, 41]. Therefore, ER, PR and HER2 status provides an accessible biological molecular classifier of breast cancer with defined prognostic and predictive value and their value increases when they are used in combination where they can provide a valid practical surrogate of the GEP-defined molecular classes. Some other genes which are assessed individually in breast cancer such as Ki67 [42] have been proven of specific clinical utility. IHC expression of Ki67 is now widely used as an objective molecular measure of proliferation to overcome problems related to tumour fixation and mitotic figures identification [43, 44]. Although controversy regarding routine use of Ki67 in pathology practice, several studies have yielded promising results particularly its prognostic significance in node-negative [45, 46], ER-positive [47] and in histologic grade 2 tumours [48] and as a predictor of response to chemotherapy [49, 50].
Multigene Classifiers: Gene Expression Profiling and Molecular Taxonomy
Gene expression is a technical term to describe how a particular gene is active, or how many times it is expressed or transcribed, to produce the protein it encodes. This activity is measured by counting the number of mRNA molecules in a given cell type or tissue, though protein and not RNA is the functional product of genes. Although the first description of cDNA microarrays as a tool for profiling gene expressions was in 1987 [51], GEP technique was not widely used until advances in fluorescent labelling technologies, cDNA library construction and sequencing techniques have been achieved. The total gene expression pattern of a given sample is known as a gene expression profile and such expression data are often referred to as “signatures” or “portraits” as most tumours show certain expression profiles that are unique and related to a specific biological features [52, 53].
The introduction of the concept that breast cancer can be classified using GEP by Peru and colleagues in 2000 [54] has revolutionised breast cancer research and the number of studies classifying breast cancer using molecular classifiers have markedly exceeded our expectation. In fact GEP studies have not only contributed to our current understanding of breast cancer molecular complexity but also led to the identification of distinct molecular class of clinical relevance and the development of prognostic and predictive multigene signatures. Most GEP studies of breast cancer aimed at: (i) identifying specific molecular classes (class discovery; molecular taxonomy) that have biological and clinical relevance, which are unidentifiable by conventional means [54–56]. (ii) identifying specific molecular profile “gene signatures” that can predict tumour behaviour [57–60] and/or response to therapy [61–63] (class prediction). (iii) comparison of different “predefined” classes of breast cancer (class comparison) that aims to determine whether the expression profiles are different between these classes and, if so, to identify the differentially expressed genes [64–69].
Molecular taxonomy of breast cancer have been identified three main classes including (1) a luminal subgroup, which encompasses tumours that express ER and genes related to activation of the ER pathway; (2) a HER2 subgroup, which is characterised by overexpression of HER2 and by genes pertaining to HER2 amplicon; and (3) a subgroup of tumours that do not cluster with either luminal or HER2-positive tumours; the basal-like class, which is largely characterised by positive expression of basal cytokeratins and other genes that are characteristic of basal-like cells of the breast and by high proliferative activity [9–11, 19, 43]. Although HER2 positive and ER-positive luminal tumours were known before the advent of this GEP molecular taxonomy, BLBC class attracted attention as a novel class characterised not only by triple-negative phenotype (ER-negative, PR-negative and HER2-negative) but also by the a generally similar molecular profile (tumours clustered together at the molecular level) and the poor outcome [10, 11, 19]. Importantly, these classes showed distinct clinical outcome, with luminal subtype having the most favourable, HER2 overexpressing having the most detrimental and BLBC having poor prognosis, yet still relatively better than the HER2 overexpressing non-Herceptin treated cancers [55, 70]. The luminal class has further been classified into at least two subclasses; luminal A and luminal B with significant difference in molecular profile and clinical outcome. Luminal A tumours show high expression levels of ER-activated genes and low levels of proliferation related genes and have a good outcome whilst luminal B breast cancers show higher proliferation rates and/or HER2 expression with a significantly worse prognosis than luminal A tumours [71]. Other luminal subclasses have been described including luminal C [72] and luminal N [73] subclasses. Similarly, BLBC class has further been subclassified into subgroups such as claudin-low [74] and molecular apocrine subtype, which is characterised by androgen receptor (AR) expression and AR-related pathway with paradoxical expression of ER-related genes [75]. In addition, there are a few less defined molecular subtypes, such as normal breast-like class that displays a triple-negative phenotype but does not cluster with the basal-like centroid and is characterised by expression profiles similar to those found in normal breast tissue.
