Background
Osteosarcopenia is the concurrent occurrence of osteoporosis and sarcopenia which may be worsen with advancing age . Several clinical or research tools have been developed to identify these two conditions including measurements of bone mineral density (BMD) and muscle strength . These techniques are used to avoid devastating sequelae of these conditions including but not limited to bone fracture and falls . The current clinical bone and muscle biomarkers can detect those at risk but may still fail to provide information regarding high-risk activities . This lack of predictability in the clinical setting may be the reason why the number of falls and fractures are significantly on the rise particularly among older adults .
In addition to the consequences of age-related deterioration in bone and muscle, these conditions are costly both for individuals and the society . For instance, on average, the direct cost of osteoporotic fractures was reported between $5000 and $6500 billion in United States, Canada, and Europe per year. This estimate excludes indirect costs . Moreover, recent estimate of hospitalization cost for those with sarcopenia has been $40.4 billion (average $260/person) which was shown to be race-, gender-, and age-specific in the United States . Apart from the cost, unfortunately, patients with osteosarcopenia may be at greater risk of fall, fracture, hospitalization and significantly greater mortality rate compared with the patients with osteoporosis or sarcopenia alone . The significance of age-related conditions is further pronounced by the WHO initiative to call this the decade of healthy aging .
Another challenge that investigators may face with these patients is their limited ability to perform high-risk diagnostic experiments due to fragile bone and muscles. Thus, development of noninvasive techniques and clinical tools complementing imaging techniques are required to avoid any unintended falls or fractures during diagnoses of those with either osteoporosis and sarcopenia or both . Imaging techniques such as DXA scans are routinely used as a clinical and noninvasive tool for osteoporosis patients. However, current imaging techniques do not provide information about more dynamic scenarios such as walking or lifting. More importantly, they do not provide any information about bone and muscle function and their interactions during various activities. To address these shortcomings, numerous musculoskeletal (MSK) and computer models have been developed . These noninvasive methods can provide powerful tools that can potentially and safely predict the consequences of osteosarcopenia .
This chapter reviews the role of personalized MSK models for predicting the risk of falls and fracture in patients with osteosarcopenia toward addressing the following questions: (1) can we predict high-risk activities that lead to falls and fractures during daily activities?, (2) In a given high-risk activity, which bone is more susceptible to fracture, and finally (3) can we identify the role of individual muscles and bone during high-risk activities to eventually develop preventive and protective methods against fall and fracture? In summary, this book chapter focuses on state-of-the-art biomechanical models to develop novel and complementary therapeutic approaches toward predictive tools to screen those with sarcopenia, osteoporosis or osteosarcopenia and their associations with fractures and falls.
Why personalized musculoskeletal modeling?
Clinical assessment of osteosarcopenia considers personalized measurements of patients including previous falls, timed up and go (TUG), handgrip strength, walking speed or BMD level . However, to predict risk of structural failure of bone, fracture and falls, measurements of each individual are compared with population-based studies, which may not precisely identify the consequences of those at risk of osteosarcopenia. However, there may be a strong association between these parameters such as fall and handgrip strength or BMD level and osteoporotic fractures . For instance, bone fracture assessment using the FRAX score has been developed as a useful and simple tool based on statistical analyses of a population using several risk factors such as BMD, smoking, and BMI . Nonetheless, such scores may not fully represent the ability of the bone to maintain structure and function for an individual during different daily activities .
Bone fracture depends not only on the bone quality, but the magnitude and its rate (time to peak) of the applied load during various activities. Fracture occurs when the applied load exceeds the bone strength at a particular location (i.e., Load Strength Ratio > 1). Bone strength varies under different loading conditions (quasistatic, cyclic loading or impact loads such as those during a fall) . Moreover, different individuals exert different loads on their bones in each activity, which can affect the Load Strength Ratio in a certain scenario. Thus, the risk of fracture is subject-specific in any given activity . Consequently, bone fracture analyses require a well-defined loading conditions (i.e., loading profile versus time) and bone strength . Loading conditions in turn require an accurate measure of the muscle contribution, as major load generating actuators. Overall, there is a complex interdependence between bone and muscle strength and the generated loads in them during various activities. An accurate calculation of this interdependence can help identify the high-risk activities and anatomical locations in each individual.
