Fig. 9.1
The histogram shows the rate of increase per year in scientific publications indexed by PubMed, regarding the most frequent types of cancer: breast, lung, prostate, colon, and thyroid
Moreover, from a biological point of view, the mammary gland represents a valid model to study gene expression, epigenetics, differentiation, and cancer. Indeed, the mammary gland is one of the few organs undergoing adult cyclical morphogenesis during the fertile life span of the woman, being for large part under the influences of hormones, growth factors, neighboring cells, and molecules of the extracellular matrix (ECM) [2–4].
The Mammary Cancer Scenery
Figure 9.2 shows a representative image of a primary breast cancer tissue, which highlights crucial aspects of its basic phenomenology. It is well known that breast cancer includes a large number of histo-types and subgroups, but all cases are characterized by some essential steps of the progressive neoplastic transformation.
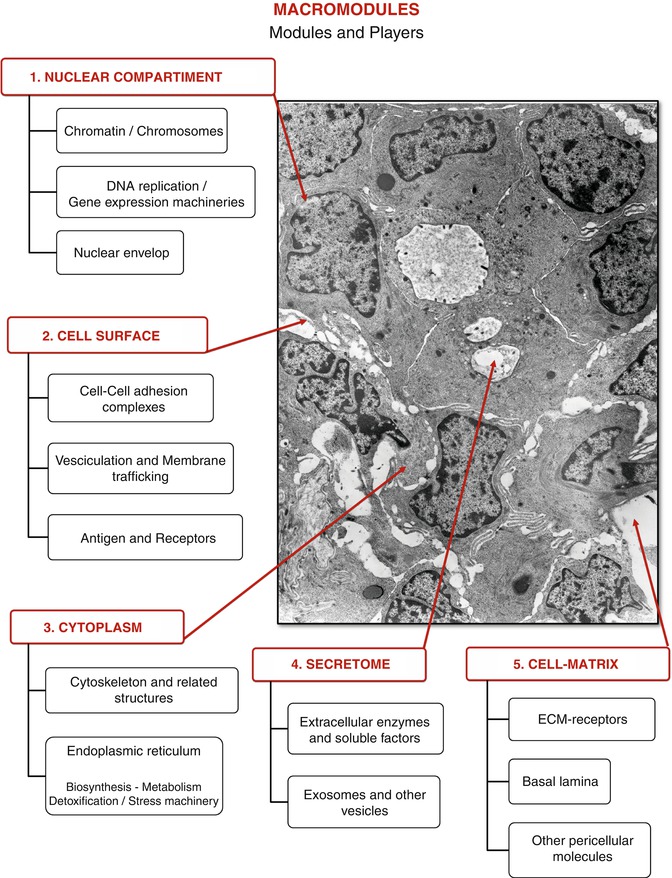
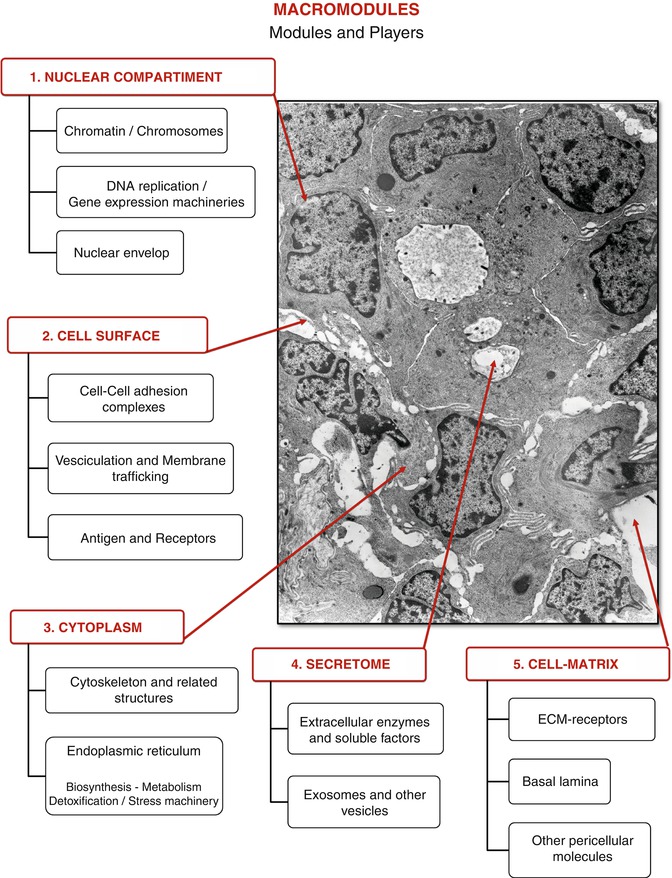
Fig. 9.2
The electron micrograph is a section of a ductal infiltrating carcinoma, where hypothetical macromodules and modules, each including the molecular players, are outlined (original magnification: 10,500×)
In an attempt to rationalize the complexity of this figure, we can subdivide the cytohistological components into macromodules, modules, and players, each interacting with the other, at different levels of complexity.
