Survivors of AYA cancer
No history of cancer
p-value
Adjusted expenditures [95 % CI]
Adjusted expenditures [95 % CI]
All sources of payment
$7,417 [6,133, 8,700]
$4,247 [4,142, 4,352]
<0.001
Source of payment
Out of pocket
$765 [684, 846]
$686 [670, 701]
0.044
Private health insurance
$3,083 [2,312, 3,854]
$1,825 [1,758, 1,892]
<0.001
Medicare
$1,246 [898, 1,594]
$948 [901, 996]
0.051
Medicaid
$541 [361, 721]
$380 [342, 418]
0.056
Other
$876 [578, 1,174]
$411 [387, 435]
<0.001
Service type
Ambulatory care
$2,409 [1,851, 2,968]
$1,376 [1,335, 1,417]
<0.001
Inpatient care
$1,605 [1,115, 2,096]
$1,169 [1,116, 1,221]
0.043
Prescription medications
$1,466 [1,241, 1,691]
$1,034 [970, 1,099]
<0.001
Other services
$820 [694, 946]
$686 [658, 714]
0.026
33.2.1 Nonmedical Costs of Care
Nonmedical costs of care for AYA cancer patients include monetary losses associated with lost productivity from premature death (known as mortality costs), time lost from work due to long-term cancer- or treatment-related morbidity (known as morbidity costs), and time spent receiving cancer treatment [14]. Mortality costs evaluate the potential dollar value lost to society when an individual dies prematurely based on their age and projected income. It is important to note that mortality cost estimates are highly dependent on the approach used to quantify lost productivity after death. In contrast, morbidity cost can be appreciated as lost productivity due to disease, treatment, or late effects. To calculate morbidity costs, absences from work can be assigned dollar figures based on projected income. Morbidity cost may also be influenced by diminished volume or quality of work and reduced participation in household or educational endeavors. Finally, patients are burdened by time costs which may be highly variable as they account for the time allocated to care.
33.3 Mortality Costs
Mortality costs assess the potential dollar value lost to society when an individual dies prematurely. There are two common approaches for assessing the economic value of mortality loss. The human capital approach gives annual age- and sex-specific earnings data for each year of life lost, representing a figure that reflects loss of projected income. This creates an approach that directly applies more weight to higher earning years of life. The willingness–to–pay (WTP) approach, in contrast, uses survey data that assesses how much individuals would be willing to pay for an extra year of life at different ages and health states [15, 16]. A third, less commonly used approach, is the friction cost method, which takes the employer’s (rather than the patient’s) perspective on lost productivity. Using this method, time is only counted as lost until another employee takes over the patient’s work [17]. These three methods can produce quite different results. The friction cost method will often result in a lower cost. The human capital and willingness-to-pay approaches will typically produce similar results in a young adult population, but tend to diverge (with the human capital approach producing lower costs) toward the end of adulthood [15]. Limited information exists on the mortality cost of AYA cancer.
33.4 Morbidity Costs
Cancer-related long-term morbidity can cause varying degrees of productivity loss. Also, depending on the type and severity of cancer-related morbidities, the economic implications may manifest in different ways. This logic justifies the broad array of existing metrics that economists may use to calculate the morbidity cost. While morbidity cost assessments are derived at least partially from estimates of lost work, morbidity cost analyses of wider breadth can also incorporate productivity loss in other realms, including education losses or inability to participate in household or routine practices.
33.4.1 Employment
There are several measures and indicators related to workplace productivity that can be useful in measuring the impact of AYA cancer and its treatment. At the simplest level, rates of employment and unemployment can be compared for survivors of cancer diagnosed in adolescence and young adulthood over time, relative to a population of individuals without a history of cancer. A meta-analysis of 36 studies found that cancer survivors were more likely than healthy control participants to be unemployed (33.8 % vs. 15.2 %; pooled relative risk [RR], 1.37; 95 % confidence interval [CI], 1.21–1.55) [18].
Employment metrics can also address alternate measures of productivity loss that may offer the benefit of increased sensitivity to detect otherwise unappreciable outcomes. A simple example of this is evaluating work intensity, either by examining the proportion of the employed population who work full time versus part time or calculating and comparing average hours worked per week [19]. Another commonly used measure is employment disability (being unable to work due to health limitations).
