Databases
Utility
Ensembl
Genome sequencing data
UCSC Genome Browser
Entrez Gene
Genome annotation data
KEGG, HPRD, DIP, MIPS, MINT, BioGRID, IntAct
Gene Ontology Annotation Database
Universal Protein Knowledgebase
Genome Reviews
Kyoto Encyclopedia of Genes and Genomes
Biochemical pathways and functional associations
Gene Ontology
The SEED
MetaCyc
BioCyc
Transport DB
Database of Quantitative Cellular Signaling
Protein interaction networks
Protein interaction networks Database of Interacting Proteins
Molecular INTeraction Database
Mammalian Protein–Protein Interaction Database
Protein interaction networks Database of Interacting Proteins
Gene Expression Omnibus 50
High-throughput genome-scale data
Stanford Microarray Database
Transcriptomics
Proteomics Identifications Database
Proteomics
Cancer Program Datasets Portal: www.broadinstitue.org/gsea/msigdb/
Molecular Signature Databases (MsigDB): www.broadinstitute.org/gsea/msigdb/
Achilles Project Datasets 1.0 (1.02 cell lines): www.broadinstitute.org/icbp
B Cell Interactome: http://amdec-bioinfo.cugenome.org/html/BCellInteractome
Memorial Sloan Kettering Cancer Center: http://www.pathwaycommons.org
Oregon Health and Science University (OHSU), SAGE Bionetworks, SYNAPSE: heiserl@ohsu.edu
DREAM Breast Cancer Prognosis Challenge: https://synapse.sagebase.org
Kmethylome: http://cbbiweb.uthscsa.edu/
Hormone Receptor Target Binding Loci Database (HRTBLDB): http://motif.bmi.ohio-state.edu/hrtbldb/
miR2Disease: http://www.mir2disease.org/
Linear Programming
Linear programming-based machine learning techniques are used to increase the accuracy as well as objectivity of diagnosis and prognosis of breast cancer. Linear programming helps the physician and patient by providing the information to plan the treatment that may eliminate the need for the prognostic surgical biopsy procedure. One of the Xcyt image analysis programs has been used to perform the analysis of cytological features based on a digital scan in cancer patients. It diagnoses the image as malignant or benign, along with the estimated probability of accuracy, and predicts the recurrence of cancer. This system was first used by a surgical oncologist in 1993 to classify 131 cases with 100 % accuracy clinically. Another example of linear programming is the use of a recurrence surface approximation (RSA) program for predicting the recurrence of cancer after its surgical removal [26]. Linear programming also gives a probability of malignancy that allows a patient to compare the specific diagnosis with hundreds of cases reported previously.
Statistically Derived Models of Breast Cancer and Molecular Networks
Statistical models of breast cancer can be divided into two types. The first type of model employs unbiased statistical inference using appropriate algorithms, and the second one incorporates a priori constraints of specific biological interactions from data [11]. These models help researchers to develop, quantify, and test various treatment hypotheses quickly and efficiently. Statistical models at the chromosomal, genetic, transcriptomics, and pathway levels provide critical insights into molecular mechanisms and consequences of malignant tissue transformations, despite incomplete information of underlying biological interactions [11]. These methods are helpful in the elucidation of key bimolecular events and pathways involved in oncogenesis. Numerous studies have sought to infer the structure of small- and large-scale bimolecular networks in human cells.
Efforts have been made to craft network-based statistical models of cancers, including breast cancer, in which the architecture of regulatory networks for a portion of the human genome is characterized [27–30], e.g., the Bayesian model. The Bayesian model discriminates between physical and functional interactions between several thousands of genes [31]. The related probabilistic Boolean network formalism model has been used to construct other cancer types, e.g., gliomas [32].
Different transcriptional classifiers have been developed for the identification and discrimination of cancer types, subtypes, and grades: hierarchical clustering, k-means clustering, support vector machines, artificial neural networks, and classifiers based on the relative expression of gene pairs [33–36]. Transcriptomic signatures have also been applied to model relapse and overall survival in different types of cancers and are used to predict the tumor response to chemotherapeutic agents. For example, Gene Set Enrichment Analysis (GSEA) and related tools have been developed and applied to identify pathway perturbations in human cancers on the basis of transcriptomic data including breast cancer [37–39]. These models often start with genome-scale microarray data and through computational, or combined with, experimental analyses derive prognostic classifiers consisting of a lesser number of highly relevant transcripts. The statistical signatures have potential utility for informing small-molecule, radiological, and surgical treatment choices which cannot be measured by standard histopathological and clinical analyses [11]. Statistically inferred network models can be used to study the topology of complex cellular systems and to explain important genetic interactions and control points [11]. These are used to map the status of regulatory agents into qualitative states. The availability of larger high-throughput datasets encoding different facets of regulatory interactions, combined with innovative methods for their integration, would enable the construction of precise numerical network models [11].