During daily activities, muscles, and bone work in concert. Smooth performance of a daily activity occurs because of proper activation of several muscles that can move the joints and bones in a right direction. This process applies mechanical loads via muscles and ligaments to the bones and joints while the whole body keeps its balance during a dynamic situation. However, at any given time, any internal (e.g., muscle weakness or lack of synergy) or external (e.g., trip or slip scenarios) perturbations could affect the balance thus leading to a likely fall or fracture. Moreover, following mechanical perturbation, the strategy in recovering from perturbations is subject-specific though some overall approaches have been identified involving the ankle or hip strategies . Thus, understanding underlying causes of fall and fracture in bone and muscle quality and their anatomical variations (both function and forms) should be considered in implementing personalized methodologies.
Personalized MSK models are powerful tools that have shown a promising pathway toward such an individualized prediction of muscle and bone loading due to internal (e.g., intraabdominal pressure) or external loading (e.g., ground reaction forces) to the body . In these modeling approaches, muscle and bone morphology and their quality are computationally quantified for each individual and a set of mathematical and physical equations are used to quantify the interaction between bone-muscle and how their function/form could lead to fall and fractures . Next, we will discuss how a personalized MSK modeling (a.k.a., computer-based, or biomechanical modeling) can be created and eventually help clinicians to better screen osteosarcopenia and predict consequences of such age-related conditions.
Personalized musculoskeletal modeling as a predictive tool
Prediction of high-risk activities leading to osteoporotic fractures
MSK models use physics-based models of human movement and can be used to predict the forces that cause the motion or vice versa. Let us consider Newton’s second law where F = m × a . F is the force on a mass ( m ) such as a body segment where it causes the mass to accelerate (i.e., a ). If we can assess forces on the human body (e.g., muscles, external forces, etc.), one can predict how the joints move ( a ) given the mass. Similarly, if we can measure joint motions ( a ), one can predict forces ( F ) that cause the joint motions. This simple explanation is the basis of MSK modeling using engineering principles.
Bone fracture occurs when mechanical load (L) exceeds its strength (S) or load/strength ratio (LSR) is greater than 1, i.e., LSR > 1. To better understand the risk of fracture, LSR needs to be quantified during daily activities for an individual. Several experimental and computational methods have been developed to measure these variables to identify those at risk of fracture . Mechanical crush testing of cadaveric specimens using material testing machines has been extensively used to quantify strength of bone for a variety of loading rates and can eventually help predict bone strength of others using correlation and regression analyses . Moreover, sensors placed on the intervertebral joint to measure disc pressure can be related to intervertebral loading . However, these methods are extremely invasive and are not practical for those with osteosarcopenia at risk of fracture and fall. On the other hand, image-based methods have been developed to predict bone strength and the risk of fracture, such as Quantitative computed tomography (QCT) and Dual-energy X-ray absorptiometry (DEXA) . These imaging techniques have also been combined with Finite Element Analysis (FEA), a stress analysis method to predict bone strength (FEA) . Though such methods are noninvasive, they are not free of side effects including high dose of X-rays and costs due to high computational and labor efforts to develop these complex models .
These imaging methods are still the best way to measure (or predict) bone strength in a noninvasive manner. Though, biomechanical loading experienced by bone cannot be predicted by only imaging techniques. It is because such a loading strongly depends on the type of a movement, joint motions, and external forces. In addition, most imaging techniques are conducted in stationary situation while most of daily activities are dynamic. MSK models enable us to obtain internal loading given joint motions and external loading, e.g., ground reaction forces (GRFs).
