Recognized gene/region
Position
Mapped by
Allele incidence
Recognized/feasible role
BRCA1
17q21
Linkage
Rare
DNA repair/genome stability
BRCA2
13q13.1
Linkage
Rare
Recombinational repair
TP53
17p13.1
Linkage
Rare
Li–Fraumeni syndrome, apoptosis
ATM
11q22.3
CS
Rare
DNA repair
BRIP1
17q23.2
CS
Rare
DNA repair, associated with BRCA1
CHEK2
22q12.1
CS
Rare
DNA repair/cell cycle
PALB2
16p12.2
CS
Rare
Associated with BRCA2
RAD51C
17q22
CS
Rare
Homologous recombination repair
PTEN
10q23.3
Linkage
Rare
Cowden disease, cell signaling
STK1 (LKB1)
19p13.3
Linkage
Rare
Peutz–Jeghers syndrome, cell cycle arrest
CDHå1
16q22.1
Linkage
Rare
Intercellular adhesion: lobular BC
FGFR2
10q26
GWAS
Common
Fibroblast growth factor receptor
TOX3(TNRC9)//RBL2
16q12
GWAS
Common
Chromatin structure/cell cycle
MAP3K1
5q11.2
GWAS
Common
Cellular response to growth factors
LSP1
11p15.5
GWAS
Common
Neutrophil motility
8q24
8q24
GWAS
Common
Intergenic, enhancer of MYC proto-oncogene?
2q35
2q35
GWAS
Common
–
CASP8
2q33
GWAS
Common
Apoptosis
SLC4A7/NEK10?
3p24.1
GWAS
Common
Cell cycle control?
COX11/STXBP4?
17q22
GWAS
Common
Transport?
MRPS30?
5p12
GWAS
Common
Apoptosis?
NOTCH2/FCGR1B?
1p11.2
GWAS
Common
Signaling/immune response?
RAD51L1
14q24.1
GWAS
Common
Homologous recombination repair?
CDKN2A/CDKN2B?
9p21
GWAS
Common
Cyclin-dependent kinase inhibitors?
MYEOV/CCNDL?
11q13
GWAS
Common
Cell cycle control/fibroblast growth factors?
ZNF365?
10q21.2
GWAS
Common
Zinc finger protein gene
ANKRD16/FBXO18?
10p15.1
GWAS
Common
Helicase?
ZMIZ1?
10q22.3
GWAS
Common
Regulates transcription factors?
Perhaps one of the unexpected findings from these studies is a greater-than-anticipated role for noncoding variants in common diseases [27]. From the analysis of population sequences [28], 30 % of common variants associated with disease are annotated as, or in linkage disequilibrium with, non-synonymous (coding) variation. This supports the view that many of the common disease variants have gene regulatory roles. Among the set of well-established common susceptibility genes are variants in intron 2 of the FGFR2 gene [29], which, among the common variants, are likely to make one of the larger contributions to relative risk, at least for postmenopausal disease. Easton et al. [30] found that the rs2981582 SNP (allele frequency 0.38) contributes odds ratios of 1.23 and 1.63 for heterozygote and homozygote genotypes, respectively. The FGFR2 gene encodes a fibroblast growth factor (FGF) receptor. FGFs and their corresponding receptors are involved in regulation of the proliferation, survival, migration, and differentiation of cells. The considerable importance of FGF signaling in a range of tumor types is now becoming recognized [31].
SNPs within intron 2 are involved in FGFR2 upregulation, and aberrant signaling activation induces proliferation and survival of tumor cells [32]. The identification of this gene, which was unanticipated as a cancer gene, has prompted research into related genes and their potential roles in cancer. Other FGFs (e.g., FGF-8) appear to be involved in breast cancer cell growth through stimulation of cell cycle and prevention of cell death [33].
Other low-penetrance variants that have been identified through GWAS include CASP8 (caspase 8), which encodes an apoptotic enzyme [34]. The variant rs1045485 is protective, contributing odds ratios of 0.89 and 0.74 for heterozygotes and rare homozygotes, respectively. Recently, variants in CASP8 have been shown to alter risks (in a protective direction) in individuals with a family history of breast cancer [35].
Breast tumors are classified according to whether they have receptor proteins that bind to estrogen and progesterone. Such cells are termed ER + and PR + and require estrogen and progesterone to grow. Conversely, ER − and PR − tumors lack the protein that allows the hormones to bind. Tumor classifications manipulate the choice of treatment regimes for the patient. A further classification arises through tumors that overexpress the human epidermal growth factor receptor 2 (HER2) genes, which are termed HER2+ (conversely, HER2−). The triple-negative subtypes are ER−, PR−, and HER2− and are characterized by aggressive tumors and reduced range of effective treatment options. Several common gene variants are more strongly associated with specific cancer subtypes. These include the TOX3 gene, formerly called TNRC9 in which variant rs3803662 contributes a 1.64-fold homozygote risk, specifically in ER + cancer [36]. This gene encodes a high-mobility group chromatin-associated protein, and increased expression is implicated in bone metastasis [37].
Fine mapping has shown that hypothesized susceptibility variants lie in an intergenic region consistent with a gene regulatory function [38]. These authors note there remains uncertainty as to whether the causal variant is actually involved in the regulation of the nearby retinoblastoma-like gene 2 (RBL2) gene, which is involved in cell cycle regulation, given gene expression evidence.
The mitogen-activate protein kinase (MAP3K1) breast cancer gene [30] is a member of the Ras/Raf/MEK/ERK signaling pathway (as is FGFR2) and is involved in regulating transcription of a number of cancer genes. MAP3K1 has been found to be more strongly associated with ER + and PR + tumors than ER−/PR − subtypes. There is also a stronger association with HER2+ tumors [39]. The LSP1 gene was identified as breast cancer susceptibility locus by Easton et al. [30] who identified a SNP within the intron as the most strongly associated. LSP1 encodes lymphocyte-specific protein 1, which is an F-actin binding cytoskeletal protein. The same study also identified a breast cancer variant in the 8q24 region containing no known genes. This region is also associated with prostate cancer [40]. Stacey et al. identified a SNP on 2q35, a region with no known genes, as associated with breast cancer in Icelandic patients with ER + breast cancer [36]. Milne et al. also found an association with ER − disease, although there was a stronger signal for ER+ [41]. Other breast cancer associations include signals on 3p24, potentially relating to the genes SLC4A7 or NEK10, and on 17q22, perhaps related to COX11. These SNPs contribute odds ratios of 1.11 and 0.97 for heterozygote and homozygote genotypes, respectively [42]. Additionally, a common variant close to MRPS30 on 5p12 was found to confer higher risk of ER + disease [43]. Turnbull et al. described five new associations on chromosomes 9, 10 (three regions), and 11 [44]. Two further signals reported by Thomas et al. [45] include a SNP in the pericentromeric part of chromosome 1, within a region containing NOTCH2 and FCGR1B, and a signal associated with another double-strand break repair gene (RAD51L1) on 14q24.1. There is evidence that the chromosome 1 locus is more strongly associated with ER + disease.
Considerable additional follow-up investigation will be required to establish the relationships between many of the SNPs and the actual causal variant(s) and to further elucidate the role in disease for many of these common genes.
Genome-Wide Association Studies
In modern years, the research of low-penetrance allelic variants was conducted mainly through GWAS. These studies use a large number of common genetic SNPs to identify associations with disease that rely upon patterns of linkage disequilibrium in the human genome [22]. The power of GWAS is to evaluate the association of genetic variants at different loci on different chromosomes in large series of cases versus controls, analyzing a panel of 100,000 SNPs simultaneously, to identify new alleles of susceptibility to BC [23]. In the human genome, it has been estimated that there are 7 million common SNPs that have a minor allele frequency (m.a.f.), 45 %, and because recombination occurs in different hot spots, the nascent polymorphisms are often strongly correlated. These studies therefore supply a powerful tool to recognize novel markers for susceptibility and prognosis of disease [46–48].
In the GWA studies, the accumulation of a large number of data is crucial. Houlston and Peto have estimated the number of cases required to identify low-penetrance alleles conferring a relative risk of two both in an unselected population and in families with first-degree relatives affected [47]. In an unselected population, the identification of a susceptibility allele with a frequency of 5 % requires over 800 cases. In the same population, the identification of a susceptibility allele with a frequency of 1 % requires over 3,700 unselected cases, whereas about 700 would be enough if three affected families are selected. Therefore, the power of association studies can be significantly increased using selected cases with a family history of cancer because fewer cases are required to demonstrate the association with the disease [47].
The potential of the association studies of cases with a family history to identify low-penetrance alleles conferring a relative risk of 2 has been demonstrated by the mutation CHEK2 1100delC in patients with BC. This variant carried by 1 % of the population confers an increased risk of 1.7-fold. The frequency was not significantly increased in unselected cases (1.4 %), but it was strongly increased in familial cases without BRCA1 and BRCA2 mutations (5.1 %) [49].
In the past several years, several novel risk alleles for BC were identified by four recent GWA studies: Breast Cancer Association Consortium, Cancer Genetic Markers of Susceptibility, DeCode Islanda, and Memorial Sloan–Kettering Cancer Center [29, 30, 43, 50]. In each of them, the association study was shared in three phases: the first phase identifies the common SNPs in cases and controls, the second phase evaluates how many of the above SNPs are common to a greater number of cases and controls, and, finally, the third phase aims to identify new alleles of susceptibility to BC. Easton et al., in their study, identified five independent loci associated with increased susceptibility to BC (Po10_7) [30]. This multistage study involved in the first stage 390 BC cases with a strong family history and 364 controls and 3,990 cases and 3,916 controls in the second stage. To define the risk associated with the 30 most significant SNPs, a third stage of the study was conducted involving 21,860 cases and 22,578 controls from 22 additional studies in the Breast Cancer Association Consortium. These combined analyses allowed the observation that the SNPs showing a stronger statistical evidence of association with an increased familial risk were rs2981582 in intron 2 of FGFR2, rs12443621 and rs8051542 within TNRC9, rs889312 in a region that contains the MAP3K1 gene, rs3817198 in intron 10 of lymphocyte-specific protein 1 (LSP1), and rs2107425 within the H19 gene.
