Interpretation of Functional Genomics
The paradigm of “nature or nurture” juxtaposes genetically determined traits to the formative environment. When we consider gene expression in a given cell or organism, these apparent opposites converge. Genes required for cell lineage determination and genes required for cellular responses to environmental conditions are equally transcribed into RNA. Not all genes however are expressed in all cells at all times. Thus, the “transcriptome,” or the genes that are expressed in a given cell at a given time, is only a fraction of the genome. The transcriptome integrates cell lineage, cellular functions, activity of regulatory or oncogenic pathways, and response to external factors. In hematologic malignancies, the quantitative analysis of the transcriptome has refined disease classification and provided powerful prognostic information. In addition, profiling of the transcriptome revealed activation of distinct oncogenic pathways, the importance of which can be experimentally tested by targeted genetic interventions using short complementary RNAs that reduce expression of a specific gene. In some cases, these approaches have led to the discovery of oncogenic mutations, thus linking the “structural” genetic information to the “functional” genomic characteristic of the sample under study. In recent years, whole genome sequencing technologies have been widely applied in oncology and are rapidly generating a comprehensive map of tumor mutations. Functional genomics will likely continue to be a powerful tool to study the role of these mutations in tumor biology.
Here, we focus on discussing general concepts of functional genomic methods and illustrate their application with examples primarily from the study of lymphoid malignancies.
GENE EXPRESSION PROFILING TO CAPTURE THE TRANSCRIPTOME
Two major techniques are now available to capture the complement of genes expressed in a cell: DNA microarrays and RNA sequencing. DNA microarrays consist of solid supports onto which probes have been attached that detect the presence of a specific RNA. Each array consists of thousands of such probes and each probe specifically hybridizes to one distinct RNA. A type of microarray technology commonly used employs oligonucleotide probes attached to a solid support. Affymetrix GeneChip® arrays are commercially available oligonucleotide arrays that depending on the specific array type can quantify the expression of approximately 47,000 transcripts (Human Genome U133 Plus 2.0). Novel sequencing technologies have made it possible to determine the sequence of all RNAs in a given sample. In addition to the actual sequence information, this technology also provides a highly quantitative measure of the relative abundance of a given RNA in the sample.
GENE EXPRESSION SIGNATURES IN MOLECULAR DIAGNOSIS, OUTCOME PREDICTION, AND TARGETED CANCER THERAPY
Microarray experiments typically yield several thousand data points per sample. The amount of data generated in such studies can easily overwhelm the researcher and statistician alike and makes “eyeball” analysis of the data virtually impossible. A number of analytical techniques aid in the interpretation of microarray data.1–4 In a so-called unsupervised analysis, statistical methods are used to visualize patterns of shared gene expression and to identify distinct groups of samples. This approach is independent of external data. “Supervised” approaches instead rely on statistical tests to relate gene expression characteristics to known biologic or clinical characteristics.
Unsupervised Analysis: Pattern Discovery by Hierarchical Clustering
One commonly used unsupervised strategy is called hierarchical clustering.1 This analysis identifies genes that share a similar expression pattern across all samples. For example, hierarchical clustering will group genes together that are highly expressed in one group of samples and lowly expressed in a second group. Genes that are involved in the same cellular function are often coordinately expressed and thus form a distinct “gene expression signature” of a particular biologic process.5 Gene expression signatures capture biologic characteristics, including cell type, differentiation state, cellular functions, and activity of signaling pathways, and thereby provide a framework in which the complexity of microarray data can be related to the biology of the study samples. Hierarchical clustering is a valuable tool to discover such patterns of coordinately expressed genes. The strength of this analysis is the focus on distinct biologic functions, represented by sets of genes contributing to the same process rather than isolated genes. For example, to proliferate, a cell simultaneously expresses a set of hundreds of genes involved in cell cycle progression, DNA replication, and metabolism, which upon hierarchical clustering can be visualized as a proliferation signature. Gene expression studies often use a single array per sample. The apparent lack of replicates is sometimes felt to make such data inferior. However, signature-based analysis strategies intrinsically are based on numerous replicates, which are more valuably biologic as opposed to technical replicates.
Hierarchical clustering can not only identify genes with coordinate expression across samples but also group samples that share a common pattern of gene expression. Hierarchical clustering can thereby dissect the heterogeneity of tumor samples that may be very important clinically.6–8 Thus, hierarchical clustering is an especially useful tool for “question-driven” as opposed to “hypothesis-driven” analysis of a data set and can uncover unexpected associations.
Experimentally defined gene expression signatures are catalogued and made available for statistical analysis.3,5,9 Signature-based analysis algorithms can provide molecular classifications of cancer types, establish prognoses, identify cancer subtypes with sensitivity to specific pharmacologic interventions, establish optimal drug combinations, and facilitate the discovery of novel pathway inhibitors. Strategies that have proven particularly effective are gene set enrichment analysis (GSEA) and the connectivity map. GSEA provides a statistical measure of the probability that a set of genes contains a predefined functional signature.4 This method can test whether the gene expression difference expressed between two tumor types are due, for example, to differential activity of the nuclear factor kappa B signaling pathway; similarly, the effect of a drug can be related to a distinct signaling pathway. To link these characteristics of cancer and drug, the connectivity map was developed.3 In essence, the gene expression profile is used to match tumor biology with the mechanism of action of a pharmaceutical agent. Such methods aid in drug development and may guide the clinical use of cancer therapy by identifying patient populations likely to benefit from a given intervention.
