3 Sarah Brown Fleming (1982) Fleming proposes a one-stage, two-stage and multi-stage design requiring specification of response rates under the null and alternative hypotheses and type I and II error rates. Decision criteria are based around rejecting the null hypothesis that the response rate of the experimental treatment is not less than some pre-specified response rate, typically defined as the expected response rate of the current historical control treatment. Sample size is based on normal approximation to the binomial distribution. This is a widely used design and programs are readily available (e.g. Machin et al. 2008). Fazzari et al. (2000) Fazzari and colleagues propose modifications to previously published phase II designs. The modifications include: incorporating a patient population that is more representative of the intended phase III trial population, by reducing the eligibility restrictions and increasing the number of centres; increasing the sample size to allow more accurate estimates of the treatment activity; using an outcome measure that is more representative of that to be used in phase III, recommending a k-year progression-free survival (PFS) or overall survival (binary) outcome measure for advanced-stage disease populations; taking the upper limit of the 75% confidence interval of the activity of previous treatments as the minimum activity required to be observed to warrant moving to phase III. The methodology for the design of the study is based on rejecting the minimum activity required from an x% confidence interval around the estimate of treatment activity with, say, 80% probability. Sample size is generated using Monte Carlo simulation which will require programming. A’Hern (2001) A’Hern presents an adaptation of Fleming’s design (Fleming 1982). Calculation of sample sizes and cut-offs is based on exact binomial distributions as opposed to normal approximation. Trials based on exact distributions are typically larger than those using the normal approximation; however, they avoid the possibility that confidence intervals around the estimate of activity at the end of the trial will incorrectly contain the lower rejection proportion due to approximation to the normal distribution. As for Fleming, this design is widely used and programs are readily available for its implementation. The choice between Fleming and A’Hern should be based on the sample sizes and the requirement for exact testing. Chang et al. (2004) Chang and colleagues propose a design whereby the sample size, and thus the test statistic, is calculated using exact unconditional methods. This design may be used when the historical control data are based on only a few patients (say up to 120). The number of patients on which the historical data are based is required to be known as analyses take into account the pooled variance of the historical control and experimental data. Tables and software are available to calculate sample sizes. Mayo and Gajewski (2004) Mayo and Gajewski propose sample size calculations for a single-arm single-stage trial with binary outcome, using Bayesian informative priors (pessimistic/optimistic). This is an extension of the two-stage designs proposed by Tan and Machin (2002). Prior information regarding expected response rate and level of uncertainty in this value is required to determine sample sizes using either the mode, median or mean approach. Programming is required for the median and mean approaches, possible in Matlab. Sample sizes will vary depending on the approach used. Gajewski and Mayo (2006) Gajewski and Mayo describe Bayesian sample size calculations where conflicting opinions on prior information can be incorporated. Information required to design the trial includes prior distributions, cut-off for the posterior probability that the true response rate is greater than some pre-specified value and an error term relating to a small increase in the target response rate. Sample size calculation is iterative; therefore some computation is required to identify the design characteristics, for which no software is detailed but for which formulae are given to enable implementation. This design differs from the earlier design proposed by Mayo