One of the most comprehensive biomechanical modeling studies was performed using the Framingham Heart Study (FHS) database, where MSK models were used to predict spinal loading and the risk of vertebral fracture at every intervertebral joint. A total of 250 individuals from the FHS database were recruited in the study . Subject-specific MSK models were developed by scaling a generic MSK model, and each model was adjusted for the major muscles in terms of their respective cross-sectional area, moment arms where QCT data was available, as well as the position of each vertebrae from X-ray images . Inverse dynamic and joint reaction analyses were performed in OpenSim to predict individuals’ muscle forces (for over 500 muscle fascicles) and all 17 interverbal joint across the spine for 126 different activities . In the meantime, the vertebral strengths were predicted based on Quantitative Computed Tomography-based Finite Element Analysis (QCT-FEA) models and then a regression analyses provided the strength of each vertebra ( Fig. 1 ) . QCT data provide 3D geometry models and BMD levels (associated with bone strength) then using a mathematical model, i.e., FEA, one can predict the stress/strain level of bone including its strength. Having both intervertebral joint loading (L) and their respective vertebral strength (S), the risk of fracture (load/strength or LSR) was predicted for every spinal level for each person performing all 126 activities. The results revealed high-risk activities due to maximum LSRs along the spine including: “ lateral bending with a weight in one hand (upper thoracic), holding weights with elbows flexed (lower thoracic) and forward flexion with weight (lumbar) .” The findings indicated that a wide range of asymmetric activities of weightlifting, carrying nonsymmetric postures should be evaluated in the future studies of vertebral fracture. Such outcomes from biomechanical modeling could eventually be used for clinical practice when proven in a longitudinal human study. Finally, these findings provide evidence on variety of high-risk activities and static postures that need to be scrutinized in more dynamic scenarios in the future to study osteoporotic vertebral fracture biomechanics. We envision that future studies of MSK modeling approaches can improve their usability so that they become a part of clinical practice. It should be noted that the protocols developed in this study can be used for other joints as a novel tool to predict a risk of bone fracture.
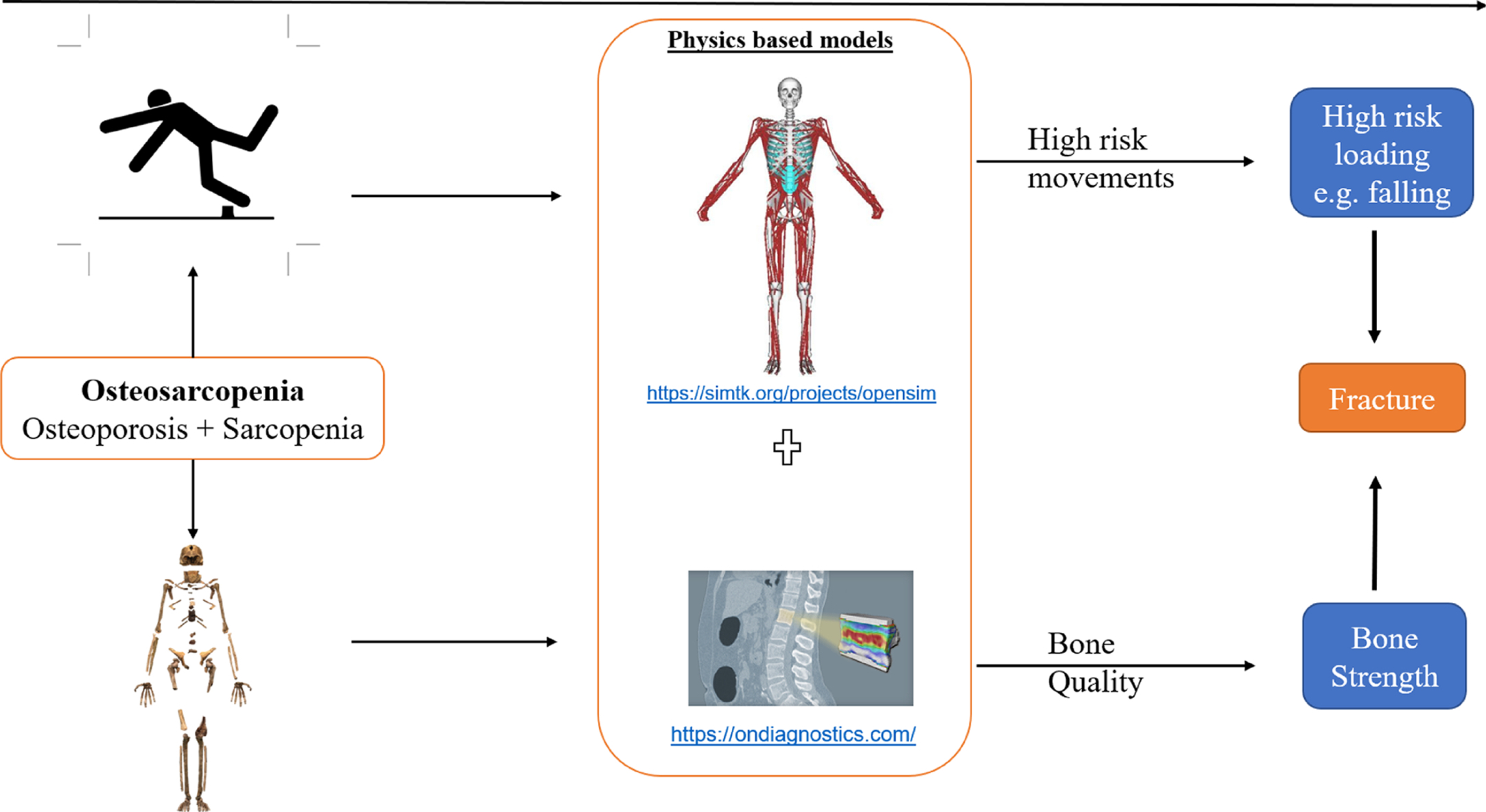
Prediction of risk of falls following sarcopenia
Patients with sarcopenia may suffer from future falls . Generally, falls occur when the body is unable to maintain its balance in a given activity. A fall is defined as an incidence in which an individual comes to a resting or lower position while muscles cannot prevent such unintentional movement (WHO definition). The musculoskeletal system is responsible for keeping the balance. Age-related conditions such as sarcopenia adversely affect muscle mass, strength, and possibly neural activities, affecting stability and