Early GEP studies suggest that the luminal class of breast cancer is characterised by ER positivity and other features characteristic of luminal mammary epithelial cells but is heterogeneous with respect to the expression of other genes and outcome. Subsequent studies [76] have demonstrated that that precise positioning of an individual within the spectrum of luminal breast cancer is based on expression of other genes. For instance luminal A tumours are characterised by high expression of luminal epithelial CKs and other luminal associated markers including ER and genes associated with ER function such as LIV1, hepatocyte nuclear factor 3 alpha (FOXA1), X-box binding protein 1 (XBP1) and GATA-binding protein 3 (GATA3) [77]. Whereas the luminal B group is characterised by low-to-moderate expression of the luminal A genes mentioned above, but is further distinguished by high expression of additional genes, mainly related to proliferation such as v-MYB, GGH, LAPTMB4, NSEP1 and CCNE1 [76]. Luminal tumour subclasses are also characterised by distinct type of genomic copy alteration and different level of amplification [78]. Although the class of breast cancer that is characterised by expression of markers characteristic of basal/myoepithelial cell was actually described many years ago [79, 80], they have attracted a lot of attention in recent years after their identification in GEP as a molecularly distinct subtype of breast cancer [54]. These tumours are known as BLBC represent 16 % up to 37 % of all breast cancer cases [68, 72, 81]. This class has received attention because of its nature as a poor prognostic group and as a good candidate for development of new targeted therapy. Most studies have shown that basal tumours are mainly included within a cluster in the ER-negative and HER2 negative tumours and are largely characterised by positive expression of basal cytokeratins (CK) and other genes characteristic of basal cells of the breast. Several basal class gene products identified are important structural elements of the basal cells of the breast [54, 82–84] and extracellular matrix (ECM) receptor proteins [85]. Other gene products included several proteins that activate signalling pathways, which are commonly deregulated in cancer [86] and gene products that have been implicated in cellular proliferation, suppression of apoptosis, cell migration, invasion and extracellular remodelling have been identified [87–89]. Basal class of breast cancer (BLBC) shows a high recurrence score and a poor 70-gene profile [90]. However, these results were not confined to BLBC and found also in the HER2 and Luminal-B subtypes. When BLBC were characterised using comparative genomic hybridisation (CGH) a greater genetic complexity with high frequency of DNA losses and gains was identified when compared to other breast cancer subtypes, suggesting a greater degree of genetic instability [91–93]. These tumours seem to harbour a dysfunctional BRCA1 pathway [94] and tumours arising in BRCA1 mutation carriers often display a basal-like phenotype [95, 96].
Although molecular taxonomy of breast cancer has attracted a lot of attention and speculation that this would result in dramatic improvements in breast cancer management, to date actual practical adoption appears limited. Certain critical issues have been raised such as validation, reproducibility and clinical utility. Most luminal tumours are ER-positive and can be identified in routine practice using IHC. ER expression and not luminal phenotype is recognised as a validated predictor to hormone therapy. The significance of luminal ER-negative tumours is not defined. Similarly, HER2-positive breast cancer patients are likely to be offered anti-HER2 therapy when indicated regardless of their molecular classification, while it is currently not justified to offer patients with cancers classified in the HER2-positive class if their tumours did not show evidence of HER2 gene amplification. Clinical relevance needs to be considered and factored into any emerging classification system to ensure that patients are treated appropriately. Furthermore, the so-called “normal breast-like” class is not well-defined [97] and the proportion of some classes defined by GEP varied substantially [98–100]. Moreover, it remains unknown how many molecular classes exist and more importantly how many classes can be reliably identified with the currently available data. From 4 [54] up to 10 [101] classes have been described. There remain major limitations in the ability to consistently assign a molecular class to new cases of breast cancer. The four main molecular classes frequently reported can be considered as an oversimplification of a novel molecular classification system and add little to our understanding of the biology and behaviour of breast cancer. The clinical difference between BLBC (GEP) and triple-negative (IHC) is disputed with triple-negativity provides more practical routinely applicable classification preferred by oncologists. Lumping of pure tubular carcinoma with micropapillary, invasive lobular or ductal NST carcinomas into a single “luminal” class or high-grade ductal NST, high-grade metaplastic and medullary carcinomas with low-grade adenosquamous and adenoid cystic carcinomas into “BLBC” class cannot be justified biologically or clinically.
It is also important to mention that other studies have reported different number and definition of molecular classes. For instance, in a study of 2000 breast cancers using an integrated approach of copy number and gene expression in a discovery and validation sets of 997 and 995 primary breast cancers, 10 novel molecular subgroups were reported [101]. These molecular classes were names as integrative clusters since they were defined based on both geneomic and transcriptomic profiles with consideration of the impact of somatic copy number variation on the transcriptome [101].
