Fig. 10.1
Factors affecting breast cancer prognosis. (a) Characteristics of the tumor will affect likelihood of recurrence and metastasis, and will also determine treatments to be given. (b) The effects of treatments on outcomes may be modified by genomic variation in drug metabolism and other pathways, as well as epigenetic silencing our activation of important pathways. (c) Treatment outcomes may also be modified by numerous lifestyle factors, including physical activity, body size, and dietary factors
Breast Cancer Is Not One Disease
Over the last two decades, breast cancer research at the cellular and molecular level has allowed for better understanding of the extensive heterogeneity of breast cancer, with wide differences identified between tumors among populations, and also molecular heterogeneity within tumors. Molecular characterization of tumors has informed likely prognostic outcomes, and also led to targeted therapies. Tumor characteristics indicate treatments to be given, but efficacy, as well as side effects of treatment, may be affected by genetic, epigenetic, and non-genetic factors, as shown in Fig. 10.1.
Investigations of the estrogen receptor (ER) began in the early 1970s (McGuire 1975; Jensen 1975), and within less than a decade, the anti-estrogen, tamoxifen, was being used to treat ER positive breast cancer (Fisher et al. 1981). Discovery of the HER2/neu proto-oncogene in the 1980s and identification of its important role in breast cancer prognosis then led to development of trastuzumab, a monoclonal antibody directed against HER2 (Slamon and Pegram 2001), which has greatly improved survival for women with HER2 positive breast cancer (Baselga et al. 2006). For many years, testing for ER, progesterone receptor (PR) and HER2 has guided breast cancer treatments, in addition to standard chemotherapy regimens. Even with ER+ breast cancer, however, there are notable differences in treatment outcomes, and additional molecular tests, such as Oncotype Dx recurrence score, have been used to further stratify patients for adjuvant chemotherapy treatments (Paik et al. 2004).
Finer classifications of breast cancer subtypes were identified with the advent of multi-gene arrays and expression analyses (van de Vijver et al. 2002; Perou et al. 2000), and the intrinsic subtypes have been shown to be associated with breast cancer prognosis (Carey et al. 2006). Importantly, classifications obtained using these arrays can be approximated using immunohistochemical (IHC) markers to classify ER+ breast cancer into Luminal A and Luminal B, with the latter having more proliferative indices and associated with poorer prognosis than Luminal A. Further refinement of ‘triple negative’ breast cancer (ER−, PR−, HER2−) into basal-like cancers (ER−, PR−, HER−, ck5/6+, EGFR+) with poorer prognosis is also possible using IHC markers. More recently, the PAM50 assay, which can now be performed using formalin-fixed paraffin-embedded tissue, builds upon classifications based on IHC markers, with reassignment of a fair proportion of tumors to other intrinsic subtype groups based upon the more detailed analyses, further refining prognostic estimates (Nielsen et al. 2010; Cheang et al. 2012; Caan et al. 2014).
Recent research from the Molecular Taxonomy of Breast Cancer International Consortium (METABRIC) in the United Kingdom and Canada, and the NCI-led Tumor Cancer Genome Atlas (TCGA) Network has provided more comprehensive portraits of breast cancer, with classifications into subgroups. With fresh frozen tumor samples from more than 2,000 women with breast cancer, METABRIC examined copy number and gene expression in discovery and validation sets (Curtis et al. 2012). Analyzing paired DNA and RNA samples, they identified novel subgroups with different clinical outcomes, including a high-risk ER+ subgroup, and a group with better prognosis whose tumors had no copy number variants. Their work highlighted a limited number of gene regions that likely harbor ‘driver’ genes. The next step recommended by the authors is to follow up with sequencing efforts for mutational profiles, particularly in cancers with no copy number aberrations.
In the TCGA, breast tumor and germline samples were available from 825 patients, and were analyzed on a number of platforms for assessment of copy number variants, DNA methylation, exome sequencing, messenger RNA arrays, and analysis of microRNAs and proteins (Cancer Genome Atlas Network 2012). Combining data from several platforms, tumors were classified into four main classes, although there was extensive heterogeneity within these groups. In fact, mutations were more diverse and recurrent in luminal A and luminal B tumors than within basal-like and HER2 enriched. The most frequently mutated genes in luminal A tumors were PIK3CA (45 %) and others including MAP2K4, which was also identified as a key cancer gene in the METABRIC analysis (Curtis et al. 2012). In basal-like tumors, 80 % of the cases had p53 mutations. These projects have provided a wealth of data and information which helps to elucidate breast cancer subtypes and may also provide clues for therapeutic targets to be followed. The TCGA study also showed a number of similarities between basal-like tumors and serous ovarian cancer, suggesting common therapeutic approaches.