and Gajewski (2004) as it allows incorporation of pessimistic and optimistic priors, as opposed to one informative prior. Vickers (2009) Vickers proposes a design using historical control data to generate a statistical prediction model for phase II trial. The observed trial data for the experimental arm are then compared to the predicted results to give an indication of whether patients treated with the experimental agents are doing better than expected, based on the prediction model. The authors note that the methodology hinges on quality historical control data relevant to the patient population under study. Step-by-step methodology is presented which incorporates bootstrapping on both the historical data set and the observed data set and a comparison of the predicted and actual outcomes. Example Stata code is given in the appendix to the manuscript to allow implementation of the statistical analysis, as well as assessment of power. No references identified. Zee et al. (1999) Zee and colleagues propose single-stage and multi-stage single-arm designs considering a multinomial outcome, in the context of incorporating progressive disease as well as response into the primary outcome measure. Analysis is based on the number of responses and progressions observed, compared with predetermined stopping criteria. A computer program written in SAS identifies the operating characteristics of the designs. This is not noted as being available in the paper; however, detail is given to allow implementation. Lu et al. (2005) Lu and colleagues propose a design (one-stage or two-stage) to look at both complete response (CR) and total response (or other such outcome measures whereby observing one outcome implies the other outcome is also observed). The design recommends a treatment for further investigation if either of the alternative hypotheses is met (i.e. for CR or for total response) and rejects the treatment if neither is met. The designs follow the general approach of Fleming’s single-stage (Fleming 1982) or Simon’s two-stage (Simon 1989) approach whereby the number of CRs and total responses are compared to identified stopping boundaries. Tables are provided for some combinations of null and alternative hypotheses; however, formulae are given and at the time of manuscript publication programs were in development. The design differs from others in this section in that one outcome measure is a sub-outcome measure of the other, whereas other designs consider discrete outcome measures such as partial response (PR) versus CR. Chang et al. (2007) Chang and colleagues propose a single-stage and a two-stage design for window studies which aim to assess the potential activity of a new treatment in newly diagnosed patients. Treatment is given to patients for a short period of time before standard chemotherapy, and each patient is assessed for response or early progression (both binary outcome measures). The alternative hypothesis is based on both the response rate being above a pre-specified rate and the early progressive disease rate being below a pre-specified rate. The outcomes follow a multinomial distribution. A SAS program is noted as being available from the authors to identify designs. Stallard and Cockey (2008) Stallard and Cockey propose single-arm, one- and two-stage designs for ordered categorical data, where the rejection region for the null hypothesis is defined based on the likelihood ratio test. The null region over which the type I error is controlled considers a weighting of the proportion of patients in each response category, in a similar manner to that of Lin and Chen (2000). The focus of the paper is on response with three levels; however, the design may be extended to more than three levels. Programs are noted as being available from the first author to allow identification of designs. No references identified. Mick et al. (2000) Mick and colleagues propose a design based on the growth modulation index (ratio of time to progression of experimental treatment relative to that on the patients’ previous course of anti-cancer treatment). The outcome measure is novel and the authors justify its use for trials of cytostatic treatments where outcome measures such