Highlights
- •
Identified 12 gut microbial taxa potentially linked to prostate cancer risk using MR analysis.
- •
Employed robust statistical methods to ensure reliability of causal inference results.
- •
Explored gut microbiota’s role in modulating prostate cancer treatment outcomes.
- •
Highlighted connections between gut microbiota and other cancers like pancreatic cancer.
Abstract
Background
Numerous studies indicate that the gut microbiome is closely associated with prostate cancer (PCa), however, owing to various confounding factors, the causal relationship between gut microbiota and PCa remains unclear.
Methods
A 2-sample Mendelian randomization (MR) analysis utilized genome-wide association study (GWAS) data on the gut microbiota of 18,340 participants and GWAS summary statistics on PCa involving 46,3010 participants. Inverse variance weighted (IVW) served as the primary method, complemented by the MR-Egger method, weighted median method (WME), simple mode method (SM), and weighted mode method (WM). Finally, to confirm the robustness of the results, heterogeneity test, pleiotropy test, and leave-one-out sensitivity test were conducted.
Results
IVW analysis revealed that 12 specific gut microbial taxa were potentially causally associated with PCa; the genera Victivallis, Akkermansia, Odoribacter, Butyrivibrio, and the families Enterobacteriaceae, Verrucomicrobiaceae, as well as the orders Verrucomicrobiales, Enterobacteriales and the class Verrucomicrobiae, were found to be positively associated with PCa risk. Conversely, the genera Eubacterium ruminantium group, Candidatus Soleaferrea, and RuminococcaceaeUCG003 were negatively associated with PCa risk.
Conclusions
Our MR study’s results support a genetically predicted causal relationship between the gut microbiota and PCa, and we identified 12 specific gut microbial taxa. These findings could offer new targets for PCa screening and treatment.
1
Background
Prostate cancer (PCa) represents the second most common form of cancer in men globally and significantly impacts men’s health [ ]. According to global cancer statistics for 2022, PCa constitutes approximately 27% of cancers in men [ ]. Therefore, actively exploring the risk and prognostic factors of PCa is of paramount clinical importance.
PCa is characterized as a heterogeneous disease with etiological mechanisms that remain to be fully elucidated, but recognized risk factors include race, familial inheritance, age, obesity, and environmental factors [ ]. In recent years, researchers have increasingly focused on the etiology of PCa and its association with the microbiome, particularly the potential impact of the intestinal microbiota on PCa.
Gut microbes are a vital component of the human body, maintaining stability and diversity over time [ ]. Recent years have seen an exponential growth in research on the gut microbiota. Although the gut microbiota plays a crucial role in maintaining human health, it is implicated in several common diseases, including liver disease, inflammatory bowel disease, and obesity-related disorders [ ]. Notably, recent studies published in Science (2018) highlight the critical role of the gut microbiome in cancer development and treatment. These studies underscore that intestinal microorganisms not only influence the host’s metabolism and immune response but may also modulate tumor development through various mechanisms [ ]. Particularly, in studies of the intestinal microbiota in liver cancer and melanoma, it has been found that the gut microbiota influences the cancer treatment process by regulating antitumor immune response as well as other mechanisms [ ]. This evidence provides an important basis for investigating the potential connection between the gut microbiota and PCa. Recently, a study introduced the concept of the gut-prostate axis [ ], suggesting a potential association between the gut microbiota and PCa risk. This potential association has garnered support from observational studies. For instance, a prospective study identified an elevated abundance of Bacteroides massiliensis in the intestines of patients with prostate cancer relative to healthy individuals [ ], while another observational study indicated that Bacteroides and Streptococcus species were significantly more prevalent in the intestines of patients with prostate cancer [ ]. However, these findings do not establish a definitive causal relationship, nor have specific oncogenic gut microbes been identified. Additionally, the gut microbiota is prone to compositional changes due to gastrointestinal surgery and antibiotic use, potentially impacting the accuracy of the results [ , ]. Notably, previous observational studies have often been subject to confounding bias, information bias, selection bias, channeling bias, and reverse causality [ ]. Traditional randomized controlled trials (RCT) represent the gold standard for causal estimation, but are time-consuming, labor-intensive, and resource-intensive to conduct. In this context, the large number of gut microbiota and, more importantly, the long latency period from exposure to PCa render RCT impractical for this study [ ]. In this study, we investigate the causal relationship between the gut microbiota and PCa by utilizing a novel causal inference approach.