Some of the more granular metrics used to evaluate productivity loss in the workplace account for changes that are not outwardly reflected in employment status. A particularly useful example of these measures is absenteeism (missed time from work). Cancer has also been reported as a causative factor in presenteeism – working while disabled from a mental or physical illness [20]. The economic consequences of presenteeism have been reported as being even greater than those attributed to absenteeism [21]. In their review on the impact of cancer on employment, Steiner et al. also suggest examining changes to work role and content that may occur as a result of cancer and its treatment [19]. Metrics that have been used to assess this outcome include change in employer; change in work type, duties, or skill; and change in work productivity, among others.
In the most comprehensive empirical study on the health and economic burden of AYA cancer to date, Guy et al. (2014) measured morbidity costs among AYA cancer survivors [4]. Among survivors of AYA cancers, total annual per capita lost productivity was $4,564, compared with $2,314 among individuals without a history of cancer (Table 33.2). Productivity loss resulting from employment disability accounted for the largest portion of total productivity loss. Survivors of AYA cancers were more likely to report employment disability and an increased number of missed work days as a result of illness or injury.
Table 33.2
Adjusted per-person lost productivity among survivors of AYA cancer and individuals without a history of cancer, United States, 2008–2011
Survivors of AYA cancer | No history of cancer | p-value | |
---|---|---|---|
Adjusted productivity loss [95 % CI] | Adjusted productivity loss [95 % CI] | ||
Total per capita total productivity loss | $4,564 [3,740, 5,387] | $2,314 [2,196, 2,432] | <0.001 |
Employment disability | $3,645 [3,051, 4,238] | $1,828 [1,735, 1,921] | <0.001 |
Absenteeism | $500 [398, 603] | $329 [316, 342] | <0.001 |
Lost household productivity | $419 [291, 546] | $157 [145, 169] | <0.001 |
33.4.2 Education
Educational metrics can be valuable tools for examining the economic burden of AYA cancer, as they can be directly indicative of economic well-being. The most commonly used (and accessible) metrics in this class are educational enrollment and completion rates: rates of enrollment and completion of high school, college, university (including advanced degrees), and vocational and technical schooling. The literature to date reveals limited analyses of educational outcomes in patients afflicted by a cancer diagnosis between the ages of 18 and 39. Dieluweit et al. in a German study reported that survivors of adolescent cancers generally achieve higher educational and vocational levels than individuals who do not have a history of cancer; however, those survivors who have neurophysiological late effects are at heightened risk for failing to graduate from college [22]. There are several studies that have examined similar outcomes in childhood cancer survivors (e.g., [23, 24]). Most of these studies conclude that specific subsets of the childhood cancer population (typically those with tumors or treatment affecting the central nervous system) are at heightened risk for failing to graduate from high school and have lower rates of postsecondary school entrance and employment [24].
33.4.3 Other Morbidity Costs
Burdens faced by AYA patients are not isolated to educational and employment domains. Risk of acute or late effects of treatment and continued long-term surveillance can cause strain in other dimensions of daily living that may reduce economic potential. It is easy to conceptualize the ability of a cancer diagnosis in the AYA age range to yield a breadth of economic consequences, but again, literature is sparse to date. Guy et al. [4], referenced above, demonstrated that survivors of AYA cancers were more likely to report increased lost household productivity (inability to complete household work due to health limitations) when compared to individuals without a history of cancer. The increased loss of household productivity contributed significantly to total per capita productivity loss, along with employment disability and absenteeism (Table 33.2).
In the same study, Guy and colleagues examined a variety of measures to evaluate limitations among AYA cancer survivors relative to a population without a history of cancer. Measures included limitations in work, housework, or school, being completely unable to do activities (work at a job, do housework, or go to school), cognitive limitations, and limitations in physical functioning [4]. Survivors of AYA cancers were less likely to be employed and more likely to report limitations in work, housework, or school, cognitive limitations, limitations in physical functioning, and being completely unable to do activities compared to individuals without a history of cancer (Table 33.3). Among individuals not working, survivors of AYA cancers were more likely to report being unable to work because of illness or disability.