In brief, GWAS analyze thousands of cases and controls (huge numbers are necessary to reach sufficient statistical power) in order to compare SNPs, which makes it possible to identify genetic variants associated with disease risk. More than 100 GWAS have related a slight increase in cancer risk [51]. In breast cancer, at least 18 variants have been identified, with a 1.1- to 1.5-fold increase in risk. Interestingly, these SNPs might not only provide useful information on the risk of breast cancer, but they could also be linked to specific molecular subtype of breast cancer, such as FGFR2-RS2981582 and TNRC9-RS3803662 with positive estrogen receptor [52] and rs8170 in chromosome 19p13 with negative estrogen receptors [53]. However, the clinical impact of these approaches is not clear, and this could illustrate how techniques are developing faster than knowledge. For example, a 1.2-fold risk with one of these variants is comparable to that of delaying the age of the first pregnancy to more than 35 years [51]. In addition, these approaches give no information about other complex factors that can affect risk, and there are no data on the possible effects of combining two or more variants. Consequently, large research consortia are essential if a high number of cases and controls are to be attained. Finally, direct access to some GWAS via the Internet can generate anxiety and dangerous misinterpretation of genetic risk.
Breast Cancer Genomics Based on Biobanks
Endeavors to discover genes’ contributions to intricate diseases, such as cancer, require new study designs that incorporate an efficient use of population resources and modern genotyping technologies. There are two approaches used for the study of breast cancer, both of which incorporate the use of biobanks. One uses a cancer registry as a source of case information, which is then linked to a biobank on blood DNA. The biobank also provides samples from matched controls. After genotyping, clinical data are retrieved from hospital records, and the results can be presented for genotype-specific cancer risks or similarly for genotype-specific clinical and survival parameters. The second approach uses registered data on cancer in families or among twins. With defined groups of patients, paraffin tissue is collected by contacting the pathology departments of the hospitals where the patients were diagnosed. Tumor and healthy tissue are prepared and used for mutation, the loss of heterozygosity, or copy number analysis. In the era of whole-genome genotyping technologies, the importance of well-characterized sample sets cannot be overemphasized. Samples, rather than technologies, limit the rate of gene discovery in complex diseases [54].
Linking Genotype to Phenotype in Breast Cancer
Women with germ line BRCA1 or BRCA2 mutations are estimated to have a 45–70 % risk of breast cancer by age 70 years [55–58]. The identification of BRCA1 and BRCA2 mutations was a major step in personalizing breast cancer risk assessment, screening, and risk reduction strategies. Studies are ongoing to determine whether or not certain subgroups of BRCA mutation carriers may be at a higher risk for breast cancer. It has been proposed that certain BRCA mutations may confer a differential risk of future breast cancer development, suggesting an important genotype–phenotype linking [59, 60].
In a recent kin-cohort study in Ontario, Risch et al. observed a trend of increasing breast cancer risk associated with increasing downstream location of BRCA1 mutation with a continuous linear trend and a 32 % increase in risk associated with each additional 10 % or 559 nucleotides of downstream distance [60]. Over the past few years, a considerable effort has been made to characterize genetic abnormalities in cancer, the general idea being that tumor genotyping would be valuable in defining cancer phenotypes. In a previous study, we showed that it was possible to delineate subsets of breast tumors according to specific combinations of DNA amplifications [61]. The present work allowed us to extend the phenotypic description to prognostic significance. We show here that some of the markers tested presented prognostic significance in specific subsets of patients. This was particularly evident for MDM2 amplification and p53 mutations, which showed a strong prognostic value in the N2 subset of patients, or for the amplification of CCND1, EMS1, and FGFR1 in N1 patients. During the course of this study, we also made some observations that suggest the existence of correlation clustering in other patient subsets, such as MYC in patients under 50 years or MDM2 in ER1 patients (data not shown).
Our data constitute an attempt to delineate tumor subsets according to their genotypic specificity. Knowing the complexity of the genetic rearrangements in breast cancer, the nine events studied here probably correspond to a small portion of the genes involved in tumorigenesis. Genotyping of breast tumors will involve the analysis of an ever-larger number of parameters and sorting of the significance of complex combinations. Because different combinations of genes or genetic anomalies may bear a meaning in different populations of patients, the analysis of specific phenotypic subsets will be necessary, thus leading to an increase of the number of comparisons. This will require the analysis of very large cohorts of patients (several thousand) and consequently the use of high-throughput analytical methods [62] in association with statistical tools, especially devised for multiple-comparison analyses.
Genomics Landscape of Breast Cancer and Comprehensive Atlas of Breast Cancer Genomes for Various Applications
A pilot genome-wide sequencing exertion on breast cancers identified a total of 1,137 somatically mutated genes from 11 breast cancers, with an average of 52 non-synonymous mutations per sample. Using gene mutation incidence as the principal criterion, 140 genes were identified as candidate cancer genes that require further assessment to confirm their functions as causal contributors to tumorigenesis [63, 64]. These studies also portrayed the genomic landscape of human breast cancer that consists of a few repeatedly mutated gene “mountains” and a huge number of rarely mutated (usually <5 %) gene “hills” [64].
Besides the detection of novel candidate genes, more recent studies have delineated new aspects of breast cancer exomes. Interrogating luminal-type breast cancer genomes with clinical data revealed that somatic mutations in TP53 signaling pathway, DNA replication, and mismatch repair are associated with aromatase inhibitor resistance [65]. Determination of clonal frequencies by deep sequencing provided new insights into the initiating events of TNBCs [66]. Massively parallel paired-end sequencing technologies enable whole-genome detection of gene rearrangements at the DNA sequence level [67]. An analysis on 24 breast cancers revealed more than 2,000 gene rearrangements, enriched with tandem duplications [68]. Analysis of breast cancers across a variety of subtypes revealed that luminal B and HER2-enriched breast tumors harbor many more structural rearrangements when compared to the luminal A subtype. However, no repeatedly recurrent rearrangements have been discovered in breast cancer by earlier studies except for the MAGI3–AKT3 gene fusion detected in 4 % (9 out of 257) of breast cancers [69].
Like all cancer types, breast cancer progression is thought to be a dynamic multistep Darwinian evolution process. Independent mutations arise in a stepwise fashion, of which those conferring selective advantages promote cell proliferation and clonal expansion [70]. Through deep whole-genome sequencing of 21 breast cancers and analysis of subclonal genetic alterations, Nik-Zainal et al. proposed a model for clonal evolution that many molecular aberrations accumulate in dormant cell lineages before final expansion of the most recent common ancestor, which triggers diagnosis [71]. Integrative breast cancer studies aim at developing new definition of breast cancer subtypes with better prognostic and predictive values. A cluster analysis integrating copy number and gene expression profiles of ~2,000 breast cancers suggested a novel classification system [72]. A recent multiplatform study on hundreds of breast cancers revealed subtype-specific pattern in numerous tumor characteristics including gene mutations, microRNA expression, DNA methylation, copy number changes, and protein expression. Moreover, in whole-exome sequencing of more than 500 tumors, this study also revealed almost all repeatedly altered pathways (PI3K/AKT, TP53, RB) in breast cancer [73].
The quickly evolving sequencing technologies generate massive genomic data at an increasing rate with reduced cost. In recent years in particular, large-scale analyses of cancer genomes have produced a prosperity of information, which greatly expanded our knowledge on human breast cancer, summarized in Table 4.2. The launch of comprehensive cancer genome projects including the Cancer Genome Project (CGP) [78], the Cancer Genome Atlas (TCGA) [79], and the International Cancer Genome Consortium (ICGC) [80] facilitates the compilation of an encyclopedic catalogue of the genomic changes involved in cancer. Whole-genome sequencing studies enable observation of genetic alterations earlier undetectable by protein-coding sequence screens, including mutations in noncoding regions and large rearrangements. Primary breast cancers were reported to harbor ~7,000–10,000 somatic point mutations per genome [65, 69, 81] in which tens to hundreds reside in the protein-coding regions [63, 64, 69, 77], as well as up to hundreds (average 20–50) of somatic structural variants [65, 68, 69, 75, 81]. The minimum number of mutations necessary for tumorigenesis has been estimated to be around 5–6, according to incidence modeling of solid tumors such as breast and colorectal cancers, and this number would be smaller in leukemia and childhood cancers [82]. However, recent systematic mutational screens of cancer genomes suggested a higher number of causal gene mutations in each tumor (range 10–20 genes) [63, 83].