as tumour response may not be appropriate. Various values of the growth modulation index for null and alternative hypotheses should be considered to explore design parameters, as appropriate for the setting of the study. Each patient acts as their own control. Information is required for each patient on their time to progression on previous treatment, and an estimate of the correlation between the two times is needed. The design is identified via simulation, which allows investigation of the effect of the correlation estimate on the overall design. Although software is not detailed as being available, this has been implemented in Splus, and detail is provided to allow design implementation. Gehan (1961) Gehan proposes one of the earliest designs to assess experimental treatments in phase II trials. The methodology is based on the double sampling method and considers a phase II trial composed of a ‘preliminary’ stage and a ‘follow-up’ stage. The preliminary stage assesses whether the treatment under investigation is likely to be worth further investigation, using a confidence interval approach to exclude treatments with response rates below those of interest from further investigation. The follow-up stage assesses the activity of the treatment with pre-specified precision. The number of patients to be included in the follow-up stage is determined according to the number of responses observed during the first stage. The proposed design is intended to completely reject inactive treatments quickly, such that if the response rate of interest is excluded from the confidence interval at the end of the first stage, the trial is terminated early. Otherwise the trial continues. In the second stage the activity of the treatment is estimated with given precision, rather than providing decision criteria for continuing to a further trial. On this basis, this design may be seen as an estimation procedure for initial proof of concept trials rather than trials to determine whether or not to proceed to phase III. Fleming (1982) Fleming proposes a one-stage, two-stage and multi-stage design. The multi-stage design addresses multiple testing considerations to allow early termination in case of extreme results, employing the standard single-stage test procedure at the last test. Tables are presented for specific design scenarios using the exact underlying binomial probabilities rather than the normal approximation to these probabilities. Programs are readily available to calculate the overall sample size for a one-stage design (e.g. Machin et al. 2008), with sample sizes at each stage chosen to be approximately equal. Termination at the end of each stage is permitted for activity or lack of activity. Simon (1987) Simon introduces a two-stage design that is single arm with a binary outcome whereby the sample size is minimised under a pre-specified expected response rate, not necessarily the null or alternative response rate. Where this expected response rate corresponds with the null hypothesis response rate, this design is equivalent to the optimal design proposed in the subsequent paper summarised below (Simon 1989). The current design is optimised by keeping the size of the first stage small, making the probability of rejecting an inactive drug high, and not allowing too high a sample size in the second stage. Early termination is permitted at the end of stage 1 only for lack of activity. A table is provided with limited design scenarios; however, the designs detailed below (Simon’s optimal and minimax) are more widely used and may be considered ahead of this earlier design. Simon (1989) Simon proposes a single-arm two-stage design based on minimising the expected number of patients under the null hypothesis (optimal), as well as an additional design that minimises the maximum sample size (minimax). This is a well-known and widely used two-stage design, based on null and alternative response rates, power and significance level, and the observed number of responses at the end of each stage is used to assess stopping rules. The outcome of interest is binary and the trial may only be terminated at the end of the first stage for lack of activity. Extensive tables are provided for