Highlights
- •
Patients undergoing partial nephrectomy with multiple comorbidities face a higher risk of postoperative acute kidney injury (AKI).
- •
The risk of AKI escalates with increased warm ischemia time, particularly in patients with 3 or more comorbidities.
- •
Our study found that AKI and comorbidity-based risk groups not only predicted AKI but also correlated with poorer long-term functional outcomes.
- •
Preoperative counseling is crucial for patients with comorbidities, especially when complex surgeries when a prolonged ischemia is foreseen.
Abstract
Background
The impact of warm ischemia time (WIT) on renal function after partial nephrectomy (PN) remains debated. This study investigates the effect of WIT on the relationship between preoperative comorbidities and postoperative renal function impairment in renal cell carcinoma (RCC) patients.
Methods
Patients undergoing PN for T1 RCC at a European high-volume center (2000–2023) were analyzed. Logistic regressions assessed the association between patient comorbidities and acute kidney injury (AKI). Patients were stratified into low (LR), intermediate (IR), and high-risk (HR) groups based on a weighted comorbidity score derived from odds-ratio obtained from the logistic regression analysis. Interaction terms and a weighted local polynomial smoother function assessed the impact of WIT on AKI. Cox regressions and cumulative incidence were used to assess the chronic kidney disease (CKD) upstage ≥IIIB risk according to AKI and risk groups.
Results
Of 1,048 patients, 802 underwent PN with warm ischemia. Among these, 339(42%), 208(26%), 255(32%) were classified as LR, IR and HR. IR (OR:1.82, P = 0.018) and HR (OR:3.01, P < 0.001) patients had a higher AKI risk compared to LR. The increase in WIT had little impact on the LR AKI probability compared to IR (OR:1.06, P = 0.001) and HR (OR:1.08, P < 0.001). The 10-year risk of CKD-upstage ≥IIIB was higher (36% vs. 12%, HR:2.40, P = 0.004) after AKI, and in the HR group (HR:2.42, P = 0.008)
Conclusions
WIT predominantly affected the risk of AKI in HR patients for renal function impairment after surgery. Preoperative counseling is essential for comorbid patients, especially when planning complex surgeries with prolonged ischemia, to mitigate AKI and long-term renal impairment.
Graphical abstract