Table 4.2
Summary of genome sequencing studies of breast cancer
Study | Cancer type | Sequencing target | T/N pairsa | Findings |
---|---|---|---|---|
Stephens et al. [74] | Breast | Protein kinome (518 genes) | 25 | Identified diverse patterns of somatic mutations in breast cancer |
Wood et al. [64] | Breast, Colorectal | All RefSeqGene (18,191 genes) | 11 + 24 per tumor type | The first sequencing effort of all coding regions in cancer genomes. Identified 280 candidate genes and revealed the mutation landscape of breast and colorectal cancer genomes |
Shah et al. [70] | Breast (metastasis) | Whole genome whole transcriptome | 1b | Demonstrated single-nucleotide mutational heterogeneity and mutational evolution in breast tumor progression |
Stephens et al. [68] | Breast | Whole genome | 24 | The first genomic screen for somatic rearrangements in tumor samples. Revealed the genome landscape of somatic rearrangements in breast cancer |
Ding et al. [75] | Breast | Whole genome | 1c | Indicated that metastasis may arise from a minority of cells within the primary tumor |
Edgren et al. [76] | Breast | Whole transcriptome | 4 | Discovered novel fusion genes (e.g., VAPB–IKZF3) with potential functional role in breast cancer |
Nik-Zainal et al. [71] | Breast | Whole genome | 21 | Identified distinct nucleotide substitution signatures, observed localized hypermutation, and constructed a model of breast cancer evolution |
Ellis et al. [65] | Breast | Whole genome (n = 46), | 77 + 240 | Identified novel significantly mutated genes (e.g., GATA3, TBX3, ATR, RUNX1, LDRAP1, STMN2, AGTR2, SF3B1) in luminal breast cancer and revealed pathways (e.g., TP53, DNA replication, MMR) associated with aromatase inhibitor response |
whole exome (n = 31) | ||||
Shah et al. [66] | Breast | Whole genome (n = 15), | 65 | Revealed mutations and structural alterations with clonal frequency and suggested involvement of cytoskeletal gene mutations in breast cancer |
whole exome (n = 54) | ||||
Stephen et al. [77] | Breast | Whole exome | 100 + 250 | Revealed multiple mutation signatures of breast cancers and identified novel driver mutations (e.g., AKT2) |
Banerji et al. [69] | Breast | Whole genome (n = 22), | 108 + 235 | Identified novel recurrent mutations in CBFB and a recurrent fusion gene MAGI3–AKT3 |
whole exome (n = 130) | ||||
TCGA [73] | Breast | Whole exome | 507 | Revealed molecular subtype-specific patterns of mutations and identified novel candidate genes |
Distinguishing the driver mutations from passengers cannot be accomplished by analyzing genetic data alone but requires functional validation of the cancer-relevant activities. Since most functional assays are relatively labor- and time-intensive, prioritization of the genes for functional studies presents a great challenge in cancer genomic data interpretation. Several measurements have been adopted to identify the most promising driver mutations. First, analyzing the ratio of non-synonymous mutations to synonymous mutations of a given gene would indicate whether the mutations have been under positive selection during tumor development, thus a higher than expected ratio always suggests driver mutation [84, 85]. Second, assessment of the mutation prevalence in genes also identifies drivers that contribute to cancer if they are highly unlikely to be mutated by chance [63]. Third, several tools have been employed to predict the effect of non-synonymous single nucleotide variants on protein function based on phylogenetic conservation and physical considerations (e.g., Sorting Intolerant From Tolerant (SIFT) [86], Polymorphism Phenotyping (PolyPhen) [87], Panther [88], MutationTaster [89], etc.). Last but not least, as the number of pathways involved in cancer is much smaller than that of cancer genes and a variety of mutations in multiple cancer genes from the same pathway would likely to have similar pathological effects [90], evaluation of the combined prevalence of somatic alterations at the pathway level provides strategies for identification of cancer-associated processes [91, 92].
In the past few years, comprehensive mutation interpretation implementing most, if not all, of these measurements has been introduced into cancer genome analyses. For example, Carter and her colleagues developed a computational pipeline for cancer-specific high-throughput annotation of somatic mutations (CHASM), which takes a total of 49 predictive features into account for driver identification [93]. Another example is a package for determination of mutational significance in cancer (MuSiC), designed by Dees et al. MuSiC is the first software suite that integrates clinical data with coverage data and database references to identify drivers from large mutational discovery sets [94].
Although many tools can help to prioritize the candidates of interest for downstream analyses, only the evidence from functional assays and biological studies can fully credential a candidate gene as a bonafide cancer gene. It is clear from cancer genome resequencing efforts that not all cancer genes are mutated at high prevalence. On the contrary, despite conferring selective advantage, the vast majorities of cancer genes are not frequently mutated and are therefore difficult to identify through sequencing of a limited number of samples. In order to discover these infrequent driver mutations, systematic screens of large cohorts of patients are required. For example, it was estimated that 500 tumor samples of a particular tumor type are needed in whole-exome sequencing studies to get an ~80 % detection power of genes with ~3 % true mutation frequency [80].
Classification of Breast Cancer Based on Genome Profile
Gene expression profiling has proven to be a useful and reliable tool for classifying breast cancers into subgroups that reflect different histopathological characteristics as well as differential prognostic outcome. It has been suggested that estrogen receptor-negative and estrogen receptor-positive breast cancers can be subdivided into Her-2 positive basal-epithelial-like, normal breast-like, and luminal-like [95]. The potentially different origins of the tumor cells may signify distinct pathways of tumorigenesis and differences in the clinical course of the disease. Germ line mutations in the BRCA1 and BRCA2 genes together account for a significant portion of hereditary breast cancers. They have been shown to leave a characteristic imprint on the panel of genes expressed by the tumors [96], with BRCA1-dependent tumors exhibiting a transcriptional profile similar to the basal subtype of tumors [97]. These findings suggest that the cellular origin of BRCA1- and BRCA2-mutation-positive tumors may differ or that these tumors traverse down separate pathways in their progression toward malignancy [96]. Furthermore, the molecular subclassification of non-BRCA1/2 familial breast cancers into homogeneous subgroups underscores the potential differences in cellular origin and/or disease progression due to the presence of multiple diverse underlying genetic alterations, which is reflected in the phenotype of the tumors [98].
The diversity of breast cancer has been acknowledged for decades, but recent technological advances in molecular biology have given detailed knowledge on how extensive this heterogeneity really is. Traditional classification based on morphology has given limited clinical value, mostly because the majority of breast carcinomas are classified as invasive ductal carcinomas, which show a highly variable response to therapy and outcome [99]. The first molecular subclassification with a major impact on breast cancer research was proposed by Perou and colleagues where the tumors were subdivided according to their pattern of gene expression [95, 100]. Five groups were identified and named luminal A, luminal B, basal-like, normal-like, and HER-2-enriched subgroups. These intrinsic subgroups have been shown to be different in terms of biology, survival, and recurrence rate [95, 97]. The molecular subgroups have been extended to also include a sixth subgroup, which has been named the claudin-low group, based on its low expression level of tight junction genes (the claudin genes) [101].
Different methods for the assignment of individual tumors to its molecular subgroup are proposed, each based on the expression levels of different sets of genes [97, 102, 103]. The agreement between methods on how to classify individual tumors are not optimal, and how to establish more robust single sample predictors is actively debated [104–107].
Aneuploidy is the presence of an abnormal number of parts of or whole chromosomes and is one feature that clearly separates cancer cells from normal cells. This was proposed as being important in cancer nearly a century ago by Theodor Boveri. With array-based comparative genomic hybridization (aCGH), a genome-wide profile of the copy number alterations in the tumor can be obtained. These patterns are related to the molecular subtypes with distinct differences in the number of alterations between the subtypes [108–111]. These copy number alterations (CNAs) alter the dosage of genes and highly influence the level of expression [112, 113]. This frequently affects the activity in oncogenes and tumor suppressor genes, and in this way CNAs are important for the carcinogenic process.
CNAs in tumors are a result of deregulated cell cycle control and of DNA maintenance and repair [114]. Different patterns of copy number alterations have been identified with distinct differences; simplex profiles are characterized by few alterations and complex genomic profiles have extensive changes [115]. Complex genomic rearrangements are areas with high-level amplifications and have prognostic value in breast cancer even when they do not harbor known oncogenes, suggesting that the phenotype of defect DNA repair may be associated with more aggressive disease [115, 116]. Alterations in the expression pattern are caused by changes at the genomic level, and a robust classification of breast cancer for clinical use should probably take these more into account. Changes at the genomic level include point mutations, changes in copy number, and epigenetic events. These are characteristics that enable and drive carcinogenesis together with tumor-promoted inflammation [117].
Risk Assessment and Prognosis by Genetic Test: Market Players in Genomics-Based Personalized Diagnosis, Prognosis, and Therapy
Risk Assessment
Hereditary alterations have been associated with 10–15 % of all breast cancer cases; however, disease etiology in the majority of women appears to be sporadic, lacking a momentous family history. Because sporadic breast cancer may be influenced by a number of lifestyle and environmental factors as well as common low-risk variant in a number of genes, numerous models have been developed in an endeavor to quantify individualized breast cancer risk:
The Gail model measures risk based on patient age, age at menarche, number of prior breast biopsies, age at first live birth, and number of first-degree relatives affected by breast cancer [118].
The Claus model estimates risk based on the number of affected relatives and their respective age at diagnosis [119].
The BRCAPRO model calculates risk of developing breast cancer based on the probability of carrying a BRCA1 or BRCA2 mutation [120].
These models have been widely used to predict risk and direct patient care, but each model has limitations, because no model accounts for the spectrum of risk factors influencing breast cancer. For example, the Gail model considers only first-degree relatives without regard to age at diagnosis or presence of ovarian cancer, thus potentially underestimating genetic risk. The Claus and BRCAPRO models only consider family history, potentially underestimating risk in women with other risk factors [121]. In addition, these models were developed 10–20 years ago, when incidence of breast cancer in the general population was lower than it is today, and use of lower baseline risk estimates may contribute to an underestimation of recent risk [122]. More recent models, such as the Tyrer–Cuzick model, utilize family history, endogenous estrogen exposure, and presence of benign disease to model breast cancer risk [123], but contributions from other factors such as mammographic breast density, weight gain, steroid hormone levels, and susceptibility genes have not been incorporated [124].
