7 Electronically Supported Outcome Measurement
Introduction
In the context of the current chapter, the technical term “outcome measurement” refers to studying the outcome of any kind of medical measures. Sensible outcome measurement is not limited to studying the efficacy of medical measures alone, but focuses very specifically on the benefit achieved. As patients search medical advice in order to enjoy either longer or better lives, any medical measure is beneficial only as far as it helps them achieve these goals. With steadily expanding possibilities, options, and costs in medicine, asking for what is actually beneficial for patients becomes more important than ever.
In this respect, the assessment of patients’ subjectively perceived quality of life (QoL) has shown to be a valuable tool. This approach may be of interest for small studies and large multicenter efforts alike. In the setting of routine care-based research, however, regular measurement of quality of life is practised merely at a minority of places. This might be due to traditional attitudes of the scientific community, which still prefers “objective” physiological parameters to “subjective” questionnaire results. Although comprehensive scientific sources and validated, practical instruments exist, substantial knowledge of methodology, the significance of results and financial resources may still be deficient. Practical obstacles, in any case, may be overcome by modern tools, and any insight retrieved by their application is indispensable for medical decision making, individual evaluation, and optimization of therapy and quality assurance.
Necessary investments are relatively small compared to other fields of medical science: there are limited monetary expenses for technical equipment and acquisition of know-how, and time must be provided for training, data collection, and analysis and discussion of results. Some non-technical local conditions may merely demand creativity. Just like in any other research or quality assurance project, involved staff have to identify and to exploit available sources of know-how, and to understand and avoid possible sources of bias. This can be facilitated by applying methods from the discipline of evidence-based medicine.
Basic Methodological Considerations
Evidence-Based Medicine
A study design must fulfill certain requirements in order to avoid results which may be biased or misleading.
For several years, the movement of evidence-based medicine has propagated the application of the best available knowledge in the care for each individual patient, at the same time providing methods enabling every medical professional to locate up-to-date knowledge and to identify possible sources of bias in scientific publications.
Whoever uses these methods regularly can also learn to avoid bias in their own studies, and may probably practise reliable documentation and explorative analysis of routine data before calling for another randomized, double blind trial for each upcoming question (10, 11).
Efficacy and Benefit
Occasionally, patients may ask their physician whether a certain measure may actually help. Medical professionals, economists, and politicians do routinely ask whether a certain measure is efficacious (and maybe efficient)–with demonstrated efficacy (or efficiency) they take the resulting benefit for granted. As a consequence, efficacy (and maybe efficiency) has been studied and demonstrated for a variety of measures, but actual achievable benefit has not.
A patient with hay fever, for example, may feel immediate relief of symptoms when using a certain drug. However, the same drug may make the patient so tired that its actual benefit is questionable.
The amount of benefit that a measure can achieve may also depend upon how reliably it is targeted to adequately selected patients: Thus a drug that may lower blood pressure in any given patient by 10–20mmHg is effective in each of them. One death due to a stroke or heart attack may be avoided by giving this treatment to three patients with a diastolic blood pressure of 115–129mmHg for 1.5 years. If the same approach, however, is applied to patients with a diastolic blood pressure of 90–109mmHg, the same result requires treatment of 128 patients over 5.5 years (10).
Prolonged Survival, Historical Controls, Screening
The duration of survival achieved under a recently introduced treatment may be compared to the duration of survival once achieved in historical controls. For example, a study of a new therapy for women with stage II breast cancer (free lymph nodes) might show a five-year survival rate of 78 %, whereas a study of conventional therapy, initiated 12 years ago, resulted in a five-year survival rate of only 65 %.
The second study started seven years after the first one and, in this time, ultrasound and computed tomography (CT) scanning resolution may have improved. Specifically, more advanced devices may have shown some suspicious lymph node, which might have been overlooked using the older ones. Thus, some patients may have been included in the stage II group 12 years ago, while patients with exactly the same level of disease, but examined with better diagnostic technology, would have been included in the stage III group five years ago.