Table 33.3
Employment and functional limitations of survivors of AYA cancer and individuals without a history of cancer, United States, 2008–2011
Survivors of AYA cancer | No history of cancer | p-value | |
---|---|---|---|
% | % | ||
Employment | |||
Not employed | 33.4 | 27.4 | <0.001 |
Reason for not working | |||
Could not find work | 20.7 | 21.8 | 0.621 |
Retired | 41.0 | 44.9 | 0.026 |
Unable to work because of illness/disability | 34.1 | 23.9 | <0.001 |
Maternity/paternity leave | 2.3 | 1.2 | 0.049 |
Going to school | 7.6 | 9.2 | 0.237 |
Taking care of home or family | 16.5 | 17.3 | 0.649 |
Other | 6.3 | 6.1 | 0.904 |
Never worked for pay | 7.5 | 8.4 | 0.389 |
Functional limitations | |||
Any limitation in work, housework, or school | 17.0 | 10.5 | <0.001 |
Completely unable to do activities | 11.9 | 6.7 | <0.001 |
Cognitive limitation | 11.1 | 5.7 | <0.001 |
Limitations in physical functioning | 20.8 | 14.6 | <0.001 |
33.5 Time Costs
Patient and caregiver time costs account for the time spent by patients and caregivers traveling to and from treatment and follow-up care and time spent waiting for and receiving care. However, this information is not routinely collected. Time cost estimates can be calculated among patients and caregivers by combining the time spent receiving care or providing assistance with the human capita approach, willingness-to-pay method [14], or the friction cost [17] estimates of the value of that time. Although patient and caregiver time costs have been estimated among cancer survivors of all ages [25], limited information exists among AYA cancer survivors.
33.6 Methods for Economic Evaluation
The evaluation of medical interventions necessarily involves review of clinical outcomes and effectiveness. However, mounting healthcare costs and the associated strain imposed on patients, payers, and society has stimulated active consideration of the economic consequences of medical interventions. There is a range of economic evaluation tools for this purpose, each of which provides different types of information and requires different data and assumptions.
Economic evaluation of a healthcare intervention is founded on the premise that resources are finite and hence that using one diagnostic or therapeutic approach diverts resources away from others and perhaps from other health conditions. Each economic evaluation tool sheds light on whether diverting resources to the particular approach in question is, on balance, “efficient,” meaning that it improves population health relative to targeting resources to an alternative therapy or strategy. Efficiency is, of course, only one consideration in healthcare decision-making. Other factors may include, for example, equity and budget impact (affordability). However, understanding how efficiently resources are being allocated is a primary element of any healthcare cost analysis.
The following discussion reviews three approaches to cost evaluation in medical interventions: cost minimization analysis, cost-effectiveness analysis, and cost-benefit analysis. A cost minimization analysis compares the cost of two interventions that confer the same clinical benefits (e.g., two programs that each prevents the same number of breast cancer cases in similar populations). A cost-effectiveness analysis relaxes the requirement that the compared interventions confer equivalent clinical benefits. Finally, cost-benefit analysis assesses an intervention’s cost and clinical benefits, all measured in dollars, without reference to another health intervention. In short, cost-benefit analysis assesses the worth of a medical intervention relative to non-health alternative expenditure options.
In all cases, the analyst must select a perspective that determines which costs will be included. Common perspectives include (1) the patient and the patient’s family (including patient co-payments, which can be substantial in the case of certain medications, and time spent by family members caring for the patient); (2) the healthcare system (including both health delivery institutions and healthcare payers, such as private insurance companies and public programs like Medicare and Medicaid); and (3) societal (all costs, including those just described, and others, such as productivity losses absorbed by employers and workers).
The perspective of an analysis can influence its results. For example, consider an intervention that shifts care away from formal institutions and increases the amount of care provided by a patient’s family members. That intervention could appear to increase costs from the patient/patient family perspective, decrease costs from the healthcare system perspective, and perhaps show relatively little change in costs when assessed from the societal perspective.
Which perspective is most appropriate depends on the purpose of the analysis and its audience. Economists favor the societal perspective because it helps to identify interventions that are optimal in some global sense, whereas narrower perspectives are vulnerable to crediting interventions that merely shift costs away from one party to another. On the other hand, the societal perspective arguably suffers from a lack of relevance, at least in the US, because in this country, there is no “societal decision-maker” allocating all of society’s healthcare resources. Instead, it may make sense to assess an intervention’s impact from the healthcare perspective (e.g., if the analysis audience is payers) or from the patient/family perspective if the goal is to determine what burden alternatives impose on those most personally involved. Indeed all three perspectives have important roles to play, and the societal perspective has the potential to inform decisions made by agencies such as Medicare and Medicaid.