The discovery of the BRCA1 and BRCA2 genes advanced risk assessment in families affected by hereditary breast and ovarian cancer, but identification of molecular markers associated with increased breast cancer risk in patients without a family history of breast cancer has remained far more challenging. Without a strong family history, linkage approaches involving large pedigrees such as those used to identify BRCA1 and BRCA2 are not applicable. Sporadic breast cancer is not usually associated with other cancers, such as ovarian or male breast cancer, and unlike BRCA1-positive carcinomas, which exhibit specific histological characteristics, sporadic breast cancer cases comprise a vast array of phenotypes. Early approaches to identify sporadic breast cancer susceptibility genes compared the frequency of DNA variants in genes from molecular pathways believed to be involved in breast cancer development between cases with disease and healthy matched controls. An association study using candidate genes recently identified caspase 8 (CASP8) as a low-risk susceptibility gene where the major (H) allele of the D302H polymorphism had a protective effect on the development of breast cancer [125]. Despite success in identifying CASP8, candidate gene approaches have not been widely successful in identifying additional breast cancer susceptibility genes [126].
Whole-Genome Approaches
Candidate gene approaches are rapidly giving way to genome-wide association studies (GWAS), which evaluate a dense array of genetic markers representing common variation throughout the genome. Completion of the human genome sequence and subsequent identification of single-nucleotide polymorphisms (SNPs) now permits millions of informative SNPs across the genome to be assayed simultaneously. GWAS are useful for mapping genes of interest to small, localized regions of the genome and for detecting the effects of common (>5 % minor allele frequency) alleles on disease risk [127]. Moreover, GWAS are performed without a priori knowledge of the underlying genetic defect(s), which may be advantageous since many genes identified through whole genome approaches were not previously suspected to influence the disease under investigation [128].
Recent GWAS have identified a number of loci that appear to be associated with breast cancer susceptibility. For example, the fibroblast growth factor receptor 2 (FGFR2), mitogen-activated protein kinase kinase kinase 1 (MAP3K1), lymphocyte-specific protein 1 (LSP1), and trinucleotide repeat-containing 9 (TNRC9/LOC643714) genes, along with a 110-kb region of chromosome 8q24, have been associated with breast cancer in large studies involving thousands of subjects [29, 30]. Associations with other chromosomal regions—2q35, 5p12, 6q22, and 16q12—also have been reported [36, 43, 50]. Further analysis has shown that allelic variation at FGFR2, TNRC9, 8q24, 2q35, and 5p12 is associated with physiological characteristics of breast tumors, such as ER status [36, 43, 129], and specific FGFR2, MAP3K1, and TNRC9 variants may interact with BRCA1 and BRCA2 mutations to increase breast cancer risk [130].
Despite recent success in identifying genetic determinants of breast cancer, susceptibility alleles identified through GWAS are believed to account for only ~5 % of breast cancer risk [131]. If future studies are to be successful in identifying additional low-risk susceptibility alleles and low-frequency, highly penetrant variants [132], interactions between genes and environmental exposures must be assessed [133], and methods must be developed to evaluate mechanisms by which DNA variants in intronic or intergenic regions contribute to disease. As risk associated with susceptibility alleles may vary between racial/ethnic populations due to differences in frequency, patterns of disequilibrium, and interactions with environmental factors [5, 30, 36], sufficiently powered genetic studies in women from various ethnic groups are needed to improve risk reduction strategies for all women.
Direct-to-Consumer Testing
New susceptibility variants identified by GWAS have not yet been incorporated into genetic tests with beneficial clinical utility for breast cancer patients. However, genetic analysis and risk assessment are available commercially through direct-to-consumer (DTC) testing. A number of for-profit companies offer personal genetic information based on DTC tests—the largest and most recognized companies include 23andMe, deCODEme, Navigenics®, and Knome®, Inc. (Table 4.3). For a fee of $99 to $99,500 consumers provide a blood, buccal, or saliva sample for targeted SNP analysis or whole-genome sequencing. Genetic information provided to the consumer varies greatly among companies, from trivial facts such as earwax type and ancestry information to information on risk for disease [134, 135]. Although DTC tests epitomize “personalized genomics” by providing consumers with individual genotypes, critics note that the clinical utility of such tests is limited and often incongruent with marketing claims. Because information on family history and environmental exposures is usually not accounted for, DTC risk estimates may not be sufficiently accurate to enable consumers to make appropriate medical decisions [136, 137].
Table 4.3
Leading direct-to-consumer genetic testing companies
Company | Headquarters | Website | Price (USD) | Genetic counseling | Breast tumor susceptibility variants |
---|---|---|---|---|---|
23andMe | Mountain View, CA | $399 | No | 2 SNPS | |
deCODEme | Reykjavik, Iceland | $985a | Yes | 11 variantsb | |
Knome | Cambridge, MA | Customc | Yes | DNA sequence | |
Navigenics | Foster City, CA | $999d | Yes | Unknown |
The majority of genetic risk assessments developed thus far focus on DNA variants; however, a new RNA-based signature has been developed for noninvasive breast cancer screening using peripheral blood samples. Although based on a small number of cases (n = 24) and controls (n = 32), a subset of 37 genes in the assay correctly classified 82 % of patients [138]. Despite a relatively high misclassification rate, DiaGenic (www.diagenic.no) has since developed this gene expression signature into a clinical screening tool, currently available only in India as BCtect™ India.
Breast Cancer Personalized Prognostics
Pathological Characterization of Breast Cancer
Human breast carcinomas exhibit diverse pathological characteristics that are associated with different clinical outcomes and thus are routinely used to guide treatment options. Accordingly, an accurate definition of prognosis is dependent on the ability to detect and quantify differences in tumor attributes, such as rates of proliferation and propensity to metastasize. Routine tumor evaluation currently includes (1) histopathological classification; (2) grade determination; and (3) quantification of tumor size, surgical margin status, and lymph node involvement.
Histopathological characterization, based on microscopic cellular morphology, classifies breast carcinomas into common subtypes (ductal or lobular carcinoma), which tend to have similar prognoses [139]; or less common forms such as mucinous, tubular, and papillary (favorable prognosis) [140]; or inflammatory breast cancer (poor prognosis) [141]. Increasing tumor size has long been associated with poor prognosis [142], but improved mammographic detection of smaller tumors has decreased the prognostic utility of tumor size [143]. Presence of positive surgical margins has been associated with local recurrence, but only 27 % of patients with extensively positive margins will have recurrent disease [144, 145].
Likewise, the Nottingham Histological Score, widely used for assessing histological grade, is clinically useful for stratifying patients into low-risk (low-grade disease, 95 % 5-year survival) and high-risk (high-grade disease, 50 % 5-year survival) groups [146, 147], but the reliability of breast tumor grade in predicting survival is hampered by subjectivity associated with its assessment [148]. Axillary lymph node status is the most reliable predictor of survival, differentiating women who are likely to have >90 % 5-year survival (patients with negative nodes) from those who are likely to have <70 % survival (women with nodal metastasis) [149]. Although these clinical attributes are currently the standard of care for breast cancer patients, many are imprecise in their ability to accurately predict outcomes.
Immunohistochemistry
Molecular markers have the prospective to provide additional prognostic information to supplement traditional pathological assessments for disease management in breast cancer patients. As mentioned above, traditional immunohistochemistry (IHC) markers routinely used in the classification of breast cancer include ER, PR, and HER2. Tumors positive for ER and PR expression frequently have low cellular proliferation rates, tend to exhibit lower histological grade, and are associated with more favorable prognosis [150]. ER and PR expression also is useful for identifying patients who will likely benefit from hormonal therapy, as women with ER- and PR-negative breast cancer do not gain a survival benefit from antiestrogen tamoxifen [151].
The HER2 gene is a member of the epidermal growth factor receptor family with tyrosine kinase activity and is amplified at the DNA level and/or overexpressed in 15–25 % of breast cancers. Carcinomas with amplified/overexpressed HER2 exhibit high histological grade and usually have a poor prognosis [152, 153]. Some patients with positive HER2 status (15–20 %) are eligible to receive trastuzumab, a monoclonal antibody targeting HER2, in combination with standard chemotherapy [154].
Rigorous clinical studies have shown that evaluating ER, PR, and HER2 status provides additional prognostic information beyond that normally achieved by histological assessment alone. For example, breast carcinomas that are ER negative and PR negative and do not have HER2 overexpressed (triple negative) are marked by aggressive behavior, but because women with triple-negative disease are not eligible for tamoxifen or trastuzumab treatment, they usually have relatively low long-term survival [155]. Other markers such as nuclear antigen Ki67 are not routinely used to guide treatment selection, but hold great promise for monitoring the effectiveness of neoadjuvant chemotherapy and predicting recurrence-free survival [156–158].
Individual estimates of outcome using clinical and pathological characteristics of breast tumors, including age, menopausal status, comorbid conditions, tumor size, number of positive lymph nodes, and ER status, have been incorporated into a computer program, Adjuvant! Online (www.adjuvantonline.com/index.jsp), which is available over the Internet as a decision aid for patients and their physicians [159]. The program estimates the efficacy of endocrine therapy and chemotherapy as well as overall and disease-free survival in a user-friendly format that effectively brings patients into the decision-making process regarding personalized treatments.