Breast Cancer Risk Assessment Tool (BCRAT) and International Breast Cancer Intervention Study Model (IBIS)
Different breast cancer risk models are used by clinicians for patients considered at average and above-average risk based largely on their family history as well as genetic factors [40]. The Breast Cancer Risk Assessment Tool (BCRAT), based on the Gail model, is used to determine whether a woman meets the minimum risk threshold of a 5-year risk of at least 1.67 % in order to consider tamoxifen for chemoprevention [41, 42]. This is the most frequently used breast cancer risk assessment tool in the United States [43]. Current age, age at menarche, age at first live birth, number of previous biopsies, history of atypical hyperplasia, race/ethnicity, and number of affected first-degree female relatives are included in this model. However, this model does not include information on the BRCA1/2 mutation status or extended family history. In comparison, the Intervention Study Model (IBIS) includes BRCA1/2 mutation status and extended family history, along with other nongenetic risk factors, including age at menarche, parity, age at first live birth, age at menopause, history of hormone replacement therapy used, etc. The BCRAT model has been used in several large cohorts and has been found to be well calibrated for women at average risk [44–47]. However, the short-term and lifetime breast cancer risks assigned to a woman by the BCRAT and IBIS models vary considerably. The BCRAT model tends to assign lower risk than the IBIS model to women who have a strong family history of breast cancer [48]. Therefore, the BCRAT model is not recommended for risk assessment for these women, nor for women under the age of 35 or those with a personal history of lobular or ductile carcinoma in situ. Quante et al. compared the two models and concluded that the IBIS model performed better in a cohort of women whose risks span the continuum of breast cancer risk [49]. Extending models that already capture the extended family history and genetic information like the IBIS model may help risk models play a major role in disease prevention.
Network-Based Models
Computational prediction and prioritization have proven to be complementary to genetic mapping, in terms of integrating existing information on disease biology and relatively unbiased whole-genome measurements [50]. Interdependent interactions of genes and proteins form complex cellular networks—signaling networks, gene regulatory networks, and metabolic networks. The computational models of breast cancer involving these pathway networks provide insights into molecular etiology and consequences of malignant transformations.
For modeling and evaluating, the structure of proteins actively involved in breast cancer, amino acid sequences are retrieved from UniProtKB/Swiss-Prot. This provides descriptions of a set of proteins, their function, domain structure, posttranslational modifications, and variants. Template selection and target structure modeling includes structural homologous entries, obtained for proteins from local alignment search using Basic Local Alignment Search (BlastP). Comparison of homology models with known template reveals similarities between biochemical and biological functions to be inferred. Homology modeling is based on the notion that new proteins evolve gradually from the existing ones by amino acid substitution, deletion, addition, and three-dimensional structures and functions. This method tries to identify structures similar to target proteins via sequence comparison [51].
A brief introduction about networks is important to understand the modeling processes. A network is defined as an efficient abstraction of biological systems [52]. Nodes and vertices in a molecular network are used to represent biomolecules, such as genes, proteins, and metabolites. Edges or links between nodes have been used to indicate physical or functional interactions, including transcriptional binding, protein–protein interaction, genetic interaction (such as synthetic lethal), biochemical reactions, and many others [50]. An edge on a network (if it happens in the cell) shows that two molecules are functionally related with each other, and the distance on a network is correlated with functional similarity [53]. Network/graph theory provides multiple definitions and tools to measure the distance/proximity between two nodes on a network, which makes network analysis particularly suitable for the quantitative modeling of gene–gene and gene–disease relationships [50]. Network analysis has been found to provide powerful tools to fully exploit the potential in human disease study; for example, in genome-wide screening studies on cancer mutation, it was found that though ~80 mutations can be present in a typical cancer, they tend to fall into a few functional pathways [54]. Network-based approaches have been used to predict the disease genes, with a much better performance than traditional approaches of disease gene prediction.
