Fig. 8.1
Illustration of predictive factors of response to treatment. The upper panel gives typical survival obtained after treatment and after control in biomarkers positive (left) and biomarker negative (right) patients. The lower panel presents the same data in a different way as survival curves are given for each biomarker category in the treatment arm (left) and in the control arm (right)
8.3 Randomized Design of the SHIVA Trial
The primary objective of the SHIVA trial was to compare the efficacy in terms of PFS of molecularly targeted therapy based on molecular profiling versus conventional therapy as selected by the investigator in patients with solid tumors refractory to standard treatments. The concept appeared particularly attractive for less common or rare tumor types for which dedicated randomized trials of MTAs are usually not carried out (Le Tourneau et al. 2010). This supported the idea to include all solid tumor types that can be evaluated for efficacy using the same criteria. A very large set of MTAs is on the market or under development, with various levels of evidence of activity depending on the stage of development. We decided to use only approved drugs (that can be assumed to have demonstrated clinical benefit in some tumor types) in order to control for this source of heterogeneity.
8.3.1 Flowchart
The flowchart of the study is provided in Fig. 8.2. The SHIVA trial included an observation cohort study as well as a randomized trial. In brief, the molecular profile of a patient tumor was performed on a mandatory biopsy/resection of a metastasis and analyzed by a molecular biology board (MBB) composed of biologists, physicians, and bioinformaticians. If one or several molecular alterations were identified, the molecular biology board applied a predefined algorithm to select the best MTA. Patients were then randomized between receiving the selected MTA and receiving a conventional treatment according to the investigators choice (that is based on tumor type, histopathological characteristics, etc.). The investigator and the patient were blinded to the molecular profile (Le Tourneau et al. 2014). A crossover was allowed at disease progression for patients in both treatment arms (patients who received conventional chemotherapy are proposed the MTA and vice versa).
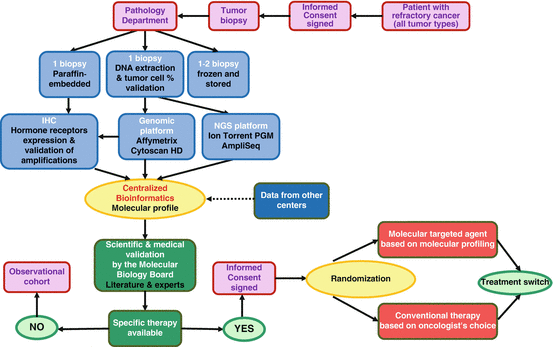
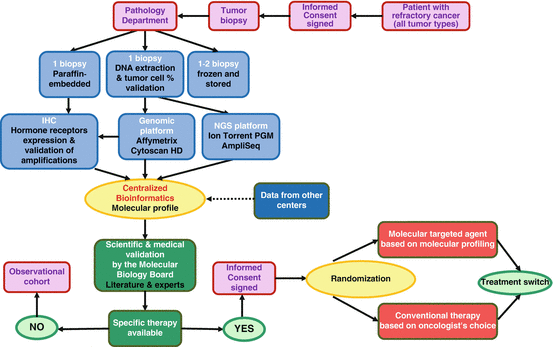
Fig. 8.2
Flowchart of the SHIVA trial
8.3.2 Endpoints
Progression-free survival was defined as the delay between randomization and progression according to RECIST 1.1 (Eisenhauer et al. 2009) or death, whatever the cause. Secondary efficacy objectives were to investigate the tumor growth according to the treatment arm, to explore the possible variation in treatment effect according to the altered pathway (interaction test), and to compare the tumor growth obtained with the MTA and the standard treatments for patients who crossed over. Tumor growth was defined quantitatively as the sum of the size of the targeted lesions identified using RECIST 1.1 standardized by the delay between measurements. This endpoint, conceptually appealing, raises several practical issues: new lesions and clinical progressions are not part of the quantitative measures, which may entail conflicting evaluations compared to standard criteria. Furthermore, patients with early progression or death without CT scan measurements are excluded. Alternatively, joint modeling of both radiological and clinical outcomes can be envisaged, but statistical analyses are then challenging.
