Highlights
- •
Neighborhood factors may improve prediction of aggressive prostate cancer.
- •
Differences in neighborhood factors were found among black and white men.
- •
Social determinants of health should be considered for risk prediction models.
Abstract
Introduction
Black men are diagnosed with high-grade prostate cancer (PCa; Gleason sum ≥7) at greater rates than White men. This persistent disparity has led to mortality rates among Black men that are twice the rate of White men. Risk prediction tools can aid clinical decision making for PCa screening, biopsy, and treatment. However, research has not integrated neighborhood-level risk factors that are associated with rates of high-grade PCa. This study sought to determine whether inclusion of neighborhood-level variables can improve prediction of high-grade PCa over the existing Prostate Cancer Prevention Trial (PCPT) calculator.
Methods
Existing PCa cases from 2005 to 2017 were ascertained from urology, radiation, and medical oncology clinics at Fox Chase Cancer Center/Temple University Health System (FCCC/TUHS). Existing databases from patient medical records, biosamples, pathology, and neighborhood data from the U.S. census were linked via geocodes. Informed by prior studies that selected social environmental variables, a series of logistic regression models were completed to predict the probability of high-grade PCa on prespecified sets of variables from the PCPT.
Results
Our best fitting, multilevel model included PCPT variables (i.e., PSA, digital rectal exam, age, race, prior biopsy, family history) as well as insurance status, neighborhood-level poverty, residence in a high risk PCa cluster, and % of Employed Men in Protective Service Occupations. However, the AUC for this model (0.673) was only marginally improved from the initial model of only PCPT variables (0.671). Further, in separate analyses by race (White, Black) the % of Employed Men in Protective Service Occupations was only significant among White men.
Discussion
Study findings demonstrate the potential for neighborhood variables to enhance current risk prediction models and identify interaction effects revealing differences across subgroups, such as race. The lack of significant associations between neighborhood variables and Black men highlight the complexity of systemic racism and neighborhood-level variables on PCa outcomes.
1
Introduction
Despite high survival rates, prostate cancer (PCa) is still the most common noncutaneous malignancy and the second leading cause of cancer death among U.S. men [ ]. Black men are more likely to be diagnosed with high-grade PCa (Gleason sum ≥7) and twice as likely to die of PCa compared to White men [ ]. This disparity is the largest of any cancer and has persisted for the past 3 decades [ ]. To reduce PCa disparities, improvements in risk assessments within PCa screening are needed to better identify and target men at risk for high-grade PCa at an initial diagnosis [ ]. This is particularly important as high-grade PCa can require more aggressive treatments with harmful side effects, particularly for men who have to undergo androgen deprivation therapy [ ].
PCa screening involves a prostate specific antigen (PSA) blood test and digital rectal exam (DRE) and higher PSA levels can sometimes be indicative of having PCa and more aggressive disease [ , ]. However, PSA is an imperfect indicator of PCa disease presence or severity and screening guidelines have changed frequently over the past decade due to concerns such as overdiagnosis and subsequent overtreatment for PCa [ ]. Consequently, recent studies suggest that changes in screening guidelines have led to increases in PCa diagnosis, driven by initial diagnoses with advanced stages, particularly in Black men [ ]. Thus, racial disparities in PCa morbidity and mortality continue to disproportionately impact Black men [ ]. Contributing to the persistent disparities is the lack of understanding of PCa etiology. The few known risk factors include age, family history of PCa, common genetic variants, and African genetic ancestry [ ]. This subsequently leads to an overall low understanding of who is at risk of high-grade PCa and should receive more aggressive primary treatments.
Up to 70% of PCa clinicians use nomograms or risk prediction tools for clinical decision making [ ] related to PCa screening, biopsy, and treatment. One such tool is the Prostate Cancer Prevention Trial (PCPT) calculator for predicting high-grade (Gleason sum ≥7) vs. low-grade PCa (Gleason sum <7) [ ]. The PCPT calculator, along with most other PCa prediction tools, only incorporate clinical variables (e.g., age, race, PSA, PCa family history, DRE, prior biopsy). No current risk assessment tools predict with perfect accuracy, and few have been adequately tested in Black men [ ]. Thus, additional predictive markers need to be considered [ ] and tested in more diverse populations, given the role disparities play in PCa diagnoses.