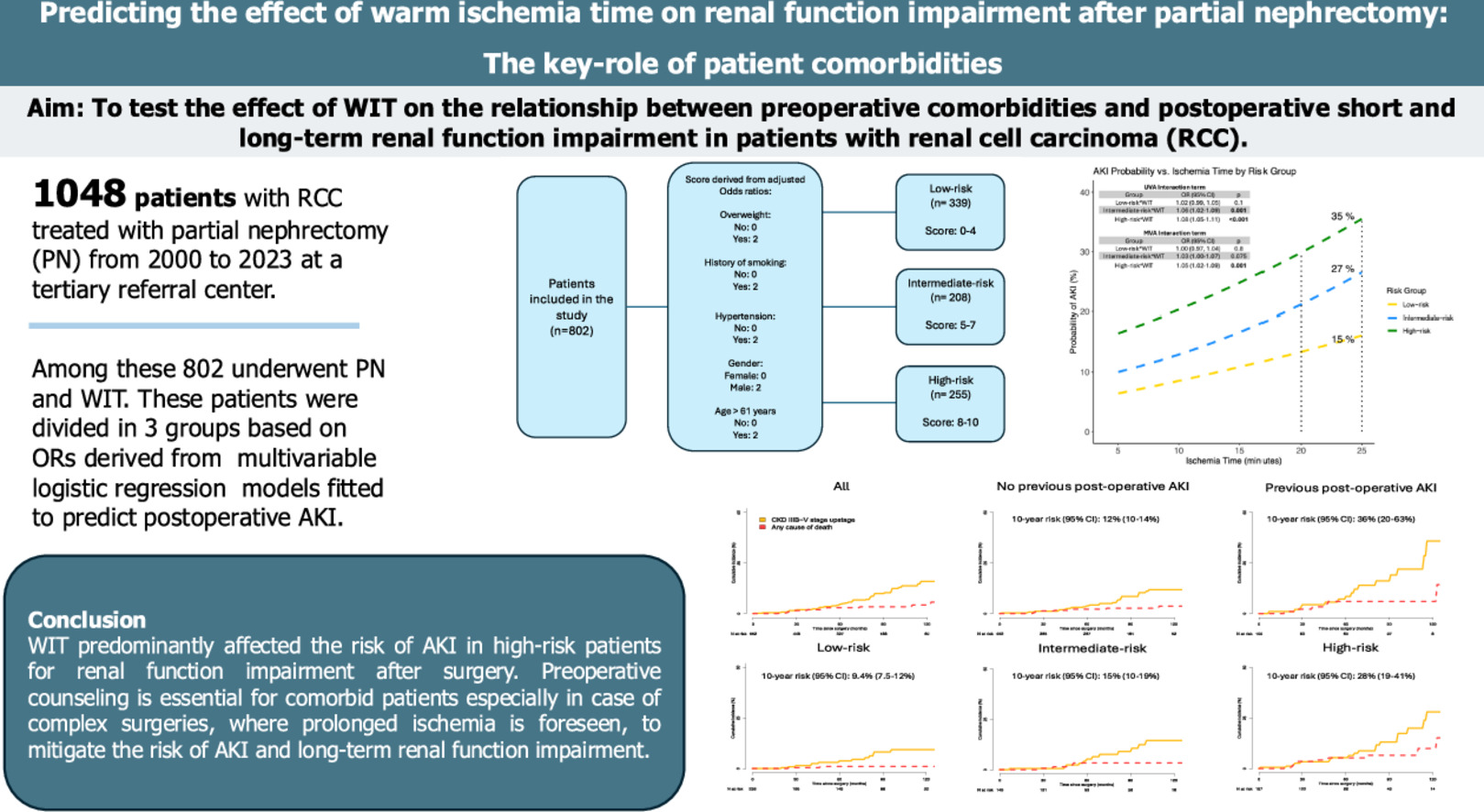
1
Introduction
Partial nephrectomy (PN) represents the gold standard treatment for patients diagnosed with cT1 renal cell carcinoma (RCC), when technically feasible [ ]. Specifically, nephron-sparing surgery shows comparable oncologic outcomes, relative to radical surgery and mitigates the risk of long-term renal function impairment [ , ].
While previous studies examined the effect of several clamping techniques, such as off clamp vs. selective clamping vs. super-selective clamping on postoperative renal function [ ], controversial findings have been shown regarding the impact of WIT on acute kidney injury (AKI) after PN [ ]. Existing evidence advocates for limiting WIT to 20 minutes to minimize renal function damage. Nonetheless, conflicting studies indicate that up to 30 minutes of WIT might be tolerated before showing a significant increase in the risk of AKI [ ].
Previous studies investigated the role of several comorbidities and other peri-operative risk factors on the risk of long-term renal function impairment after kidney surgery. However, no data exist regarding the association between highly prevalent comorbidities (e.g., hypertension, being obese/overweight, smoking cigarettes, hypercholesterolemia, diabetes) and the interaction of these comorbidities with WIT in predicting the risk of short- and long-term renal function impairment. More specifically, smoking cigarettes, hypertension, body mass index or diabetes can correlate with baseline vascular deterioration and may potentially vary the effect of WIT on renal function after kidney surgery [ ].
To fill this void, this study aimed to evaluate how WIT affects both short- and long-term renal function in patients with preoperative comorbidities undergoing PN at a high-volume center.
2
Materials and methods
2.1
Study population
After institutional board approval, our study relied on a prospectively maintained database including 4,019 patients who underwent renal surgery between 1998 and 2023. We specifically focused on patients who underwent open, laparoscopic, or robot-assisted PN for cT1 RCC performed at our center, from 2000 to 2023. Exclusion criteria and the full patient selection process are shown in Supplementary Figure 1 . The final cohort after exclusion was of 1,048 eligible patients.