This advanced molecular work using a number of platforms supports the initial classification of the intrinsic breast cancer subgroups, but also points to the significant heterogeneity within classes. Further research along these lines will hopefully not only inform our understanding of etiologic pathways, but perhaps lend guidance for development of targeted therapeutics. For cancer prevention and control, however, it is unclear how fine-grained classification of tumors needs to be to categorize for studies of lifestyle or other interventions. There are a growing number of studies showing, for example, that PAM50 classifications better predict treatment outcomes in breast cancer patients than IHC subgroups alone. Would this more refined classification also better inform studies evaluating the effects of, for example, physical activity and recurrence? These are studies that will likely need to be done to be able to best target those most likely to benefit from interventions.
Does Genetic Make-up Influence Recurrence and Survival?
For many years, scientists focused on characteristics of breast tumors as predictors of cancer outcomes. Although there had been awareness of the concept of pharmacogenetics, genetic variability in drug metabolism, from before the 1950s, it was primarily in relation to adverse outcomes for subsets of the population when being treated with drugs, such as isoniazid (Weber and Cohen 1968). It is only within the last two decades that there has been growing interest in the role of genetic variability in relation to breast cancer treatment outcomes. Initial studies investigating the role of genetic variability in relation to treatment outcomes focused on specific drug regimens and their metabolic pathways, taking a candidate gene approach in pathways for cyclophosphamide, anthracyclines and taxanes, with few consistent results [reviewed in Yao et al. (2012); Westbrook and Stearns (2013)].
The anti-estrogen tamoxifen is metabolized by cytochrome p450 enzymes, with CYP2D6 primarily investigated because it produces endoxifen, considered to be the primary activity metabolite responsible for the anti-estrogen activity of tamoxifen (Hoskins et al. 2009). Initially, there was enthusiastic interest and recommendations for genotyping for CYP2D6 variants in the clinic to predict drug efficacy and side effects, with the goal to be able to titrate doses based upon genotypes to enhance efficacy while reducing adverse outcomes, or for selection of alternate agents. This may be particularly important when using tamoxifen in a prevention setting among high risk patients. However, numerous studies have resulted in inconsistent results, leading to some controversy. In commenting on results from two trials showing null results, the accompanying editorial stated that “this matter has likely been laid to rest” (Kelly and Pritchard 2012), but other researchers believe that the lack of replication may be due to a number of methodological issues, including use of tumor DNA and problems with genotyping (Pharoah et al. 2012), as well as potential confounding by use of other medications that may induce or inhibit CYP2D6, or other differences in study populations (Stearns et al. 2003). In particular, a number of serotonin reuptake inhibitors (SSRIs) and norepinephrine reuptake inhibitors (SNRIs) used to treat depression and hot flashes also inhibit CYP2D6 activity (Jin et al. 2005; Borges et al. 2006). In breast cancer patients, CYP2D6 inhibition by co-medication with these drugs results in lower levels of the active metabolite endoxifen and potentially, reduction of efficacy of treatment with tamoxifen (Goetz et al. 2007). Although there is drug label information regarding the potential for drug interactions between tamoxifen and SSRIs and SNRIs, it is likely that these medications are still prescribed together, and may contribute, in part, to the inconsistency in study results regarding CYP2D6 genotypes, tamoxifen, and breast cancer outcomes (Binkhorst et al. 2013).
This example (tamoxifen and CYP2D6) illustrates the challenges faced when hoping to apply pharmacogenetics in a clinical setting to prediction of treatment outcomes. Here was a drug where there was bountiful data showing the functional activity of genotype variants and direct associations between genotypes and levels of the active metabolite. It seemed relatively straightforward to then extrapolate that there would be associations between genotypes and tamoxifen-related side effects as well as outcomes. However, results have been disappointing, perhaps due to the complexity of drug metabolic pathways, interactions between tamoxifen and CYP2D6 inhibitors, issues with study design, and heterogeneity of study populations.
Although investigations of drug metabolism pathways and breast cancer outcomes have not yielded definitive results with direct relevance for the clinic, there has been great anticipation for the use of genome wide association studies (GWAS) to identify key genes and/or gene variants that are associated with treatment side effects and recurrence and survival. Using an agnostic approach, genomic DNA from patients is genotyped for thousands of single nucleotide polymorphisms (SNPs), and genomic profiles compared between patients with and without the outcomes of interest. It is clear that not all patients respond similarly to cancer therapy, and it is likely that genetic variability, in part, plays a role in these differential responses.