In this scenario, baseline risks of both resulting stage II groups are not comparable at all, and advances in the diagnostic, not therapeutic, field of medicine might cause the observed survival gain. To avoid this stage migration bias, experimental and control groups should be studied at the same time, and historical controls should be avoided.
In another example, a study among women diagnosed with breast cancer might find one group who attended regular screening examinations with an average survival time of eight years, and another group without screening and an average survival time of five years. Apparently, screening prolonged survival by three years. This conclusion, however, is invalid: It is generally expected that screening might contribute to the discovery of lethal diseases in an earlier stage, and thereby improve the odds for curative therapy. However, screening might just as well discover the same lethal disease earlier, while it may still be impossible to change the course of the disease in any way. As efficacious screening delivers the diagnosis earlier than clinical symptoms would have done, no matter whether the course of the discovered disease can be changed or not, any introduction of screening will result in longer times between diagnosis and death. Time is absolutely certainly added to the observed interval at its beginning (lead time bias), but not necessarily at its end, where we would hope for additional survival. This is especially important as the diagnosis of a malignant disease may itself affect a patient’s quality of life.
To avoid this kind of bias, survival time should not be measured from the point in time of primary diagnosis, when studied measures affect this point by themselves. Instead, subjects must be randomized into experimental and control groups before any of the studied events take place. As a consequence, large patient populations must be observed for long periods to obtain unbiased knowledge on the effects of screening.
To assess the benefits of breast cancer screening, such trials have actually been conducted. When their results are discussed, the toolchest of evidence-based medicine should be used: In the age group 50–69 years, let us assume a single woman’s risk of dying due to breast cancer is 0.345 % or 0.00345 (control group event rate). Let us assume annual screening is able to reduce this risk to 0.252 % or 0.00252 (experimental group event rate). Politicians or business representatives might praise such a result as a “reduction by 27 %,” or “almost one third” (Relative Risk Reduction, RRR), and rather not quote the less impressive absolute difference of 0.093 percent points or 0.00093 (Absolute Risk Reduction, ARR). The reciprocal value of that, 1/0.00093, equals 1075, and this is the number of women who would need to be screened (Number Needed to Treat, NNT), over 10 years to avoid one death (4). Similar simple math can be used when considering how many women can be screened in the same period before one of them receives a false-positive result, is erroneously treated, and consequently harmed (Number Needed to Harm, NNH).
The last example illustrates that the true meaning of an impressive relative risk reduction depends upon the baseline risk (which, in the example of breast cancer, depends upon age), the quality of medical measures and their side-effects. Consequently, recent controverse discussion of breast-cancer screening found a demand for additional data (5, 7).
Spontaneous Course of Disease and Motivation
Some considerations support the assumption that our knowledge about the spontaneous course of many diseases must be limited: A patient experiencing an especially favorable course of a certain disease may possibly not want to see a physician at all. A patient with an especially unfavorable course may possibly not manage to see one in time-and the truly fatal disease may later be overlooked or misdiagnosed. For those patients already selected who actually see a physician, pressure resulting from patients’ presentation, expectations, their environment, or from the physician, may cause diagnostic and therapeutic measures by far exceeding anything warranted by scientific literature or professional experience. Also, the fact that activity rather than watchful waiting generates monetary and academic rewards may unfortunately influence treatment decisions and research designs alike.
Applicability of Study Results
Medical studies often enrol highly selected groups of patients, and they are conducted in specialized and well-equipped environments. As a consequence, the studied population and the studied intervention may both be different from everyday settings. Thus, even if a study fulfills every methodologic requirement, it remains difficult to estimate how well its results may apply to an individual case in routine patient care.