The discrete mutational events that are found in the cancer genome and epigenome substantially modulate the transcriptional profile within the cancer cells. Models based on these perturbed gene expressions can be applied for the diagnosis and prediction of disease subtypes and stratification of different tumor grades [11]. The Gene Ontology Consortium has been devised as a controlled vocabulary for describing molecular functions and biological processes of genes based on information given in the literature and from available databases. Classification of the mutated gene is available on Osprey [55]. Lin and colleagues identified 50 mutated genes and 77 mutations belonging to calcium ion binding group involved in breast cancer disease [56]. The authors used as- Different models (of multidimensional analysis of mutates genes) of sequence similarity, functional annotation, and protein interactions were used and it was found that five groups were associated with extracellular matrix organization and biogenesis, extracellular matrix cellular cell–cell adhesion, microtubule binding, and actin binding [56]. Different transcriptional classifiers have been developed, including hierarchical clustering, support vector machines, and artificial neural networks. In addition to these, classifiers based on the relative expression of genes pairs have also been developed [33–36].
Disease genes and other information are mapped to the network, and a scoring scheme scores each candidate gene according to its relative position on the network, as well as additional information. The score is supposed to reflect the probability of the candidate gene causing the disease. Finally, all candidate genes are ranked according to the score, and the top genes are predicted as disease-causing genes. The predictability of this proposed approach is often assessed by cross-validation with known gene–disease relationships. Therefore, the scoring scheme is the key to a disease gene prediction method [50].
Cellular Networks
Cellular networks are the core basis of the biological complexity of cancer cells. Cellular networks include:
Protein interaction networks: encode the information of proteins and their physical interactions.
Signaling networks: illustrate inter- and intracellular communications and the information process between signaling proteins.
Gene regulatory networks: describe the regulatory relationships between transcription factors and/or regulatory RNAs and genes and metabolic networks of biochemical reactions between metabolic substrates and products [57].
Regulatory networks: consist of hub genes as global transcription factors; they may govern a large amount of genes in response to signals (external/internal).
Jeong and Lee have developed candidate regulatory network in human breast cancer cells and compiled a set of 425 transcriptional factors and 548 signal transduction from the gene ontology site [58]. The curated cluster 1,424 has been found to have 49 genes related to cell cycle and 26 genes related to cell division. The cluster was having activities responsible for cell growth [59]. The authors validated the gene ontology-enriched cluster using the TRANSFEC and HPRD databases. TRANSFEC has transcription factor target relationship and HPRD has information regarding protein–protein interactions.
Networks are presented as directed or undirected graphs. Protein interaction networks are modeled as undirected graphs where nodes represent proteins and links represent physical interactions between proteins. Directed graphs are used to present gene regulatory and metabolic networks. In the case of gene regulatory networks, nodes represent transcription factors or genes, while links represent regulatory relations between regulated genes or transcription factors [57]. Signaling networks are presented as graphs containing both directed and undirected links. In these networks, nodes represent proteins, directed links are used to present the activation or inactivation relationships between proteins, and undirected links are used to represent physical interactions between proteins. Signaling networks are far more complex in terms of the relationships between proteins in comparison to cellular networks, e.g., nodes may represent different functional proteins such as kinases, growth factors, ligands, receptors, adaptors, scaffolds, transcription factors, and others. All these have different biochemical functions and might be involved in different types of biochemical reactions characterizing a specific signal transduction machinery [57].
Integrative Network Analysis of Breast Cancer-Associated Genes
A particular type of phenotype is the result of a collaborating network of a group of genes, which might not belong to the same functional category. Therefore, the integration of microarray-generated gene lists onto the cellular networks would help in analyzing and interpreting the biological significance of the genes in a network [57]. This provides a structured network knowledge-based strategy to analyze genome-wide gene expression profiles in the context of known functional interrelationships of genes, proteins, and their phenotypes. Mutated cancer genes were studied from literature to uncover their intrinsic properties with the help of a human protein interaction network which was constructed from the entire human genome using an ontology-based method [57, 60]. In this study, a total of 346 genes encoding 509 protein isoforms were mapped onto the network. This analysis showed that cancer proteins have, on average, twice as many interaction partners as other proteins in the network, therefore, implying the evolutionary aspects of cancer genes [57].
Cancer proteins have been reported to display a high ratio of highly promiscuous domains, in terms of the number of different proteins with which they interact. This indicates that they play central roles in many biological processes and mutations in these proteins, which could lead to a higher cancer incidence [57]. The most frequently found domains in the cancer protein population have functionalities particularly focusing on DNA regulation and repair, such as zinc-finger, PHD-finger, BRCT, and paired-box domains (i.e., all transcription factors) [57].