Microarray studies have primarily generated using invasive ductal carcinomas of no special type. However, subsequent studies have studied the gene expression pattern of some special types including medullary [102, 103], metaplastic [104], adenoid cystic carcinoma [105] and others [105]. Interestingly, tubular, mucinous and neuroendocrine carcinomas consistently displayed a luminal phenotype, whereas adenoid cystic, medullary and metaplastic carcinomas a basal-like phenotype [106]. For classification system that is applicable to all breast cancer patients, future studies of breast cancer classification should include samples of special types that comprise 25 % of breast cancer to provide standardised classification system and unravel the molecular basis of their specific morphologic patterns.
Multigene Signatures
In addition to the molecular classes, a number of multigene signatures have been identified through analysing GEP-derived data using different statistical/bioinformatics approaches. These gene signatures were developed based on the differential expression of a selected set of genes in a specific subgroup of tumours. Expression of these genes when analysed in combination can predict specific endpoint that is used to generate it. Multigene signatures include “prognostic gene signature” that can predict outcome. Other gene signatures were developed based on prediction of response to specific therapy and are used as predictive signatures. Prognostic signatures include the 70 gene signature or MammaPrint [81], the 76 gene signature [59], the genomic grade index (GGI) [107] and the Recurrence Scores (RS) of OncotypeDXTM [108]. A common character shared by all these signatures is the use of combinations of genes, rather than using single genes, to predict a certain outcome which appears to reflect the overall genetic derangements underlying the complex tumour biology. Of critical importance is the very minimal to negligible degree of overlap between these signatures regarding their gene sets/lists [109]. This minimal overlap has mostly been attributed to the different/limited breast cancer tissue samples used in these studies, different gene chips utilised and varied approaches followed for data analysis [110]. Nonetheless, the widely accepted assumption is that although gene lists overlap minimally, distinct genes may track similar biological processes and the overall predictive power of different array-based models shows significant concordance [90, 111].
There is growing consensus that multigene prognostic tests provide useful complementary information to well-established traditional clinicopathological variables. Importantly, these tests primarily rely on quantification of ER and proliferation-associated genes and combine these into multivariate prediction models. Due to the higher proliferation rates in ER-negative cancers, the prognostic value of these tests in ER-negative cancers is limited. It is not surprising that clinically useful prognostic signatures for ER-negative cancers are still non-existing [112]. Therefore, these signatures have so far not replaced the currently used prognostic/predictive factors in the management of breast cancer. Nevertheless, the two currently clinically utilised assays, MammaPrint and OncotypeDX, are used in conjunction with the clinicopathologic factors [113, 114].
Immunohistochemical Molecular Classification
Due to the aforementioned technical, cost and reproducibility issues, some groups have pioneered the use of expression data of IHC biomarkers in the routinely utilised paraffin-embedded breast cancer tissue sections. Many studies, using surrogate immunohistochemical panels of markers, have recapitulated these prognostic classes with considerable success and reproducibility [115–118]. The feature common to GEP subtying and their IHC surrogates is the use of a group of genes in the former, or tissue markers in the latter to define classes which has proven to be prognostically more informative than using these genes/markers individually. The choice of these biomarker panels was essentially based on the realisation that the GEP-derived molecular subtypes are a reflection of the ER status, HER2 status and proliferation status in breast cancer [119].
Different authorities have utilised IHC expression data to classify breast cancer patients into distinct subtypes significantly different in prognosis. For instance, data for the expression levels for a selective panel of 25 BC-related biomarkers evaluated using IHC on tissue microarray (TMA) was analysed using supervised classification approaches and artificial neuronal network. Markers were those related to epithelial cell lineage, differentiation, hormone and growth factor receptors and gene products known to be altered in some forms of breast cancer. Six groups or BC classes were identified which were significantly different in clinicopathological parameters and patient outcome in terms of overall and disease-free survival, independent of standard prognostic parameters; grade, tumour size and lymph node stage [117]. Of note, is the HER2 group was only 7 % of the studied patient population, which is below those reported in literature and in other studies.