While potential drivers of PCa racial disparities span across multiple levels from genetics to an individual’s behavior to social circumstances [e.g., insurance access, socioeconomic status (income, education, employment), social support, housing insecurity] [ ], few risk prediction tools consider multilevel risk factor information. In the absence of individual level data, neighborhood or area-level factors at small enough geography can serve as a surrogate for individual social circumstances [ ]. Our prior association, machine learning, and geospatial analyses have found that neighborhood socio-environment, including the employment, housing, poverty, and transportation structure of a neighborhood, are both associated with and potentially predictive of advanced stage and grade PCa in both Black and White men [ ]. Further, using available patient addresses, neighborhood variables are readily obtainable by researchers or clinicians for inclusion in predictive models.
The objectives of this study were to extend our previous research by (1) assessing if select neighborhood social environmental variables from our prior studies were associated with diagnosis of high-risk PCa and (2) determining whether inclusion of such variables can improve prediction of advanced grade PCa over the existing PCPT calculator that includes primarily clinical variables only. Interrogating whether social environmental factors are associated with and/or predictive of high-grade prostate can inform disparities research, as well as Precision Medicine, which suggests that consideration of social environmental factors may improve patient care.
2
Materials and methods
2.1
Study population
Existing PCa cases were ascertained from the FCCC/TUHS electronic medical records (EMR), drawing patients from urology, radiation, and medical oncology from 2005 to 2017. Approximately 10% of patients were excluded as their addresses were either incomplete or incorrect and could not be matched in ArcGIS during geocoding or were listed as P.O. boxes. Existing databases from patient medical records, biosamples, pathology, and neighborhood data from the U.S. census were linked via geocodes. Full addresses, including street number, street name, city, state, and zip code were obtained for each patient for the purposes of geocoding. Geocoding is the process of assigning a location, usually in the form of latitude and longitude coordinate values (i.e., points), based on a residential address. The North American Association of Central Cancer Registries (NAACCR) Geocoder and ArcGIS 10 (ESRI, Redlands, CA) software was used for geocoding. Geographic identifiers (in this case, Federal Information Processing Standard or FIPS codes associated with each census tract) were used to link the geocoded data with U.S. Census data variables from 2010. The study was approved by the Fox Chase Cancer Center Institutional Review Board.
2.2
Primary outcome
Our main outcome measure of high-grade PCa was based on the PCPT calculator definition, which included race, age, family history of PCa, PSA (ng/ml) and DRE at time of diagnosis, and prior biopsy (before diagnostic biopsy). We additionally tested a more recent definition of aggressive PCa (staging category of T4, N1, or M1, or Gleason sum ≥8) suggested for association studies [ ]. As findings for high-grade PCa within the PCPT calculator and the recent definition of aggressive PCa were similar, we only present results from the PCPT calculator.
2.3
Clinical and neighborhood variables
Clinical variables (listed in Table 1 ) were ascertained from existing EMR data in FCCC/TUHS clinical datasets using manual data entry by research staff. Marital status and health insurance status were those listed at time of data abstraction. Our research team verified the data entry manually checking each clinical factor for all patients in the EMR. U.S. Census variables were downloaded from www.socialexplorer.com . Our prior research informed selection of social environmental variables for this study. Specifically, in our prior research we ran 2 different machine learners [sparse group lasso (SGL) and Bayesian additive regression trees (BART)] on our more recent Pennsylvania PCa registry data (2005-2016) to identify and narrow down social environmental predictors [ ]. Additionally, we tested batches of social environmental variables (US census; n > 13,000 variables) [ ]; environmental variables (air, water, land quality; n = 5 variables); socioeconomic status (SES) indices ( n = 10 variables); landscape variables related to building and green space ( n = 11 variables); and patient level variables (age, date of diagnosis) separately by race (Black/White men) in our Fox Chase Cancer Center clinical dataset [ ] and in the PA State PCa registry [ ]; unpublished results]. A wide selection of neighborhood-level variables (>13,026) were initially included in the machine learning models to capture the variety of social factors (e.g., economic, physical, social) characteristics of neighborhoods [ ]. The 4 social environmental variables that continued to replicate across these association and geospatial studies were included in the present study: (1) % living below poverty (quantiles), and continuous measures related to (2) PCT_C2401002: % of Employed Men in Protective Service Occupations – Firefighting and prevention, law enforcement workers, including supervisors; (3) PCT_B08301012: % Workers 16 Years and Over: Public Transportation – Streetcar or Trolley Car (Carro Publico in Puerto Rico); and (4) PCT_B25121019; % Household Income by Value of Owner-Occupied Housing Units – Both Income and Value $10,000 to $19,999. Further, we previously conducted geospatial analyses that identified areas where rates of high-grade PCa were higher than expected in the State of PA [ , ]. These clusters are specific geographic areas with greater-than-expected numbers of high-risk PCa cases [ , ],Thus, location in a high-risk cluster (yes/no) was additionally included as a social environmental indicator.