GWAS have been used with some success for identification of susceptibility loci for etiology of a number of cancers, many of which are multifactorial and likely caused by numerous exposures and genetic variants. Thus, the thinking has been that genetic factors likely play a large role in differences in experience of grade 3 and 4 toxicities among patients receiving specific chemotherapy agents. Unlike using a candidate gene approach, GWAS may reveal pathways involved in side effects and outcomes that may not have been previously hypothesized. For example, Ingle and colleagues performed a GWAS to identify SNPs that were associated with musculoskeletal adverse events (AEs) among breast cancer patients treated with aromatase inhibitors (AIs) (Ingle et al. 2010). The SNPs identified were near the T-cell leukemia 1A (TCL1A) gene; follow-up with functional studies revealed effects of differential TCL1A expression on cytokine receptor genes and on transcription of NF-kB, indicating that the musculoskeletal AEs are related to an inflammatory response (Liu et al. 2012). Genetic variability in this pathway had not been previously hypothesized to be associated with AI-related side effects, and opens doors for new approaches for prevention of these AEs.
GWAS has also been used to investigate the basis for neurotoxicities often experienced among breast cancer patients treated with taxanes. In a Cancer and Leukemia Group B clinical trial, the loci identified that were associated with paclitaxel-induced neuropathy were in genes involved in axon outgrowth (Baldwin et al. 2012; Chhibber et al. 2014). Although, in retrospect, these findings make sense, they provide a new perspective or paradigm for pharmacogenetics. Previously, pathways that could modify the treatment agent effects were investigated, such as drug activation, detoxification, and DNA repair. These findings regarding neurotoxicity and SNPs in genes in axonal outgrowth, as well as the finding for AIs and musculoskeletal AEs, illustrate the value of GWAS in relation to treatment outcomes. By revealing pathways that were previously not hypothesized, new targets can be identified for prevention efforts.
The Interface Between Genomics and the Environment
Genomic DNA sequences are inherited, are in every cell in the human body, and account for a large portion of variations in phenotypes and conditions. Unlike DNA sequences, however, epigenetics, defined as covalent modifications of DNA base and chromatic alterations, affect DNA function without altering sequence. The most commonly studied epigenetic mechanism is DNA methylation, which is known for its plasticity, and is influenced by genetics as well as external exposures (Bernstein et al. 2007). DNA methylation is tissue specific, undergoing dynamic changes and influenced by factors such as aging, smoking, alcohol, and dietary intake (Rakyan et al. 2011; Langevin and Kelsey 2013; Langevin et al. 2011). There have been a number of studies of DNA methylation in breast tumors in relation to breast cancer characteristics, risk factors and prognosis, the majority of which have been performed targeting genes known to be commonly mutated in breast cancer, such as p16, ER, cyclin D2, RASSF1A, TWIST, RATB and HiN1 (Fackler et al. 2004; Swift-Scanlan et al. 2011; Tao et al. 2009). More recently, platforms have been used to examine thousands of loci for methylation. In the Sister Study, using a 27K platform, 250 differentially methylated loci were identified from blood samples that distinguished women who later developed breast cancer from those who did not (Xu et al. 2013). Using the same platform, Fackler and colleagues found that DNA methylation classified tumors into ER positive and ER negative, with 100 methylated loci significantly associated with disease progression (Fackler et al. 2011). We recently used the Illumina 450K platform and DNA from African-American and European American women, and found that methylation classified tumors according to ER status (Ambrosone et al. 2014), similar to Fackler et al. We also noted that there were more differentially methylated loci by race among women with ER negative breast cancer than those with ER positive disease, suggesting that etiologic pathways of ER negative breast cancer could differ between racial groups (Ambrosone et al. 2014).
With the refinement of approaches to conducting Epi-Genome Wide Associations Studies (EWAS), it is likely that this biomarker, which incorporates genetic and biologic factors with environmental exposures, will be used increasingly to establish risk prediction models and to identify genes that impact the disease process when altered through methylation. Although still in the early stages, there are also groups who are studying changes in methylation over time with cancer therapies and in response to interventions. Because methylation has been shown to differ between current, ex- and never smokers (Harlid et al. 2014), as well as between obese and non-obese individuals (Almén et al. 2014), there are hopes that it may eventually be used to monitor efficacy of interventions to reduce risk of breast cancer recurrence. In fact, in a 6 months exercise intervention, DNA from adipose tissue showed distinct methylation differences before and after the exercise intervention (Rönn et al. 2013). Of interest, the exercise intervention resulted in differential methylation of 39 candidate genes for obesity and type 2 diabetes. Thus, this marker holds promise for prognostic studies among breast cancer survivors, particularly to monitor efficacy of interventions.