33.6.1 Cost Minimization Analysis
Because a cost minimization analysis limits attention to differences in cost among interventions, it is appropriate only when the decision-maker is not concerned with differences in clinical implications or when the differences in clinical impacts are minimal. For example, Green et al. [26] compared 6-month and 15-month treatments for children with Wilms tumor. The two groups did not differ significantly in terms of a 4-year relapse-free survival, the key clinical outcome. As a result, the relevant comparison was the difference in costs.
Drummond et al. [27] outline considerations for cost minimization analysis. A prime concern is cost assigned to medical services. That is because the amount charged may not correspond to the value of the resources consumed. One situation in which this occurs is when the difference between charges and costs is a monopoly profit for the provider of the services (i.e., a profit exceeding the normal rate of return earned in a competitive market). Whether the funds covering profit are a “cost” depends on the analytic perspective. From a societal perspective, monopoly profits represent a transfer, not an expenditure (and loss) of resources. That is because the cost imposed on the payer (in this case the patient) corresponds directly to the gain accrued by the service provider. Hence, the monopoly profit is a “wash.” For an analysis conducted from the patient perspective, payment covering “profit” is properly considered a cost.
Several other considerations are particularly relevant for diseases that have long-term impacts, like cancers affecting AYAs. First, how the time of family and caregivers is valued can influence the results. Time can be valued based on wage rates or on the value people place on their leisure.
Second, cost estimates depend on the analytic “time horizon.” Although clinical trial study outcomes may extend for a relatively limited period due to short-term follow-up, treatment impacts can extend for many years into the future. Such impacts can include morbidity associated with cancer treatments. It can also be reflected in reduced survival for cancer survivors. The analyst therefore faces a trade-off between limiting attention to empirically measured outcomes that omit relevant long-term impacts and estimating long-term impacts without the benefit of empirical data.
Whichever outcomes are included, future impacts should be discounted to reflect time preference. In brief, a cost incurred in the future has a lower “present value” than the same nominal cost incurred sooner. The present value of a cost C incurred n years in the future is
, where r is the annual discount rate (a value of 3 % is common) [28]. The impact of discounting can be substantial for analyses with time horizons of a decade or more, making this issue relevant in the case of AYAs. For example, at an annual rate of 3 %, discounting depresses the value of a cost incurred 30 years in the future by a factor of 2.4.

Third, the cost of a technology can change substantially over its life cycle because of rules governing patent protection [29]. In the US, manufacturers are often given a period during which they have the exclusive right to sell their product. That period is designed to allow manufacturers to recapture their research and development costs, but it means that drug prices are often substantially higher than they are following expiration of the patent and the introduction of competition by producers of generic versions of the drug. Hence, from the patient and healthcare payer perspectives, costs can change substantially over time. Average costs over a product’s lifecycle are somewhere between these two extremes.
Finally, depending on the perspective, non-healthcare costs can represent an important component of a disease’s overall costs. In the context of diseases affecting AYAs, productivity losses associated with disease can be particularly salient.
Productivity costs are relevant for societal analyses. They can also be relevant to patients to the extent that individuals lose wages because they cannot work. Companies that self-insure and can therefore be classified as “payers” can also be affected by the health conditions that impinge on worker productivity.
33.6.2 Cost-Effectiveness Analysis
A cost-effectiveness analysis compares interventions in terms of the “cost-effectiveness ratio,” which is the intervention’s added costs divided by its added health benefits. Calculation of the cost-effectiveness ratio requires estimation of health benefits, but that added information means that cost-effectiveness analysis can compare interventions that differ not just in terms of cost but also in terms of health effects.
Cost-effectiveness analysis is subject to the following considerations. First, the ratio depends on the target intervention to which the intervention is compared. The comparator could be “no treatment” (which is typically of limited relevance), or it could be an existing standard of care regimen. Relative to no treatment, an intervention’s incremental health benefits can be substantially greater than when the same intervention is compared to an existing treatment. Whether the incremental costs differ as much as the incremental benefits depends on how costly the standard of care is.