The work carried by researchers in this direction provides a biological insight into the global protein interaction network properties of cancer proteins and uncovers one of the most striking properties of cancer proteins—that cancer-associated proteins are network hubs playing a central role in biological systems. Each hub of cancer proteins reflects a specific domain of a cellular function, which suggests that mutations of an individual or a few hub proteins together may lead to oncogenesis or cancer progression [57]. However, these studies provide little insight into the oncogenic mechanisms, simply because protein interaction networks have limited information compared to signaling networks in which protein regulatory (activation and blocking) information is encoded.
In a biological system, cells use a sophisticated communication between proteins to perform a series of tasks such as growth and maintenance, cell survival, apoptosis, and development. Signaling pathways are important in order to maintain cellular homeostasis and determine cell behavior. Therefore, alterations in the expression of genes and their regulators would reflect on these cellular signaling pathways, thus leading to tumor development and/or the promotion of cell migration and metastasis. In fact, mutations in genes which encode for signaling proteins have been commonly seen in many types of cancers, including breast cancer [61]. Structural analysis of a literature-mined human cellular signaling network containing 500 proteins has shown that signaling pathways are intertwined to manage the numerous cell behavior outputs [62]. This work provided a framework for the understanding of signaling information processing within the cells. For example, in an examination involving receptor tyrosine kinases, it was observed that the complex and overlapping cross talk involved in signal transduction can be explained by linear combinations of docking affinities for downstream proteins [63]. Furthermore, interactions between microRNAs and the signaling network revealed the principles of microRNA regulation of the network [64]. These approaches hint that an integrative analysis of signaling networks with cancer proteins would highlight the characteristics of cancer proteins [57].
Computer-Aided Early Diagnosis of Breast Cancer
Computer-aided early diagnosis of breast cancer helps the physician to optimize the treatment [65, 66]. In order to improve the accuracy of diagnosis, as well as prognostic risk, a number of computer-aided diagnostic approaches have been proposed for breast cancer. The Bayes classifier combined with feature selection to diagnose breast cancer was applied by Butler and Web [67]. It reached 90 % accuracy by using X-ray scatter images. Abonyi and Szeifert obtained 95.7 % accuracy by applying supervised fuzzy clustering technique [68]. In a study carried out by Osareh and Shadgar [69], the authors investigated the issues of breast cancer diagnosis and prognostic risk evaluation of recrudescence and metastasis by using three well-known classifiers: support vector machine (SVM), K-nearest neighbors (KNN), and probabilistic neural networks (PNN). These classifiers were combined with signal-to-noise ratio, feature ranking method, sequential forward selection and principal component analysis, and feature extraction based on dataset I and gene microarray dataset II, respectively. They concluded that the best overall accuracy for breast cancer diagnosis is achieved equal to 98.80 and 98.33 %, respectively, using support vector machine classifier models against two widely used breast cancer benchmark datasets [69].
Microcalcifications
Clustered microcalcifications have been considered as important indicators of the presence of breast cancer. This system is based on the analysis of optimized visual examination of certain cancer indices. The detection of microcalcification is implemented via an algorithm based on (a) high-pass filtering, (b) variance normalization, and (c) adaptive filtering. Each microcalcification is given an estimated risk based on the flow chart built with expert’s rule. The final diagnosis consists of an estimation of risk of the suspected microcalcification cluster. The four main virtual zones of risk include:
Zone1: risk between 0 and 35 % (benign)
Zone 2: risk between 35 and 55 % (benign with doubt)
Zone 3: risk between 55 and 70 % (malignant with doubts)
Zone 4: risk between 70 and 100 % (definitely malignant)
The image-processing algorithms have helped in revealing microcalcifications from the noisy and low-contrast mammograms.
Cryosurgery
Cryosurgery (also called cryoablation or cryotherapy) is currently used as the surgical method to treat localized tumors because of its advantages over other applications. Optimization and even integration of patient-specific modeling, meshing, thermal analysis, post-processing, and prediction of the treatment outcome into a single software have become essential. In a study by Jung, a computerized treatment planning tool was developed for cryosurgery of breast cancer, taking into account patient-specific diagnostic information [70].