Although IHC analysis of ER, PR, and HER2 is widely used in the pathological evaluation of breast tumors, additional molecular signatures involving multiple genes and/or proteins are desperately needed to more accurately classify tumors and guide treatment selection. Recently, a multigene IHC-based test known as MammoStrat® (Applied Genomics, Huntsville, AL; www.applied-genomics.com/mammostrat.html) was developed to classify breast cancer patients into low-, moderate-, or high-risk categories for disease recurrence (Table 4.4) [162]. MammoStrat® uses conventional paraffin-embedded tissue to assay five markers by IHC:
Table 4.4
Selected molecular diagnostic tests for breast cancer
Test | Company | Assay type# | Number of genes/proteins | Classification | Study |
---|---|---|---|---|---|
Breast Bioclassifier™ | University genomics | qRT-PCR | 55 | Tumor subtype | Perou et al. [100] |
Therapeutic guidance | |||||
MammaPrint™ | Agendia | Microarray | 70 | Prognostic | van’t Veer et al. [160] |
Therapeutic guidance | van de Vijver et al. [161] | ||||
MammoStrat® | Applied Genomics | IHC | 5 | Prognostic | Ring et al. [162] |
MapQuant DX™ | Ipsogen | Microarray | 97 | Tumor grade | Sotiriou et al. [163] |
Loi et al. [164] | |||||
Oncotype DX™ | Genomic Health | qRT-PCR | 21 | Prognostic | Paik et al. [165] |
Therapeutic guidance | Paik et al. [166] | ||||
Rotterdam signature | Veridex | Microarray | 76 | Prognostic | Wang et al. [167] |
Tumor protein p53 (TP53)—known to play a central role in cell cycle regulation
HpaII tiny fragments locus 9C (HTF9C)—involved in DNA replication and cell cycle control
Carcinoembryonic antigen-related cell adhesion molecule 5 (CEACAM5)—aberrantly expressed in some cancers
N-myc downstream-regulated gene 1 (NDRG1)—may function as a signaling protein in growth arrest and cellular differentiation
Solute carrier family 7 (cationic amino acid transporter, y + system), member 5 (SLC7A5)—mediates amino acid transport
The MammosStrat® test may have utility for predicting patient outcomes but currently requires five separate slides (one slide per antibody), which has the potential to show variability in staining intensity and scoring between patients.
Gene Expression Signatures and Disease Risk
Molecular profiles are now being used more frequently as clinical tools to determine treatment for certain groups of patients by categorizing them into low-risk and high-risk groups. The MammaPrint™ assay (Agendia, Amsterdam, The Netherlands; www.agendia.com) is a 70-gene signature developed using tumor tissue from young women (<55 years of age) with node-negative disease, who either developed distant metastasis or remained disease-free after 5 years [160]. Overall 10-year survival for the “poor-prognosis” signature is ~55 %, while 10-year survival in women with the “good-prognosis” signature is 95 %. The probability of being free from distant metastasis after 10 years is 51 % for the poor prognosis and 85 % for the good prognosis profile [161].
A second group of researchers subsequently developed a 76-gene profile (Rotterdam signature) that could identify breast cancer patients at high risk for distant recurrence. The signature could identify patients who developed distant metastases within 5 years when traditional prognostic factors were considered (hazard ratio 5.55, 95 % CI 2.46–12.5) and could predict metastasis in both premenopausal and postmenopausal patients [167].
The gene expression signatures outlined above were refined from global expression profiling experiments involving thousands of genes and flash-frozen tumor specimens. An alternative approach relied on an extensive literature search to identify candidate genes (n = 250) believed to be involved in disease development based on known function. Gene expression levels were assayed in 447 patients with ER-positive, node-negative breast cancer to identify a small subset of 16 genes (plus five reference genes) amenable to analysis by real-time PCR (RT-PCR) on RNA isolated from formalin-fixed, paraffin-embedded (FFPE) specimens. The resulting 21-gene signature, known as Oncotype DX® (Genomic Health, Redwood, CA; www.genomichealth.com/), provides a probability of recurrence score for women with early-stage (stage I or II), ER-positive, node-negative breast cancer and categorizes patients as low, intermediate, or high risk.
In validation studies using patients from the National Surgical Adjuvant Breast and Bowel Project (NSABP) clinical trial B-14 who received tamoxifen, the probability of distant recurrence at 10 years for the three risk categories was low risk, 6.8 % (95 % CI 4.0–9.6); intermediate risk, 14.3 % (95 % CI 8.3–20.3); and high risk, 30.5 % (95 % CI 23.6–37.4). Recurrence scores also correlated significantly with relapse-free interval and overall survival [165]. In a subsequent study, Oncotype DX™ was used to assess the benefit of adjuvant chemotherapy in ER-positive, node-negative patients. Because the highest benefit was observed in patients with high-risk scores, while women with low-risk recurrence scores did not benefit from chemotherapy [166], Oncotype DX™ may be useful in guiding treatment options in ER-positive, node-negative patients.
Clinical trials of the MammaPrint™ and Oncotype DX™ assays are currently in progress. In the Microarray In Node negative Disease may Avoid ChemoTherapy (MINDACT) trial, 6,000 node-negative women will be assigned to treatment groups based on risk stratification by traditional clinical–pathological factors (Adjuvant! Online) and the MammaPrint™ molecular signature [168]. Patients classified as low risk by both methods will not receive chemotherapy, while those considered high risk for relapse by both methods will be given the opportunity to receive adjuvant chemotherapy. Patients of primary interest, those with discordant results, will be randomized to treatment based on either Adjuvant! Online or MammaPrint™ to determine which test is more effective in defining treatment in node-negative patients.
The Trial Assigning Individualized Options for Treatment (TAILORx) is examining whether hormone receptor-positive patients with an intermediate Oncotype DX™ risk recurrence score benefit from chemotherapy. The trial is recruiting 10,000 hormone receptor-positive patients with HER2-negative and lymph-node-negative disease. Treatment will be based on the risk recurrence score as follows: <10, hormone therapy alone; >26, hormone and chemotherapy; and intermediate scores, randomization to either hormone therapy alone or to hormone therapy and chemotherapy. The goal is to integrate Oncotype DX™ into the clinical decision-making process and refine the utility of the assay in clinical practice [169].
Molecular signatures have improved the ability to predict outcome and identify breast cancer patients who would most likely benefit from systemic therapy, thus providing an additional layer of personalized medicine. However, no current molecular signature is 100 % accurate, and 5–10 % of patients now classified as low risk are likely to relapse. Furthermore, current classification systems were developed to predict only short-term (<5 years) outcomes; thus, there is a need to develop signatures that identify patients with protracted disease progression who may benefit from prolonged therapy [170]. Although outcome prediction tends to be similar between gene expression signatures, overlap among genes comprising the signatures is relatively low, suggesting that these profiles assess common biological pathways but have not identified the actual genes driving tumor behavior and outcome [171].
Pharmacogenomics of Breast Cancer
Pharmacogenomics in breast cancer assesses the effect of inherited genomic variation on patient response or resistance to treatment. Genetic variability is commonly measured at the DNA level in the form of chromosomal alterations or DNA sequence variants (Table 4.5). Conversely, somatic genomic changes (DNA variants and gene expression profiles) in breast tumors can influence rates of apoptosis, cell proliferation, and DNA damage repair, which may have direct effects on response to treatment and survival. To be most effective, personalized medicine must incorporate information from innate genetic variation as well as somatic mutations in diseased tissue [183].
Table 4.5
Selected genetic polymorphisms affecting response to therapy in breast cancer patients
Treatment | Gene | Variant | Functional change | Response to treatment | Study |
---|---|---|---|---|---|
Chemotherapy | |||||
Doxorubicin | CBR3 | 11G > A | Decreased enzyme activity | Hematological toxicity | Fan et al. [174] |
Anthracyclines | MnSOD | Ala16 | Higher levels of reactive oxygen species | Decreased mortality | Ambrosone et al. [176] |
MPO | —463GG | Higher levels of reactive oxygen species | Decreased mortality | Ambrosone et al. [176] | |
GSTP1 | 313A > G | Altered drug transport | Hematological toxicity | Zárate et al. [177] | |
MTHFR | 1298A > C | Altered drug metabolism | Non-hematological toxicity | Zárate et al. [177] | |
Endocrine therapy | |||||
Tamoxifen | CYP2D6 | *3, *4, *5, *10, *41 | Reduced function/nonfunctional enzyme | Poor clinical outcome | Schroth et al. [178] |
Goetz et al. [179] | |||||
Aromatase inhibitors | CYP19A1 | Cys264, Thr364 | Decreased enzyme activity | Reduced benefit | Ma et al. [180] |
Radiotherapy | |||||
TP53 | Arg72Pro, PIN3 | Decreased apoptosis | Risk of telangiectasia | Chang-Claude et al. [181]. | |
Targeted therapy | |||||
Trastuzumab | HER2 | Heterodimer | Prevents disruption by trastuzumab | Poor response to treatment | Lee-Hoeflich et al. [182] |
Endocrine Therapy
Estrogens play an important role in the etiology of breast cancer by stimulating growth and proliferation of ductal epithelial cells in the breast; thus, the status of the estrogen receptor in breast carcinomas provided one of the earliest avenues for personalized medicine. Fortunately, hormone receptor-positive tumors usually are responsive to agents such as tamoxifen that block the function of estrogen. Tamoxifen is a potent antagonist of the ER with inhibitory effects on tumor growth that has become the gold standard for endocrine treatment of estrogen receptor-positive breast cancer in premenopausal and postmenopausal women [184]. Tamoxifen is associated with side effects such as blood clots, stroke, and increased risk of endometrial and uterine cancer, but 5-year use of tamoxifen has been shown to reduce risk of cancer recurrence by ~50 % [185]. For most patients, the benefit of using tamoxifen for hormone receptor-positive disease outweighs the risk of serious side effects; however, a small subgroup of hormone receptor-positive patients who carry specific variants in the cytochrome P450 2D6 (CYP2D6) gene do not benefit from tamoxifen. The CYP2D6 gene is a key enzyme in the metabolism of tamoxifen to its active metabolite endoxifen. Several DNA variants in CYP2D6 result in poor metabolism of tamoxifen and lower levels of endoxifen [186]. Patients who carry reduced-function or nonfunctional CYP2D6 alleles have been found to derive inferior therapeutic benefit from tamoxifen and thus are at increased risk of breast cancer recurrence [178] or have significantly shorter disease-free survival than noncarriers [179]. Studies are underway to determine the utility of CYP2D6 genotyping for making clinical decisions about tamoxifen and the potential to optimize breast cancer therapy [187, 188].