Routine Care-Based Research
The mechanisms and consequences outlined above lead to a severe lack of knowledge. This deficit may probably only be alleviated if data from routine care are routinely collected and analyzed. On the one hand, such measures may actively support the evolution of available services through continuous, feedback-controlled, quality improvement, and thus promote the best possible use of limited resources from the patients’ perspective. On the other hand, such measures must not place any additional burden on medical staff, who want to excel as medical professionals, not bookkeepers. The practicability of any attempt in this direction will consequently depend upon the use of modern information technology, which, however, needs to be guided by medical competence. Of course, both components must be adequately and reliably financed, according to their fundamental importance.
Quality of Life Measurement
Health-Related Quality of Life
Particularly in incurable diseases, prolongation of survival cannot be the only therapeutic objective. In some cases, patients and physicians may have to choose between the prospects of longer survival or better quality of life during the remaining time.
The importance of quality of life may become especially prominent in palliative care of patients with malignant diseases, or with painful diseases of the musculoskeletal system, where mitigation of symptoms may be sought; also in patients with cardiologic diseases, where treatment shall enhance physical fitness. Apart from being the primary goal of palliative measures, improved quality of life is also a byproduct of successful curative treatment.
Consequently, the term quality of life (QoL), has been used very often in recent years. Many treatments have been advertised as being “proven to enhance quality of life,” while closer examination might reveal that, e.g. only the Karnofsky Index has been used to assess the single dimension of physical fitness by proxy rating, or that freedom of movement has been measured for a single extremity in angular degrees.
The term health-related quality of life (HR–QoL), is usually derived from the WHO definition of health: “A state of complete physical, mental, and social well-being and not merely the absence of disease or infirmity” (28).
Advanced concepts discern multiple periods of time spent with different levels of quality of life, and blend quality and time into Quality-Adjusted Life Years (QUALY). Economists use data from reference populations to link certain medical conditions with respective levels of “utility” for patients, and try to optimize this measure. Whereas such blending of multiple dimensions of quality of life into simplified measures may be useful for specific tasks, this approach causes a loss of differentiated information for clinical users. To solve this dilemma, some questionnaires report results for individual dimensions as well as aggregated measures or global quality of life.
A study conducted among physicians from Finland, Austria, and Germany found that 30–40% were accustomed to the concept of quality of life, that 40–90 % accepted certain components of this concept, and that more than 90 % considered a common measure to rate the effect in clinical or economic studies as useful. The concept of “health-related quality of life” grown in medicine was more naturally accepted than the concept of economically coined “utility” (6).
Self-Assessment vs. Proxy Rating
Various studies have compared results of self-assessments with results of assessment by relatives, nurses, and physicians (proxy rating). They show that the estimation of external observers can differ substantially and systematically from the patient’s view (9).
Only patients know their own internal standards, to which they compare their current situation. An elderly lady, asked to rate her physical health, may answer: “Very good, doctor, I could climb two stairs without having to rest.” A 16-year-old boy, however, may answer: “Absolutely poor, doctor, 100 meters took me 12.4 seconds!”
Occasionally, subjective data are considered “soft data,” “less valid” or “less meaningful” than objective measures like blood pressure, tumor diameter, and so on. However, when filling in a validated questionnaire, patients transform their subjective experience into objectively measured data. The results actually belong to the hardest and most meaningful parameters available. On the one hand, a formal analysis must appreciate the fact that reduced quality of life is among the key motivations that make a patient seek medical treatment. Consequently, no parameter chosen as a mere replacement can reflect therapeutic success more accurately. On the other hand, some studies have shown that a baseline assessment of quality of life belongs to the most important prognostic parameters (9, 14).
