Highlights
- •
The calculations showed specific SNPs at 8q24 locus as noteworthy for PCa risk.
- •
The MSMB, ITGA6, SUN2, FGF10, INCENP, MLPH, and KLK3 genes were noteworthy for PCa.
- •
The genes that were noteworthy are potential biomarkers for the disease.
Abstract
Prostate cancer (PCa) is a complex disease influenced by many factors, with the genetic contribution for this neoplasia having a great role in its risk. The literature brings an increased number of Genome-Wide Association Studies (GWAS’s) that attempt to elucidate the genetic associations with PCa. However, these genome studies have a considerable rate of false-positive data whose results may be biased. Therefore, we aimed to apply Bayesian approaches on significant associations among polymorphisms and PCa from GWAS’s data. A literature search was performed for data published before April 20, 2024, whereby two investigators used a specific combination of keywords and Boolean operators in the search (“prostate carcinoma or prostate cancer or PCa” and “polymorphism or genetic variation” and “Genome-Wide Association Study or GWAS”). The records were retrieved, and the data were extracted with further application of two different Bayesian approaches: The False Positive Report Probability (FPRP) and the Bayesian False-Discovery Probability (BFDP), both at the prior probabilities of 10 -3 and 10 -6 . The data were considered as noteworthy at the level of FPRP <0.2 and BFDP <0.8. Besides, in-silico analyses by gene-gene network and gene enrichment were performed to evaluate the role of the noteworthy genes in PCa. As results, 13 GWAS’s were included, with 2,520 values for FPRP and 1,368 values for BFDP being obtained. Our study showed an extensive number of gene variations as noteworthy candidate biomarkers for PCa risk, with highlighting for those occurred in the 8q24 locus and in the MSMB, ITGA6, SUN2, FGF10, INCENP, MLPH, and KLK3 genes.
1
Introduction
Prostate cancer (PCa) is neoplasia that initiates with the inflammation of prostate gland in response to uncontrolled cell division caused by mutations that arise from DNA damage with consequent damage to cell cycle [ ].
PCa is the second most prevalent solid tumor in men; in 2020, 1,414,000 new cases of the disease were estimated worldwide [ ]. In the United States, on January 1, 2018, it was estimated that 120,400 men live with metastasis of PCa. It is expected that by 2030, 195,500 men will live with the metastatic form of PCa [ ]. Similar data were observed in Canada, with 131,718 men diagnosed with PCa in 2019 [ ]. On the other hand, there was a decrease of 7.1% in the PCa rate in the European Union between 2015 and 2020, possibly due to therapeutical and diagnosis advancements [ ].
Although the disease is influenced by many factors such as diet, lifestyle and the inflammatory microenvironment of prostate [ , ], it is the patients’ genetic aspects that promote a significant influence in the carcinogenesis of PCa [ ]. For instance, a previous study has analyzed the polygenic risk for PCa and identified that the disease risk is related with the rare variants on the Homeobox B3 (HOXB3), Breast Cancer 2 ( BRCA2 ), ATM serine/threonine kinase (ATM) and Checkpoint kinase 2 (CHEK2) genes [ ]. Besides, other findings revealed that patients carrying Cyclin-Dependent Kinase 12 (CDK12) gene mutations showed a great susceptibility for metastasis and development of castration-resistant [ ], as well as that patients with genetic variants in critical chromosome regions such as the 8q24 presented an association with PCa [ ].
The literature brings a considerable amount of Genome-Wide Association Studies (GWAS’s) with an extensive data for evaluating the association among several Single Nucleotide Polymorphisms (SNP)’s and PCa risk [ ]. In fact, many of these data bring a significant impact on the knowledge of genetic influence on prostatic cancer risk and development. However, the GWAS’s, as well as meta-analysis of observational studies, may be interfered by the p-level as threshold of statistical significance. Previous authors have developed accurate approaches to refine and reevaluate the significant results from these studies [ , ].
One previous study revaluated the significant data from genetic meta-analyses on polymorphisms in immune mediators and PCa risk [ ], whose results elucidated the relation between SNP’s and the disease with suggestion of two polymorphisms in IL1B and IL18 genes as noteworthy biomarkers for PCa. Although these data clarified the role of these factors in PCa course, a study that widely reevaluates the genetic variability related with the disease is necessary.
Hence, our study aimed at promoting a revaluation on significant statistical associations on genetic polymorphisms and PCa risk from GWAS’s available in the literature. Our data indicate several SNP’s and genes as noteworthy biomarkers for PCa screening and evaluation, what may be a reliable source for future epidemiological and clinical studies.
2
Material and methods
2.1
Protocols
We followed the Preferred Reporting Items for Systematic Reviews and Meta-Analysis ( The PRISMA Statement) [ ] to conduct this systematic revaluation. In addition, we have registered this study in the PROSPERO database under the following ID number: CRD42023488582.
2.2
Strategy search
The strategy search may be described by the screening of several databases (Google Scholar, Medline, Pubmed and Web of Science) for studies published before April 20, 2024, by 2 independent investigators (AVOM and FRPS). We used a combination of keywords, and the following Boolean operators were used in the research: “prostate carcinoma or prostate cancer or PCa” and “polymorphism or genetic variation” and “Genome-Wide Association Study or GWAS.”