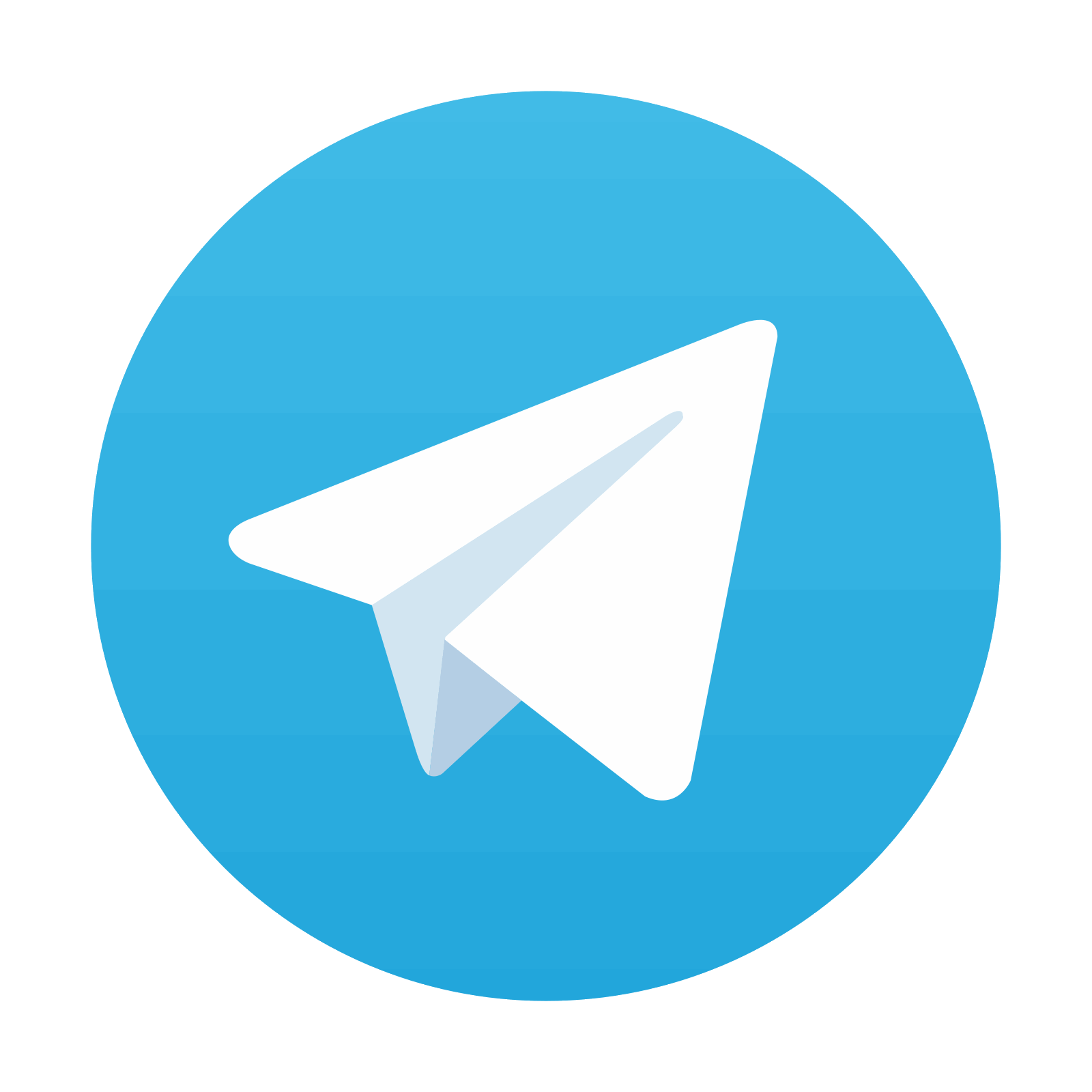
Stay updated, free articles. Join our Telegram channel

Full access? Get Clinical Tree
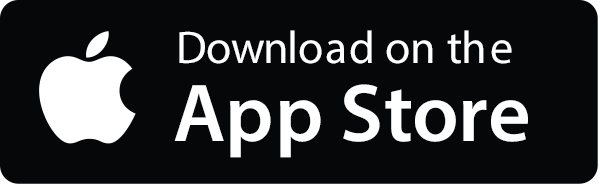