Characteristic | White ( n = 3390) | Black/African American ( n = 668) | Overall ( n = 4058) | P -value |
---|---|---|---|---|
Initial model with standard patient-level clinical variables | ||||
Age at diagnosis | < 0.001 a | |||
Mean (SD) | 64.7 (8.2) | 61.3 (8.3) | 64.1 (8.3) | |
Prior biopsy | 0.005 b | |||
Never | 3319 (97.9%) | 642 (96.1%) | 3961 (97.6%) | |
At least 1 | 71 (2.1%) | 26 (3.9%) | 97 (2.4%) | |
Digital rectal exam | 0.364 b | |||
Normal | 2511 (74.1%) | 506 (75.7%) | 3017 (74.3%) | |
Abnormal | 879 (25.9%) | 162 (24.3%) | 1041(25.7%) | |
Family history | 0.004 b | |||
No | 2654 (78.3%) | 489 (73.2%) | 3143 (77.5%) | |
Yes | 736 (21.7%) | 179 (26.8%) | 915 (22.5%) | |
PSA (ng/ml) | < 0.001 a | |||
Mean (SD) | 7.6 (13.2) | 10.1 (22.1) | 8.0 (15.1) | |
Advanced model with patient-level socio-economic indicators | ||||
Marital status | < 0.001 b | |||
Married | 2737 (80.7%) | 437 (65.4%) | 3174 (78.2%) | |
Not married | 653 (19.3%) | 231 (34.6%) | 884 (21.8%) | |
Insurance group name | 0.03 b | |||
Private insurance | 1995 (58.8%) | 430 (64.4%) | 2425 (59.8%) | |
Medicare or Medicaid | 1334 (39.4%) | 229 (34.3%) | 1563 (38.5%) | |
Other/Uninsured | 61 (1.8%) | 9 (1.3%) | 70 (1.7%) | |