Several groups have used IHC expression of ER, PR, HER2, basal cytokeratins (CKs) to devise a pragmatic molecular classification of breast cancer in routine practice. The resulting subtypes were classified as luminal, the HER2-overexpressing, and the triple negative/BLBC. The luminal subtype was subsequently subdivided into at least two subtypes, however, the criteria of defining luminal breast cancer subclasses are still based on different approaches utilising Ki67, PR expression and/or HER2 expression. It was initially proposed that those ER+ cancers overexpressing HER2 and being Ki67 high expressors (≥14 %) as luminal B, while the ER+, HER2 negative, Ki67 low as luminal A [47]. Later on, the international expert panel gathered in the St Gallen International Breast Cancer Conference in 2013, have endorsed the use of PR positive expression (≥20 %) to define luminal A breast cancer. They have recommended a Ki67 threshold of ≥20 % as an indicative of high Ki67 status in defining luminal B cancers [120].
The HER2 overexpressing/enriched breast cancers are those which showed evident unequivocal IHC expression of HER2 (3+), or those proven as HER2 amplified as assessed by in situ hybridisation (ISH) techniques. Because HER2 is an oncogene with the known impact of dismal outcome, based on the oncogene addiction theory [121], it has been proposed by many authorities, including our group, that HER2 overexpressing breast cancers should be allocated into the HER2 class/subtype irrespective of hormone receptor status [115]. The latter opinion, therefore, considers luminal B breast cancer to be those breast cancer characterised by high proliferative fraction as assessed by Ki67 expression at specific cut-off point. According to this definition, luminal B breast cancer has poorer outcome, and could benefit from adjuvant chemotherapy, which works better in tumour with high growth/proliferative fraction [122].
Probably, the most intensively debated issue within the topic IHC-defined breast cancer subtypes is BLBC. Although triple-negative breast cancer is defined by absence expression of ER, PR and HER2, there is no consensus definition, using IHC surrogate markers, for BLBC. Both TN and BLBC have poor clinical outcome and lack any modality of specific targeted therapy as those possessed by the HER2 overexpressing breast cancers. Different IHC markers have been used in defining the basal phenotype including: lack of ER, PR, HER2 expression (i.e. TN), and expressing one or more of the high-molecular-weight/basal CKs (CK5/6, CK14, or CK17) or being TN with expression of CK5/6 and/or EGFR and others [116, 123, 124]. However, comparative studies between the GEP-defined basal-like and IHC-defined BLBC are scarce [125]. This subtype is reported in the 15–20 % within most of the studied series. Therefore, this relatively low frequency hindered the development of consensus regarding BLBC. However, in their seminal meta-analysis of more than 10,000 breast cancer cases, Blows et al. reported the superior advantage of using five markers in definition of different molecular subtypes of breast cancer including the BLBC [118]. However, it is imperative that regardless of the panel of markers used in the characterisation of BLBC, most studies have reported poorer prognosis than the TN breast cancers [115, 116, 123].
The Nottingham Prognostic Index Plus (NPI+)
This algorithm devised by Abd El-Rehim et al. [56] was further refined through fuzzy rule induction algorithm to reduce the number of classifying markers/proteins to the minimum required to retain classification [126, 127]. Importantly, this approach used different multivariate clustering techniques, rather than a single clustering technique, with the main goal to derive a classification that is robust across different multivariate procedures. The initial step was dependent on the determination of the biological class of the tumour, through assessing the expression level of a ten biomarker panel; and the second step is the analysis of traditional clinicopathologic prognostic parameters. These ten biomarkers are ER, PR, HER2/c-erbB2, cytokeratin (CK) 5/6, CK7/8, p53, epidermal growth factor receptor (EGFR/HER1), c-erbB3/HER3, c-erbB4/HER4 and Mucin 1. These were selected from a large group of 25 markers of close relevance to breast cancer biology used in the original study [56] as the lowest number of markers that can preserve the same structure of the previous molecular classes [128]. This resulted in three main classes of luminal, basal and HER2, as previously established by Sorlie et al. [55] and with proportions consistent with breast cancer subtypes reported in other studies [116, 123]. Moreover, three further biologically and clinically relevant subclasses (luminal N, basal–p53 altered, and basal–p53 normal [73]) were identified. Using this panel of 10 IHC markers, we were able to subclassify unselected breast cancer series into seven distinct molecular classes that were biologically and clinically similar to those generated using 25 biomarkers [56]. These classes were used to develop a modified version of NPI by incorporating molecular and clinicopathological variables in a two-tier system [73]. Applying clinicopathological variables to each of the seven molecular classes using specific formulae generated novel prognostic index known as NPI-plus (NPI+), which improved patients’ outcome stratification that is superior to the traditional NPI.
Molecular Classification Challenges
Molecular classification of breast cancer is still in evolution. With the increasing use of more sophisticated techniques such as next-generation sequencing and other high-throughput techniques, large amounts of data will continue to emerge, which could potentially lead to identification of more molecular types of breast cancer having prognostic/predictive utilities. However, transfer of these classifications and predictive algorithms from the bench to the bedside is a lengthy process requiring mounting clinical evidence and robust validation. Moreover, cost-effectiveness and quality control of such classifications/algorithms are additional challenges.