different design scenarios and software is readily available (e.g. Machin et al. 2008). Green and Dahlberg (1992) The design described by Green and Dahlberg permits early termination for lack of activity at the end of stage 1 when the alternative hypothesis is rejected at the 0.02 significance level. At the end of the second stage a significance level of 0.055 is used to reject the null hypothesis and declare sufficient activity for further investigation. Some detail is given regarding stopping boundary and sample size calculation, although this would need to be programmed and solved iteratively to find the most suitable design. This paper also discusses adaptations to the designs of Gehan (1961), Fleming (1982), and Simon (1989), in the cases where the final attained trial sample size differs from the original planned design. Heitjan (1997) Heitjan proposes a design whereby decision-making is based on the ability to persuade someone with extreme prior beliefs that the treatment under investigation is either active or not. This requires specification of extreme priors. For a sceptic, the probability that the experimental treatment is better than the standard treatment must be at least some pre-specified value (e.g. 70%) for the treatment to be declared active (known as the ‘persuade the pessimist probability’ PPP), and for an enthusiast, the probability that the experimental treatment is worse than the standard treatment must be at least some pre-specified value (e.g. 70%) for the treatment to be declared inactive (known as the ‘persuade the optimist probability’ POP). Timing of interim analyses can either be based on numbers of patients or time during the trial. Sample size is justified by assessing the operating characteristics and calculating PPPs and POPs of the design under various scenarios. Programs are noted as being available upon request from the author. Early termination is permitted for activity or lack of activity. Herndon (1998) Herndon proposes a hybrid two-stage design that allows continuation of recruitment while the results of the first stage are being analysed. If the results of the first stage indicate the treatment is inactive, accrual is suspended and data are re-analysed including data from all patients recruited to that time point. Otherwise, the design continues to target recruitment for the second stage. The sample sizes for the first and second stages are chosen for practicality rather than via Simon’s optimal method, with overall sample size calculated to maintain pre-specified type I and II errors for study-specific null and alternative hypotheses. Critical values for suspending recruitment, reinitiating or terminating recruitment and for declaring the treatment worthy of further investigation at the end of stage 2 are calculated. To identify the critical values a numerical search is required, for which formulae are provided. If the stage I results indicate re-analysis using all patients to that time point, analysis follows similar methodology to that proposed by Green and Dahlberg (1992), detailed above, as does the analysis of stage II. Chen and Ng (1998) Chen and Ng propose a flexible design that operates in the same manner as Simon’s two-stage design (Simon 1989), but here the number of patients at the first and second stages can vary by up to eight patients to allow a period of grace in halting recruitment (in a similar manner to that described by Green and Dahlberg 1992, detailed above). A FORTRAN program is noted as being available from the authors to enable implementation, and tables are given for some scenarios. Chang et al. (1999) Chang and colleagues outline a design for continuous or binary outcomes that takes into account the number of patients on whom historical control data are based. This reflects the fact that the variances of the historical control data and the experimental data will differ. The trial may be terminated at the end of the first stage for either activity or lack of activity. Algorithms are used to determine critical values for stopping, and sample size is calculated by multiplying the single-stage sample size (formulae provided) by between 1.02 and 1.05. Hanfelt et al. (1999) Hanfelt and colleagues propose