The spatial organization and the cellular confines allow to outline at least the following macromodules:
1.
The nuclear compartment, which includes the modules of chromatin/chromosomes, the DNA replication and the gene expression machineries, and the nuclear envelop and its accessory structures
2.
The cell surface, which includes the modules of cell–cell adhesion complexes, vesiculation and membrane trafficking, antigens, and receptors
3.
The cytoplasm, which incorporates the cytoskeleton and the varieties of the endoplasmic reticulum bearing a multiplicity of functional entities, including biosynthesis, metabolism, detoxification, and stress machinery
4.
The secretome, which comprises extracellular enzymes and soluble factors, exosomes, and other released vesicles
5.
The cell–matrix compartment that from the cell side contains ECM receptors and from the ECM side the basal lamina and other pericellular constitutive molecules. Within each module, there are an undefined number of players, namely, genes/proteins, responsible for the functional orchestration of the whole system, which can be grouped into affinity clusters. The protein clustering can be done according to their structural topologies or their evolutionary relationship, or by chemical, biological, or functional criteria, as needed.
The described macromodules are not to be viewed as rigid compartments; on the contrary, the functional units need to exchange activities with each other. Indeed, functional modules are dynamic entities; for instance, a protein belonging to the cytoplasm compartment, under certain circumstances, may migrate into the nucleus and play additional important functions. As a consequence, the functional clustering of proteins is necessarily “transverse” to the structural modules.
Returning to Fig. 9.2, it can be observed that the major cellular changes involved in the conversion of a normal breast into a malignant breast are the progressive loss of the cell–cell adhesions and of the polarized morphology, typical of the stationary epithelial phenotype. Concurrently, once the cells detach from each other, the cell surfaces become unstable acquiring a ruffled appearance, with a tendency to release vesicles of a different nature. Fully detached cells usually acquire a mesenchymal-like phenotype, which explains well their ability to migrate into surrounding tissues. This tendency, recognized as EMT (epithelial/mesenchymal transition) and sustained by a defined set of genes, is manifested exaggeratedly in the primary culture from surgical breast cancer biopsies [5]. These alterations in gene expressions are able to drive the cancer progression, but the exhaustive number, identities, and functional network of the involved genes/proteins are still to be fully recognized.
The Proteomic Dream, the Proteomic Current Reality
The term “proteome” originally coined by Wilkins [6] was intended to describe “the total protein complement of a genome.” With the beginning of the development of the proteomic research, it has become clearer and clearer that this definition could probably be applied to unicellular organisms but not equally to higher organisms, especially in humans; therefore, it is more appropriate to refer to the proteome as “the complement of protein extracted from biological samples under given conditions.” The deriving information can then be used for comparative proteomics, drug responses, modulation of gene expression, etc.