8.3.3 Heterogeneity in Patients’ Prognosis
It is well known that prognosis differs depending on the tumor type, although patients with the same cancer in terms of location and histology might also display different prognosis (Le Tourneau et al. 2012). In order to control for this heterogeneity, randomization was stratified according to the signaling pathway relevant for the choice of the MTA and the patient prognosis based on the two categories of the Royal Marsden Hospital (RMH) score for oncology phase I trials (Olmos et al. 2012). Although molecular alterations may be prognostic for PFS, it was not possible to stratify the design for all possible molecular alterations. Three main signaling pathways have been arbitrarily identified: (1) the hormone receptors pathway, (2) the PI3K/AKT/mTOR pathway, and (3) the MAP kinase pathway. Therefore, combining the two levels of prognosis from the RMH score with the three molecular pathways, the randomization and the planned primary analysis were stratified on six strata. Last, quotas were introduced so that no more than 20 % of the randomized patients had the same tumor type.
8.3.4 Sample Size Calculation
The population of interest included various tumor types and various number of previous lines of treatment, similar to the population enrolled in phase I trials. The expected PFS of this population in the control arm could be derived from the one reported in phase I clinical trials of cytotoxic agents that have been eventually approved: 6-month PFS in this patient population was around 15 % (Horstmann et al. 2002). Under the hypothesis that doubling the 6-month PFS rate from 15 to 30 % was clinically relevant (i.e., HR = 0.63), a total of 142 events was required to detect a statistically significant difference in PFS between the randomized arms with a type I error of 5 % and a power of 80 % in a bilateral setting. To observe these events after an accrual time of 18 months and a minimum individual follow-up of 6 months, about 200 patients were needed to be randomized onto this trial.
8.4 Design Specificities Relating to the Use of High-Throughput Technologies
The evaluation of a complex intervention such as the algorithm to select the MTA raises specific issues that not only impact the design but also the statistical analysis and the final interpretation. First, this complex intervention combines two aspects: the treatment effect and the choice of the putative matching target. Therefore, the resulting efficacy can be related to either of the two, and the final interpretation is the evaluation of the whole strategy compared to another strategy (physician choice) which uses different treatments and a different modality to select the treatment. Second, several sources of variability related to the complexity of the intervention may contribute to the final results of the experiment. Eleven different targeted treatments have been administered based on 22 targets characterized by several dozen molecular alterations. A fundamental assumption behind the design is that the intervention has similar effects (or absence of effects) in all six strata, whatever the allocated treatment and whatever the molecular alteration used to select the treatment. This is the homogeneity assumption. In case the algorithm is only partly efficient, the power of the study is impacted. The magnitude of the impact is investigated in the following section. Third, as in any scientific experiment, the algorithm to select patients must be duly described, reproducible, and applicable to all participants. Defining the treatment algorithm was challenging as the knowledge regarding the biology of the tumors and the high-throughput platforms evolves quickly with time, and initial biological assumptions might become outdated. Platforms used the same protocol throughout the trial. Likewise, all bioinformatics analyses had to be centralized and applied to all patients regardless of recruitment center. No modification of the bioinformatics workflows was accepted during the project. Finally, all patients enrolled in the trial were analyzed in the same way. This is crucial as any research must be self-explanatory and reproducible. A treatment algorithm that would rely only on understated experts’ opinion would not be applicable outside of the center, and conclusions would not be applicable and generalizable to other samples. This is a key condition to be able to scientifically evaluate the intervention.
8.4.1 Strengths of Randomized Designs
The various aspects of the design were carefully thought to answer the primary objective, limit the bias, and maximize the power with constrained resources.
8.4.1.1 Randomization
As introduced in Sect. 8.3, a randomized clinical trial is mandatory to evaluate the added value of omic-based classifiers to guide patients’ treatment compared to standard approaches. Although the tumor biology, the mechanisms of drug resistance, and the role of the tumor environment are known to be crucial to accurately predict patient outcomes, they remain largely unknown, making it necessary to have a comparator. Furthermore, the prognosis of the highly selected patients (those whose tumors have a set of predefined molecular alterations) enrolled in such trials is not well known. Likewise, only an intent-to-treat analysis that makes full use of the randomization is appropriate. However, as shown in the next section, this is necessary, but this may not be sufficient to provide a clear picture of the benefit of the complex intervention.