Alternate forms of directed antiestrogen therapies do exist for patients with hormone receptor-positive breast cancer, including aromatase inhibitors that block the production of estrogen and compounds such as fulvestrant (Faslodex®) that downregulate and degrade the ER protein. Aromatase inhibitors such as anastrozole (Arimidex®), letrozole (Femara®), and exemestane (Aromasin®) target cytochrome P450 19 (CYP19A1 or aromatase), an enzyme involved in estrogen synthesis in peripheral organs. Premenopausal women with functional ovaries do not receive aromatase inhibitor therapy because first- and second-generation aromatase inhibitors did not effectively suppress estrogen levels and because decreased estrogen levels in peripheral tissues could be counteracted by increased estrogen synthesis in the ovaries [189]. In postmenopausal women, aromatase inhibitors are well tolerated and improve both disease-free and recurrence-free survival [190–192]. Similar to CYP2D6, the Cys264 and Thr364 variants in aromatase are associated with decreased activity and lower levels of immunoreactive protein, which may contribute to variation among patients in response to aromatase inhibitor therapy [180]. Although directed endocrine therapies provide treatments specific for patients with hormone receptor-positive breast cancer, factors such as menopausal status and innate genetic variability may alter the effectiveness of treatment.
Treatment for HER2-Positive Breast Cancer
Therapies directed at the HER2 protein provide a second avenue of targeted treatment for some patients with breast cancer. Trastuzumab (Herceptin®, Genentech, South San Francisco, CA; www.gene.com/) is a humanized monoclonal antibody that binds to the extracellular domain of the HER2 protein, blocking tumor cell growth. Trastuzumab is the current standard of care in adjuvant therapy for HER2-positive breast cancer, effective as a single agent or in combination with chemotherapeutics for the 20–25 % of patients with HER2-positive cancer [193]. However, many patients with HER2-positive disease do not derive tangible benefit from trastuzumab. Given that the cost per patient for trastuzumab ranges from $20,000 to $80,000 per year with the potential for significant adverse side effects [194], a more precise classification of HER2-positive patients who will derive benefit from trastuzumab and improved understanding of how amplification and/or overexpression of HER2 contribute to aggressive tumor biology are critical to improving patient treatment. The major oncogenic unit in HER2-positive breast cancer appears to be a heterodimer between the HER2 and epidermal growth factor receptor-3 (HER3) proteins, where HER3 functions as a necessary dimerization partner for HER2 to achieve full oncogenic signaling potential [195].
Recent studies have shown that HER2/HER3 heterodimers promote cellular proliferation in both in vitro and in vivo models, suggesting that HER3 may be an important therapeutic target in HER2-positive patients [182]. Pertuzumab has been shown to bind to the dimerization arm of HER2, blocking HER2/HER3 heterodimerization and attenuating growth of solid tumors in model systems [196]. Thus, combining pertuzumab with trastuzumab may augment therapeutic benefit by blocking HER2/HER3 signaling. Monogram Biosciences (South San Francisco, CA; www.monogrambio.com/) has developed the commercially available HERmark™ test to measure total HER2 levels and HER2 homodimers in FFPE tissue and is developing a VeraTag™ assay to quantify levels of HER2/HER3 heterodimers. These assays may allow patients with HER2-positive breast cancer to receive the most efficacious combination of new drugs targeting HER2.
Chemotherapeutics
Chemotherapy involves use of chemical agents as part of a systemic treatment targeting proliferative cancer cells. Adjuvant chemotherapy is used to reduce risk of recurrence after primary therapy in women with localized breast cancer and to provide palliative care in patients with advanced (metastatic) disease. In contrast, neoadjuvant chemotherapy is normally used to shrink moderate- to large-sized breast carcinomas prior to surgical resection, which permits use of less aggressive surgical options, including breast conservation, and may be useful in guiding longer-term treatment based on tumor response to specific drug combinations [197]. Obviously, the ability to predict which patients will benefit from adjuvant therapy and identify who will respond favorably to neoadjuvant regimens would provide an additional level of personalized care.
Gene Expression and Chemotherapeutic Agents
Gene expression profiling has been used to study the biological responses of human breast carcinomas to optimize chemotherapeutic treatments. Cell lines derived from luminal and basal epithelium have been observed to respond differently to agents commonly used in chemotherapy, such as doxorubicin (DOX) and 5-fluorouracil (5FU). In culture, luminal cell lines show low levels of expression for genes regulating cellular proliferation and the cell cycle, while basal cell lines tend to repress genes involved in cellular differentiation when exposed to DOX and 5FU [198]. Similarly, different molecular subtypes of breast cancer defined by gene expression profiling respond differently to preoperative chemotherapy, with basal-like and HER2-positive subtypes being more sensitive to paclitaxel and doxorubicin than luminal and normal-like cancers [199].
Expression signatures also have been used to predict clinical response of breast cancer patients receiving either cyclophosphamide–adriamycin or epirubicin–5FU as part of their adjuvant chemotherapy regimen [200] and to distinguish primary breast tumors that are responsive or resistant to docetaxel chemotherapy [201]. These observations further highlight the vast amount of molecular variability among breast carcinomas and emphasize the need for additional molecular signatures to more effectively guide treatment.
DNA Variation and Chemotherapeutic Agents
Clinical responses in breast cancer patients to commonly used chemotherapeutic agents vary considerably, from optimum therapeutic response to partial (beneficial) response to severe adverse events. Variation at the DNA level in an increasing number of genes is now known to affect the pharmacokinetics and pharmacodynamics of many chemotherapeutic drugs [202, 203], thus influencing toxicity and patient response. To improve the safety and efficacy of current treatments, therapies could be tailored to individual patients based on their genetic makeup [204]. For example, the carbonyl reductase 3 (CBR3) gene contributes to the reduction of DOX to doxorubicinol, a less potent metabolite, and the extent of metabolism is believed to be a source of variability in doxorubicin chemotherapy. The 11G > A variant (rs8133052) in CBR3 has been shown to influence tumor tissue expression of CBR3 and is associated with interindividual variability in clinical outcomes. Women with the 11GG genotype experience greater leukocyte toxicity and are less likely to show a reduction in tumor size than women carrying 11AA [174].
A number of chemotherapeutics generate reactive oxygen species that function by damaging DNA and triggering the apoptotic cascade. Women carrying variants in genes associated with oxidative stress, such as manganese superoxide dismutase (MnSOD), catalase (CAT), and myeloperoxidase (MPO) that result in higher levels of reactive oxygen species, tend to have better overall survival than women with genotypes associated with lower levels of reactive oxygen species when treated with chemotherapy [176]. Due to the large number of drug-metabolizing enzymes and drug transporters containing polymorphisms that affect chemotherapy-related toxicity and treatment outcomes in breast cancer patients, improved pharmacogenetic information is needed to identify individuals at risk for toxicity and poor response.
Genomics in Clinical Practice
Recent developments in the clinical arena are indicative of the emerging importance of personal genomics in the prevention, surveillance, and treatment of breast cancer. Professional organizations such as the American Society of Clinical Oncology (ASCO) have issued recommendations on the use of molecular markers for guiding therapy and determining prognosis in breast cancer patients [175].
CA 15-3 and CA 27.29 (assays to detect circulating MUC-1 antigen in peripheral blood)—contribute to decisions regarding therapy for metastatic breast cancer in conjunction with diagnostic imaging, history, and physical examination
Carcinoembryonic antigen (CEA)—contributes to decisions regarding therapy for metastatic breast cancer in conjunction with diagnostic imaging, history, and physical examination
ER/PR—should be measured on every primary invasive breast cancer to identify patients most likely to benefit from endocrine therapy
HER2—should be measured on every primary invasive breast cancer at diagnosis or recurrence to guide trastuzumab therapy
Urokinase plasminogen activator (uPA) and plasminogen activator inhibitor 1 (PAI-1)— measured by ELISA on fresh or frozen tissue for determining prognosis in newly diagnosed, node-negative breast cancer patients
Oncotype DX®—in newly diagnosed patients with node-negative, ER-positive breast cancer, can be used to predict risk of recurrence in women treated with tamoxifen
Large cancer centers such as Massachusetts General Hospital and Memorial Sloan-Kettering Cancer Center are now embracing the importance of genomics in clinical practice, recently implementing policies to routinely assay a number of breast cancer-related genes: vakt murine thymoma viral oncogene homolog 1 (AKT1) and HER2 at Memorial Sloan-Kettering, phosphatase and tensin homolog (PTEN) and TP53 at Mass General, and phosphatidylinositol 3-kinase, catalytic, alpha (PIK3CA) at both institutions [205]. As genomic medicine becomes an integrated part of health-care delivery, use of personalized genomics in the clinical treatment of breast cancer will increase.
Genomic Studies of Breast Cancer Initiation, Progression, and Metastasis
Breast cancers progress through multiple genomic and epigenomic steps. However, the evolutionary process is unlikely to be the result of a linear and more or less constant rate of successive genomic and epigenomic aberration accretion. It seems more likely that cancer progression varies between individuals and over time within an individual. The breast progenitor cell in which the tumor arises likely determines the spectrum of aberrations needed to enable progression. Evidence for this comes from studies showing that the spectrum of genomic aberrations accumulated is strongly influenced by the normal progenitor cell type in which the cancer arises [108, 206]. In general, the events associated with early aspects of breast tumor appear to be epigenomic in nature, wherein stepwise DNA methylation changes enable escape of telomerase-negative epithelial cells from proliferation barriers [207]. This leads to proliferation in the absence of telomerase and culminates in a period of high genome instability owing to checkpoint deregulation [208, 209] and/or entry into telomere crisis when telomeres become critically short [206, 210]. This barrier is highly effective but not perfectly so. As a consequence, most cells become genomically unstable and die. The increase in genome instability during breast tumor progression is illustrated in Fig. 4.1. Rarely, a single cell accumulates genomic or epigenomic alterations that reactivate telomerase and confer a proliferative advantage. This cell might be considered the tumor initiation cell and will have multiple characteristics that appear “stem cell like.” The extent to which this is related to normal stem cells remains unclear. However, it is likely that the genomic characteristics of this cell—both transcriptional and genomic—will be reflected in subsequent progeny. This may explain why tumor genomes appear to evolve relatively slowly after telomere crisis and why metastases that develop years after immortalization usually retain the genomic characteristics of the primary tumor from which they were derived [212].