The application of proteomics to surgical tissues must take into consideration several problems, some of which are inherent to the dynamic nature and the complexity of cell and tissue themselves and others related to technical implications. The former include the variable amount of extracellular components in tissue biopsies; the different turnover of proteins in a given cell, which may influence the protein concentration of the proteome; and the frequent occurrence of several posttranslational isoforms for a given primary gene product. The technical limitations are mainly related to the solubilization systems (no single procedure can be applied for the extraction of the entire set of native proteins) and to the separating systems (traditional 2D-IPG is unable to focus proteins with pI over 8.5). Therefore, the old dream to recognize a given “signature” or “constellation” into a single proteomic map, able to distinguish, for instance, a healthy cell from its neoplastic counterpart, has been profoundly reviewed. “Signature” and “constellation” should be deduced from the statistical evaluations of a significant number of data properly collected for specific targets. On the other hand, comparative proteomic profiling of cells and tissues, under the same extraction conditions, has provided an extraordinary amount of information.
The initial applications of the proteomic technologies were essentially based on the 2D-IPG protein separation, which, thanks to the introduction of gel strips with immobilized ampholyne systems commercially available, gave the opportunity to standardize exchangeable protocols among laboratories. An exciting result was the generation of several databases of 2D-IPG for protein identity search (e.g., SWISS-2DPAGE). The beginning of the history focuses on the method of the gel matching, based on the computerized analyses of the proteomic maps and spot detection, followed by N-sequencing identification (Edman degradation) and immunological validation of the selected spots.
Over the past 10 years, a number of additional technologies have been developed to analyze proteins on a large scale, first of all the mass spectrometry (MS) methods on digested proteins, namely, the electron spray ionization (ESI) and the matrix-assisted laser desorption/ionization (MALDI) [7].
The MS spectra are then matched with known sequences in databases (e.g., SEQUEST, MASCOT) to calculate masses, resulting in the identification of target proteins. This type of protein identification method is known as “peptide mass fingerprinting” [8].
More recently a new strategy—non–gel-based proteomics, defined as “shotgun proteomics”—emerged as a method that could offer advantages in speed, sensitivity, and automation over the gel-based techniques. Proteins are extracted from a biological sample and digested with a protease to produce a peptide mixture [9]. The peptide mixture is then loaded directly onto a microcapillary column and the peptides are separated by hydrophobicity and charge. The charged fragments are separated in the second stage of tandem mass spectrometry. A serious limitation of non-gel-based proteomics is the low score of protein identities, due to the well-known homologies among diverse proteins. On the contrary, the 2D-based proteomics introduce two fundamental parameters useful for protein identification, which are pI and Mw, elevating the score for protein identification.
Additional complementary proteomic approaches, based on the differential labeling of protein extracts with stable isotopes (1H and 2H, 12C and 13C, 14N and 15N), have been developed to improve the evaluation of target protein expressions: the peak intensity of the differentially labeled peptide is used for quantitative evaluations. Among the isotopic labeling-based approaches, two are currently most used: the SILAC (stable isotope labeling by amino acids in cell culture) and the ICAT (isotope-coded affinity tag). The SILAC approach requires the addition of a stable isotope-labeled amino acid (i.e., 2H-leucine or 13C-arginine) to the cell culture. The ICAT is based on the incorporation of isotopic tags after protein extraction and is suitable for experimental conditions where metabolic labeling is not feasible (i.e., protein samples extracted from tissues); reviewed by Liang et al. [10].
Proteomics of Breast Cancer Tissues to Detect Putative Spontaneous Tumor Markers
The first reason of concern in detecting putative biomarkers from cancer cells and tissues is the establishment of a “normal” reference range of qualitative/quantitative protein expression. The utilization of matched tumoral and healthy tissue adjacent to the tumor is a consolidated system to identify set of proteins specifically related to the presence of neoplastic cells. In a pilot study by our group [13], we carried out a comparative proteomic profiling of paired tumoral and non-tumoral tissue counterparts extracted from 13 selected surgery specimens from ductal infiltrating carcinomas (DIC) and processed in parallel. Results provided substantial information on qualitative and quantitative differences between the two sets of tissues and allowed the constructing of a reference map of tumoral tissue to be utilized for further comparative analyses. Figure 9.3 shows a representative map of a breast cancer tissue containing 312 identified protein forms grouped into 11 categories and reported in Table 9.1. To avoid redundancy, clusterization was accomplished following the criterion of primary function of proteins. In addition, a “transverse” category is introduced in Table 9.2. This comprises proteins with diverse primary functions, all involved in the regulation of cell proliferation and cell death, according to the DAVID bioinformatics resources [14].