Questionnaire Development
New QoL questionnaires (instruments) should be developed according to established, effective algorithms (16)
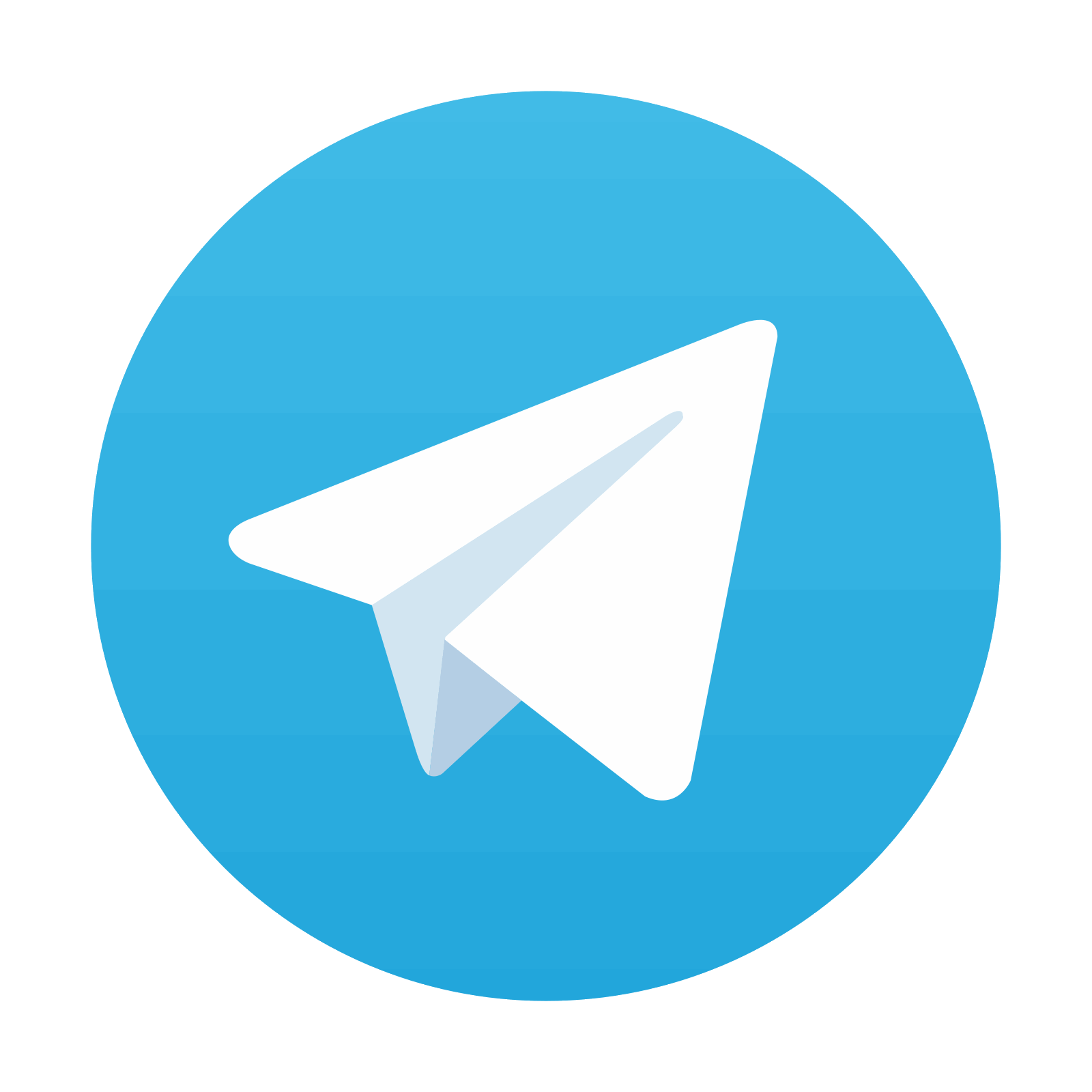
Stay updated, free articles. Join our Telegram channel

Full access? Get Clinical Tree
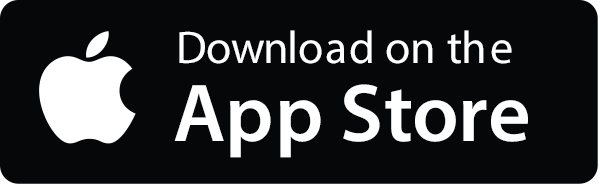
