Fig. 7.1
Variant validation steps
7.2 Technical Validation of the Variant
The first role of the molecular biologist is to technically analyze the molecular result to insure the interpretation of the detected variant. This assessment is done with the help of bioinformaticians who have developed script processes to present the list of variants and exclude any false positive with a proper selection of filters.
In fact, the technology by itself can lead to large numbers of false-positive, especially in the case of variant detections with low level of mutated alleles. Many protocols try to identify variants suggesting identification of minor subpopulations at low level. The low detection threshold will increase the risk of false positives.
Biologists need to know the technological noise background, which is dependent on the method used. With next-generation (NGS) sequencing, the error rate of the Illumina technology is estimated to be <0.4 % with MiSeq or HiSeq technologies (Quail et al. 2012). The error rate with Ion Torrent sequencing has been estimated to 2 % (Quail et al. 2012). The recurrence of those errors is one of the ways to identify them. Some variants are located at the beginning or the end of reads and then can be trimmed in the analysis.
False positive can also be generated by the quality of the DNA and sample preparation. In the formalin-fixed paraffin-embedded (FFPE) specimens, noise can result from both low quantity of DNA and cytosine deamination to uracil (Do and Dobrovic 2012). Those artifacts are nonreproducible within replicates. Several strategies can be proposed from qualification of the DNA before any enrichments or dedicated treatment either during the extraction or the amplification.
For the NGS, the risk of false negative is related to the material and the kit design as well as the bioinformatics’ process. One of the main challenges in tumor analysis is the range of allelic frequencies. As the goal of the somatic sequencing analysis is also to be able to detect low level of mutation, the threshold of the allelic frequency is often under 5 % and close to the background noise. The Sanger sequencing cannot be used in that case to validate a mutation detected by NGS since its sensitivity is limited to 10 %. The cellularity should also be considered to interpret the results as a low level of tumor cell percentage in the sample will lead to a reduction of the sensitivity. The technical validation process is also important and the biologist should be ensured that the approach is able to detect most of the recurrent somatic alterations. The use of internal and external controls is one of the means to validate the bioinformatics’ process and avoid any false negative due to changes in the process.
False negatives can finally result from a bad coverage of the analyzed genomic region. The in silico analysis of the bed file with the covered region and with the CGHa coverage and oligonucleotide position is also an important step to validate the covered region. Indeed, for TSG, there are rarely hotspots of mutations and a full coverage is needed to correctly interpret the result.
All those points are crucial to be addressed by the biologist before any interpretation. It is important to validate the design and be sure that the results are reproducible with the use of well-selected controls.
7.3 Biological Interpretation
Once the presence of a molecular alteration is technically confirmed, the biological interpretation will add a level of confidence to consider the identified variants as actionable. The main goal of the interpretation is to predict the potential functional impact and to validate mutations as “driver” and exclude any “passenger” or “polymorphism.” Finally, the variants will often remain as “unclassified” since no evidence can be identified for any classification.
7.3.1 Nucleotide Variation
The genomic analysis of tumor samples leads to the identification of variants which can be identified in all cells of the patient, germline variants, or which are only identified in the tumoral sample and then identified as somatic variants. In most the tumor characterizations, the sequencing analysis, mainly with targeted sequencing, is done without any information on the germline variants of the patient. The analysis of the germline DNA is a key step for reducing false positives (Jones et al. 2015). A different strategy using database analysis is then necessary to exclude the germline variants in a list of identified alterations. The recurrence of a variant in many samples can be a clue to identify a polymorphism. However, the recurrence of a variant in a series of tumors is not an argument in favor of a polymorphism as some mutations as in the gene KRAS or PIK3CA are recurrent.
7.3.1.1 Exclusion of Polymorphism
The germline variant database will help distinguish a polymorphism from a somatic mutation. The two databases frequently used are the 1000 genome and the Exome Variant Server (EVS). The 1000 genome gathers the information from 2500 unidentified people through the world with exome analysis. The EVS gathers the information from 5400 patients which have noncancer disease (http://evs.gs.washington.edu/EVS/). A threshold of 0.1 % can be used to distinguish SNP from somatic mutations (<0.1 %).
The database dbSNP is not recommended to directly classify variants. This database gathers many variants, which have been identified independently of the systematic screening. In this database, the curation of the variant is not strict enough as some somatic mutations have been included with reference number (rs). The current version is the build 142. The selection of SNPs with a minor allele frequency (MAF) of 1 % or greater can be a reasonable threshold for excluding germline variants common in the general population.