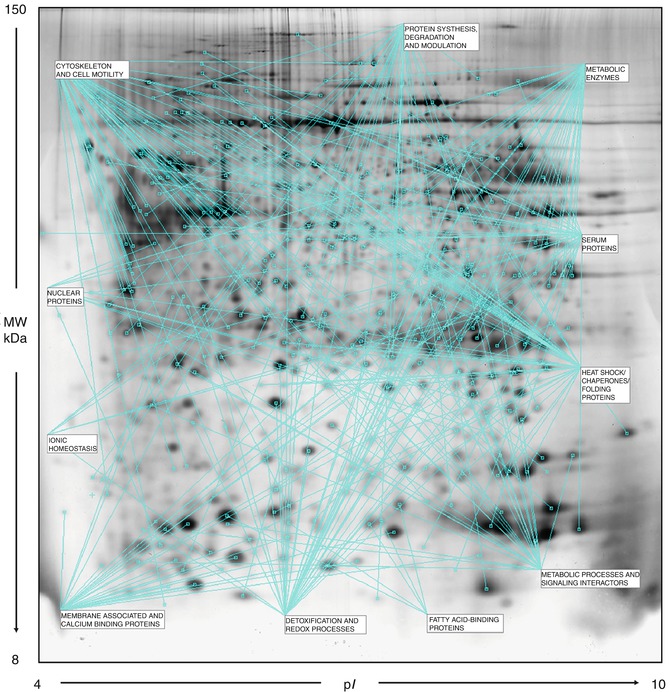
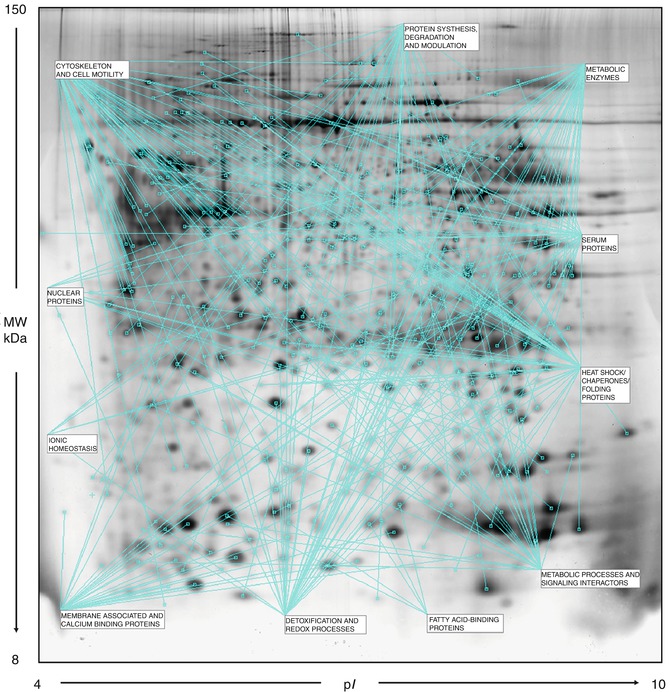
Fig. 9.3
A representative proteomic map of a breast cancer tissue (DIC) containing 312 identified protein forms grouped into 11 categories and reported in Table 9.1 [2-DE separation was performed on IPG gel strips (18 cm, 3.5–10 NL) followed by the SDS-PAGE on a vertical linear-gradient slab gel (9–16 %T). The 2D gels were analyzed using the software ImageMaster 2d Platinum]
Table 9.1
Proteins identified in breast cancer tissues and clustered according to their primary function
Metabolic enzymes | |||
---|---|---|---|
Protein name | AC number | Abbreviated name | Protein isoform Nr |
Aconitate hydratase, mitochondrial | Q99798 | ACON | 2 |
Alpha-enolase | P06733 | ENOA | 7 |
Enoyl-CoA hydratase, mitochondrial | P30084 | ECHM | |
Fructose-bisphosphate aldolase A | P04075 | ALDOA | 4 |
Fumarate hydratase, mitochondrial | P07954 | FUMH | |
Gamma-enolase | P09104 | ENOG | |
Glyceraldehyde-3-phosphate dehydrogenase | P04406 | G3P | 5 |
L-lactate dehydrogenase A chain | P00338 | LDHA | |
L-lactate dehydrogenase B chain | P07195 | LDHB | |
Malate dehydrogenase, cytoplasmic | P40925 | MDHC | |
Malate dehydrogenase, mitochondrial | P40926 | MDHM | |
Neutral alpha-glucosidase AB | Q14697 | GANAB | |
Phosphoglycerate kinase 1 | P00558 | PGK 1 | 3 |
Phosphoglycerate mutase 1 | P18669 | PGAM1 | 2 |
Pyruvate kinase isozymes M1/M2 | P14618 | KPYM | 3 |
Triosephosphate isomerase | P60174 | TPIS | 4 |
Metabolic processes and signaling interactors | |||
14-3-3 Protein gamma | P61981 | 1433G | |
3-Hydroxyisobutyryl-CoA hydrolase, mitochondrial | Q6NVY1 | HIBCH | |
Acyl-CoA-binding protein | P07108 | ACBP | |
Bifunctional purine biosynthesis protein PURH | P31939 | PUR9 | |
dCTP pyrophosphatase 1 | Q9H773 | DCTP1 | |
Glyoxalase domain-containing protein 4 | Q9HC38 | GLOD4 | |
Macrophage migration inhibitory factor | P14174 | MIF | |
N(G),N(G)-dimethylarginine dimethylaminohydrolase 1 | O94760 | DDAH1 | |
N(G),N(G)-dimethylarginine dimethylaminohydrolase 2 | O95865 | DDAH2 | |
Nucleoside diphosphate kinase A | P15531 | NDKA | |
Nucleoside diphosphate kinase B | P22392 | NDKB | |
Phosphatidylethanolamine-binding protein 1 | P30086 | PEBP | 2 |
Purine nucleoside phosphorylase | P00491 | PNPH | 2 |
Pyridoxine-5′-phosphate oxidase | Q9NVS9 | PNPO | |