Finite Difference (FDTD) Modeling of Breast Cancer
Microwave-based imaging is the most promising technology to detect breast cancer. This technique exploits the dielectric constant between normal and malignant breast tissue at microwave frequencies. Finite difference (FDTD) modeling is a numerical modeling technique used to model the propagation of electromagnetic waves in biological tissues [71]. The FDTD model critically represents the dielectric properties of normal and cancerous breast tissues and helps in the detection of the cancerous tissues. In a study carried out by Lazebnik et al., it was shown that the Debye parameters can be readily incorporated into numerical breast phantoms used in breast cancer detection and treatment applications [72].
Correlating Protein Interaction Network and Phenotype Network to Predict Disease Genes (CIPHER)
The data, including phenotypic similarity and protein networks, can be used in CIPHER (Correlating protein Interaction network and the PHEnotype network to pRedict disease genes), with drastically different formulation [73]. In this study, the researchers have chosen to directly model the correlation between disease phenotypic similarity and gene functional relatedness and have used the correlation to prioritize candidate genes [73]. The CIPHER approach has been found to accurately pinpoint the true disease genes from linkage loci or from the whole genome. CIPHER can be applied to de novo discovery without any modification, that is, to diseases without known disease genes (without mapped locus or with mapped but uncharacterized loci). In a case study of breast cancer to demonstrate CIPHER’s ability in de novo discovery of breast cancer genes, 16 known breast cancer genes were treated as non-breast cancer genes. The whole human genome is prioritized by CIPHER.
While using a shortest path measure of distance (CIPHER-SP), the well-characterized breast cancer gene BRCA1 was ranked at the top, and the other 10 of the 16 genes are ranked in the top 300, roughly the top 1 % of the human genomes. Additionally, among the top 10 % of the prioritized human genomes, the de novo prioritization has identified 15 genes which have been suggested recently among novel breast cancer genes, including AKT1, ranked at 27. ATK1 is a novel oncogene, and a transforming mutation has been identified in human breast, colorectal, and ovarian cancers [74]. Therefore, this case study shows that all the advantages of CIPHER enable us to perform genome-wide candidate gene prioritization for almost all diseases, including breast cancer, leading to a comprehensive genetic landscape of human diseases [73].
Biochemical Reaction Network
Biochemical reaction networks are constructed to represent the relationships between genes, proteins, and the chemical interconversion of metabolites within a biological system of cancer cells. Biochemical networks are better in contrast to statistically inferred networks. In these models, network links are based on preestablished bimolecular interactions rather than statistical associations; significant experimental characterization is thus needed to reconstruct biochemical reaction networks in human cells. These biochemical reaction networks require, at minimum, knowledge of the stoichiometry of the participating reactions. Additional information such as thermodynamics, enzyme capacity constraints, time-series concentration profiles, and kinetic rate constants can be added to construct more detailed dynamic models.
Stoichiometric Models
Stoichiometry is the study of the balance of energy and multiple chemical elements in biological systems. The stoichiometric model is the most basic mathematical representation of a biochemical reaction network. This model is helpful in explaining the interconversion of biomolecules purely in terms of a number of reactants and products in a biochemical reaction. The generation of stoichiometric models and analysis of their properties is a well-established process [75–77]. Genome-scale models of metabolism have been completed for a diverse range of organisms, including prokaryotes and eukaryotes [75, 78]. Among these, the most important is the reconstruction of human metabolism at the genome scale [79, 80]. Additionally, methods have been developed for reconstructing signaling networks; transcriptional, translational networks; and regulatory networks [79–82]. These models are analogous to reconstructed metabolic networks [11]. The reconstruction of stoichiometric equations can be represented mathematically to form the foundation of a genome-scale computable model [11]. Computational tools have been used to interrogate the properties of reconstructed network in silico and to facilitate the model-driven validation and refinement [83]. Generally, a stoichiometric network operates under the application of physicochemical and environmental constraints in the form of balances such as mass, energy, charge and bounds (flux capacities), and thermodynamic constraints [11]. The statement of constraints defines a solution space which comprises all of the nonexcluded network states, thereby describing possible functions or allowable phenotypes.
Constraint-based analysis of biochemical reaction network has been applied to a number of human systems. Using the reconstruction of human mitochondrial metabolic network, linear programming and random sampling have been applied to identify candidate steady states of the network under normal, diabetic, ischemic, and dietetic conditions [84]. In a study, the Monte Carlo sampling of flux spaces was used to study the enzymopathies on a human erythrocyte metabolic network [83]. The completion of a global reconstruction of the human metabolic network represents a significant milestone in systems biology. This is comprised of 1,496 genes and 3,798 reactions divided into 88 metabolic pathways and paves the path for reconstruction of metabolic models of all 200 cell types in the human body and their modified forms in various types of cancers.