Second, it is possible for the incremental cost (the ratio’s numerator) to be negative, implying that the intervention reduces costs. That can happen when an intervention reduces utilization of more expensive downstream services. It is also possible for incremental health to be negative, meaning that the intervention, on net, makes health worse. That can happen if, for example, the impact of an intervention’s side effects exceeds its therapeutic benefits.
Finally, cost-effectiveness analysis can be customized to characterize value for a particular therapy by appropriately defining the health benefits. For example, for a cancer screening intervention, benefits might be defined as the number of cancer cases prevented. Tailoring the benefits to the disease and intervention under investigation helps to make the resulting ratio more salient and intuitive. Tailoring the outcome measure in this way, however, also limits the ratio’s usefulness because it can make it impossible to compare directly interventions that have different effects. For example, it is not possible to compare an intervention that costs $100,000 per myocardial infarction prevented to another that costs $150,000 per case of leukemia prevented. Cost-utility analysis, which we now turn to, addresses this limitation.
33.6.3 Cost-Utility Analysis
Cost-utility analysis is best thought of as a cost-effectiveness analysis that quantifies benefits in terms of “quality-adjusted life years” (QALYs) saved. QALYs were developed to characterize health benefits in terms of a “common metric” [30]. QALYs reflect both longevity and health-related quality of life (HRQL), meaning freedom from pain and normal ability to engage in regular activities. Individuals gain one QALY for each year they live in a (hypothetical) state of “perfect health.” They can also gain a QALY by living more than 1 year in less than perfect health. Hence, each year lived with an adverse health condition is worth less than one QALY. A health condition’s “utility weight” refers to the annual QALY gain experienced by an individual living with that condition. More severe conditions have lower utility weights. Being dead has, by convention, a utility weight of zero. In short, a health intervention can save QALYs by extending survival, improving HRQL (and hence increasing QALYs accrued per year), or some combination of both.
Because utility weights can and have been estimated for a wide range of health conditions, cost-utility analysis can be used to evaluate and compare a large number of health interventions. Greenberg et al. [31] identified 242 cancer-focused cost-utility analyses published through 2008 and cataloged in the Tufts Medical Center Cost-Effectiveness Analysis Registry. The median cost-effectiveness ratios (2008 US dollars) by cancer category were $27,000/QALY (breast cancer), $22,000/QALY (colorectal cancer), $34,500/QALY (prostate cancer), $32,000/QALY (lung cancer), and $48,000 (hematologic cancers).
The QALY metric facilitates comparisons across disparate disease and health conditions, but it also necessitates additional assumptions – most notably, the estimated utility weight for each health condition. Weights can be estimated by administering questionnaires to determine the trade-offs individuals are willing to make between longevity and diminished HRQL. QALYs can be estimated using those weights that reflect the preference of the population with the disease in question. But eliciting preferences from the affected population is not always feasible. Instead, preferences may be elicited from proxies (e.g., parents can serve as proxies to assess conditions affecting children), medical professionals, or the general population without the disease in question.
Alternatively, utility weights can be derived from standardized indexes that rate health conditions along several dimensions. For example, the EQ-5D measure has five dimensions (or domains) of health status – mobility, self-care, usual activities, pain/discomfort, and anxiety/depression, each of which is rated as “no problems,” “some problems,” and “extreme problems.” EQ-5D is used by the UK’s National Health Service to derive utility weights for a wide range of conditions. Standardized indexes can be more readily used than direct elicitation approaches because they do not involve eliciting preferences from a sample of respondents. But these scales may not account for or be sensitive to the particular aspects of the health condition. The EQ-5D accounts for some of the condition attributes associated with cancer, but it may only indirectly address others, such as fatigue. Moreover, the 3-point rating scale may have insufficient granularity to characterize the impact of various therapies. EQ-5D is one of a family of generic preference-based measures that are frequently used to generate the utility scores used as weights in estimating QALYs. Other generic preference-based measures that are widely used in cost-utility studies include the Health Utilities Index Mark 2 (HUI2) and Mark 3 (HUI3) [32, 33], the Quality of Well-Being Scale [34], and the Short-Form 6D based on the Short-Form 36 and Short-Form 12 Questionnaires [35, 36]. The scoring functions for each of these measures are based on preferences elicited from the general population, reflecting community values (societal viewpoint), an attribute favored by a number of regulatory authorities.