Rho GDP-dissociation inhibitor 1 | P52565 | GDIR1 | |
Rho GDP-dissociation inhibitor 2 | P52566 | GDIR2 | |
SH3 domain-binding glutamic acid-rich-like protein | O75368 | SH3L1 | 2 |
SH3 domain-binding glutamic acid-rich-like protein 3 | Q9H299 | SH3L3 | |
Sialic acid synthase | Q9NR45 | SIAS | 3 |
Thiosulfate sulfurtransferase/rhodanese-like domain-containing protein 1 | Q8NFU3 | TSTD1 | 2 |
Thymidine phosphorylase | P19971 | TYPH | |
Fatty acid-binding proteins | |||
Fatty acid-binding protein, adipocyte | P15090 | FABP4 | |
Fatty acid-binding protein, epidermal | Q01469 | FABP5 | |
Fatty acid-binding protein, brain | O15540 | FABP7 | 2 |
Fatty acid-binding protein, heart | P05413 | FABPH |
Cytoskeleton and cell motility | |||
---|---|---|---|
Protein name | AC number | Abbreviated name | Protein isoform Nr |
Actin, cytoplasmic 1 | P60709 | ACTB | 15 |
Actin-related protein 2/3 complex subunit 5 | O15511 | ARPC5 | |
Adenylyl cyclase-associated protein 1 | Q01518 | CAP1 | 2 |
Cofilin-1 | P23528 | COF1 | 4 |
Coronin-1A | P31146 | COR1A | |
F-actin-capping protein subunit alpha-1 | P52907 | CAZA1 | |
Fascin | Q16658 | FSCN1 | |
Macrophage-capping protein | P40121 | CAP G | 3 |
Myosin light polypeptide 6 | P60660 | MYL6 | |
Programmed cell death 6-interacting protein | Q8WUM4 | PDC6I | |
Thymosin beta-4-like protein 3 | A8MW06 | TMSL3 | |
Tropomyosin alpha-1 chain | P09493 | TPM1 | |
Tropomyosin alpha-4 chain | P67936 | TPM4 | 3 |
Tropomyosin beta chain | P06468 | TPM2 | 2 |
Tubulin alpha-1 chain | Q71U36 | TBA1A | 3 |
Tubulin beta-5 chain | P07437 | TBB5 | 2 |
Vimentin | P08670 | VIME | 5 |
Vinculin | P18206 | VINC | 2 |
Membrane associated and calcium-binding proteins | |||
Annexin A1 | P04083 | ANXA1 | 3 |
Annexin A2 | P07355 | ANXA2 | 3 |
Annexin A4 | P09525 | ANXA4 | |
Annexin A5 | P48036 | ANXA5 | 2 |
Calmodulin | P62158 | CALM | |
Galectin-1 | P09382 | LEG1 | 2 |
Galectin-3 | P17931 | LEG3 | 2 |
Protein S100-A2 | P29034 | S10A2 | |
Protein S100-A4 | P26447 | S10A4 | 2 |
Protein S100-A6 | P06703 | S10A6 | 2 |
Protein S100-A7 | P31151 | S10A7 | 2 |
Protein S100-A8 | P05109 | S10A8 | |
Protein S100-A11 | P31949 | S10AB | 3 |
Protein S100-A13 | Q99584 | S10AD | |
Nuclear proteins | |||
Acidic leucine-rich nuclear phosphoprotein 32 family member A | P39687 | AN32A | |
Heterogeneous nuclear ribonucleoprotein A1 | P09651 | ROA1 | 2 |