thereby increasing the risk of falls and bone fractures. The clinical consequences of sarcopenia and its risk factors have been assessed using walking performance (e.g., gait speed), timed up and go (TUG) test, muscle strength and imaging techniques such as DEXA scans. One shortcoming regarding these measures is that they may not consider the role of high-risk activities that can lead to falls . Having information regarding the quality of muscles including muscle morphology, their activation patterns and strength would be valuable adjunct to predict sarcopenia’s adverse effects in addition to the current clinical tools .
A physical moment following a fall incidence, large mechanical loading is generated between the human body and environment—usually a hard surface—in which the person is in contact. Prior to or at the time of a fall, muscles and joints may respond differently compared to normal walking or sit to stand experiments which involve slow speed movements. Thus, the following question can be raised: what kind of activities can pose high-risk situations and how do individual muscles and joints respond for individuals during these activities?
To address such challenging questions, a holistic view of neuro-musculoskeletal system is required. Bioengineering methods utilizing MSK modeling can provide such a platform to give us insights regarding the high-risk situations—including perturbation-based or dual tasking scenarios —and muscle and joint contributions during these scenarios.
Currently, there are not many biomechanical studies where age-related conditions such as sarcopenia are considered . For instance, in the recent study on the role of sarcopenia on spinal loading, researchers used a MSK model approach . The authors virtually reduced the strength of certain spinal muscles to simulate age-related muscle parameters such as erector spinae’s strength. It was found that severe and very severe (36% and 24%, respectively) sarcopenia levels, led to an increase in spinal compressive load of up to 36% or 318N. These age-related changes in spinal loading increased muscle activities of up to 100% of their muscle strength . The effect of aging on spinal loading was simulated and revealed that spinal loading and even load-to-strength ratio could increase with aging (part of not published yet). These changes in muscle activation with aging could not only lead to increased risk of spinal fracture but also may affect the balance of the trunk and the whole body, which could further lead to subsequent falls.
In another recent perturbation-based study to compare healthy young and older adults, researchers found that there is no difference as a result of age on gait stability measures (i.e., margin of stability) following ipsi- and contra-lateral sway, belt acceleration/deceleration and even visual/auditory perturbations on medio-lateral direction . Moreover, a recent computational and experimental model of fall scenarios have focused on the role of lower limb muscles contribution to center of mass acceleration and how strategies of older versus younger individuals lead to loss of balance.