2.2
Variables definition
Patients were stratified and evaluated based on the presence of preoperative risk factors, which were defined considering features that could potentially determine a baseline vascular damage and renal function impairment, according to previous existing evidence [ , , ]. Specifically, smoking was categorized as a risk factor if patients were either current smokers at the time of surgery or former smokers. Additionally, vascular hypertension and overweight/obesity (Body Mass Index [BMI] ≥ 25 Kg/m 2 ), diabetes, hypercholesterolemia (LDL > 100 mg/dl or HDL < 50 mg/dl), gender (male or female) and age were identified as other potential risk factors [ , ]. Renal function was assessed using the estimated glomerular filtration rate (eGFR), calculated utilizing the CKD-EPI formula. AKI was defined based on the RIFLE criteria (Risk, Injury, Failure, Loss, and End-stage kidney disease).
Chronic kidney disease (CKD)-upstage ≥IIIB was defined as a measurement of eGFR below < 45 ml/min/1.73 m 2 for patients with a baseline eGFR ≥ 45 ml/min/1.73 m 2 .
2.3
Statistical analysis
Main clinical characteristics were summarized using medians, and interquartile ranges (IQR) for continuously coded variables.
To conduct the main analysis the following steps were followed:
- 1.
Univariable and multivariable logistic regression models were employed to evaluate the association between AKI and various preoperative comorbidities. Given that each comorbidity could contributed differently to the risk of AKI, the clinically significant predictors were selected and fitted into multiple multivariable logistic regression models. These models were adjusted for surgical approach and experience (≥ 30 surgeries), operative time, estimated blood loss, tumor size, postoperative hemoglobin, and baseline eGFR.
- 2.
The adjusted odds ratios (ORs) for the significant predictors from the multivariable analyses were then normalized and scaled proportionally using the highest OR as a reference and then scaled from 0–10 points. This allowed for the creation of a weighted scoring system, where each clinically significant factor was assigned a specific score according to its relative impact on AKI risk.
- 3.
Based on the cumulative risk score, patients were categorized into 3 risk groups: low-risk, intermediate-risk, and high-risk for developing AKI using the Q1 and Q3 of the score distribution. ( Fig. 1 )
Fig. 1
Grouping system process based on Odds-ratios derived score accounting for multiple comorbidities.
- 4.
To further explore the relationship between AKI risk and ischemia time across these risk groups, 3 separated univariable logistic regression analyses were conducted. A local polynomial smoother was applied to visually assess the risk of AKI according to the risk group and ischemia time. A univariable and multivariable (adjusted for the above mentioned variables) interaction term between ischemia time and risk groups was included to evaluate how ischemia time affected the risk of AKI across the risk categories.
Finally, cumulative incidence curves, using death from any cause as a competing event, alongside univariable and multivariable Cox regression analyses (censoring death), were used to assess the risk of CKD-upstage ≥ IIIB based on the occurrence of postoperative AKI and risk groups.
For all statistical analyses, R software environment for statistical computing and graphics (version 4.3.3) was used. All tests were 2 sided with a level of significance set at P < 0.05.
3
Results
3.1
Descriptive characteristics of the study population
Among 1,048 patients, 802 (77%) underwent warm ischemia. Minimally-invasive PN was performed in 352 (34%) vs. 696 (66%) patients undergoing open surgery. Baseline characteristics of the overall population are included in Table 1 .