8.4.1.2 Blinded Design
Blinding to the molecular profile is a crucial component to evaluate the benefit of the intervention (Boutron et al. 2006). The expectations of the physicians and of the patients in omic-based algorithms to select MTAs are high; there is a risk of bias in the follow-up as well as in the measure and interpretation of the primary outcome that are likely to favor the intervention arm. Ideally, a double-blind trial should be designed, which is delicate when several formulations, schedules, and agents are tested in the same trial.
8.4.1.3 Crossover
Crossover was allowed to patients at disease progression. Patients initially randomized in the intervention group may then receive conventional chemotherapy based on their tumor type, and patients in the control arm may receive the MTA matching the molecular alteration identified on the biopsy performed at inclusion, provided all eligibility criteria were still fulfilled at the time of progression. This gives a unique opportunity to compare both therapeutic strategies in the same patients using each patient as his/her own control. The randomization between the two arms of treatment can also be seen as a randomization between the two sequences of treatment, fulfilling one requirement of crossover designs. The statistical power of this analysis is theoretically higher than the one comparing the treatment efficacy between the two groups as it enables control for the various sources of patients related heterogeneity such as the natural history of the disease (the tumor location and histology), the history of previous treatments, etc., if the time to progression (TTP) for the two consecutive treatments are correlated (Buyse et al. 2011). Furthermore, in this planned crossover, all tumor evaluations are performed using the same criteria, the same set of target lesions identified prospectively. This gives a better and more robust assessment of the two consecutive TTP compared to retrospective assessment. However, in the likely event where a large fraction of patients cannot receive both arms (i.e., no crossover) due to clinical deterioration, for instance, the conclusions may be biased. Accordingly, the primary analysis should rely on the first period only. The main drawback of allowing crossover is to make any analysis of the overall survival meaningless.
8.4.1.4 Tumor Diversity
In clinical trials opened to all tumor types, distribution of disease depends strongly on the prevalence of the various cancers and the specific expertise of the participating centers. Yet, less common tumor types with frequent molecular abnormalities could benefit from the approach. To limit the risk that most patients have the same tumor type, which might preclude the generality of the results, heterogeneity in the tumor type can be increased by design. Setting up quotas for tumor types avoids overrepresentation of more frequent tumor types such as breast, lung, or colorectal cancers. Differences between the treatment arms would then be unlikely related to a given tumor type. This would reinforce the interest of developing new treatments based on biology first, possibly across multiple diseases. In summary, randomized designs allow for comparing two complex strategies on a valid endpoint while controlling for numerous confounding factors. A statistically significant difference between the two arms would be appropriately interpreted as the superiority of treating patients with MTAs based on molecular alterations and a predefined treatment algorithm compared to the conventional approach based on tumor location and histology. In other words, do we perform better than what we usually do for these patients?
8.4.2 Limitations of the Selected Design
The complexity of the question raised by such programs induces numerous limits to any statistical design. One may try to reduce the risk of biases or of inconclusive trial; this is however often not possible to avoid them totally.
8.4.2.1 Interpretation
An important question that will not be addressed in this type of trial is the independent effect of the algorithm. The design will not enable to disentangle the treatment effect from the algorithm effect. If a given MTA is active irrespective of the measure of the target (that is of the algorithm), we would draw the same conclusions as if the treatment worked due to the adequate selection of the patients.
The US national cancer institute sponsored M-PACT trial (NCT01827384) has been designed to specifically address the question of the added value of the algorithm regardless of the treatment effects. M-PACT focuses on four MTAs. Patients whose tumor expresses molecular alterations are randomized between the MTA matching the detected molecular alteration versus one of three other nonmatching therapy arms. In the latter case, the MTA is selected by the investigator blinded to the molecular profile. Only the added value of the algorithm is studied. Conversely, the control arm used in the M-PACT trial does not correspond to any standard of care, and the trial will not be able to conclude whether the global strategy is superior to the usual practice. Both types of trials are therefore quite complementary.