Mendelian randomization (MR) constitutes a time-efficient and cost-effective statistical approach for causal inference. This method investigates the causal relationship between exposures and outcomes by utilizing genetic variants as instrumental variables (IVs), substituting the exposure with these IVs. Since alleles for genetic variants are identified at conception and randomly assigned to offspring according to Mendelian laws, MR analyses are typically less susceptible to environmental confounding and reverse causation [ , ].
This study employed a 2-sample MR method with the objective of investigating the potential causal relationship between the gut microbiota and PCa risk, and of identifying specific gut microbial taxa.
2
Materials and methods
2.1
Research design
Genome-wide association studies (GWAS) examine millions of genetic variants in phenotypically diverse individuals to identify genotype-phenotype associations [ ]. To reduce potential confounding, we excluded SNPs associated with known risk factors for PCa, including age, BMI, race, dietary habits and hormonal levels. Utilizing a 2-sample MR framework, this study focused on gut microbiota and PCa as exposure and outcome, respectively. Summary statistics of gut microbiota and PCa were obtained from numerous GWAS to screen for suitable IVs. Subsequently, MR analysis was conducted, followed by sensitivity analyses to ascertain the robustness of the findings. The data sources for our study were open access, thus obviating the need for participants’ informed consent or ethical review. MR analyses are predicated on three key assumptions: (1) IVs must demonstrate a strong association with the exposure; (2) IVs must be independent of any confounders; and (3) IVs must not be associated with outcomes in any manner other than through the exposure [ ]. The process of this study is shown in Fig. 1 .
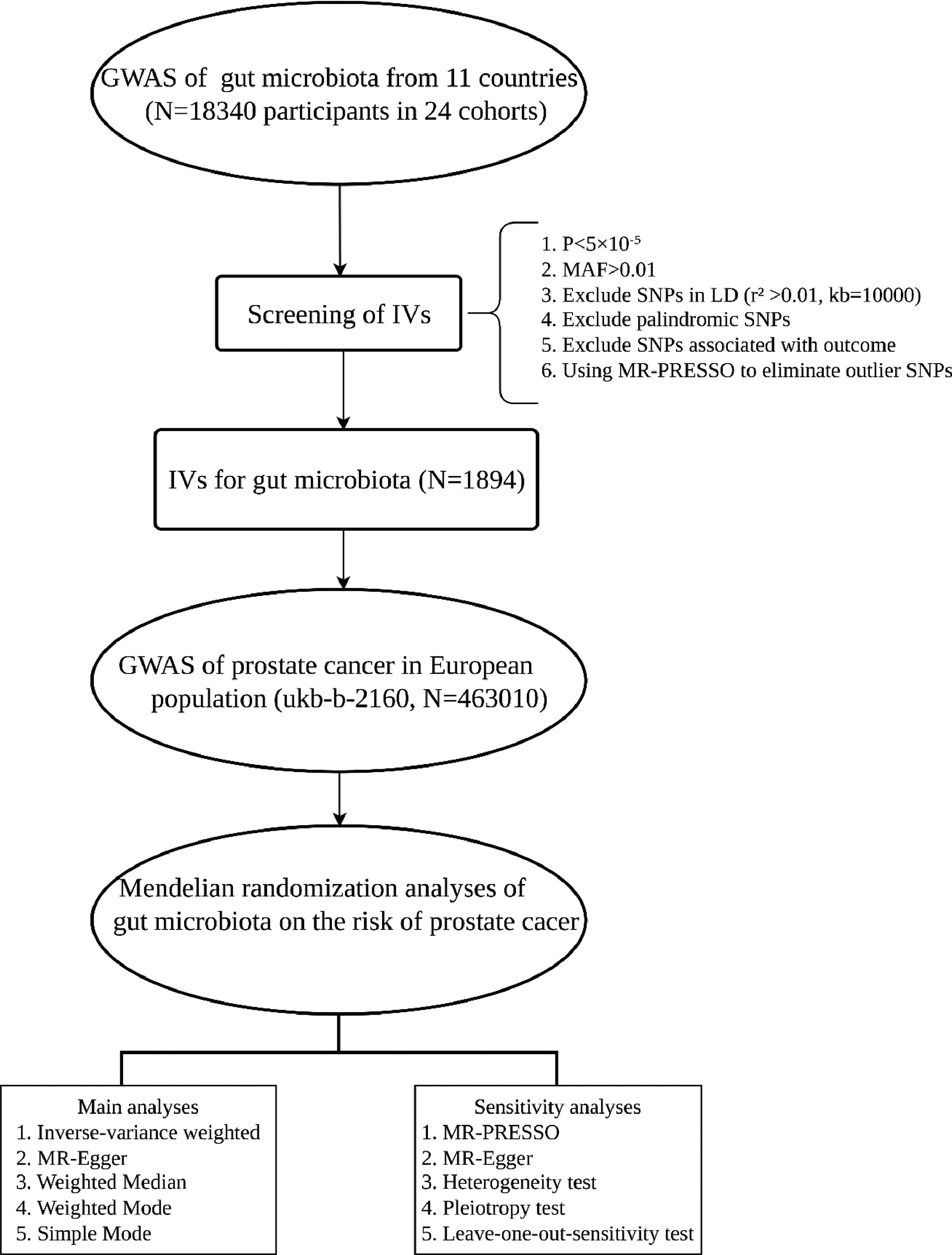
2.2
Exposure data sources