Supervised Analysis: Building Molecular Predictors of Diagnosis, Prognosis, and Treatment Response
“Supervised” analytical methods use biologic or clinical data to search for gene expression differences that are most informative for diagnosis or prognosis. To derive a molecular predictor of
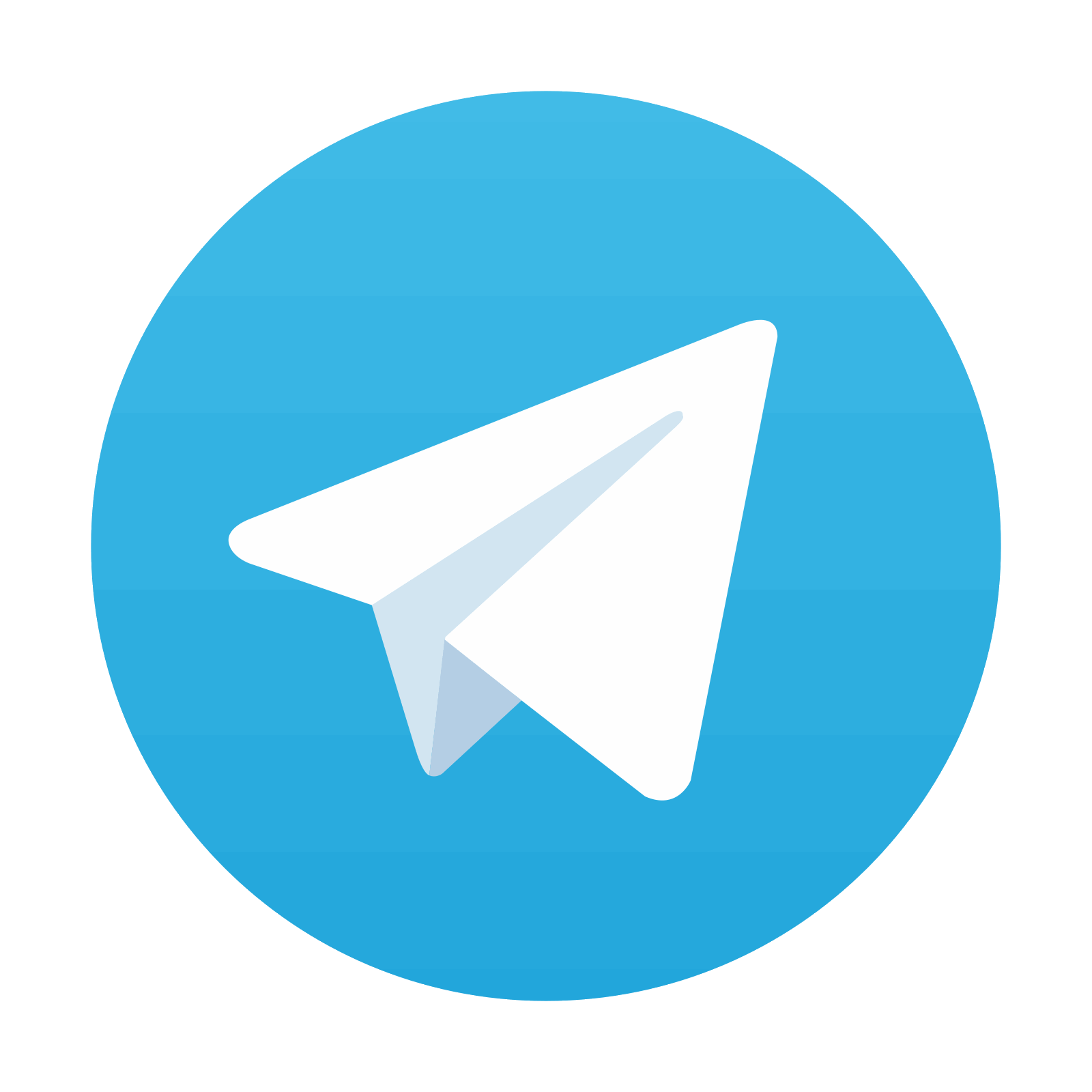
Stay updated, free articles. Join our Telegram channel

Full access? Get Clinical Tree
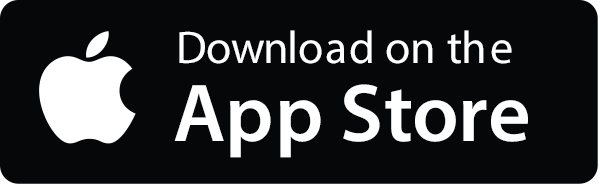