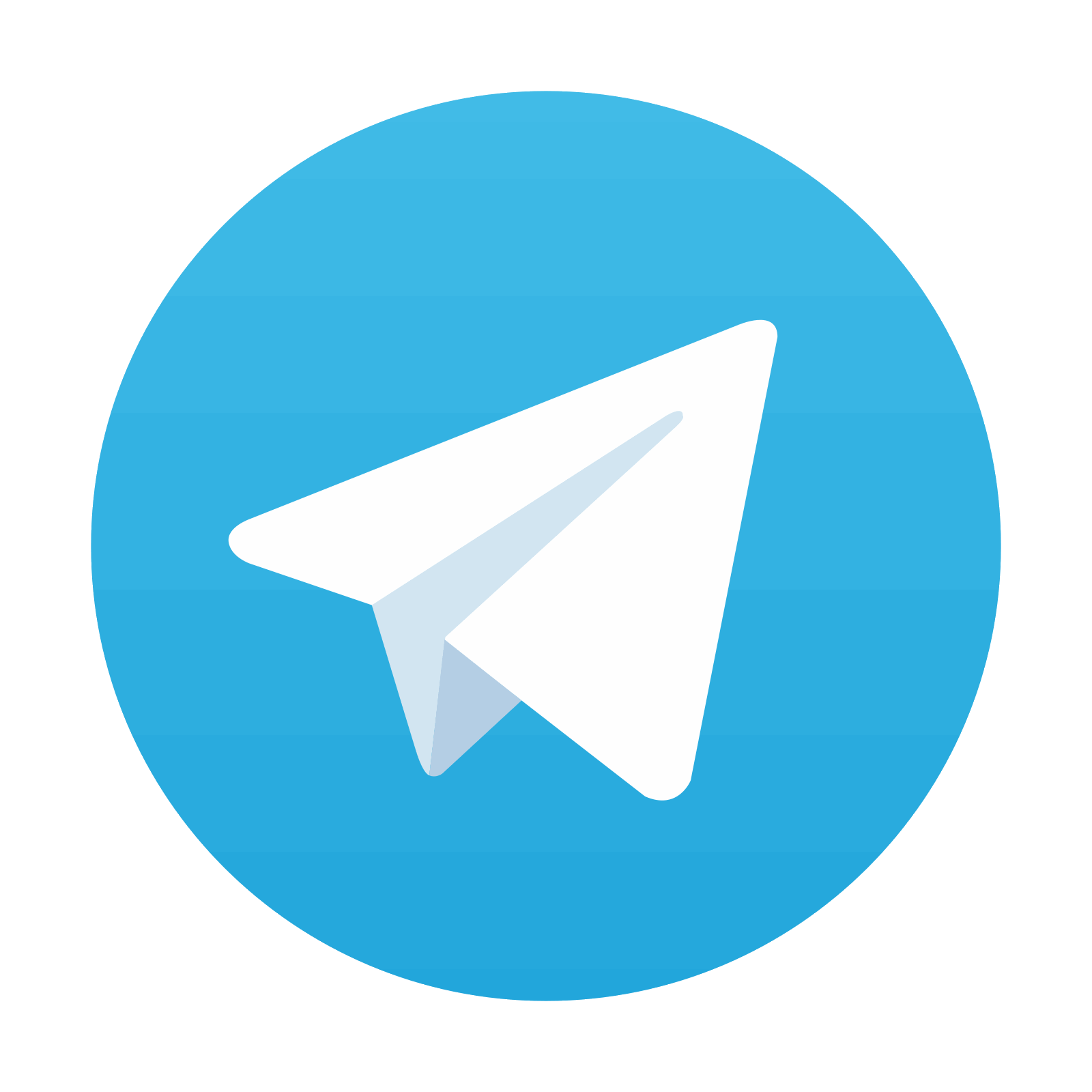
Stay updated, free articles. Join our Telegram channel

Full access? Get Clinical Tree
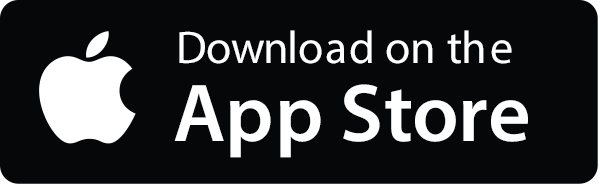