No language restriction was applied in the search.
2.3
Eligibility criteria
The studies that met the following inclusion criteria were included in the results: (1) comprise a GWAS on prostate cancer risk; (2) bring data for the possible genetic association by Odds Ratio (OR) value with 95% of Confidence Intervals (CI) and the p value for the association test. We excluded the articles identified if: (1) they did not cover the subject of genetic variations and PCa risk; (2) they did not use specific and correct statistical methods to calculate the genetic association; (3) they did not bring sufficient data for statistical analyses.
2.4
Data extraction
The data extraction was performed by two investigators (ALABL and FRPS) by means of a standardized form comprising the followed data: (1) author and year, (2) gene, (3) variant, (4) comparison, (5) evaluation, (6) Odds Ratio (OR) value with 95% of Confidence Intervals (CI) and (7) p-value for the calculations of the meta-analyses. Data were collected from statistical analyses, whose information was plotted on an Excel spreadsheet.
2.5
Statistical analysis
We applied two Bayesian approaches to assess the level of noteworthiness from the collected evidence: The False Positive Report Probability (FPRP), and the Bayesian False-Discovery Probability (BFDP). Firstly, FPRP is a test that targets the probability of possible non-true associations between the susceptible loci and the disease [ ]. The FPRP value is derived from the following parameters: (1) π – the indicator of the prior probability for a true association, (2) the lowest α value where the test is noteworthy or the obtained p-value from the studies, and (3) 1 – β, which indicates the statistical power for the finding to be noteworthy, with rejection of the null hypothesis. Then, the FPRP may be calculated by the equation below:
FPRP=α(1−π)/{α(1−π)+(1−β)}
α value can be replaced by the observed p-value and (1 – β) is calculated from a specific equation available by Wacholder et al. [ ]. In this field synopsis, two pre-specified values for the prior probabilities (10 −3 and 10 −6 ) were applied on the data. They would be, respectively, close to the expected values for a candidate gene or a random gene. Therefore, we open the chance to perform the reader’s own judgment on the evidence presented for a locus. Wacholder et al. [ ] indicated three values for OR (1.2, 1.5 and 2.0), which were valid for a noteworthy finding. Considering the results on OR included in this study, with the median value approached nearly between 1.2 and 1.5, both values (i.e., 1.2 and 1.5) were chosen. The cutoff level for FPRP is set at 0.2 as previously described in the literature.
Second, BFDP calculation was also used. It allows a description of noteworthiness by means of the measure of a false discovery and a false non-discovery, with a solid methodological basis, as a complementary information for FPRP [ ]. According to the literature, the cutoff level for BFDP is set at 0.8, derived from the assumption that a false non-discovery is four times as costly as a false discovery [ ]. BFDP is explained by the following equation, where PO is the prior odds of no association and ABF is the approximate Bayes factor, which can be assumed from OR and SE:
BFDP=(ABF×PO)/(ABF×PO+1)PO=π0/(1−π0)
Likewise, BFDP was calculated based on both prior probabilities (10 −3 and 10 −6 ). In addition, all the accompanying computations to derive BFDP were performed with the Excel spreadsheet released by [ ].
2.6
In silico analyzes
A gene-gene network from GeneMania database (version 3.3) was designed, which forms a network of a “seed list” of genes provided by a user from databases of curated biological pathway knowledge (KEGG) and InterPro. For the gene enrichment in gene ontology [ ] ( https://geneontology.org/ ) and human phenotype ontology (Monarch) [ ] ( https://hpo.jax.org/ ) of the coding genes which their polymorphisms were noteworthy, the STRING database (version 12.0) was used. For the significance of gene enrichment, STRING 12.0 default was applied for p-value adjustment to false discovery rate (FDR adj ˂0.05), using Benjamini-Hochberg correction method.
3
Results
3.1
Collected studies
Our search strategy resulted in 2,987 eligible titles. After the steps of screening, evaluation and collection, 13 studies remained in the results [ , ]. The GWAS’s were published between 2007 and 2020 totaling 308,094 participants (69,389 cases and 238,705 controls) which the studies brought data for many ethnical groups including Caucasian, Asian, African, and Mixed populations from several countries as shown in Table 1 .