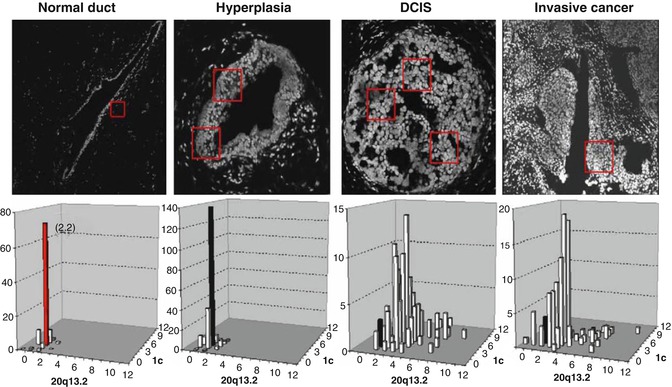
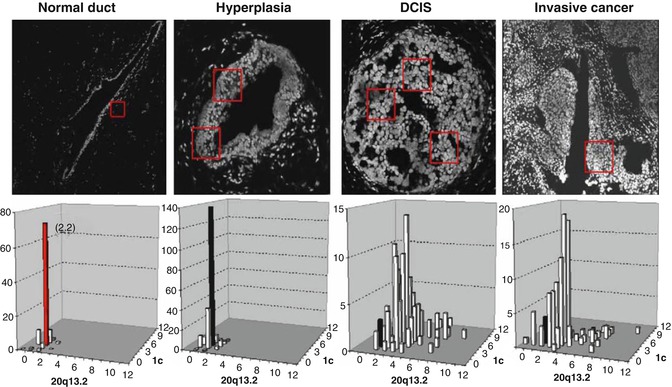
Fig. 4.1
Genome instability measured during breast tumor progression using FISH [206]. Histological sections depicting stages of evolution are illustrated above. Bivariate measures of genome copy number at the centromere of chromosome 1 and at chromosome 20q13. The onset of instability at the DCIS stage of evolution is apparent (Reprinted from Korkola and Gray [211]. With permission from Elsevier)
That said, not all breast tumors progress this way. A recent analysis of cancer progression termed Sector-Ploidy-Profiling (SPP) demonstrates that breast tumor evolution may evolve as a single major clonal subpopulation or as multiple clonal subpopulations [213]. The latter model may explain why a small percentage of metastatic cancers do not resemble the primary tumor from which they were derived. Figure 4.1 also shows that the cells that survive telomere crisis remain genomically unstable. Thus, tumors are likely to consist of a large number of cells that are not faithful genomic representations of the tumor-initiating cell and are likely to be biologically compromised. These cells are likely to be much more sensitive to treatment than the tumor-initiating cells from which they were derived. This may partially explain why breast tumors initially respond well to treatment but fail to exhibit a durable response.
Various Genomic Signatures
Unsupervised molecular classification identified three major and robust groups of breast cancers that differ in the expression of several hundred to a few thousand genes. These include basal-like breast cancers, which are negative for ER, progesterone receptor (PR), and human epidermal growth factor receptor 2 (HER2); low histological grade ER-positive breast cancers (also called luminal A); and high-grade, highly proliferative ER-positive cancers (luminal B). Several smaller and less stable molecular subsets (such as normal-like, HER-2-positive, and claudin-low) have also been proposed but are less consistently seen and are distinguished by substantially smaller molecular differences [95, 104]. Importantly, among the various molecular subsets, one group, the luminal A class that includes low-grade ER-positive cancers, stands out with a very favorable prognosis with or without adjuvant endocrine therapy. The other groups have worse but rather similar prognosis [95, 102].
If one understands these close associations between clinical phenotype, molecular class, and prognosis, it is no longer surprising that comparing gene expression profiles of breast cancers that recurred (mostly the ER-negative and the high-grade, ER-positive cancers) and those that did not (low-grade, ER-positive cancers) in the absence of any systemic therapy (or after antiestrogen therapy alone in the case of ER-positive cancers) yields a very large number of differentially expressed genes. The relative position of individual genes in a rank-ordered gene list varies greatly, but the consistency of the gene list membership is fairly high across various datasets [214].
Functional annotation indicates that the majority of these prognostic genes are proliferation-related genes, and the remainder are mostly ER-associated and, to a lesser extent, immune-related genes [215–217]. Because these genes function together in a coordinated manner in the regulation and execution of complex biological processes, such as cell proliferation, or originate from a particular cell type, such as immune cell infiltrate, many of these prognostic genes are also highly coexpressed with one another. It is therefore expected that a large number of nominally different prognostic signatures can be constructed that will all perform equally well. For example, a particular gene may be highly significantly discriminating in two datasets but it is ranked 5th among the most discriminating genes in one dataset (based on P-value or fold difference) but only 35th in another dataset (which is still very high, considering the thousands of comparisons!).
In multivariate prediction model building, the top few informative features are usually combined, and genes are added incrementally to increase the predictive performance. However, because many of the genes are highly correlated with each other, adding genes lower on the list yields less and less improvement in the model as a result of lack of independence. Therefore, the gene in question will be included in a predictor developed from the first dataset (because it is ranked as 5th) and will work well on validation in the second dataset; but if a new predictor were to be developed from the second dataset, this gene may not be included in the predictor (because it is ranked 35th).
These three features of the breast cancer prognostic gene space—the large number of individually prognostic features, the unstable rankings, and the highly correlated expression of informative genes—explain why it is easy to construct many different prognostic predictors that perform equally well even if they rely on nominally different genes in the model. However, this does not mean that all published prognostic gene signatures are equally ready for clinical use. Before adoption in the clinic, a molecular diagnostic assay has to be standardized, the reproducibility within and between laboratories and stability of results over time have to be demonstrated, and its predictive accuracy has to be validated in the right clinical context, preferably in multiple independent cohorts of patients. Most importantly, clinical utility implies that the assay improves clinical decision making and complements or replaces older standard methods, which in turn leads to better patient outcomes. Few published prognostic predictors have met these criteria [175, 218].
The predictive performance of a multivariate model largely depends on the number of independent informative genes included in the model, the magnitude of differential expression of the informative genes, and the complexity of the background. Different clinical prediction problems show different degrees of difficulty. From the discussion above, it should be apparent that prediction of ER status, histological grade of breast cancer, or better or worse prognosis associated with these clinical phenotypes should be relatively easy when considering all breast cancers together and that such prediction can therefore yield predictors with good overall accuracy. Indeed, prognostic gene signatures developed for breast cancer in general or for ER-positive cancers tend to have good performance characteristics [161, 165, 167, 217]. However, the first-generation prognostic signatures share some limitations. Because these were invariably developed by analyzing all subtypes of breast cancers together, they tend to assign high risk category to almost all ER-negative cancers (which are almost always high grade), even though a substantial majority of these cancers have good prognosis [219, 220]. Similarly, the good- and poor-prognosis ER-positive cancers, as assigned by gene profiling, tend to correspond to the clinically low-grade/low-proliferation versus high-grade/high-proliferation subsets, respectively. This strong correlation between prognostic risk as predicted by gene signatures and routine clinical variables, such as histological grade, proliferation rate, and ER status, limits the practical value of these tests.
Efforts are under way to develop simple multivariate prognostic models that use routine pathological variables (such as ER, histologic grade, and HER2 status), and these could eventually rival the performance of the first-generation prognostic gene signatures [221, 222]. However, standardization of the pathological assessment of breast cancer and reducing the interobserver variability remain an important challenge. Predicting clinical outcome, such as prognosis or response to chemotherapy, within clinically and molecularly more homogeneous subsets (such as triple-negative breast cancers or high-grade, ER-positive cancers) would be highly desirable. Unfortunately, these prediction problems seem to be more difficult [223, 224]. It seems that fewer genes are associated with outcome in homogeneous disease subsets and the magnitude of association is modest when currently available datasets are analyzed. This leads to predictors that are specific for a particular dataset from which they were developed. These prediction models are fitted to the dataset and rely on features that have no or limited generalizability. This means that they fail to validate when applied to independent data or may demonstrate only nominally significant predictive value (i.e., they may predict outcome slightly better than chance). Also, the discriminating value may not be substantial enough to be clinically useful [225, 226]. For example, if the good-prognosis group has a recurrence rate of 30 % compared with 50 % in the poor-risk group, these may be significantly different, but the risk of recurrence in the good-risk group is still too high to safely forego adjuvant chemotherapy.
Genomic Mechanism of Breast Cancer Dormancy and Recurrence
Factors that determine the length of the dormancy period remain unclear [227]. Current data have led to various experimental models that address the phenomenon of tumor dormancy. It appears that dormant cancer cells can persist either by completely withdrawing from the cell cycle (mitotic arrest) or by continuing to proliferate at a slow rate that is counterbalanced by cell death [228, 229]. These two types of dormancy are not mutually exclusive; both forms of latency could coexist in the entire population of disseminated tumor cells (DTC) of a particular cancer patient.