Characteristic | All (N(%)) |
---|---|
Total | 1,048 (100) |
Age, years | |
Median [Interquartile range (IQR)] | 62 (52–70) |
Gender | |
Male | 726 (69) |
Female | 322 (31) |
Preoperative eGFR, ml/min | |
Median (IQR) | 86 (67–98) |
Size, cm | |
Median (IQR) | 3.00 (2.20–4.00) |
Hypertension | 536 (51) |
Smoker (former or current) | 557 (53) |
Diabetes | 146 (14) |
Body mass index, kg/m 2 | |
Median (IQR) | 25.7 (23.3–28.1) |
Overweight (≥ 25 Kg/m 2 ) | 597 (57) |
Hypercholesterolemia | 209 (20) |
Estimated blood loss, mL | |
Median (IQR) | 295 (100–500) |
Operative time, min | |
Median (IQR) | 160 (124–195) |
Ischemia time, min | |
Median (IQR) | 15 (8–20) |
Surgical approach | |
Open | 696 (66) |
Minimally-invasive | 352 (34) |
Ischemia type | |
No ischemia | 234 (22) |
Cold ischemia | 12 (1.1) |
Warm ischemia | 802 (77) |
Of patients undergoing warm ischemia, the median age at surgery was 61 years (IQR: 51–70 – Supplementary Table 1 ). Of the entire cohort, 53% of patients (n=429) were either smokers at the time of surgery or former smokers. Hypertension was observed in 390 patients (49%), 453 (56%) were classified as overweight/obese, 109 (13%) had diabetes and 154 (19%) had hypercholesterolemia. The median warm ischemia time was 17 minutes (IQR: 13–21).
3.2
Risk of AKI according to risk group