Data on gut microbiota-associated single nucleotide polymorphisms (SNPs) were sourced from the largest GWAS dataset within the MiBioGen consortium ( https://mibiogen.gcc.rug.nl/ ) [ ]. The consortium, multiethnic in nature, comprised approximately 3-quarters of participants of European origin. It included gene sequencing profiles of 16SrRNA and genotyping data from 18,340 participants across 24 cohorts from 11 countries. The consortium focused on locating and analyzing trait loci of the gut microbiota to delineate microbial compositions and taxa, and host genetic variations [ ]. Furthermore, the researchers factored in adjustments for sex, age, technical covariates, and genetic principal components [ ]. A total of 211 gut microbial taxa were incorporated in this MiBioGen consortium GWAS data.
2.3
Outcome data sources
SNPs associated with PCa were derived from the MRC-IEU consortium (ID: ukb-b-2160) in the GWAS database, which included 4,63010 participants of European ancestry, with 3,436 patients with prostate cancer and 4,59574 controls. A total of 9851867 SNPs were included in this dataset. GWAS summary data can be accessed via the website https://gwas.mrcieu.ac.uk/datasets/ukb-b-2160/ .
2.4
Selection of instrumental variables
Due to 15 gut microbial taxa being unidentified, our analysis ultimately encompassed 196 gut microbial taxa, including 119 genera, 32 families, 20 orders, 16 classes, and 9 phyla. Initially, SNPs significantly associated with the exposure were selected as IVs ( P < 5 × 10 −8 ). However, this resulted in only a limited number of SNPs being screened, prompting the relaxation of screening criteria to obtain a greater number of SNPs as IVs ( P < 5 × 10 −5 ). Subsequently, SNPs exhibiting linkage disequilibrium (LD) (r² > 0.01 and kb < 10000) were censored to mitigate the influence of LD on the results. SNPs associated with the exposure were extracted from the outcome data, and those directly related to the outcome were excluded. Subsequently, the merged data underwent harmonization to eliminate palindromic SNPs and incompatible SNPs. Finally, the F statistic was calculated for each SNPs. SNPs with an F statistic < 10, typically considered as weak IVs, were excluded from further analysis to prevent their influence on the results. The F statistic calculation followed the formula: F = R 2 (n-k-1)/k(1-R 2 ), R 2 = 2 × MAF × (1 – MAF) × β 2 (n denotes the sample size and k the number of IVs) [ ].