Heterogeneous nuclear ribonucleoproteins A2/B1 | P22626 | ROA2 | 3 |
Nuclear transport factor 2 | P61970 | NTF2 | |
Nucleophosmin | P06748 | NPM | |
Prelamin-A/C | P02545 | LMNA | 2 |
RuvB-like 1 | Q9Y265 | RUVB1 | |
Ionic homeostasis | |||
Carbonic anhydrase 1 | P00915 | CAH1 | |
Inorganic pyrophosphatase | Q15181 | IPYR | 2 |
Selenium-binding protein 1 | Q13228 | SBP1 | |
V-ATPase subunit F | Q16864 | VATF | |
Voltage-dependent anion channel protein 2 | P45880 | VDAC2 |
Protein synthesis, degradation and modulation | |||
---|---|---|---|
Protein name | AC number | Abbreviated name | Protein isoform Nr |
26S protease regulatory subunit 8 | P62195 | PRS8 | |
40S ribosomal protein SA | P08865 | RSSA | |
60S acidic ribosomal protein P0 | P05388 | RLA0 | |
60S acidic ribosomal protein P2 | P05387 | RLA2 | 2 |
Cathepsin D | P07339 | CATD | 3 |
Cystatin-A | P01040 | CYTA | |
Cystatin-B | P04080 | CYTB | |
Cytosol aminopeptidase | P28838 | AMPL | |
Elongation factor 1-beta | P24534 | EF1B | |
Elongation factor 2 | P13639 | EF2 | 3 |
Eukaryotic translation initiation factor 6 | P56537 | IF6 | |
Proteasome activator complex subunit 1 | Q06323 | PSME1 | |
Proteasome subunit alpha type-1 | P25786 | PSA1 | |
Proteasome subunit alpha type-5 | P28066 | PSA5 | |
Proteasome subunit alpha type-6 | P60900 | PSA6 | |
Proteasome subunit beta type-3 | P49720 | PSB3 | |
Ribosome-binding protein 1 | Q9P2E9 | RRBP1 | |
Small ubiquitin-related modifier 1 | P63165 | SUMO1 | |
U3 small nucleolar RNA-interacting protein 2 | O43818 | U3IP2 | |
Ubiquitin carboxyl-terminal hydrolase isozyme L1 | P09936 | UCHL1 | |
Ubiquitin-60S ribosomal protein L40 | P62987 | RL40 | 2 |
Ubiquitin-conjugating enzyme E2 N | P61088 | UBE2N | |
Ubiquitin-conjugating enzyme E2 variant 2 | Q15819 | UB2V2 | |
Ubiquitin-like protein Nedd8 | Q15843 | NEDD8 | |
Detoxification and redox processes | |||
Alcohol dehydrogenase [NADP(+)] | P14550 | AK1A1 | 2 |
Aldehyde dehydrogenase, cytosolic | P00352 | AL1A1 | |
Aldo-keto reductase family 1 member B10 | O60218 | AK1BA | 2 |
Aldose reductase | P15121 | ALDR | 2 |
Chloride intracellular channel protein 1 | O00299 | CLIC1 | 2 |
Dihydrolipoyl dehydrogenase, mitochondrial | P09622 | DLDH | |
Flavin reductase (NADPH) | P30043 | BLVRB | |
Glutathione S-transferase P | P09211 | GSTP1 | |