Among patient comorbidities, being obese/overweight [OR: 1.76, 95% Confidence interval (CI), 1.20–2.62, P = 0.004], history of smoking (OR: 1.54, 95% CI, 1.07–2.24, P = 0.023), hypertension (adjusted OR: 1.55, 95% CI, 1.04–2.33, P = 0.034), being male (OR: 1.60, 95% CI, 1.04–2.52, P = 0.036), and older age (> 61 years; OR: 1.58, 95% CI, 1.01–2.49, P = 0.048) emerged as significant predictors of AKI. ( Table 2 ) Of note patients who experience AKI has similar baseline eGFR to those who did not (86 ml/min/1.73 m², IQR: 66–98 vs. 86 ml/min/1.73 m², IQR: 67–96).
Variables | Univariable analysis | Multivariable analysis | ||||
---|---|---|---|---|---|---|
OR | 95% CI | p | OR | 95% CI | p | |
Overweight (Body Mass Index ≥ 25 Kg/m 2 ) | ||||||
No | Ref | – | – | Ref | – | – |
Yes | 1.80 | 1.25, 2.63 | 0.002 | 1.76 | 1.20, 2.62 | 0.004 |
History of smoking | ||||||
No | Ref | – | – | Ref | – | – |
Yes | 1.64 | 1.15, 2.37 | 0.007 | 1.54 | 1.07, 2.24 | 0.023 |
Diabetes | ||||||
No | Ref | – | – | |||
Yes | 0.98 | 0.58, 1.60 | >0.9 | |||
Hypertension | ||||||
No | Ref | – | – | Ref | – | – |
Yes | 1.65 | 1.16, 2.37 | 0.005 | 1.55 | 1.04, 2.33 | 0.034 |
Hypercholesterolemia | ||||||
No | Ref | – | – | |||
Yes | 1.18 | 0.75, 1.80 | 0.5 | |||
Gender | ||||||
Female | Ref | – | – | Ref | – | – |
Male | 1.57 | 1.05, 2.37 | 0.030 | 1.60 | 1.04, 2.52 | 0.036 |
Age > 61 years | ||||||
No | Ref | – | – | Ref | – | – |
Yes | 1.52 | 1.07, 2.18 | 0.020 | 1.58 | 1.01, 2.49 | 0.048 |
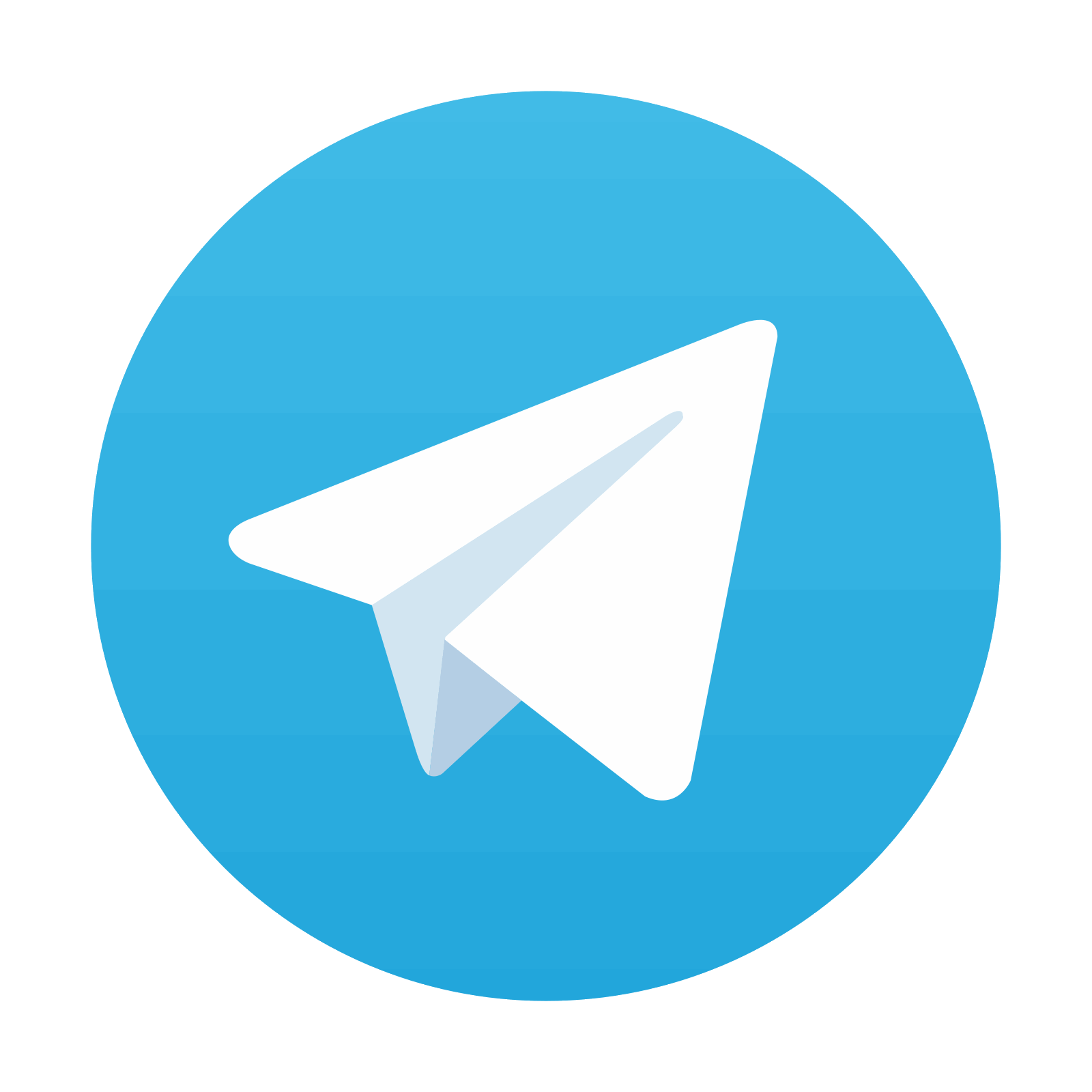
Stay updated, free articles. Join our Telegram channel

Full access? Get Clinical Tree
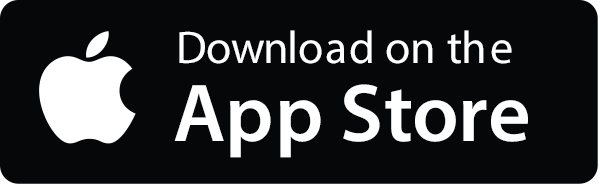

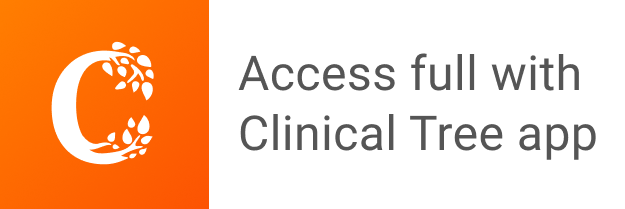