2.5
MR analysis
The “TwoSampleMR” package within R software was utilized for conducting 2-sample MR analysis, employing 5 methods: Inverse-variance weighted (IVW), MR-Egger, weighted median (WME), simple mode (SM), and weighted mode (WM). The IVW method, serving as the primary statistical approach for multiple IVs, assumes the validity of all IVs and integrates the Wald ratio estimates of each SNPs to derive the overall effect estimates [ ]. The remaining 4 methods served as supplements to the IVW approach, with IVW acting as the primary reference in cases where the 5 MR methods yielded disparate results. The WME method assigns greater weight to valid IVs and continues to provide reliable causal effect estimates even when some IVs are invalid [ ]. An intercept value of P < 0.05 in MR-Egger regression suggests the potential for horizontal pleiotropy among SNPs [ ]. The plurality-based estimation models in the SM and WM methods cluster SNPs with similar causal effects, consistently estimate the true causal effect, and offer robustness against horizontal pleiotropy [ ]. A strong causal association was inferred when the results across all five MR analysis methods aligned in the same direction.
2.6
Sensitivity analysis
To enhance the robustness assessment of our findings, a series of sensitivity analyses were conducted, encompassing heterogeneity tests, pleiotropy tests, and leave-1-out sensitivity tests. Each gut microbe-associated and PCa-associated SNPs was subjected to heterogeneity testing using Cochran’s Q test. In cases where heterogeneity was detected ( P < 0.05), the random effects model of IVW was implemented [ ]. The MR-PRESSO method was applied to detect horizontal pleiotropy. Should SNPs inducing bias be identified, they were removed and causal relationships re-evaluated [ ]. Finally, leave-1-out sensitivity analyses were employed to ascertain whether individual SNPs impacted the overall IVW effect.
These analyses were conducted using the “TwoSampleMR” and “MRPRESSO” packages in R software (version 4.3.1), presenting the results as odds ratios (ORs) and 95% confidence intervals (CIs), with a P -value of <0.05 denoting statistical significance.
3
Results
3.1
Screening results for SNPs
MR analysis included 196 gut microbial taxa. A total of 2,818 mutually independent SNPs, significantly associated with the 196 gut microbial taxa, were identified using the criteria of P < 5 × 10 −5 , LD r 2 < 0.01 and kb = 10000. The number of SNPs linked to each gut microbial taxon varied between 3 and 16. The F-statistics for these SNPs ranged from 14.58 to 88.42, all surpassing the threshold of 10, thereby indicating the absence of weak IVs among the selected SNPs ( Supplementary Table 1 ).
3.2
Results of MR analysis of the gut microbiota and PCa
A total of 1,959 SNPs significantly associated with gut microbiota were extracted from the outcome data, and 1,894 of these SNPs were harmonized for inclusion in the final 2-sample MR analysis. Two-sample MR analysis was conducted for each of the 196 gut microbial taxa ( Supplementary Table 2 ), identifying a potential causal relationship between 12 specific gut microbial taxa and PCa risk as indicated by the IVW method with P < 0.05 ( Fig. 2 ) . We found that genus Victivallis (OR:1.001, 95%CI:1.000–1.002, P = 0.007, IVW), genus Akkermansia (OR:1.002, 95%CI:1.000–1.004, P = 0.038, IVW), genus Odoribacter (OR:1.004, 95%CI:1.001–1.007, P = 0.014, IVW), genus Butyrivibrio (OR:1.001, 95%CI:1.000–1.002, P = 0.008, IVW), family Enterobacteriaceae (OR:1.003, 95%CI:1.000–1.005, P = 0.026, IVW), family Verrucomicrobiaceae (OR:1.002, 95%CI:1.000–1.004, P = 0.037, IVW), order Verrucomicrobiales (OR:1.002, 95%CI:1.000–1.004, P = 0.037, IVW), order Enterobacteriales (OR:1.002, 95%CI:1.000–1.004, P = 0.029, IVW) and class Verrucomicrobiae (OR:1.002, 95%CI:1.000–1.004, P = 0.037, IVW) were causally associated with an elevated risk of PCa. Additionally, the genus Eubacterium ruminantium group (OR:0.999, 95%CI:0.997–1.000, P = 0.030, IVW), genus Candidatus Soleaferrea (OR:0.998, 95%CI:0.997–1.000, P = 0.041, IVW) and genus RuminococcaceaeUCG003 (OR:0.997, 95%CI. 0.995–0.999, P = 0.007, IVW) were causally associated with reduced PCa risk. Nonetheless, the confidence intervals for some gut microbial taxa approached 1, indicating that the findings were not statistically significant and thus should be interpreted with caution.