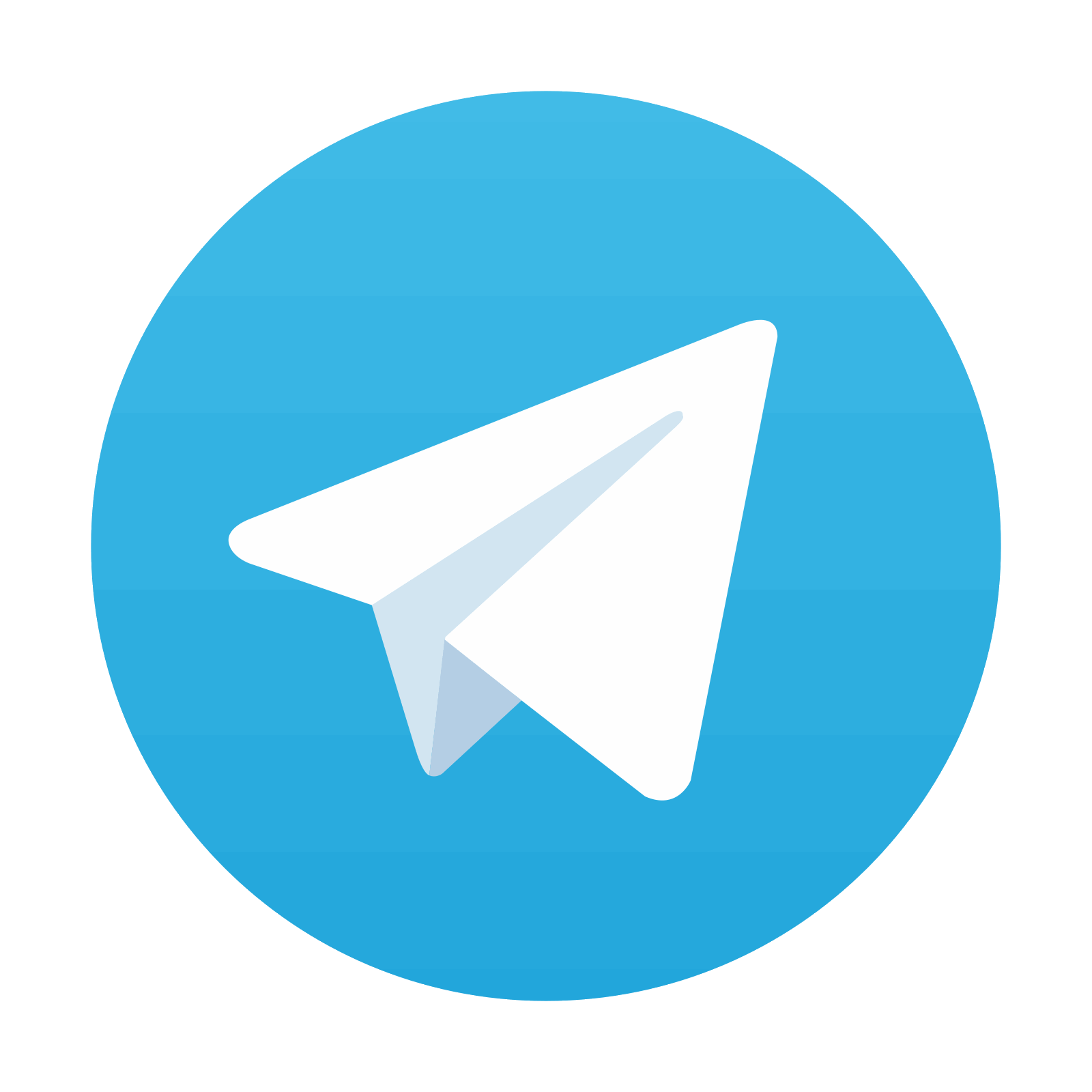
Stay updated, free articles. Join our Telegram channel

Full access? Get Clinical Tree
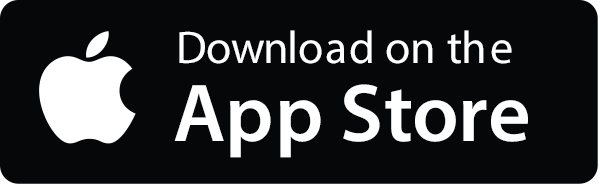