Again, such an investigation may have found no significant difference between muscle contributions to the overall center of mass acceleration due to advancing age. However, older people needed to take several steps (shorter and faster initial step in recovery) and then adjust their upper body flexion across the recovery stage versus those elders with single step recovery strategy . These insignificant outcomes for muscle contributions to the overall movements could be partially explained by the fact that participants were healthy in these two young and old groups. Though such MSK models are in their infancy, they may still suggest targeting possible interventions on neuromuscular control of certain muscles such as Gastrocnemius, Soleus, Vasti and Hip Abductors that can enhance the balance recovery performance . However, more detailed MSK models of those with osteosarcopenia are needed to better understand how muscles and joints will undertake recovery maneuvers following simulated fall risk as we age . Individualized MSK model can quantify the interaction between bone and muscle and adjust for individual changes with aging. Such a modeling approach could help better identity high-risk activities and develop personalized recovery strategies particularly for those with sarcopenia.
Prediction of consequences of osteosarcopenia
The modeling approaches discussed in previous sections for either osteoporosis or sarcopenia can be applied to study the consequences of osteosarcopenia given the relevant information on bone and muscle quality during human movement. The current literature provides enough evidence that using MSK modeling to predict the consequences of osteoporosis or sarcopenia are promising . For instance, epidemiological observation of spinal fracture is being explained by MSK modeling , while the same modeling approaches could provide bone fracture risk assessment including hip fracture risk assessment during exercises recommend for enhancing bone health .
Risk of falls were investigated by MSK models using wearables, lab-based motion analyses, or Artificial Intelligence (AI) or Machine Learning (ML) based models . As a result, using MSK modeling for diagnosis of MSK conditions seems to be plausible for those at risk of osteosarcopenia. As shown in Fig. 1 , regardless of type of MSK conditions (sarcopenia, osteoporosis), MSK models can consider quality of bone and muscles thus provide useful information on fall and fractures.
MSK models use several input variables including external loads, joint motions, and anatomical variations, to predict internal biomechanical variables including joint loadings, muscle activations . If one could adjust the MSK models to predict osteoporosis and age-related sarcopenia separately , the same MSK could provide insights as to whether the patient might suffer from both. Moreover, MSK models do not require an assumption of the underlying conditions while their consequences (fall or fracture) can be quantified. Later on, having access to a large database of MSK models and their outcomes as we have shown recently , other researchers can potentially develop novel thresholds for possible falls or fractures or categories by investigating muscle and bone interactions in a wholistic view of both conditions.
Again, MSK models are powerful tools that can be used for prediction of fall and fractures; however, more needs to be done in terms of changes over time in both bone and muscle quality and their interactions. One can develop a model of human at a given time, but MSK models ( Fig. 1 ) with longitudinal data can better evaluate people with osteosarcopenia. Mechanobiological models combined with MSK models are being developed to consider such changes over time, but still in their infancy .
Future directions
Development of advanced MSK models combined with imaging data and cutting-edge machine learning techniques will be a promising pathway to detect, screen and evaluate those at risk of osteosarcopenia. We envisage that current imaging scans routinely taken in the clinics can be repurposed and be input into MSK models such that the existing preventive measures can be improved. Such improvements could eventually reduce devastating consequences of those with osteosarcopenia and improve quality of life of older adults.
