Fig. 10.1
Summary of a typical treatment planning process (The image is courtesy M. Lewis from imPACT)
More recently, there has been interest in using prior treatment planning information, referred to as knowledge-based treatment planning (KBTP), possibly generated by experts to aid daily practice. The motivation for such an approach lies in reducing current complexity and time spent on generating a new treatment plan from each incoming patient. Clinicians typically have their own set of manual templates that they often use, which may give rise to inconsistency and increased patient risk [4–6]. It is believed that such a standardization process based on KBTP can help enhance consistency, efficiency, and plan quality.
10.2 Framework for Knowledge-Based Treatment Planning
The development of a framework for KBTP would require accumulation of information from past experiences related to patient, disease, imaging, treatment setup, dose, etc. A depiction of such system is shown in Fig. 10.2, in which a retrieval system allows the user to query “similar” cases from their archive and propagate such information to optimize the current plan at hand.
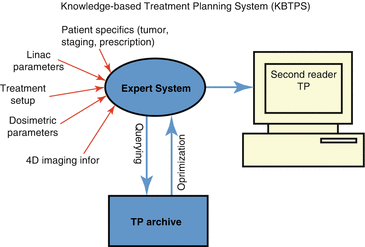
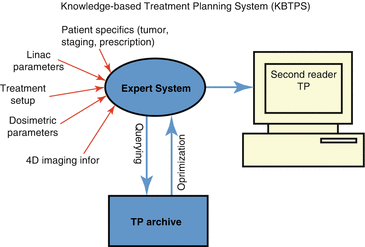
Fig. 10.2
Schematic of a hypothetical KBTPS, in which the user builds a query using features related to patient, disease, imaging, treatment setup, dose, etc for the treatment plan (TP). The database returns a set of similar treatment plans that the user could select from to optimize and compare with the current one according to the query
10.3 Clinical Applications
The implementation of knowledge-based approaches has taken several interesting directions as quality control of treatment and as a process to generate new IMRT plans as briefly discussed in the following.
10.3.1 Treatment Assessment Tools
Zhang et al. developed a machine learning approach for predicting normal tissue complications from dose-volume planning constraints without the need for explicit plan computation and demonstrated their method in cases of prostate and head and neck cancers [7]. Moore et al. developed and evaluated a model for predicting the organ-at-risk (OAR) dose from its overlap with the PTV and the prescription dose, and they demonstrated that the dose received by the parotid gland or the rectum could be indeed reduced using their model [5]. This work was extended to predict achievable dose-volume histograms (DVHs) using skewed Gaussian distributions from individual patient anatomy as shown in Fig. 10.3 [8].
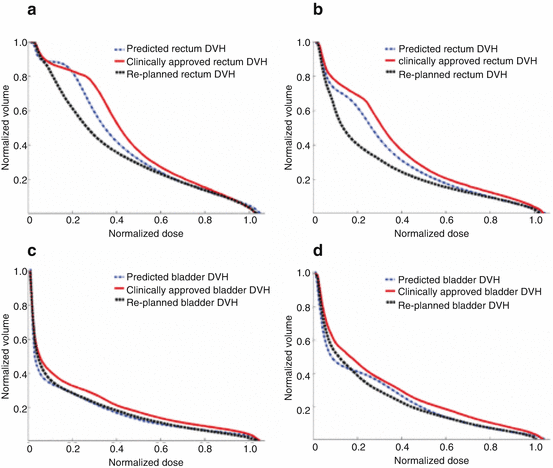
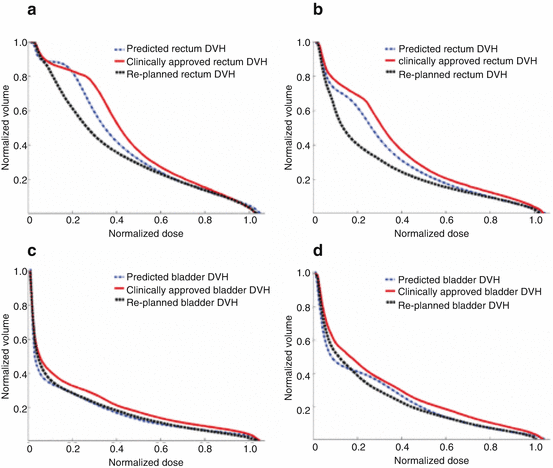
Fig. 10.3
Clinically approved DVHs compared to refined predicted DVHs and replanned DVHs for the (a, b) rectum and (c, d) bladder
10.3.2 IMRT Planning
As a demonstration of the feasibility of applying KBTP to the generation of IMRT plans in prostate cancer, Chanyavanich et al. presented a semiautomated method based on mutual information to identify similar patient cases by matching 2D beam’s eye-view projections of contours [4]. This approach was further evaluated on a larger pool of patients, and reported reductions were significantly lower for the rectum with 40 % of cases; the KBTP plan had better DVHs for rectum and bladder; in 54 % of cases, the comparison was equivocal; in 6 % of cases, it was inferior for both bladder and rectum as shown in Fig. 10.4 [6].
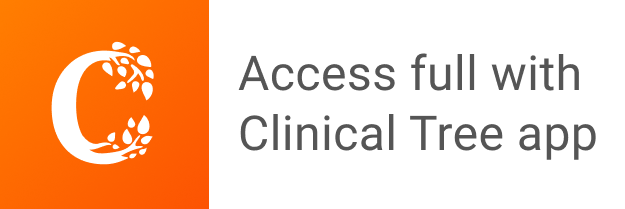