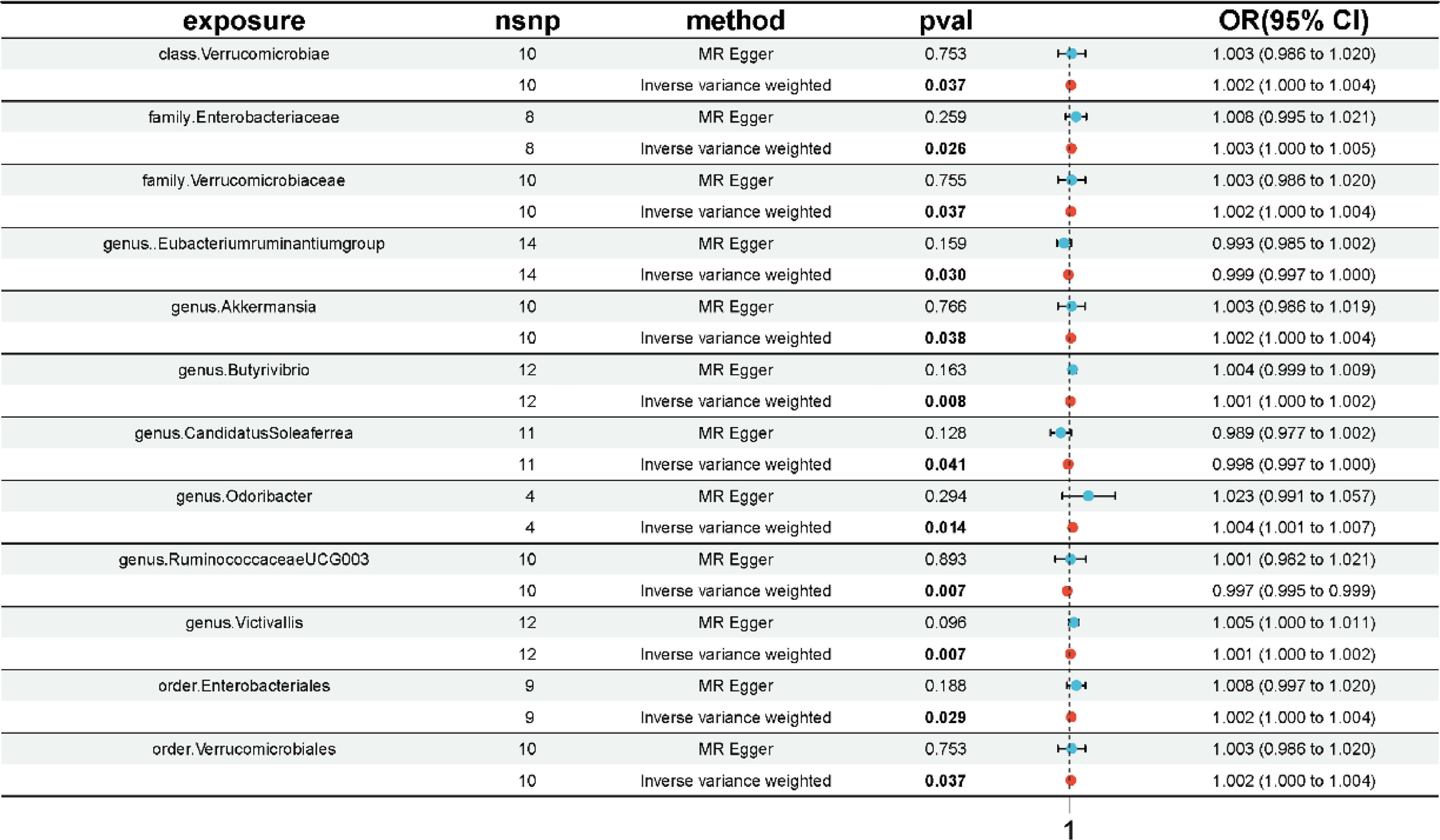
3.3
Sensitivity analyses
Cochran’s Q test, conducted on 12 gut microbial taxa potentially causally associated with PCa, revealed no significant heterogeneity in the IVW and MR-Egger methods ( Table 1 ) . The MR-Egger intercept analysis indicated an absence of potential horizontal pleiotropy between gut microbiota and PCa ( Table 2 ). Moreover, the MR-PRESSO analysis did not identify any outliers. The leave-1-out sensitivity analysis re-evaluated causality by sequentially removing individual SNPs. Among the 12 gut microbial taxa identified, only the genera Victivallis, Butyrivibrio, and RuminococcaceaeUCG003 demonstrated robustness in the leave-1-out sensitivity analysis, emphasizing the need for cautious interpretation of these findings ( Fig. 3 ). Refer to Supplementary Figs. 1 , 2 and 3 for forest, funnel and scatter plots respectively.