Mendelian disease can also help for the classification of variants. In fact, if the variant is related to a Mendelian disease, it should not be a polymorphism. The TP53 mutations are related to Li–Fraumeni syndrome which can help to classify variants. Identically, the mutations of the von Hippel–Lindau tumor-suppressor gene are specific for the clear-cell histologic subtype of renal cell carcinoma related to von Hippel–Lindau syndrome. Several locus specific databases exist as UMD database for the gene BRCA1 and BRCA2. The ClinVar database (http://www.ncbi.nlm.nih.gov/clinvar/) is an attempt to gather all the information about germline variants throughout all the genes.
7.3.2 Somatic Mutation Database
Most driver mutations are recurrent in several tumoral processes and should be identified into the tumor databases. The Catalogue of Somatic Mutations in Cancer (COSMIC) contains a comprehensive catalog of over 136,000 somatic coding mutations in over 500,000 tumor samples. The limit of COSMIC is the fact that the statistics are based on different technical approaches of screenings with mainly hotspot analysis for mutations’ detection.
The whole exome sequencing (WES) of thousands of tumors as compared to germline exome sequencing to exclude SNPs has now been performed both by individual groups and through collective efforts such as the International Cancer Genome Consortium (ICGC) and the Cancer Genome Atlas (TCGA). The tumor bioportal give the access to the exome from different tumors (www.tumorportal.org or www.cbioportal.org). The list of somatic mutated genes looks finally limited in number when crossing all the TCGA from 12 major cancer types (Kandoth et al. 2013). The somatic variant database can help to identify some driver mutations, yet they can contain “passenger” variants. Consequently, the identification of recurrence of the variant in those databases could be a positive argument in favor of driver impact of the mutation.
7.3.3 Functional Evidence
Once the variant is not considered as a polymorphism or constitutional variant, other impacts need to be assessed. First of all, a variant can have impact on the splicing, which can cause the deletion of several nucleotides of one or several exons in the RNA transcript leading to functional impact. Secondly, a missense variant can lead to a functional impact by switching the amino acid. To identify those effects, one can assess the predictive value of biomarker for the splicing and for the protein function. For the splicing, the MaxENtScan is the best tool available and proves to be the most performant (Houdayer et al. 2012). Splicing can lead to oncogenic effect as the MET skipping of exon 14 in lung cancer which activates the tyrosine kinase activity (Seo et al. 2012). The splicing information is also very useful for predicting an inactivation of the suppressor genes.
On the other hand, the SIFT and PolyPhen algorithms help to understand the effect of the mutation on the amino acid. The validation of this system has been tested in Mendelian disease (Valdmanis et al. 2009). The validity is less obvious for oncogenes as the prediction is only about loss of function, whereas in oncogene, a functional gain is needed.
For oncogenes, the protein expertise is very useful. The alignment interspecies can be proposed and are integrated in SIFT and PolyPhen data bases. The alignment between proteins with the same function can be also a way to bring some information.
That is the case of the tyrosine kinase receptors (EGFR, MET, ERBB2, etc.). The alignment of those proteins will help to have a good knowledge of the most sensitive region which could have an impact on the activation of the protein and more specifically their kinase domain.
That is why the functional assays are the best solution to explore and definitely give an interpretation of the functional impact of the variants. The case of PIK3CA gene is very interesting since several mutations have been reported but the functional impact is very different for each mutation (Gymnopoulos et al. 2007). Currently, without enough rapid testing, the functional informations in the literature on variants are very useful for the classification. One would speculate that prospective in vivo assays might be possible to be performed in the furure to contribute to the interpretation of rare variants.
7.3.4 Copy Number Variants
The most common approach to identity copy number variants is the CGH-array (CGHa) approach or SNP-array approach. Identically to the NGS analysis, several steps are needed to reduce the list of alterations to targetable variants. On the CGHa, some background noise can be interpreted as amplification or deletion. The validation of the quality of the profile is the first important steps. Secondly, a minimal number of oligonucleotides implied in the alteration (i.e., more than 3) are the parameter used to exclude such false positive.
The existence of copy number polymorphisms should also be examined to be excluded. The comparative hybridization of the germline DNA is really the best way to exclude germline copy number variants. If no germline DNAs are available, the use of database can be the solution. The database of copy number polymorphisms is the Database of Genomic Variants (http://projects.tcag.ca/variation/). This database gathered copy number variants (CNVs), insertions/deletions (InDels), inversions, and inversion breakpoints annotated observed in healthy individuals and involving segments of DNA that are larger than 1000 bp. Insertions/deletions of 50 bp or larger are also included.