a modification to Simon’s two-stage design (Simon 1989) that minimises the median number of patients required under the null hypothesis, as opposed to the expected number of patients. A program is noted as being available from the authors that performs the design search. The design differs very little to that of Simon, other than when the response rate of the treatment is much less than the null hypothesis rate. Termination at the end of the first stage is for lack of activity only. Shuster (2002) The minimax design proposed by Shuster follows the same format as, for example, Simon’s design (Simon 1989), although it allows early termination for activity at the end of the first stage, as well as for lack of activity. Sample sizes and cut-offs are calculated based on exact type I and II errors, and the smallest expected maximum sample size is calculated. The author shows that the proposed design generates the smallest sample sizes under the null, alternative and maximum scenarios, compared to Chang et al. (1987) and Fleming (1982). The author advises use of the proposed minimax design when early termination for activity is beneficial (giving as an example the setting of paediatric cancer). A table of specific design scenarios is presented; otherwise the design will require programming. Tan and Machin (2002) Tan and Machin propose two Bayesian designs: the single threshold design (STD) and the dual threshold design (DTD). The designs are intended to be user-friendly and easily interpreted by those familiar with frequentist phase II designs. They provide an alternative approach to the design, analysis and interpretation of phase II trial data, allowing incorporation of relevant prior information and summarising results in terms of the probability that a response proportion falls within a pre-specified region of interest. The following design parameters are required: target response rate for a new treatment; prior distribution for the experimental treatment being tested; the minimum probability of the true response rate being at least the target response rate at the end of stage 1 (for the STD, λ1) and at the end of the study (λ2). For the DTD, the lower response rate of no further interest is also required, and here λ1 represents the probability that the true response rate is lower than the rate of no further interest at the end of stage 1. The STD focuses on ensuring, at the end of the first stage, that the final response rate of the drug has a reasonable probability of passing the target response rate at the end of the trial. The DTD, however, focuses on ensuring, at the end of the first stage, that the final response rate at the end of the trial is not below the response rate of no further interest. Tables are given for a number of design scenarios and the designs are compared with the frequentist approach of Simon (1989). Programs have been developed and are available in Machin et al. (2008). Case and Morgan (2003) Case and Morgan outline a design with survival outcomes which are dichotomised to give survival probabilities at pre-specified time points of interest, incorporating all available information. The design is aimed to avoid the drawbacks of extended follow-up periods and breaks in recruitment during follow-up between stages. The design does not require a halt in recruitment between stages as Nelson–Aalen estimates of survival are used to incorporate all survival information up to the time point of interest, at the time of interim analysis. Early termination is permitted only for lack of activity. FORTRAN programs are noted as being available upon request from the authors, to identify the optimal design, and the proposed design is also available in Machin et al. (2008). Jung et al. (2004)
Designs for single experimental therapies with a single arm
3.1 One-stage designs
3.1.1 Binary outcome measure
3.1.2 Continuous outcome measure
3.1.3 Multinomial outcome measure
3.1.4 Time-to-event outcome measure
3.1.5 Ratio of times to progression
3.2 Two-stage designs
3.2.1 Binary outcome measure
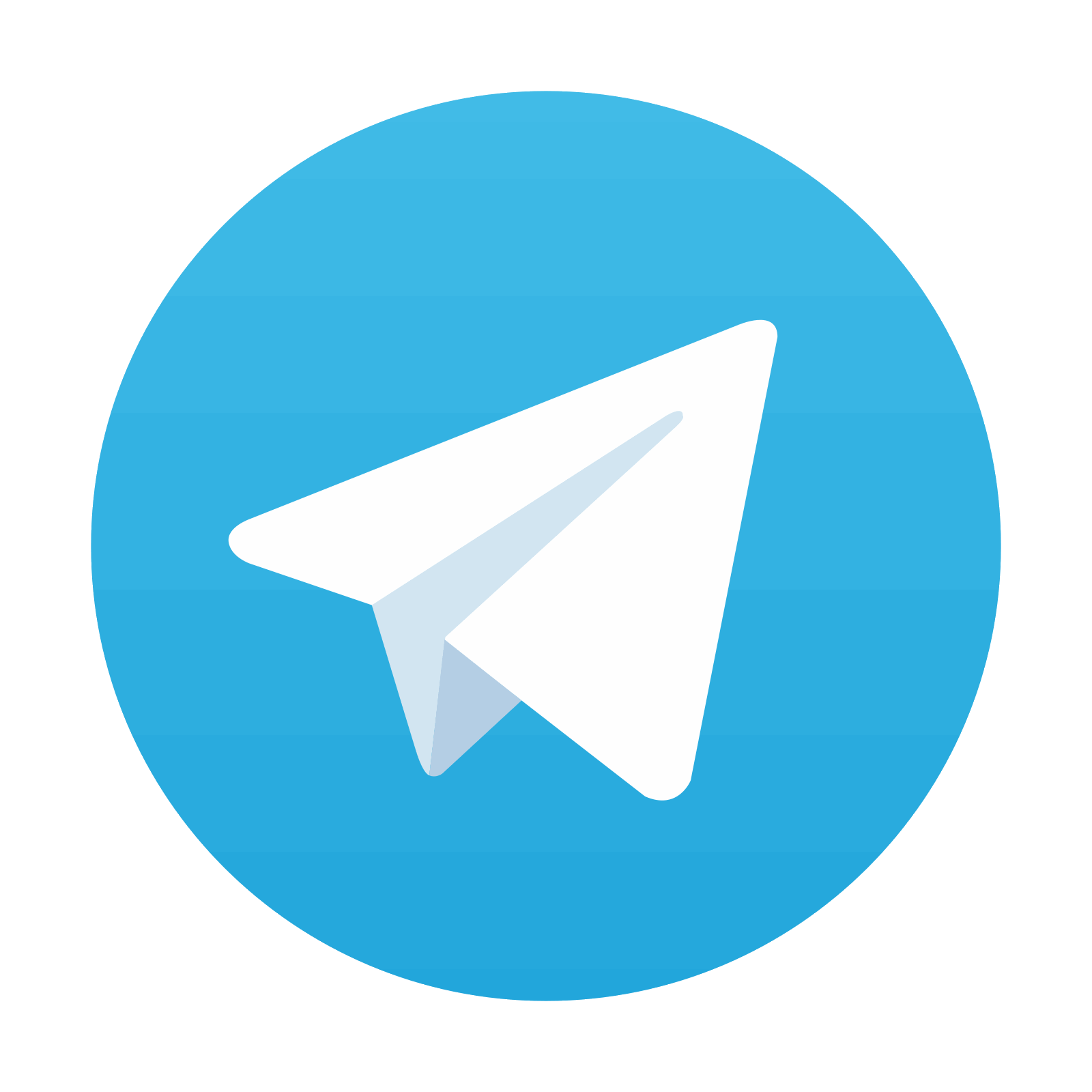
Stay updated, free articles. Join our Telegram channel

Full access? Get Clinical Tree
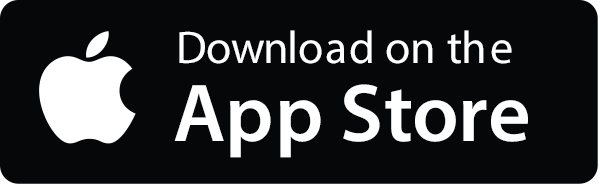