References
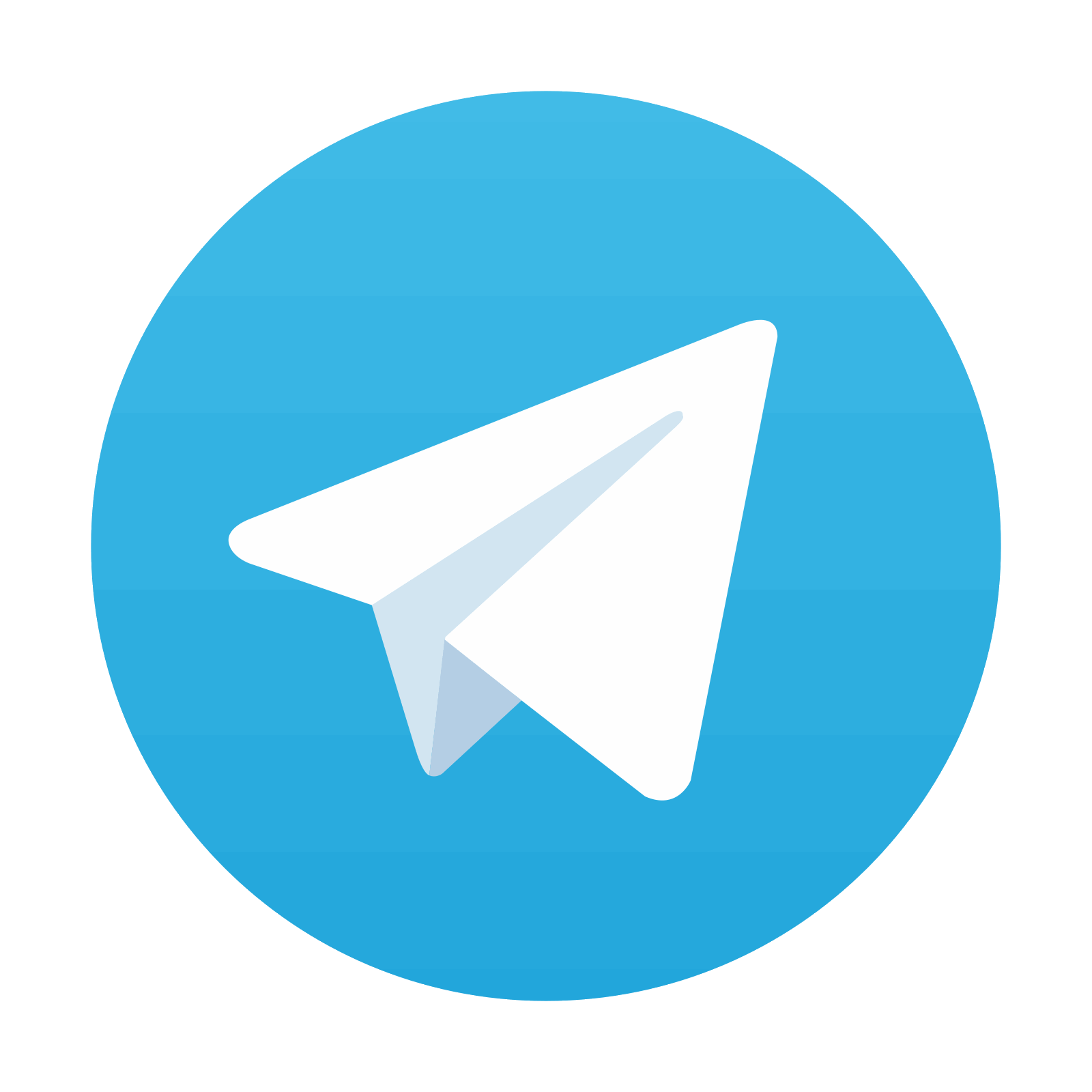
Stay updated, free articles. Join our Telegram channel

Full access? Get Clinical Tree
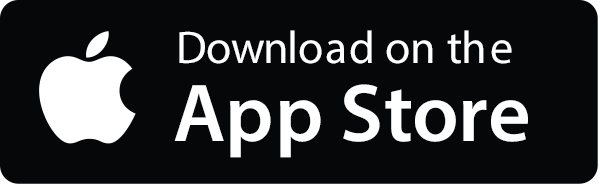

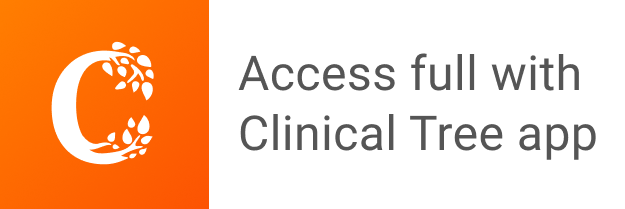