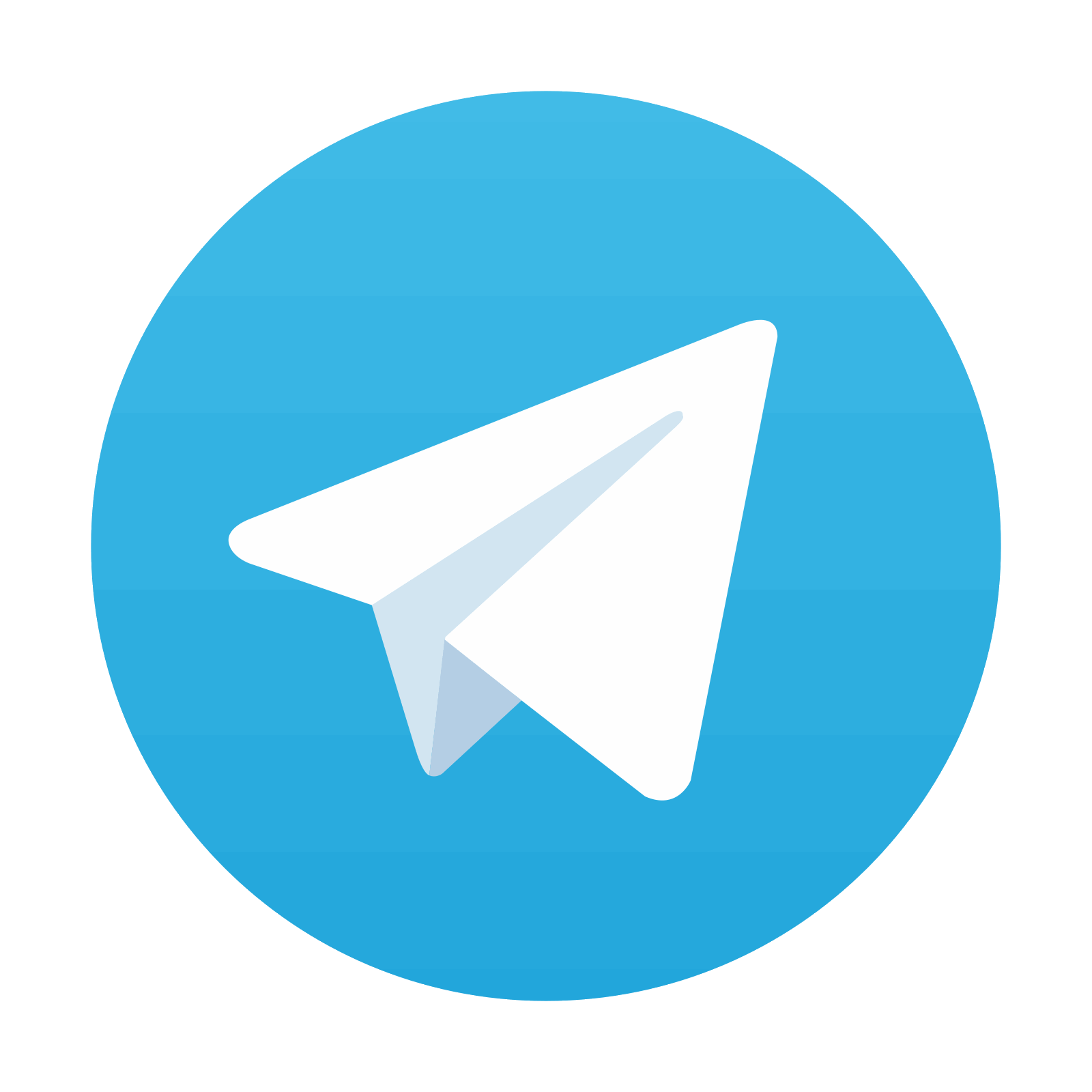
Stay updated, free articles. Join our Telegram channel

Full access? Get Clinical Tree
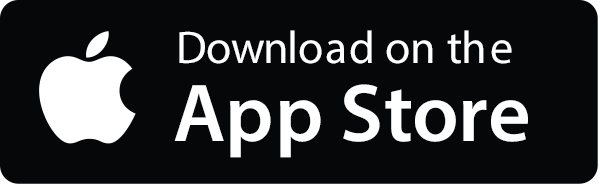