Outcome | Exposure | Method | Q | Q_pval |
---|---|---|---|---|
Prostate cancer | Class Verrucomicrobiae | MR Egger | 5.4621 | 0.707235 |
IVW | 5.472774 | 0.791303 | ||
Prostate cancer | Family Enterobacteriaceae | MR Egger | 6.385758 | 0.381392 |
IVW | 7.182692 | 0.410109 | ||
Prostate cancer | Family Verrucomicrobiaceae | MR Egger | 5.464168 | 0.707007 |
IVW | 5.474201 | 0.791169 | ||
Prostate cancer | Genus Eubacterium ruminantium group | MR Egger | 6.211433 | 0.905051 |
IVW | 7.65095 | 0.865574 | ||
Prostate cancer | Genus Akkermansia | MR Egger | 5.473007 | 0.706029 |
IVW | 5.479963 | 0.790624 | ||
Prostate cancer | Genus Butyrivibrio | MR Egger | 3.254312 | 0.97479 |
IVW | 4.371023 | 0.957781 | ||
Prostate cancer | Genus Candidatus Soleaferrea | MR Egger | 12.99162 | 0.162986 |
IVW | 15.91923 | 0.101968 | ||
Prostate cancer | Genus Odoribacter | MR Egger | 1.0805 | 0.582603 |
IVW | 2.436269 | 0.486919 | ||
Prostate cancer | Genus RuminococcaceaeUCG003 | MR Egger | 4.904285 | 0.767756 |
IVW | 5.089742 | 0.826416 | ||
Prostate cancer | Genus Victivallis | MR Egger | 9.716189 | 0.465736 |
IVW | 11.73393 | 0.383972 | ||
Prostate cancer | Order Verrucomicrobiales | MR Egger | 5.4621 | 0.707235 |
IVW | 5.472774 | 0.791303 | ||
Prostate cancer | Order Enterobacteriales | MR Egger | 6.405815 | 0.493242 |
IVW | 7.559954 | 0.47759 |
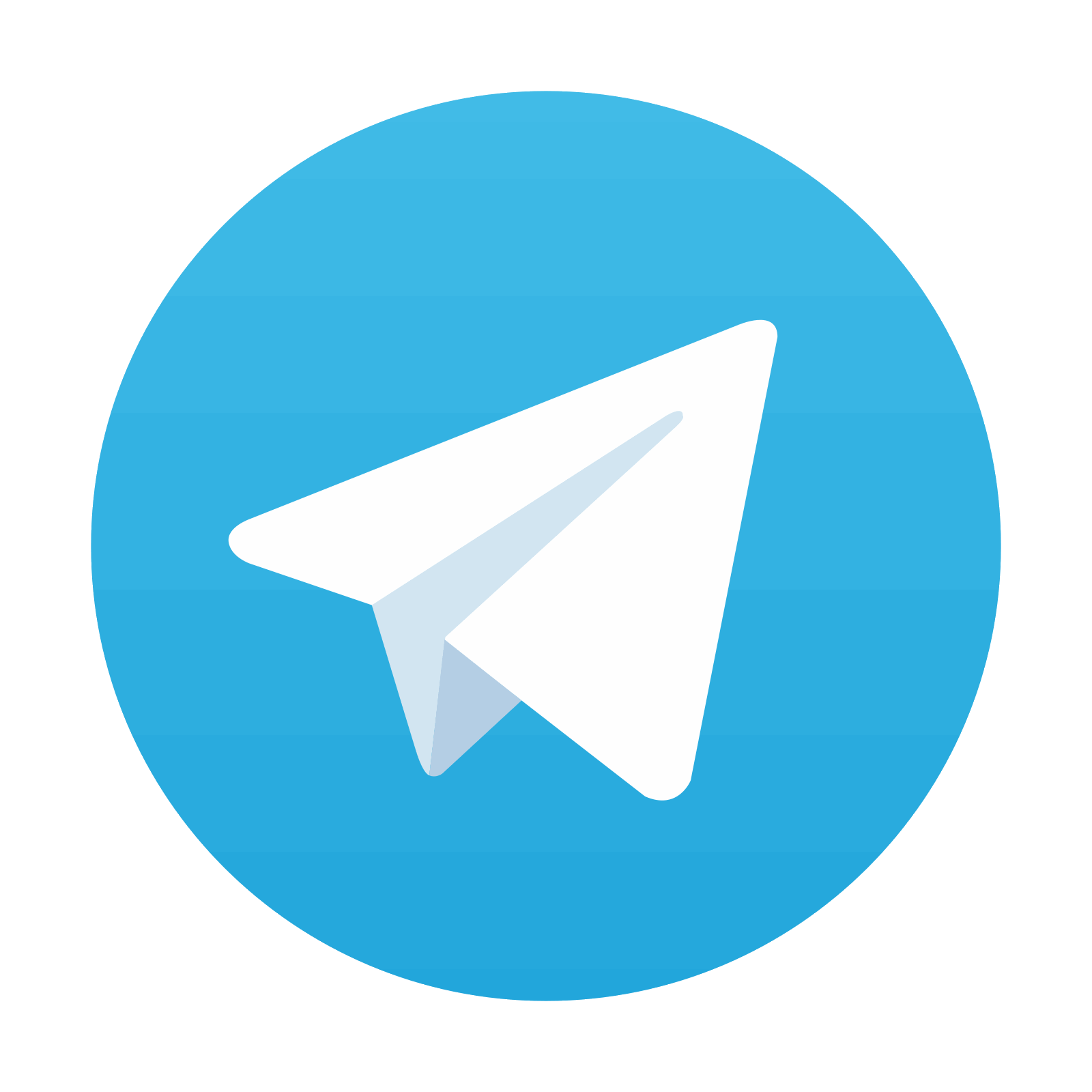
Stay updated, free articles. Join our Telegram channel

Full access? Get Clinical Tree
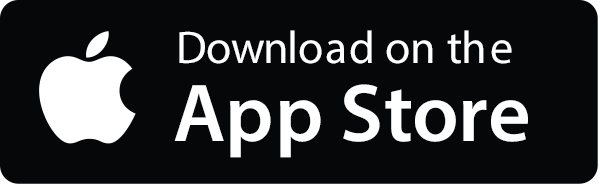

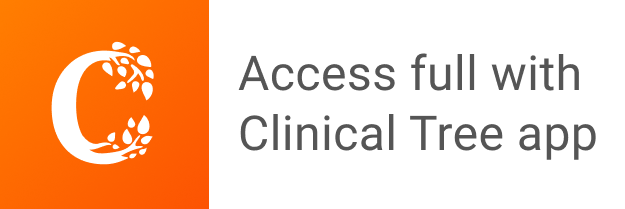