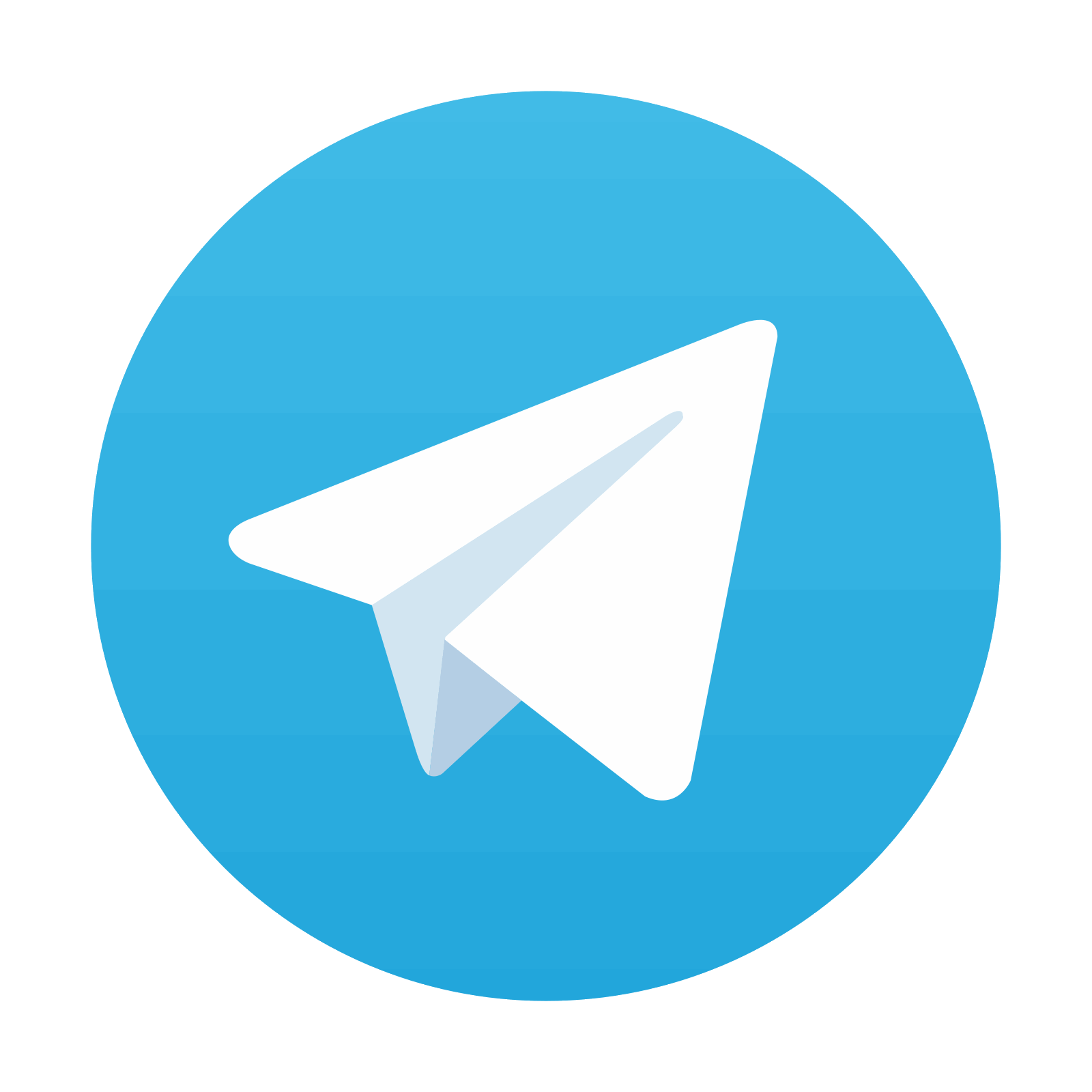
Stay updated, free articles. Join our Telegram channel

Full access? Get Clinical Tree
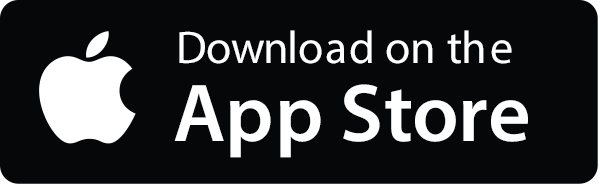