Glutathione synthetase | P48637 | GSHB | |
Glutathione transferase omega-1 | P78417 | GSTO1 | 2 |
Isocitrate dehydrogenase [NADP] cytoplasmic | O75874 | IDHC | 2 |
Peroxiredoxin 6 | P30041 | PRDX6 | 2 |
Peroxiredoxin-1 | Q06830 | PRDX1 | 5 |
Peroxiredoxin-2 | P32119 | PRDX2 | 3 |
Peroxiredoxin-3 | P30048 | PRDX3 | |
Peroxiredoxin-4 | Q13162 | PRDX4 | |
S-formylglutathione hydrolase | P10768 | ESTD | 3 |
Superoxide dismutase [Cu-Zn] | P00441 | SODC | 2 |
Superoxide dismutase [Mn], mitochondrial | P04179 | SODM | 2 |
Thioredoxin | P10599 | THIO | 2 |
Heat-shock/chaperones/folding proteins | |||
---|---|---|---|
Protein name | AC number | Abbreviated name | Protein isoform Nr |
Calreticulin | P27797 | CALR | |
60 kDa heat shock protein, mitochondrial | P10809 | CH60 | 2 |
94 kDa glucose-regulated protein | P14625 | ENPL | |
Endoplasmic reticulum resident protein 29 | P30040 | ERP29 | |
Glucosidase 2 subunit beta | P14314 | GLU2B | |
75 kDa glucose-regulated protein | P38646 | GRP75 | |
78 kDa glucose-regulated protein | P11021 | GRP78 | 4 |
Heat shock protein HSP 90-alpha | P07900 | HS90A | |
Heat shock protein HSP 90-beta | P08238 | HS90B | |
Heat shock 70 kDa protein 1A/1B | P08107 | HSP71 | |
Heat shock 70 kDa protein 4 | P34932 | HSP74 | |
Heat shock cognate 71 kDa protein | P11142 | HSP7C | 4 |
Heat shock protein beta-1 | P04792 | HSPB1 | 5 |
Parkinson disease protein 7-Oncogene DJ1 | Q99497 | PARK7 | 4 |
Protein disulfide isomerase | P07237 | PDIA1 | |
Protein disulfide isomerase A3 | P30101 | PDIA3 | 4 |
Peptidyl-prolyl cis-trans isomerase A | P62937 | PPIA | 5 |
Peptidyl-prolyl cis-trans isomerase B | P23284 | PPIB | |
Ras-related protein Rab-18 | Q9NP72 | RAB18 | |
Cellular retinoic acid-binding protein 2 | P29373 | RABP2 | 2 |
Transitional endoplasmic reticulum ATPase | P55072 | TERA | 2 |
Stress-induced phosphoprotein 1 | P31948 | STIP1 | 2 |
Serum proteins | |||
Alpha-1-acid glycoprotein 2 | P19652 | A1AG2 | |
Alpha-1-antitrypsin | P01009 | A1AT | 2 |
Alpha-2-macroglobulin | P01023 | A2MG | |
Alpha-1-antichymotrypsin | P01011 | AACT | |
Serum albumin | P02768 | ALBU | 2 |
Apolipoprotein A1 | P02647 | APOA1 | 2 |
Beta-2-microglobulin | P61769 | B2MG | |
Complement component 1 Q | Q07021 | C1QBP
![]() Stay updated, free articles. Join our Telegram channel![]() Full access? Get Clinical Tree![]() ![]() ![]() |