8.4.2.2 Population Heterogeneity
If randomization guarantees that the two groups of patients have comparable characteristics and the same overall prognosis, heterogeneity may dilute the expected benefit if the intervention effect is somehow modified by patients’ characteristics. Heterogeneity impacts any clinical research, but several sources of potential heterogeneity across patients are specific for (or more likely with) this kind of trials of limited sample sizes: the location and histology of the tumor, the molecular alterations, the assays used to identify the molecular alterations, and the diversity of treatments under study may impact the treatment effect. Stratification of the randomization and of the analysis on the RMH prognostic score and on the signaling pathway is an efficient mean to control heterogeneity in the prognosis of these various groups, assuming no interaction between the strata and the treatment effect (i.e. if the algorithm is relevant for all pathways as discussed in the following paragraph). It was impossible to stratify on the numerous tumor types. On the contrary, as noted in the previous section, we tried to increase the diversity of the tumor types to be able to draw conclusions that would be broadly applicable. This source of heterogeneity is intrinsic to the question addressed by the SHIVA trial, and we tried to build on it while controlling for the other identified sources of heterogeneity.
8.4.2.3 Treatment Effect Heterogeneity and Power
Beyond the expected heterogeneity in the population prognosis, there is a risk of heterogeneity in the effect of the MTA selected based on the molecular alteration. In other words, the algorithm to select the right treatment would be efficient for some molecular alterations (or equivalently for some treatments) and not for the others. For instance, in the SHIVA trial suppose that the treatment selected in case of an alteration on the PI3K/AKT/mTOR pathway is not active in this subset of patients, this would reduce the power of the primary analysis. Our ability to detect a 50 % reduction in the rate of progression or death at 6 months would be lower than the planned 80 %. This is illustrated by the forest plots in Fig. 8.3. Each line represents the MTA effect in a different stratum. For simplicity, PFS at 6 month is treated as a binary variable as if no patient was censored before a minimal follow-up at 6 months; the treatment effect is quantified by odds-rati (OR). In panel A, we have homogeneity of the treatment effect across all strata: whatever the signaling pathway and the prognostic group, the PFS rate is increased by 50 %. Conversely, in panel B, no treatment effect is observed in one of the strata, and the overall power of the primary analysis is reduced from 80 to 66 %.
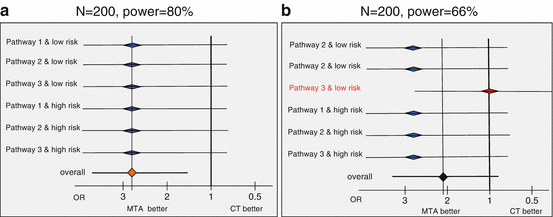
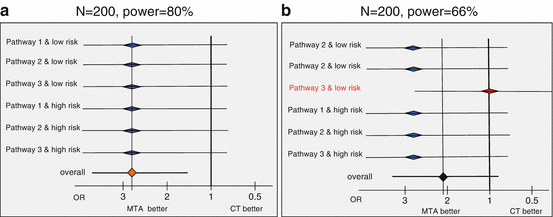
Fig. 8.3
Illustration of the loss of power if only part of the heterogeneity predicts the effect of treatment. Point estimates and 95 % confidence intervals (horizontal lines) are provided. (a) Homogeneous benefit of the targeted treatment selected based on molecular alterations in all strata; (b) benefit of the targeted treatment selected based on molecular alterations in all but one stratum. OR stands for odds ration; MTA for molecular targeted treatments; CT for chemotherapy; N gives the total sample size
The magnitude of the power loss depends on the number of strata where the MTA is not active, as shown in Table 8.1. The size of each stratum is also directly related to the power (results not shown). Homogeneity tests (or interaction tests) are part of the statistical analysis plan in order to detect this pattern of results. However, interaction tests are notoriously underpowered as shown in Table 8.1, and a strong heterogeneity may remain statistically undetected at the 5 % level.
Table 8.1
Power of the randomized comparative trial to detect an overall increase in the progression-free survival rate at 6 months from 15 to 30 % in case of heterogeneity assuming balanced prevalence of all six strata groups
Number of strata with MTA better
![]() Stay updated, free articles. Join our Telegram channel![]() Full access? Get Clinical Tree![]() ![]() ![]() |
---|