Single-Cell dormancy
In the single-cell dormancy model, isolated tumor cells detached from the primary tumor arrive at the future metastatic organ and enter a prolonged state of mitotic arrest. This model of arrested apoptosis contrasts with the micrometastatic dormancy model, in which proliferation in micrometastatic foci is counterbalanced by cell death. Speculations about the metabolic status of minimal residual disease (MRD) during dormancy are not yet sufficiently investigated. Cell cycle regulation mechanisms are highly complex, with an assortment of stimuli interacting at numerous cell cycle checkpoints to determine the proliferative status. The presence of tumor cell dormancy due to a growth arrest of cancer cells is supported by evidence that within tissues where primary tumors are developing or tissues that harbor disseminated cells and have a functional vasculature, tumor cells are found to be in a nonproliferative mode [230–232]. In numerous studies, dormant cancer cells have been demonstrated to be in a G0–1 arrest; this was linked to negative staining for proliferation markers (e.g., Ki67, PCNA) [233, 234]. Dormant tumor cells may gain a survival advantage by blocking the receptors for tumor necrosis factor-related apoptosis-inducing ligand (TRAIL), which would result in arrested apoptosis. Two exemplary mechanisms of TRAIL-receptor blocking have been described and may be of relevance to dormant tumor cells. TRAIL receptors in cancer cells can be blocked by osteoprotegerin, an important member of the tumor necrosis factor receptor superfamily [235, 236]. Interestingly, bone marrow stromal cells from breast cancer patients secrete enough osteoprotegerin to inhibit apoptosis in vitro [237]. More recently, c-Src (a tyrosine-specific kinase involved in breast cancer progression) was demonstrated to support cancer cell survival in the bone marrow microenvironment by conferring resistance to TRAIL [238].
Micrometastatic Dormancy
In contrast to dormancy due to mitotic arrest, dormancy of a micrometastasis seems to be caused by a balance of cell proliferation and apoptosis, such that the tumor does not increase in size. This constant balance is regulated by proangiogenic proteins and angiogenic inhibitors produced by tumor and stromal cells, as well as immunologic, hormonal, or other microenvironmental switches [239]. According to Naumov et al., a failure to activate the angiogenic switch can maintain a group of cancer cells in a dormant state [240]. Indraccolo et al. reported that a short-term perturbation in the tumor microenvironment, in the form of a transient angiogenic burst, could suffice to interrupt tumor dormancy [239]. Genetic data support the interpretation that minimal residual cancer might be divided into “active” and “dormant” groups, in which an advantageous mutation is acquired shortly before a highly aggressive metastatic clone appears [241]. At present, there is no definite answer to the question of which model best represents tumor dormancy in breast cancer. Hussein and Komarova hypothesized that indolent breast cancers might fit into the single-cell dormancy model, while more aggressive diseases are linked to the micrometastatic dormancy model [229]. Indeed, in a series of experiments, Barkan et al. demonstrated that more aggressive basal-type cell lines, such as MDA-MB-231, proliferated readily, while estrogen receptor (ER)-positive MCF7 remained in a state of mitotic arrest, potentially linking the dormancy type associated with arrested growth with the less aggressive disease phenotype [242].
Genes driving recurrence may be encoded in the gene expression profile of primary tumors. Transcriptomic analysis of a variety of primary tumors by Ramaswamy and colleagues identified a 17-gene signature present in a wide range of human primary tumors that predicted metastasis and poor clinical outcome [243]. Although the genomes of clinically similar breast cancers are remarkably different, some regions of the genome are recurrently aberrant. The number of genes mapping to regions of significant abnormality is remarkable. In fact, correlative analyses of genome copy number and gene expression indicate that 10–15 % of the entire genome is deregulated by recurrent genome copy number abnormalities [108, 109]. Analysis of CGH data suggests the existence of at least three general classes—those with “simple” genomes displaying relatively few genome aberrations, those with “complex” genomes displaying many aberrations, and those displaying high-level amplification [109, 244]. It is likely that these subtypes may be caused by differences in DNA repair defects. For example, complex genomes typically carry p53 mutations while simple genomes do not.
CGH profiles for tumors from BRCA1 carriers also display high genome complexity, although the spectrum of recurrent abnormalities differs between sporadic and heritable breast cancers. In particular, tumors from BRCA1 mutation carriers typically display recurrent aberrations at chromosomes 3 and 5 that sporadic tumors do not have [245]. Relatively few recurrent mutations have been found in breast cancers. High-frequency somatic mutations (i.e., present in >3 % of reported cases) include PIK3CA, TP53, CDH1, CDKN2A, and AKT1 (http://www.sanger.ac.uk/genetics/CGP/cosmic). However, almost 200 others have been reported as recurrently mutated but at lower frequency [63]. Prevalent germ line mutations that contribute to breast cancer genesis involve BRCA1, BRCA2, CHEK1, and TP53. The mechanisms by which low-level copy number abnormalities contribute to cancer pathophysiology have not yet been determined definitively. However, we have suggested that these deregulate transcription of large numbers of genes that collectively increase metabolic activity [109]. The contributions of regions of amplification at 8p11–12, 8q24, 11q13, 12q13, 17q11–12, 17q21–24, and 20q13 as somewhat better understood since each encodes a known oncogene (e.g., FGFR1, MYC, CCND1, MDM2, ERBB2, PS6K, and ZNF217, respectively).
However, substantial evidence is now emerging that demonstrates that multiple genes in each region of amplification contribute to the pathophysiology of the disease. Functionally important coamplified genes demonstrated so far include LSM1, BAG4, and C8orf4 at 8p11–12 [246]; MYC and PVT1 at 8q24 [247]; CCND1 and EMSY at 11q13 [248]; and ERBB2 and GRB7 at 17q21 [249]. Recent studies also suggest that amplifications of regions that are well separated in the normal genome are not independent events. For example, coamplification of regions at 8p11–8p12 and 11q12–11q14 [250] or 8q24 and 17q21 [251] have been reported to contribute collectively to breast cancer pathophysiology. These interactions may be just the tip of the iceberg since dozens of genes have been identified as overexpressed as a result of recurrent, high-level amplification and most have not been functionally assessed [109]. Recurrent mutations, although relatively infrequent in breast cancer, provide particularly strong evidence of functional importance. Bringing all of these observations together, recent integrative analyses of homozygous deletions, high-level amplification, and recurrent mutations suggest that genomic deregulation of pathways involving ERBB2, EGFR, and PI3K signaling and DNA topology is particularly important in breast cancer genesis and progression [252].
Epigenomic alterations also contribute to breast cancer pathophysiology [253, 254]. Targeted and genome-wide analyses of promoter region methylation in breast cancer have shown that genes with known or suspected tumor suppressor function including BRCA1, CCND2, CDKN2A, RARβ, and RASSF1A [255, 256] and members of the WNT signaling pathway [257] are recurrently downregulated by hypermethylation. Consistent with this, genes found to be recurrently mutated in large-scale genome analysis studies are frequently targets of hypermethylation, and hypermethylation is usually mutually exclusive from genetic changes [258]. At the chromatin level, modification via acetylation and/or phosphorylation directly modifies estrogen receptor alpha and other steroid hormone receptors superfamily in breast cancer, thereby modifying ligand sensitivity and hormone antagonist responses [259]. Chromatin remodeling also has been implicated in growth and metastasis. In particular, SATB1 has been reported to be a genome organizer that tethers multiple genomic loci and recruits chromatin-remodeling enzymes to regulate chromatin structure and gene expression. Overexpression of this “master chromatin regulator” appears to drive breast cancer progression by upregulating metastasis-associated genes and downregulating tumor-suppressor genes [260].
Various Genomic Approaches and Their Outcome in Breast Cancer Research
To facilitate understanding of the scope of epigenetic modifications that occur in normal and cancer cells, a range of gene-specific or genome-wide technological approaches have been developed. We present an overview of recent technological developments and discuss the merits and the limitations of these approaches with respect to studies on cancer cells.
DNA Methylation
DNA methylation is the first identified epigenetic mechanism of gene regulation. The initial methods of detection of methylation were restricted to the quantitation of total 5-methylcytosine content by high-performance liquid chromatography (HPLC) or high-performance capillary electrophoresis (HPCE) [261] and the study of DNA methylation of selected sequences using restriction enzymes that can distinguish between methylated and unmethylated recognition sites in genes of interest [262]. For restriction-enzyme-based methods, incomplete restriction-enzyme digestion and the limitation of enzyme-recognition sites restricted their extensive application. These technical limitations caused delay in advances in the cancer epigenetic field in contrast to the rapid development in cancer genetics field. The development of a bisulfite-conversion technique that reproducibly changes unmethylated cytosines to uracil but leaves methylated cytosines unchanged [263] was a key development that drastically sped up progress in the field.
Several sensitive DNA methylation detection techniques (summarized in Table 4.6) were developed upon the basis of bisulfite conversion, including bisulfite sequencing, methylation-specific PCR (MSP), combined bisulfate restriction analysis (COBRA), and so on [263–265]. Among these technologies, MSP is the most popular and powerful method for DNA methylation detection, which needs limited amounts of DNA material [264]. Since MSP is a gel-based assay, it cannot provide quantitative information and is subjective. Several real-time methylation-specific PCR methods, such as bisulfite treatment in combination with MethyLight™ [266], quantitative multiplex MSP (QM-MSP) [267, 268], or pyrosequencing [269], have been developed and used in DNA methylation studies, which have improved features to detect minimal amounts of aberrant DNA methylation in a quantitative manner.
Table 4.6
Technologies for assessing and profiling DNA methylation and histone modifications
Technology | Description | Study |
---|---|---|
Gene–specific detection of DNA methylation | ||
Bisulfite sequencing | Bisulfite-converted DNA is PCR-amplified to enrich the target templates. The purified DNA templates are subjected to sequencing analysis directly or after cloning into plasmid | Fraga and Esteller [261] |
Methylation-specific PCR (MSP) | This technique takes advantage of the altered sequence of bisulfite-converted unmethyated and methylated DNA for designing primers, which can amplify DNA in a methylation-state-specific manner
![]() Stay updated, free articles. Join our Telegram channel![]() Full access? Get Clinical Tree![]() ![]() ![]() |