Multilevel models including patient and neighborhood-level variables | ||||
% Living below poverty (Quartiles) | < 0.001 b | |||
Q1 | 1683 (49.7%) | 98 (14.7%) | 1781 (43.9%) | |
Q2 | 912 (26.9%) | 131 (19.6%) | 1043 (25.7%) | |
Q3 | 538 (15.9%) | 155 (23.2%) | 693 (17.1%) | |
Q4 | 256 (7.6%) | 284 (42.5%) | 540 (13.3%) | |
% of employed men in protective service occupations: firefighting, prevention, and law enforcement (PCT_C2401002) | < 0.001 a | |||
Mean (sd) | 0.90 (1.2) | 1.5 (1.8) | 1.0 (1.4) | |
% Household income by value of owner-occupied housing units: both income and value $10,000 to $19,999 (PCT_B25121019) | < 0.001 a | |||
Mean (SD) | 0.14 (0.54) | 0.56 (1.35) | 0.21 (0.75) | |
% Workers taking public transport: streetcar or trolley car (PCT_B08301012) | < 0.001 a | |||
Mean | 0.05 (0.35) | 0.38 (1.27) | 0.11 (0.62) | |
Living in a high risk cluster for high-grade prostate cancer | < 0.001 b | |||
Yes | 117 (3.5%) | 129 (19.3%) | 246 (6.1%) | |
No | 3273 (96.5%) | 539 (80.7%) | 3812 (93.9%) |
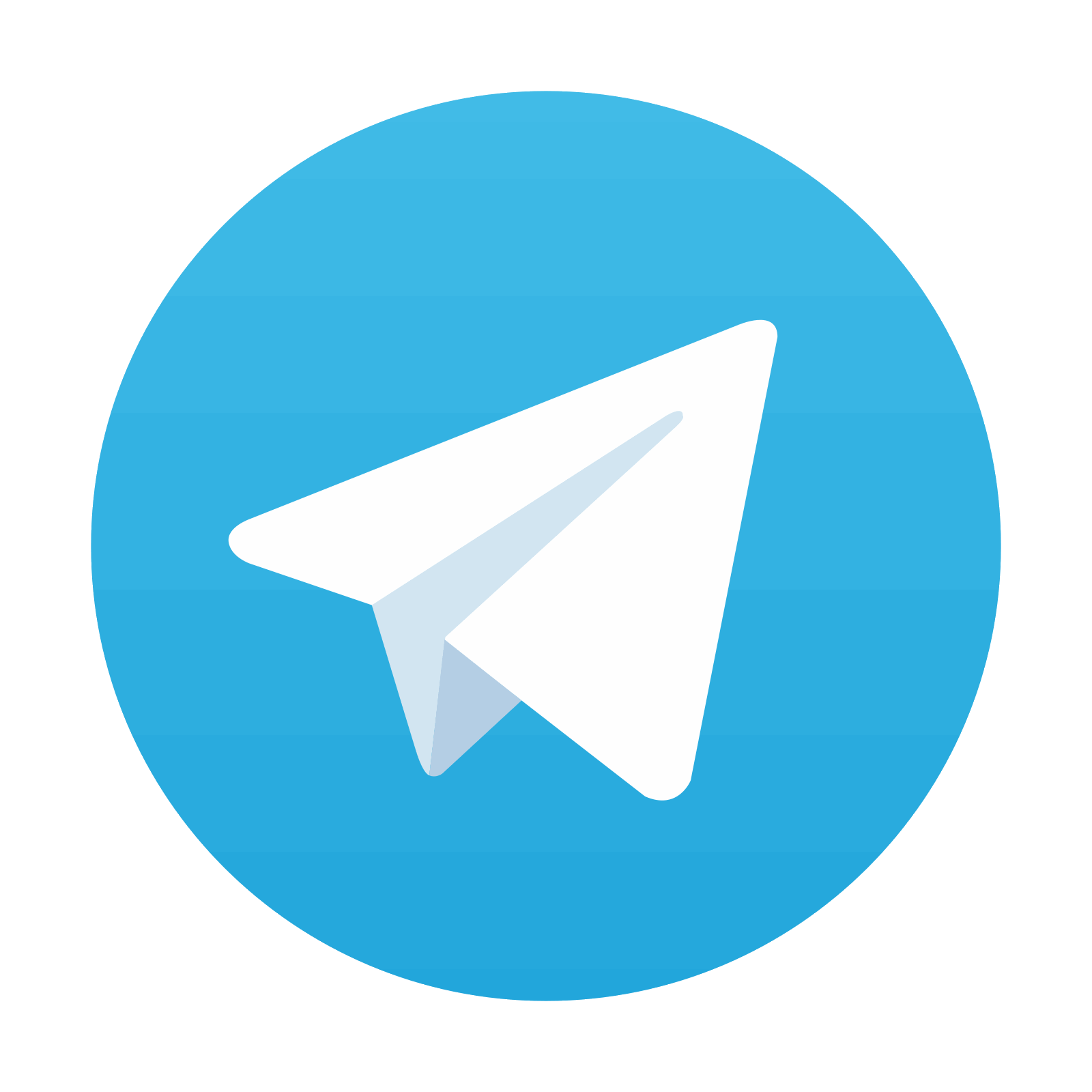
Stay updated, free articles. Join our Telegram channel

Full access? Get Clinical Tree
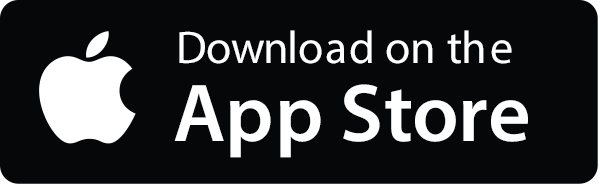

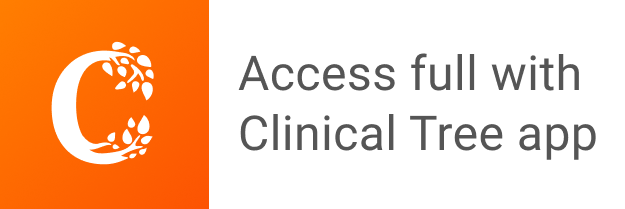