Author | Year | Ethnicity | Total Case | Total Control |
---|---|---|---|---|
Brezina | 2020 | Austrian | 351 | 87 |
Du | 2020 | Latino | 2820 | 5293 |
Eeles | 2009 | United Kingdom and Australia | 16229 | 14821 |
Gudmundsson | 2009 | Icelandic | 1980 | 7000 |
Gudmundsson | 2007 | Icelandic | 1453 | 3064 |
Haiman | 2011 | African-Americans | 3425 | 3290 |
Hoffmann | 2015 | Non-Hispanic white, African American, Asian and Latino | 7783 | 38595 |
Ishigaki | 2020 | Japanese | 5408 | 103939 |
Na | 2013 | European, African American, Japanese, and Chinese | 1922 | 2175 |
Nam | 2011 | North American | 316 | 229 |
Oh | 2017 | Koreans | 1001 | 2641 |
Thomas | 2008 | European | 3941 | 3964 |
Yeager | 2007 | European | 4296 | 4299 |
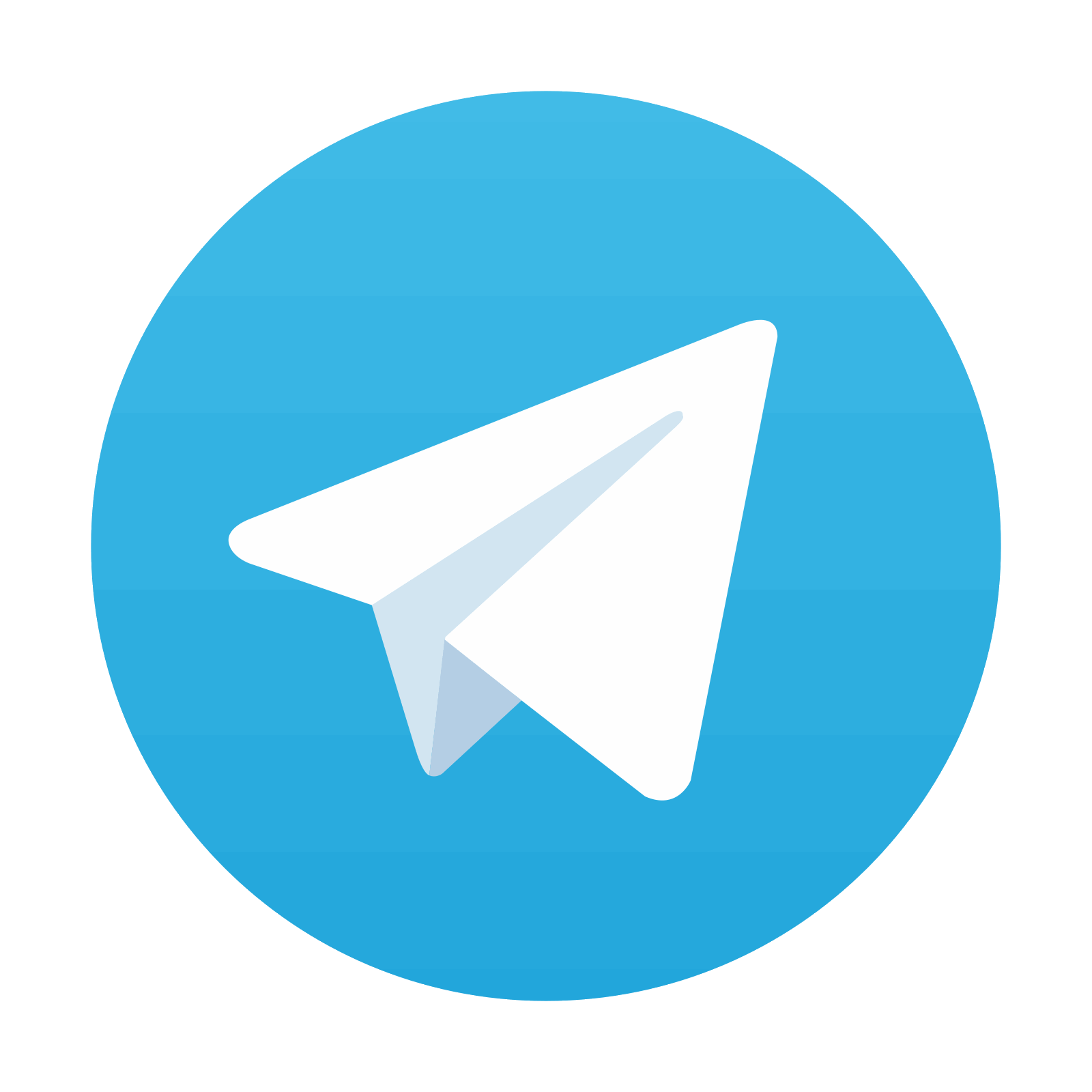
Stay updated, free articles. Join our Telegram channel

Full access? Get Clinical Tree
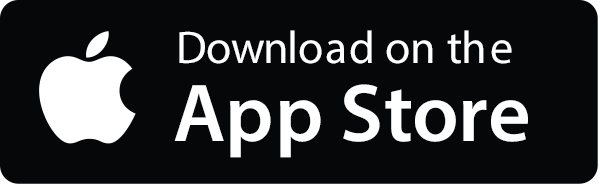

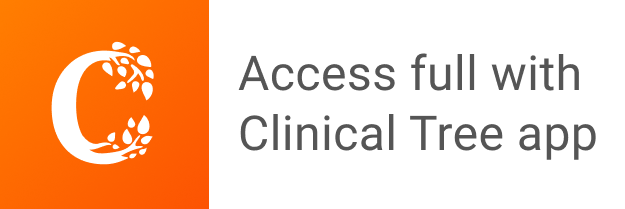